Coexpression and expression quantitative trait loci analyses of the angiogenesis gene-gene interaction network in prostate cancer
Introduction
Prostate cancer (PCa) accounted for 26% of cancer incidence and 9% of cancer deaths in American men in 2015 (1). PCa is a clinically heterogeneous disease but its risk classification is insufficient. About 30% of men are classified as a low risk group, but these men developed to high-grade cancer (2). Due to this inadequate classification, it is difficult for physicians to select a suitable treatment plan for PCa patients. Thus, understanding PCa etiology mechanisms and identifying biomarkers for improving prediction accuracy of PCa aggressiveness is essential to treating patients.
Angiogenesis plays an important role in prostate tumor growth and development. Angiogenesis involves the division and migration of endothelial cells and leads to microvasculature formation (3,4). Based on the genome-wide association studies (GWAS), angiogenesis genetic variants or single nucleotide polymorphisms (SNPs) in or near IL-16 (rs4072111) and FGFR2 (rs11199874, rs10749408 and rs10788165) are significantly associated with PCa aggressiveness (5,6). In candidate gene studies, several angiogenesis SNPs are shown to be associated with PCa prognosis. The pro-angiogenic genes include VEGFs, FGF, EGF, HIF, TGF-β and TNF-α; the anti-angiogenic genes include endostatin, IFN, MMPs, and ILs; and pro-/anti-angiogenic genes include MMPs and ILs (7). The functional mechanism of the majority of these genetic variants remain unclear.
It has often been shown that single gene effects are not sufficient to explain the complicated relationships among genes (8-11). Our previous study suggested an angiogenesis gene-gene interaction network associated with PCa aggressiveness (12). This network was built upon the SNP-SNP interactions of five gene pairs (MMP16-ROBO1, MMP16-CSF1, FBLN5-CSF1, CSF1-HSPG2, and MMP16-EGFR) and published protein-protein interactions. There are 12 genes identified (FBLN5, ROBO1, E2F1, STAT1, HSPG2, MMP16, ITGB3, ESR1, EGFR, CSF1, SP1, and AP1) in this network.
Among these angiogenesis genes, a majority of them were shown to be associated with PCa risk or prognosis. A key biological role of FBLN5 in human cells is communication among cells and between cells to matrix. FBLN5 is consistently downregulated in prostate tumors in data from expression microarray and RT-PCR (13). A recent study suggested ROBO1 as a tumor suppressor in PCa (14). Several groups consistently reported an association between an overexpression of E2F1, a cell cycle-specific transcription factor, with progression of PCa, especially PCa metastasis (15-18). Patterson et al. (19) suggested that STAT1 expression affects the chemoresistant phenotype especially to docetaxel treatment. Therefore, STAT1 plays a key role in docetaxel resistance in PCa treatment. The HSPG2 is a five-domain proteoglycan that interacts with extracellular matrix components and cell-surface molecules. Expression of HSPG2 is associated with high Gleason scores (20), prostate tumor growth, and enhanced angiogenesis (21). Overexpression of HSPG2 is required for invasion of prostate tumors (22). MMP16 has been shown to be down-regulated in malignant prostate tissues (23). Xu et al. [2103] identified ITGB3 as one of the genes related to tumor metastasis in various PCa cell lines (24). Estrogen receptor 1 (ESR1) is a ligand-activated transcription factor with domains for binding to hormones and DNA. ESR1 is associated with PCa risk because it stimulates proliferation of prostate cells and deregulates apoptosis (25). The EGFR binds the epidermal growth factor (EGF) and has been shown to play an important role in regulating prostate cellular growth and function (26-28). Overexpression of SP1 was observed in several cancers, including PCa, and increased angiogenesis and decreased cancer cell death (29). AP1 activation is shown to be essential for inducing proliferation and anchorage independence in PCa cells (30).
This angiogenesis gene-gene interaction network is novel, but its functional mechanism is unclear. SNPs may impact the process of angiogenesis through influencing gene expressions. Gene expressions are involved in many important biological processes. It has been shown that 83% of genes are differentially expressed among individuals; evaluating gene expression variations may provide useful information for disease or other phenotype development (31). For identifying functional roles of genetic variants, using expression quantitative trait loci (eQTL) analyses to evaluate gene expressions as intermediate phenotypes has been applied to identify downstream genes (32,33). In addition to evaluating each gene individually, it has been shown that coexpressions can be used to demonstrate that these genes have a functional relationship, such as physical interaction between the encoded proteins. Evaluating coexpressions can also be used to identify a group of interactive genes (34). Therefore, the goal of this study is to evaluate functional mechanisms of this reported angiogenesis gene-gene interaction network through performing coexpression and eQTL analyses.
Methods
Gene and SNP selection
The 11 candidate genes (FBLN5, ROBO1, E2F1, STAT1, HSPG2, MMP16, ITGB3, ESR1, EGFR, CSF1, and SP1) were selected based on the reported angiogenesis gene-gene interaction network associated with PCa aggressiveness (12). The AP1 was not included in the analysis due to lack of availability in the TCGA data. A total of 434 SNPs in these 11 angiogenesis genes was evaluated.
The Cancer Genome Atlas (TCGA) study population and genetic data
The TCGA SNP and gene expression data were used in this study. TCGA is a large-scale study led by the National Institutes of Health (NIH) to map the genomic changes that occur in more than 30 human cancer types. Its goal is to support new discoveries and accelerate research aimed at improving the prevention, diagnosis, and treatment of cancer (35). The eQTL and gene coexpression analyses were performed for Caucasians in this study due to small sample sizes of other race groups. Among 438 PCa patients recruited between 2009 and 2013 in the TCGA study, we applied the program LAMP-LD (36) to estimate ancestry based on the HapMap (37) data for the two major populations (Caucasian and African American). The ancestry test was performed using 347,481 SNP data from the blood samples. Caucasians were defined as European and those with greater than 80 percent European ancestry (38,39). The genotype call rate for our candidate SNPs was >95%. Among 411 PCa patients with both valid genotype and gene expression data, 350 patients were defined as Caucasian. RNA profiling was performed using Illumina HiSeq 2000 RNA Sequencing. The normalized RNAseq level 3 expression data were used in our analyses. The details are listed on the TCGA website (http://cancergenome.nih.gov).
Statistical analyses
All possible pairwise coexpressions among the candidate genes were evaluated using the Spearman coefficient (r). To our knowledge, there is no well-defined cutoff to define a strong coexpression. To identify a meaningful coexpression cutoff, we generated a null distribution of coexpressions from a set of 10 randomly selected genes with all 20,503 genes in the testing array. In this null distribution for coexpression (Figure 1), there were only 0.12% gene pairs with r≥0.6. Thus, we used a conservative cutoff of r=0.6 to define strong coexpressions as those with a r≥0.6.
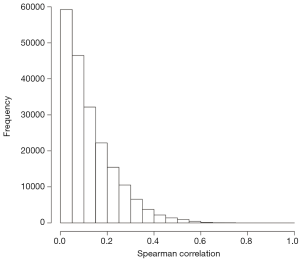
The eQTL analyses were applied to evaluate associations between one SNP and one gene expression. There are two types of eQTLs. Cis-eQTLs are eQTLs that map the approximate location of their gene-of-origin gene. In contrast, trans-eQTLs are those that map far from the location of their gene-of-origin gene. We performed a total of 4,774 (=11 gene expression × 434 SNPs) pairwise eQTL analyses, including both cis- (locally) and trans- (at a distance) eQTL analyses. The difference of gene expressions among the three genotypes (homozygous wild, heterozygous and homozygous variant types) was evaluated using the Kruskal-Wallis test. Adjusting for multiple comparisons, the false discovery rate (FDR) q value (40) was applied. We declared significance by using the FDR q value less than 20% as the cutoff. Linkage disequilibrium (LD), correlations between SNPs, was evaluated using r2. The strong LD was defined as r2>0.8.
Results
The participants’ demographic and clinical characteristics are summarized in Table 1. All 55 possible pairwise coexpressions among the 11 genes were evaluated using the Spearman correlations (Table 2). The ITGB3 and CSF1 are co-expressed with several genes in this angiogenesis network. Among them, 19 pairs (34.5%) had a correlation ≥0.5 and nine gene pairs (16.4%) had a strong coexpression (r≥0.6). These top co-expressed gene pairs are EGFR-SP1 (r=0.73), ITGB3- HSPG2 (r=0.71), ITGB3- CSF1 (r=0.70), MMP16-FBLN5 (r=0.68), ITGB3-MMP16 (r=0.65), ITGB3-ROBO1 (r=0.62), CSF1-HSPG2 (r=0.61), CSF1-FBLN5 (r=0.6), and CSF1-ROBO1 (r=0.60). All these strong coexpressions have a P value <1×10−13.
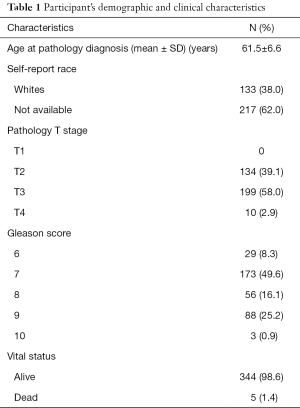
Full table
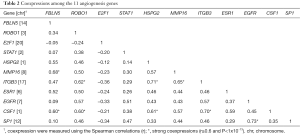
Full table
Among the 4,774 eQTL tests, there were 21 significant tests with a FDR q value<0.2 (Table 3). These associations were found in a total of five gene pairs (MMP16-ESR1, ESR1-ROBO1, CSF1-ROBO1, HSPG2-ROBO1, and FBLN5-CSF1) and one SNP-expression pair within the same gene (MMP16, cis-eQTL). The most significant test is the association between rs2982705 in ESR1 and MMP16 expression (raw P=7.5×10−5, FDR q=0.12). rs162268 in ROBO1 is significantly associated with ESR1 expression (raw P=1.1×10−4, FDR q=0.12). rs6788511 in ROBO1 is associated with CSF1 gene expression (raw P=1.3×10−4, FDR q=0.12). The box plots of the top six eQTL tests are shown in Figure S1. In the top eQTL list, several SNPs in the same gene were shown. We further evaluated LD among these SNPs in the same gene. The LD plots are listed in Figure S2. The majority of SNPs in the same gene have strong LD (r2>0.8).
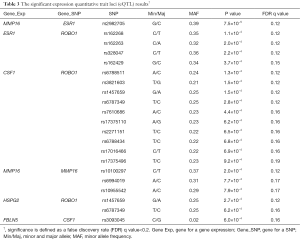
Full table
The results of coexpression and eQTL analyses are summarized in Figure 2 and Table 4. Our findings demonstrate 11 gene-gene interactions in the angiogenesis network. Among the previous SNP-SNP interactions in the five gene pairs (MMP16-ROBO1, MMP16-CSF1, FBLN5-CSF1, CSF1-HSPG2, and MMP16-EGFR), two of them (FBLN5-CSF1 and CSF1-HSPG2) are supported by direct coexpression and/or eQTL results. The interaction between FBLN5 and CSF1 was observed in both coexpression (r=0.6) and eQTL analyses (rs3093045 in CSF1 associated with the FBLN5 expressions, P=6.0×10−4). The interaction between CSF1 and HSPG2 was supported by the strong coexpression (r=0.61). For three other gene pairs (MMP16-ROBO1, MMP16-CSF1, and MMP16-EGFR), no direct gene interactions were observed but they may have interacted through another gene. As shown in Table 4, our findings suggest that the interaction of MMP16 and ROBO1 may be through ESR1 or ITGB3. For MMP16 and CSF1, they may be interacted through FBLN5 or ITGB3, while the crosstalk of MMP16 and EGFR may be through ITGB3.
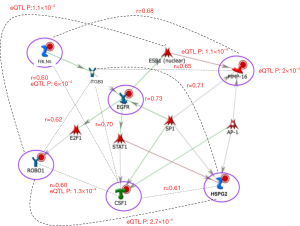
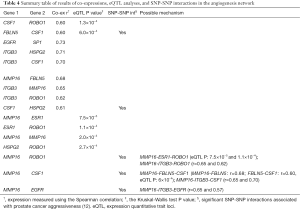
Full table
Conclusions
Our findings reveal potential functional mechanisms for the angiogenesis gene-gene interaction network, which has a reported association with PCa aggressiveness (12). This study successfully provides direct and indirect functional evidence of these gene-gene interactions. Among the five gene pairs (MMP16-ROBO1, MMP16-CSF1, FBLN5-CSF1, CSF1-HSPG2, and MMP16-EGFR) with SNP-SNP interactions (12), two of them (FBLN5-CSF1, CSF1-HSPG2) are supported by direct coexpression and/or eQTL results; the other three gene pairs may interact indirectly through another gene in this network.
Among 55 possible gene pairs in the angiogenesis network, 12 gene pairs (21.8%) have strong coexpressions or significant eQTL (or SNP-expression) results. Among them, the interactions of two gene pairs (CSF1-ROBO1 and FBLN5-CSF1) are supported by both coexpressions and eQTL results in this study. The relationships between these genes are understudied, although these genes individually are shown to associate with PCa risk and progression. Among the coexpression gene pairs identified, CSF1 and ITGB3 were involved in multiple coexpression pairs, thus a network correlation was implied. To assess a potential biological relevance of these genes and explore the underlying functional mechanism, we updated our genetic regulatory network, which were reported previously (41) (Figure 2). The interconnectedness of biochemical process, coexpression and eQTL networks of the identified genes showed that the six proteins were involved directly or indirectly in the EGFR signaling pathway. This network suggested that these genes are regulated by multiple proteins like receptors and transcription factors. The most prominent protein in the network is CSF1 which was directly co-expressed or interacted with the four angiogenesis proteins (Table 4, FBLN5, ROBO1, HSPG2, and ITGB3). Indeed the CSF1-ITGB3 pair showed a strong coexpression (r=0.7). Although studies of crosstalk between ITGB3 and CSF1 in PCa are limited, both CSF1 and ITGB3 were included in a gene signature of vitamin D exposure in breast cancer (42).
Potential functional role, relation to angiogenesis, and relation to prostate etiology of the 11 candidate genes are briefly summarized in Table 5. The exact role of colony stimulating factor-1 (CSF1) in PCa is not fully established yet. However, previous studies on other cancers, such as that of breast, ovary and endometrial tissues, reported that an overexpression of CSF1 was observed in cancer patients (69,70), increased tumor angiogenesis (69), promoted metastatic potential in breast cancer (64) and was associated with poor outcome in ovarian cancer (65). These biological roles of CSF1 were also confirmed in a murine pancreatic cancer study (41). Recently in their preclinical study, Garcia et al. [2014] demonstrated that CSF1 can be a potential target for PCa treatment (66).
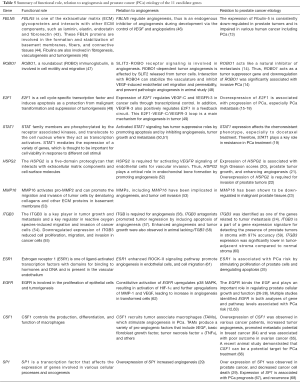
Full table
Integrins play important roles in signal transduction and are known to be involved in carcinogenesis, including colorectal (54), lung (71), and prostate cancers (59). The ITGB3 is a key player in tumor growth and metastasis and a key regulator in reactive oxygen species-induced migration and invasion of cancer cells (54). Ni et al. reported that downregulated expression of ITGB3 reduced cell proliferation, migration, and invasion in cancer cells (55). For PCa, ITGB3 is a part of a gene expression signature for detecting the presence of prostate tumors in stroma with 97% accuracy (59). ITGB3 expression was significantly lower in tumor-adjacent stroma compared to normal stroma (60). These results were consistent in the immunohistochemistry assays: ITGB3 protein showed lower protein expression in tumor-adjacent stroma compared to the normal stroma.
Among 12 interacted gene pairs, only EGFR-SP1 interaction was previously reported. The epidermal growth factor receptor (EGFR) is involved in the proliferation of epithelial cells and tumorigenesis. Our previous study and a meta-analysis study identified EGFR in both analyses of gene and pathway levels associated with PCa risk (12,63). Specificity protein 1 (Sp1) is a transcription factor that affects the expression of genes involved in various cellular processes and oncogenesis. Further, expression of Sp1 is associated with the prognosis of patients (67) and can contribute to predicted PCa recurrence (68). Among two SNPs in the promoter region of EGFR, -216G/T was located in a Sp1 recognition site. Transient transfection assay showed significantly increased promoter activity in the -216G allele as compared with the -216T allele. These findings were confirmed in an additional transient transfection assay in the Sp1-deficient cell line and electrophoretic mobility shift assay, which confirmed a significantly higher binding efficiency of Sp1 protein to the T allele compared with the G allele (72). Liu et al. demonstrated that -216 SNP in the EGFR promoter was associated with altered promoter activity by Sp1 binding and gene expression both in vitro and in vivo (72). SP1 has been shown to directly or indirectly regulate some PCa related genes, of which EGFR is one (73).
ROBO1, a roundabout (ROBO) immunoglobulin, is involved in the cell motility and migration (47). Khusial et al. [2010] reported that expression of ROBO1 affect process of motility in cancer cells (74). Parray et al. demonstrated that ROBO1 acts as a tumor suppressor gene and downregulation of ROBO1 was significantly associated with invasive PCa. Further, the study suggested that ROBO1 is a promising biomarker to differentiate metastatic cases from early stage cases. ROBO1 acts like a natural inhibitor of metastasis; therefore, this protein provides an opportunity to develop novel therapies targeting ROBO1 for treating metastatic PCa (14). Regarding Fibulin 5 (FBLN5), it is one of the extracellular matrix (ECM) glycoproteins and interacts with many ECM components, such as laminin, elastin, endostatin, and fibronectin (43). These FBLN proteins are involved in the formation and stabilization of basement membranes, fibers, and connective tissues (44). Furthermore, fibulins are involved in fibrogenesis, vasculogenesis, and tumorigenesis (45). The expression of Fibulin-5 is impaired in various human cancer tissues including PCa (13). These expression data were confirmed by immunohistochemistry results. The study also reported that Fibulin-5 was predominantly located in the stroma, (with a strong gradient from the periurethral to the peripheral zone) and lost in prostate tumors.
This study evaluated both cis- and trans-eQTLs in the angiogenesis network. Among the six primary gene pairs of eQTL results, only one set of MMP16 contains cis-eQTLs. Five other sets are gene pairs that interacted at a distance. Our study findings demonstrate that the gene-gene interaction network, conducted based on the SNP-SNP interaction and bioinformatics approach, can be an effective tool for identifying potential trans-genes, which is beneficial for understanding the etiology of PCa prognosis. Another large-scale eQTL study also has demonstrated that some disease-associated SNPs affect multiple genes in trans-eQTLs, which are known to be changed for individuals with diseases, and these results have been successfully replicated (75).
The strength of this study is that it defines strong coexpressions (r≥0.6) based on the empirically null distribution of coexpressions in the same testing dataset. To our knowledge, there is no standard cutoff to define strong coexpressions. Using these conservative criteria, we still can identify nine strong coexpressions. The confirmed coexpressions, which are validated in several datasets, can be used to identify gene clusters with functional interactions. A study examined mRNA coexpressions in 60 human datasets and identified 8,805 gene coexpressions in at least three datasets (34). The clinical use of coexpressions is also promising. It has been shown that coexpression provides a better prediction and classification than single-gene expression in PCa progression even after controlling for clinical variables (76).
These findings provide functional evidence to support the association among genes in the angiogenesis network. The conventional prediction model only considers an additive effect of individual biomarkers. Several studies show that interactions of multiple biomarkers are more powerful than individual biomarkers (12,76-78). The integrated biomarkers (such as SNP-SNP interaction, coexpression, or eQTL results) of these genes may be useful for building a risk prediction model for PCa aggressiveness. Future large scale gene expression, eQTL, protein expression studies, or functional experiments are warranted to further validate the interactions of angiogenesis genes.
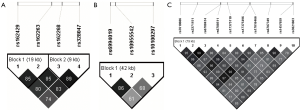
Acknowledgments
We thank our anonymous reviewers for their valuable comments on this manuscript, which have led to much many improvements to the article.
Funding: This study was supported by the National Cancer Institute (R01CA128813, PI: JY Park and R21CA202417, PI: HY Lin).
Footnote
Provenance and Peer Review: This article was commissioned by the editorial office, Translational Cancer Research for the series “Social Behavioral and Genetic Risk factors for Cancer”. The article has undergone external peer review.
Conflicts of Interest: All authors have completed the ICMJE uniform disclosure form (available at http://dx.doi.org/10.21037/tcr.2016.10.55). The series “Social Behavioral and Genetic Risk factors for Cancer” was commissioned by the editorial office without any funding or sponsorship. DTC served as the unpaid Guest Editor of the series and serves as an unpaid editorial board member of Translational Cancer Research. HYL served as the unpaid Guest Editor of the series. The authors have no other conflicts of interest to declare.
Ethical Statement: The authors are accountable for all aspects of the work in ensuring that questions related to the accuracy or integrity of any part of the work are appropriately investigated and resolved. The study was conducted in accordance with the Declaration of Helsinki (as revised in 2013).
Open Access Statement: This is an Open Access article distributed in accordance with the Creative Commons Attribution-NonCommercial-NoDerivs 4.0 International License (CC BY-NC-ND 4.0), which permits the non-commercial replication and distribution of the article with the strict proviso that no changes or edits are made and the original work is properly cited (including links to both the formal publication through the relevant DOI and the license). See: https://creativecommons.org/licenses/by-nc-nd/4.0/.
References
- Siegel RL, Miller KD, Jemal A. Cancer statistics, 2016. CA Cancer J Clin 2016;66:7-30. [Crossref] [PubMed]
- Hutchinson L. Closing the controversies gap in prostate cancer? Nat Rev Clin Oncol 2014;11:299. [Crossref] [PubMed]
- Weis SM, Cheresh DA. Tumor angiogenesis: molecular pathways and therapeutic targets. Nat Med 2011;17:1359-70. [Crossref] [PubMed]
- Carmeliet P, Jain RK. Molecular mechanisms and clinical applications of angiogenesis. Nature 2011;473:298-307. [Crossref] [PubMed]
- Thomas G, Jacobs KB, Yeager M, et al. Multiple loci identified in a genome-wide association study of prostate cancer. Nat Genet 2008;40:310-5. [Crossref] [PubMed]
- Nam RK, Zhang W, Siminovitch K, et al. New variants at 10q26 and 15q21 are associated with aggressive prostate cancer in a genome-wide association study from a prostate biopsy screening cohort. Cancer Biol Ther 2011;12:997-1004. [Crossref] [PubMed]
- Amankwah EK, Sellers TA, Park JY. Gene variants in the angiogenesis pathway and prostate cancer. Carcinogenesis 2012;33:1259-69. [Crossref] [PubMed]
- Cordell HJ. Detecting gene-gene interactions that underlie human diseases. Nat Rev Genet 2009;10:392-404. [Crossref] [PubMed]
- Moore JH, Williams SM. New strategies for identifying gene-gene interactions in hypertension. Annals of medicine 2002;34:88-95. [Crossref] [PubMed]
- Moore JH. The ubiquitous nature of epistasis in determining susceptibility to common human diseases. Hum Hered 2003;56:73-82. [Crossref] [PubMed]
- Onay VU, Briollais L, Knight JA, et al. SNP-SNP interactions in breast cancer susceptibility. BMC Cancer 2006;6:114. [Crossref] [PubMed]
- Lin HY, Amankwah EK, Tseng TS, et al. SNP-SNP interaction network in angiogenesis genes associated with prostate cancer aggressiveness. PLoS One 2013;8:e59688 [Crossref] [PubMed]
- Wlazlinski A, Engers R, Hoffmann MJ, et al. Downregulation of several fibulin genes in prostate cancer. Prostate 2007;67:1770-80. [Crossref] [PubMed]
- Parray A, Siddique HR, Kuriger JK, et al. ROBO1, a tumor suppressor and critical molecular barrier for localized tumor cells to acquire invasive phenotype: study in African-American and Caucasian prostate cancer models. Int J Cancer 2014;135:2493-506. [Crossref] [PubMed]
- Ren Z, Kang W, Wang L, et al. E2F1 renders prostate cancer cell resistant to ICAM-1 mediated antitumor immunity by NF-kappaB modulation. Mol Cancer 2014;13:84. [Crossref] [PubMed]
- Davis JN, Wojno KJ, Daignault S, et al. Elevated E2F1 inhibits transcription of the androgen receptor in metastatic hormone-resistant prostate cancer. Cancer Res 2006;66:11897-906. [Crossref] [PubMed]
- Zheng C, Ren Z, Wang H, et al. E2F1 Induces tumor cell survival via nuclear factor-kappaB-dependent induction of EGR1 transcription in prostate cancer cells. Cancer Res 2009;69:2324-31. [Crossref] [PubMed]
- Libertini SJ, Tepper CG, Guadalupe M, et al. E2F1 expression in LNCaP prostate cancer cells deregulates androgen dependent growth, suppresses differentiation, and enhances apoptosis. Prostate 2006;66:70-81. [Crossref] [PubMed]
- Patterson SG, Wei S, Chen X, et al. Novel role of Stat1 in the development of docetaxel resistance in prostate tumor cells. Oncogene 2006;25:6113-22. [Crossref] [PubMed]
- Datta MW, Hernandez AM, Schlicht MJ, et al. Perlecan, a candidate gene for the CAPB locus, regulates prostate cancer cell growth via the Sonic Hedgehog pathway. Mol Cancer 2006;5:9. [Crossref] [PubMed]
- Savorè C, Zhang C, Muir C, et al. Perlecan knockdown in metastatic prostate cancer cells reduces heparin-binding growth factor responses in vitro and tumor growth in vivo. Clin Exp Metastasis 2005;22:377-90. [Crossref] [PubMed]
- Warren CR, Grindel BJ, Francis L, et al. Transcriptional activation by NFkappaB increases perlecan/HSPG2 expression in the desmoplastic prostate tumor microenvironment. J Cell Biochem 2014;115:1322-33. [Crossref] [PubMed]
- Jung M, Romer A, Keyszer G, et al. mRNA expression of the five membrane-type matrix metalloproteinases MT1-MT5 in human prostatic cell lines and their down-regulation in human malignant prostatic tissue. Prostate 2003;55:89-98. [Crossref] [PubMed]
- Xu L, Wang Z, Li XF, et al. Screening and identification of significant genes related to tumor metastasis and PSMA in prostate cancer using microarray analysis. Oncol Rep 2013;30:1920-8. [PubMed]
- Langley RE, Godsland IF, Kynaston H, et al. Early hormonal data from a multicentre phase II trial using transdermal oestrogen patches as first-line hormonal therapy in patients with locally advanced or metastatic prostate cancer. BJU Int 2008;102:442-5. [Crossref] [PubMed]
- Migliaccio A, Castoria G, Di Domenico M, et al. Crosstalk between EGFR and extranuclear steroid receptors. Ann N Y Acad Sci 2006;1089:194-200. [Crossref] [PubMed]
- Bonaccorsi L, Nosi D, Muratori M, et al. Altered endocytosis of epidermal growth factor receptor in androgen receptor positive prostate cancer cell lines. J Mol Endocrinol 2007;38:51-66. [Crossref] [PubMed]
- Léotoing L, Manin M, Monté D, et al. Crosstalk between androgen receptor and epidermal growth factor receptor-signalling pathways: a molecular switch for epithelial cell differentiation. J Mol Endocrinol 2007;39:151-62. [Crossref] [PubMed]
- Li L, Davie JR. The role of Sp1 and Sp3 in normal and cancer cell biology. Ann Anat 2010;192:275-83. [Crossref] [PubMed]
- Parra E, Ferreira J, Ortega A. Overexpression of EGR-1 modulates the activity of NF-kappaB and AP-1 in prostate carcinoma PC-3 and LNCaP cell lines. Int J Oncol 2011;39:345-52. [PubMed]
- Storey JD, Madeoy J, Strout JL, et al. Gene-expression variation within and among human populations. Am J Hum Genet 2007;80:502-9. [Crossref] [PubMed]
- Matsunami K, Miyagawa S, Nakai R, et al. Modulation of the leader peptide sequence of the HLA-E gene up-regulates its expression and down-regulates natural killer cell-mediated swine endothelial cell lysis. Transplantation 2002;73:1582-9. [Crossref] [PubMed]
- Fehrmann RS, Jansen RC, Veldink JH, et al. Trans-eQTLs reveal that independent genetic variants associated with a complex phenotype converge on intermediate genes, with a major role for the HLA. PLoS Genet 2011;7:e1002197 [Crossref] [PubMed]
- Lee HK, Hsu AK, Sajdak J, et al. Coexpression analysis of human genes across many microarray data sets. Genome Res 2004;14:1085-94. [Crossref] [PubMed]
- The Cancer Genome Atlas Data: Navigating the Data Portal and the Cancer Genomics Hub. National Cancer Institute/National Human Genome Reserasch Institute; 2014.
- Baran Y, Pasaniuc B, Sankararaman S, et al. Fast and accurate inference of local ancestry in Latino populations. Bioinformatics 2012;28:1359-67. [Crossref] [PubMed]
- International HapMap C. The International HapMap Project. Nature 2003;426:789-96. [Crossref] [PubMed]
- Permuth-Wey J, Kim D, Tsai YY, et al. LIN28B polymorphisms influence susceptibility to epithelial ovarian cancer. Cancer Research 2011;71:3896-903. [Crossref] [PubMed]
- Permuth-Wey J, Lawrenson K, Shen HC, et al. Identification and molecular characterization of a new ovarian cancer susceptibility locus at 17q21.31. Nat Commun 2013;4:1627. [Crossref] [PubMed]
- Storey JD. A direct approach to false discovery rates. J R Stat Soc Series B Stat Methodol 2002;64:479-98. [Crossref]
- Pyonteck SM, Gadea BB, Wang HW, et al. Deficiency of the macrophage growth factor CSF-1 disrupts pancreatic neuroendocrine tumor development. Oncogene 2012;31:1459-67. [Crossref] [PubMed]
- Simmons KM, Beaudin SG, Narvaez CJ, et al. Gene Signatures of 1,25-Dihydroxyvitamin D3 Exposure in Normal and Transformed Mammary Cells. J Cell Biochem 2015;116:1693-711. [Crossref] [PubMed]
- Papke CL, Yanagisawa H. Fibulin-4 and fibulin-5 in elastogenesis and beyond: Insights from mouse and human studies. Matrix Biol 2014;37:142-9. [Crossref] [PubMed]
- Timpl R, Sasaki T, Kostka G, et al. Fibulins: a versatile family of extracellular matrix proteins. Nat Rev Mol Cell Biol 2003;4:479-89. [Crossref] [PubMed]
- de Vega S, Iwamoto T, Yamada Y. Fibulins: multiple roles in matrix structures and tissue functions. Cell Mol Life Sci 2009;66:1890-902. [Crossref] [PubMed]
- Sullivan KM, Bissonnette R, Yanagisawa H, et al. Fibulin-5 functions as an endogenous angiogenesis inhibitor. Lab Invest 2007;87:818-27. [Crossref] [PubMed]
- Gonda Y, Andrews WD, Tabata H, et al. Robo1 regulates the migration and laminar distribution of upper-layer pyramidal neurons of the cerebral cortex. Cereb Cortex 2013;23:1495-508. [Crossref] [PubMed]
- Chen CY, Tsai CH, Chen CY, et al. Human placental multipotent mesenchymal stromal cells modulate placenta angiogenesis through Slit2-Robo signaling. Cell Adh Migr 2016;10:66-76. [Crossref] [PubMed]
- Engelmann D, Mayoli-Nussle D, Mayrhofer C, et al. E2F1 promotes angiogenesis through the VEGF-C/VEGFR-3 axis in a feedback loop for cooperative induction of PDGF-B. J Mol Cell Biol 2013;5:391-403. [Crossref] [PubMed]
- Stephanou A, Latchman DS. STAT-1: a novel regulator of apoptosis. Int J Exp Pathol 2003;84:239-44. [Crossref] [PubMed]
- Huang S, Bucana CD, Van Arsdall M, et al. Stat1 negatively regulates angiogenesis, tumorigenicity and metastasis of tumor cells. Oncogene 2002;21:2504-12. [Crossref] [PubMed]
- Ishijima M, Suzuki N, Hozumi K, et al. Perlecan modulates VEGF signaling and is essential for vascularization in endochondral bone formation. Matrix Biol 2012;31:234-45. [Crossref] [PubMed]
- Cao L, Chen C, Zhu H, et al. MMP16 is a marker of poor prognosis in gastric cancer promoting proliferation and invasion. Oncotarget 2016; [Epub ahead of print]. [PubMed]
- Lei Y, Huang K, Gao C, et al. Proteomics identification of ITGB3 as a key regulator in reactive oxygen species-induced migration and invasion of colorectal cancer cells. Mol Cell Proteomics 2011;10:M110.005397.
- Ni R, Huang Y, Wang J. miR-98 targets ITGB3 to inhibit proliferation, migration, and invasion of non-small-cell lung cancer. Onco Targets Ther 2015;8:2689-97. [PubMed]
- Brooks PC, Clark RA, Cheresh DA. Requirement of vascular integrin alpha v beta 3 for angiogenesis. Science 1994;264:569-71. [Crossref] [PubMed]
- Brooks PC, Montgomery AM, Rosenfeld M, et al. Integrin alpha v beta 3 antagonists promote tumor regression by inducing apoptosis of angiogenic blood vessels. Cell 1994;79:1157-64. [Crossref] [PubMed]
- Reynolds LE, Wyder L, Lively JC, et al. Enhanced pathological angiogenesis in mice lacking beta3 integrin or beta3 and beta5 integrins. Nat Med 2002;8:27-34. [Crossref] [PubMed]
- Jia Z, Wang Y, Sawyers A, et al. Diagnosis of prostate cancer using differentially expressed genes in stroma. Cancer Res 2011;71:2476-87. [Crossref] [PubMed]
- Zhu J, Pan C, Jiang J, et al. Six stroma-based RNA markers diagnostic for prostate cancer in European-Americans validated at the RNA and protein levels in patients in China. Oncotarget 2015;6:16757-65. [Crossref] [PubMed]
- Li S, Dang Y, Zhou X, et al. Formononetin promotes angiogenesis through the estrogen receptor alpha-enhanced ROCK pathway. Sci Rep 2015;5:16815. [Crossref] [PubMed]
- Kim D, Dai J, Park YH, et al. Activation of Epidermal Growth Factor Receptor/p38/Hypoxia-inducible Factor-1α Is Pivotal for Angiogenesis and Tumorigenesis of Malignantly Transformed Cells Induced by Hexavalent Chromium. J Biol Chem 2016;291:16271-81. [Crossref] [PubMed]
- Wang Y, Chen J, Li Q, et al. Identifying novel prostate cancer associated pathways based on integrative microarray data analysis. Comput Biol Chem 2011;35:151-8. [Crossref] [PubMed]
- Lin EY, Nguyen AV, Russell RG, et al. Colony-stimulating factor 1 promotes progression of mammary tumors to malignancy. J Exp Med 2001;193:727-40. [Crossref] [PubMed]
- Chambers SK, Kacinski BM, Ivins CM, et al. Overexpression of epithelial macrophage colony-stimulating factor (CSF-1) and CSF-1 receptor: a poor prognostic factor in epithelial ovarian cancer, contrasted with a protective effect of stromal CSF-1. Clin Cancer Res 1997;3:999-1007. [PubMed]
- Garcia AJ, Ruscetti M, Arenzana TL, et al. Pten null prostate epithelium promotes localized myeloid-derived suppressor cell expansion and immune suppression during tumor initiation and progression. Mol Cell Biol 2014;34:2017-28. [Crossref] [PubMed]
- Sankpal UT, Goodison S, Abdelrahim M, et al. Targeting Sp1 transcription factors in prostate cancer therapy. Med Chem 2011;7:518-25. [Crossref] [PubMed]
- Bedolla RG, Gong J, Prihoda TJ, et al. Predictive value of Sp1/Sp3/FLIP signature for prostate cancer recurrence. PLoS One 2012;7:e44917 [Crossref] [PubMed]
- Espinosa I, Edris B, Lee CH, et al. CSF1 expression in nongynecological leiomyosarcoma is associated with increased tumor angiogenesis. Am J Pathol 2011;179:2100-7. [Crossref] [PubMed]
- Kacinski BM. CSF-1 and its receptor in breast carcinomas and neoplasms of the female reproductive tract. Mol Reprod Dev 1997;46:71-4. [Crossref] [PubMed]
- Zhao B, Han H, Chen J, et al. MicroRNA let-7c inhibits migration and invasion of human non-small cell lung cancer by targeting ITGB3 and MAP4K3. Cancer letters 2014;342:43-51. [Crossref] [PubMed]
- Liu W, Innocenti F, Wu MH, et al. A functional common polymorphism in a Sp1 recognition site of the epidermal growth factor receptor gene promoter. Cancer Res 2005;65:46-53. [PubMed]
- Li Y, Vongsangnak W, Chen L, et al. Integrative analysis reveals disease-associated genes and biomarkers for prostate cancer progression. BMC Med Genomics 2014;7:S3. [Crossref] [PubMed]
- Khusial PR, Vadla B, Krishnan H, et al. Src activates Abl to augment Robo1 expression in order to promote tumor cell migration. Oncotarget 2010;1:198-209. [Crossref] [PubMed]
- Westra HJ, Peters MJ, Esko T, et al. Systematic identification of trans eQTLs as putative drivers of known disease associations. Nat Genet 2013;45:1238-43. [Crossref] [PubMed]
- Zhao H, Logothetis CJ, Gorlov IP, et al. Modified logistic regression models using gene coexpression and clinical features to predict prostate cancer progression. Comput Math Methods Med 2013;2013:917502.
- Milne RL, Fagerholm R, Nevanlinna H, et al. The importance of replication in gene-gene interaction studies: multifactor dimensionality reduction applied to a two-stage breast cancer case-control study. Carcinogenesis 2008;29:1215-8. [Crossref] [PubMed]
- Lin HY, Chen YA, Tsai YY, et al. TRM: a powerful two-stage machine learning approach for identifying SNP-SNP interactions. Ann Hum Genet 2012;76:53-62. [Crossref] [PubMed]