Renal cell carcinoma: the search for a reliable biomarker
Introduction
Renal cell carcinoma (RCC) is the third most common genitourinary malignancy in the UA, with an estimated 63,990 new cases and 14,400 deaths expected in 2017 alone (1). A particular challenge in treating RCC is the heterogeneity of disease, as a renal mass may range from benign (e.g., oncocytoma) to clinically indolent [e.g., papillary type I, chromophobe RCC (chRCC)] to aggressive with a high potential for metastasis [e.g., papillary type II or high-grade clear cell renal cell carcinoma (ccRCC)] (2). Therefore, the ability to properly categorize and then appropriately treat a renal mass is of prime importance to urologists, interventional radiologists, and medical oncologists. Biomarkers offer a unique opportunity to improve the care of patients with RCC.
Biomarkers are broadly defined as objective, quantifiable characteristics of biological processes that measure a physiological state and may be used as surrogate endpoints to predict outcomes (3). Biomarkers may be classified based on their parameters, including diagnostic biomarkers (i.e., detection of a disease state), disease prognosis biomarkers, and predictive biomarkers (i.e., prediction of clinical response to a therapy). Currently, a wide array of biomarkers exist that may help guide individualized care of kidney cancer patients (4). This review will discuss the various serum, imaging, and immunohistological biomarkers available in current practice as well as future directions for the development of novel RCC biomarkers.
Imaging biomarkers
One of the primary goals of imaging biomarkers is to non-invasively identify the histology of a renal mass, resulting in treatment of aggressive molecular subtypes, while avoiding overtreatment of indolent tumor subtypes and benign renal masses (5). Moreover, imaging may help predict potential responses to treatment by identifying molecular features. Imaging biomarkers are one piece of the biomarker armamentarium and, along with serum/urine and immunohistological biomarkers, help us move towards the goal of precision oncology (4).
Molecular imaging
Positron emission tomography (PET) imaging techniques using various tracers have been studied as both prognostic and predictive biomarkers in RCC (6). Novel molecular imaging biomarkers take advantage of pathologic overexpression of cellular components specific for various RCC subtypes and may allow for non-invasive characterization of RCC subtypes and differentiation of benign from malignant renal masses (7). Further, PET imaging may be used to predict and monitor response to systemic targeted therapy (TT) in patients with advanced disease (7).
The most well studied radiotracer for RCC is 18F-fluoro-deoxy-glucose (FDG). Uptake of FDG on PET/CT is limited in localized RCC due to variable uptake in primary tumors and high background activity in normal parenchyma and radiotracer excretion in the urine; the reported sensitivity of FDG PET/CT for diagnosis of localized RCC is only 22% (7). As such, FDG PET/CT is not currently recommended for the diagnosis or staging of localized RCC. However, FDG PET/CT has shown some ability to predict survival in patients with advanced RCC. Nakaigawa et al. demonstrated in 101 patients with advanced RCC (metastatic or locoregional disease) that the standardized uptake value (SUV)max on FDG PET/CT prior to systemic therapy independently predicted overall survival (OS) (8). Similarly, median SUVmax on a baseline FDG PET/CT prior to systemic TT significantly predicted OS and progression free survival (PFS) in patients with advanced RCC (9).
There is also promising evidence that FDG PET/CT can be used to assess for response to TT in metastatic RCC (mRCC). Kayani et al. enrolled 44 patients with untreated mRCC in a prospective phase II trial and performed FDG PET/CT at baseline and after 4- and 16-week sunitinib therapy. At 16 weeks, but not 4 weeks, FDG PET/CT disease progression correlated with both PFS and OS (9). However, a recent review by Caldarella et al. concluded that the role of FDG PET/CT for evaluation of TT treatment efficacy is currently not well defined, with significant heterogeneity among the available data (10).
While the role of FDG PET/CT in predicting response to TT is uncertain, FDG PET/CT has shown excellent ability for the detection of metastases, especially in cases with equivocal findings on contrast-enhanced CT. For instance, Gofrit et al. systematically reviewed the literature regarding FDG PET/CT and found a 94% detection rate of metastases (versus 89% for CT alone), with 100% detection rate of bone metastases in particular (7). Certain subtypes of RCC, such as those that are dependent on aerobic glycolysis due to dysfunction in one of their Krebs cycle enzymes [e.g., hereditary leiomyomatosis and renal cell cancer (HLRCC)-associated papillary type II RCC or succinate dehydrogenase (SDH)-associated RCC], may be especially well suited to imaging with PET/CT (11).
One of the most recently explored imaging biomarkers is carbonic anhydrase IX (CA-IX), a protein that is overexpressed in von Hippel-Lindau (VHL)-mutated pathways such as ccRCC but low-expressed in normal renal parenchyma and non-ccRCC (12). Iodine-124 (124I)-cG250 is a monoclonal antibody that binds to CA-IX and serves as an imaging radiotracer (13). The primary study evaluating CA-IX as a biomarker was the REDECT trial, a multi-center, phase III trial of patients with renal masses imaged with 124I-cG250 PET prior to resection. Among the 195 patients, the average sensitivity was 86.2% and average specificity was 85.9% (12). This study was the first clinical validation of 124I-cG250 PET as a biomarker for RCC. In a critical assessment of the data from the REDECT trial, Farber et al. discussed that the main utility of molecular imaging techniques is in the workup of the small renal mass (SRM), yet the REDECT trial included renal masses up to 22 cm. In addition, when looking at a T1a subgroup analysis, a sensitivity of just 70.8% was determined for masses less than or equal to 2 cm without providing PPV, NPV or specificity (13).
The chief disadvantage of using the monoclonal antibody 124I-cG250 is that its half-life is several days, requiring prolonged waiting times after injection before tumor to background ratios are adequate. An alternative radiotracer is 18F-VM4-037, a molecule that also binds to the biomarker CA-IX but has a short half-life and consequently greater clinical applicability. In a phase II clinical trial, Turkbey et al. reported the results of utilizing 18F-VM4-037 PET/CT to detect ccRCC in 11 patients with kidney masses; localized tumors (n=9) were excised and metastatic lesions (n=2) were biopsied. The authors found that localized ccRCC tumors showed significant uptake of the 18F-VM4-037 radiotracer; however, there was a high level of background uptake in normal kidney parenchyma, which limited the contrast between tumor and normal parenchyma (14). Interestingly, metastatic lesions showed greater uptake than localized lesions, and were also free of any background uptake. These findings suggest a more limited use in localized RCC, though there is strong potential for accurate diagnosis of metastatic ccRCC. The main limitations to this study are the small sample size, especially with respect to evaluation of metastatic lesions, and the inclusion of only ccRCC histology.
Prostate-specific membrane antigen (PSMA)-targeted 18F-DCFPyL is another molecular imaging biomarker that may predict response in patients with mRCC. PSMA is a cell surface protein that is expressed in tumor neovasculature, making it a highly targetable biomarker for vascular tumors such as RCC. Rowe et al. examined the utility of PSMA-targeted 18F-DCFPyL PET/CT in the detection of metastatic disease in five patients with mRCC. The patients were imaged with both the novel tracer and conventional [i.e., contrast-enhanced CT or magnetic resonance imaging (MRI)] imaging. They found that 18F-DCFPyL PET/CT revealed 28 lesions suspicious for disease, compared to 18 lesions with conventional imaging, for a sensitivity of 94.7% vs. 78%, respectively (15). Of note, PSMA-targeted PET/CT was able to identify sub-centimeter lymph nodes and subtle bone lesions that were not detected with conventional imaging. Additionally, one case study of a patient with diffuse mRCC showed that 18F-DCFPyL PET/CT detected additional metastatic lesions that were not identified on FDG PET/CT (16). Based on the results of these small studies, PSMA-targeted 18F-DCFPyL PET/CT appears to be a very sensitive imaging technique for detecting mRCC at diverse anatomic sites such as bone, brain, lymph node, and soft tissue (15). The major limitations to this preliminary work include the lack of pathologic confirmation of disease at all the sites of tracer uptake and a small sample size. Larger studies are required to fully assess the role of PSMA-based PET imaging compared to other novel imaging biomarkers (e.g., 18F-VM4-037) in mRCC prognosis, detection, and response to systemic therapy.
An additional challenging diagnostic task is the differentiation of oncocytoma from chRCC or hybrid oncocytic/chromophobe tumors (HOCTs). Gorin et al. examined 50 patients with T1 renal masses imaged with 99mTc-MIBI SPECT/CT prior to surgical extirpation (17). They found that 99mTc-MIBI SPECT/CT correctly diagnosed 5/6 (83.3%) oncocytomas and 2/2 (100%) HOCTs with only two false positives, resulting in a sensitivity of 87.5% and specificity of 95.2%. Thus, this preliminary data suggests that 99mTc-MIBI SPECT/CT has an emerging role when evaluating patients with oncocytic neoplasms.
MRI
MRI may serve as an imaging biomarker by predicting tumor subtype and by assessing response to therapy. Perfusion MRI (pMRI) and diffusion MRI are the two primary techniques with which these aims have been studied (18).
pMRI assesses tissue perfusion at the microcapillary level and includes three methods: dynamic contrast enhanced (DCE), dynamic susceptibility contrast (DSC) and arterial spin labeling (ASL). The former two methods require intravenous contrast, while the latter does not. All three methods have been employed to characterize renal masses and assess tumor histology and grade.
Lanzman et al. prospectively evaluated 34 patients with renal masses and performed ASL pMRI prior to surgery. Using postoperative histopathology to establish the diagnosis, they found that ASL perfusion levels reliably allowed for differentiation of oncocytoma from RCC, and papillary RCC from other RCC subtypes (19). In addition to predictive ability regarding histology, some data suggests a role for ASL pMRI in the evaluation of response to systemic therapy in mRCC. The largest series was reported by de Bazelaire et al., who imaged patients with ASL at 1- and 4-month after tyrosine kinase inhibitor therapy and found that early tumor blood flow changes predicted clinical outcome (20). While the application of pMRI is promising in the management of RCC patients, the technical aspects and expertise required to consistently obtain high quality images remain obstacles to the routine implementation of pMRI in clinical practice (Figure 1) (18).
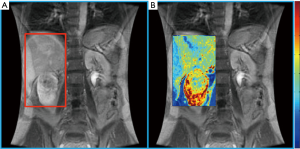
Diffusion MRI examines the tissue differences of water molecule motion and also provides the capability for tumor histology prediction. A systematic review and meta-analysis by Kang et al. of diffusion-weighted imaging (DWI) studies in the characterization of renal masses showed moderate accuracy for distinguishing between benign vs. malignant lesions (86% sensitivity and 78% specificity) and low- vs. high-grade ccRCC (AUC of 0.83) (21). They found insufficient evidence, however, for discrimination of ccRCC from other histologies.
Texture analysis and radiomics
Texture analysis is a method of automated image analysis that acquires a large array of imaging parameters to make quantitative decisions about defined tumor regions and predict tumor phenotype and biological behavior (13). The rationale is that a tumor’s genomic heterogeneity may be expressed phenotypically on imaging as intratumoral heterogeneity. For instance, Gaing et al. reported that texture analysis of DWI was able to differentiate 9 of 15 subtype pairs of renal tumors in patients with pathology-proven RCC (22).
Similarly, radiomics is predicated on the fact that different genomic alterations (GAs) within a tumor may manifest with different imaging features. Radiomics utilizes higher-order statistical models and bioinformatics tools to convert conventionally obtained medical images along with patient-specific data into higher-dimensional data models that may guide clinical decision making (23). The Cancer Genome Atlas-RCC imaging research group, for example, reported that one particular genomic mutation (BAP1) was associated with very specific imaging features (e.g., poorly defined tumor margins and calcifications), whereas a different genomic mutation (MUC4) was associated with separate imaging findings (exophytic tumor growth) (24). Overall, the utilization of radiomics and texture analysis are expected to make more of an impact as we learn how to more efficiently harness the large amounts of unexamined data generated with conventional imaging and combine it with the rapid proliferation of tumor sequencing from ongoing precision oncology initiatives.
Serum and urine biomarkers
With the rapid expansion of therapeutic options for mRCC, there is a need to prospectively select patients most likely to respond to a particular treatment. Cytokines and angiogenic factors (CAFs) are a promising area of investigation with the potential to identify easy-to-obtain and clinically meaningful biomarkers. Urine biomarkers and “liquid biopsy” with circulating tumor DNA (ctDNA) are also areas of active investigation.
Serum biomarkers
Vascular endothelial growth factor (VEGF) is central to the angiogenesis of RCC and the VEGF pathway is targeted by the majority of drugs approved by the US FDA for use in mRCC (4). As a result, the use of baseline serum VEGF level as a prognostic biomarker and predictive biomarker for VEGF TT response has been investigated. The treatment approaches in renal cancer global evaluation trial (TARGET) study evaluated sorafenib versus placebo in the second-line (2L) setting and also examined the utility of serum VEGF levels as a prognostic and predictive biomarker of sorafenib treatment benefit (25). Baseline VEGF levels correlated inversely with PFS and OS in patients treated with placebo. Multivariate analysis including ECOG performance status (PS) and Memorial Sloan-Kettering Cancer Center (MSKCC) score demonstrated that baseline VEGF was an independent prognostic factor for PFS in patients receiving placebo but not sorafenib-treated patients. Baseline VEGF level was independently prognostic of OS in both placebo- and sorafenib-treated patients (25). Analysis of VEGF as a predictive biomarker of treatment response to sorafenib was carried out using the median baseline VEGF level (131 pg/mL) to define high- and low-VEGF groups. This analysis found that both groups benefited from sorafenib treatment and no significant difference was found. However, exploratory analysis using the 75th percentile of baseline VEGF (254 pg/mL) to define high- and low-VEGF groups demonstrated that the high-VEGF group derived more benefit from sorafenib than the low-VEGF group.
Additional biomarkers were tested in a follow up study using the same population of patients: high baseline levels of plasma CA-IX, TIMP-1, and Ras p21 were prognostic for reduced OS in mRCC patients and TIMP-1 remained independently significant in a multivariable analysis that included MSKCC score and ECOG PS (26). However, none of these additional biomarkers was predictive of sorafenib benefit.
Using data from a phase II trial of sorafenib vs. sorafenib plus interferon-α (IFN-α), Zurita and colleagues evaluated 52 CAFs to identify candidate predictive and prognostic biomarkers (27). In this analysis, patients with low (below median concentration) baseline osteopontin or VEGF who received sorafenib alone had lower PFS than those who received combination therapy with sorafenib plus IFN-α. Additionally, this study found that a 6-biomarker panel [osteopontin, soluble CA-IX (sCA9), VEGF, tumor necrosis factor-related apoptosis-inducing ligand (TRAIL), collagen IV (ColIV), and soluble VEGF receptor-2 (sVEGFR2)] was significantly predictive of PFS with sorafenib + IFN-α versus sorafenib alone (27). Despite these promising findings regarding the use of VEGF as a predictive biomarker, other studies that have evaluated VEGF have not confirmed these results (28,29).
Interleukin 6 (IL-6) is another promising biomarker, as there is evidence that it is secreted by RCC cells when they are exposed to hypoxia (30). Using data from phase II and III studies of pazopanib in the 2L setting, Tran and colleagues screened, confirmed and validated several prospective biomarkers (29). In the initial screening, low serum levels of hepatocyte growth factor (HGF), IL-6, and interleukin 8 (IL-8) correlated with greater tumor shrinkage with pazopanib therapy. Additionally, low IL-6 and high E-selectin were associated with prolonged PFS. Considering the results of the previously discussed sorafenib study (27), osteopontin and VEGF were also considered during the validation phase. In patients treated with pazopanib, low levels of IL-8, HGF, osteopontin and TIMP-1 were all associated with significantly longer PFS (29). In patients receiving placebo, IL-6, IL-8 and osteopontin were all prognostic of PFS. Additionally, these three markers were all stronger prognostic markers than any clinical classification (MSKCC score, ECOG PS, or Heng criteria). IL-6 was the only significant predictive biomarker in this study. While high IL-6 levels were associated with shorter PFS in the placebo group, these patients had a greater benefit from pazopanib therapy than those with low IL-6 levels (29). While IL-6 is a promising candidate predictive biomarker, it has not yet been studied in a prospective fashion. Indeed, no level 1 evidence currently exists for a biomarker predictive of survival with VEGF-directed therapy (31).
Work has also been done to examine predictors of targeted therapies against the mammalian target of rapamycin, or mTOR. Lactate dehydrogenase (LDH) is an enzyme involved in anaerobic glycolysis and regulated by the PI3-K/AKT/mTOR pathway (32). High serum LDH is an established poor prognostic factor in RCC and is part of the MSKCC risk score. Armstrong and colleagues evaluated serum LDH as a potential predictive biomarker of mTOR inhibitor therapy in mRCC (32). They confirmed that high serum LDH is a poor prognostic marker with a HR for death of 2.81 for patients with LDH greater than the upper limit of normal (32). Additionally, elevated LDH predicted OS benefit with temsirolimus as compared to interferon therapy (32).
Recently, Voss et al. (33) collected serum biomarkers from the RECORD-3 trial, a comparative study of first-line sunitinib vs. first-line everolimus in patients with treatment naïve mRCC (34). The RECORD-3 trial showed that the mTOR inhibitor everolimus was not non-inferior to the VEGF inhibitor sunitinib for first-line therapy in mRCC, concluding that sunitinib is the better first-line regimen. However, the tumor biology of patients enrolled in this study was heterogeneous, as the study had no molecular or histologic selection criteria and both ccRCC and non-ccRCC patients were included. Thus, the possibility existed that everolimus is a better first-line agent in a specific subgroup of patients. With this aim in mind, Voss et al. identified the 5 biomarkers (CSF1, ICAM1, IL-18BP, KIM1, TNFRII) with the strongest association for everolimus PFS and created a composite biomarker score (CBS) (33). Everolimus-treated patients with high CBS had significantly better PFS than those with low CBS. Further, CBS did not correlate with PFS in sunitinib patients, suggesting CBS as an everolimus-specific set of biomarkers and the potential of serum biomarkers for prediction of treatment outcome (4).
Various non-CAF serum biomarkers have been incorporated into prognostic models to predict survival of mRCC patients. The Heng score is a validated model to predict median OS in mRCC patients receiving VEGF TT and includes prognostic serum biomarkers of hemoglobin, calcium, neutrophil count, and platelet count (35). Similarly, the MSKCC score, or Motzer score, employs three serum biomarkers—hemoglobin, calcium, and LDH to predict median OS in mRCC patients (36) (Tables 1,2).
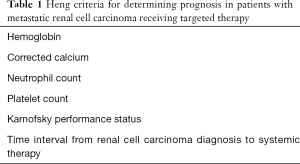
Full table
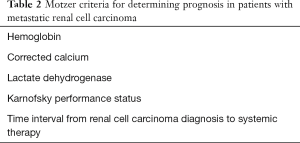
Full table
Additional non-CAF prognostic serum biomarkers of recent interest are those related to systemic inflammation, as chronic inflammation may suppress anti-tumoral immune system activity. The neutrophil-lymphocyte ratio (NLR) is one such biomarker and is linked to systemic and local inflammation. A recent review by Boissier et al. identified seven studies examining the prognostic value of NLR in mRCC or locally advanced RCC (37). They found that a high NLR independently predicted decreased OS (HR =1.55; 95% CI, 1.36–1.76) and PFS (HR =3.19; 95% CI, 2.23–4.57). Moreover, Ohno et al. demonstrated that NLR independently predicts OS in patients with mRCC undergoing cytoreductive nephrectomy (CN) (38). Patients with mRCC undergoing CN with NLR >4.0 did not have an OS benefit compared to those patients not undergoing CN. Similar in concept to NLR, C-reactive protein (CRP) is a systemic marker of inflammation and shows prognostic promise with respect to RCC outcomes. In a cohort of 587 patients with localized RCC, CRP independently predicted OS, CSS, and metastasis-free survival (MFS) on multivariate analysis (39). For patients with mRCC, CRP was again shown to be an independent predictor of OS in 88 patients treated with metastasectomy for mRCC (40).
Urine biomarkers
Urine biomarkers are also an attractive method for acquiring diagnostic and prognostic data, though research in this area is more limited. Many candidate urinary biomarkers for kidney cancer, such as neutrophil gelatinase-associated lipocalin (NGAL) and kidney injury molecule-1 (KIM-1), have demonstrated low sensitivity and specificity and cannot differentiate benign renal conditions from malignancy. Two urinary biomarkers that have shown promise are the exosomal proteins aquaporin-1 (AQP-1) and perilipin-2 (PLIN2). Morrissey et al. collected urine samples from 36 patients with ccRCC or papillary renal cell carcinoma (pRCC), as well as controls, and found that patients with kidney cancer (compared to controls) had 23- and 4-fold greater levels of AQP1 and PLIN2, respectively. Further, AQP1 and PLIN2 levels both decreased by over 80% after surgical removal of the tumor, suggesting these urinary biomarkers may have diagnostic relevance in RCC patients. Another novel urinary biomarker with promising utility is glycosaminoglycans (GAGs) score, as GAGs are transcriptionally upregulated in mRCC. Gatto et al. collected both urine and serum GAG levels in 23 patients with metastatic ccRCC (41). They found that urine GAG score significantly independently predicted both PFS (P=0.003) and OS (P=0.009), while none of the serum GAG scores achieved statistical significance.
Liquid biopsy
ctDNA obtained from peripheral blood has recently emerged as another promising biomarker. Establishing a tumor’s genomic profile via tissue-based (e.g., surgical or biopsy derived) sampling is limited by sampling bias, difficulty in obtainment, and risk to the patient. Conversely, sampling ctDNA (i.e., “liquid biopsy”) is advantageous in that it is non-invasive and allows for serial measurements for dynamic monitoring of tumoral genomics or response to treatment. In a recent abstract by Pal et al., ctDNA was collected from 224 patients with mRCC and GAs were compared in patients receiving first- or second-line therapy (42). They found that second-line patients who previously underwent first-line VEGF-directed therapy had stark differences in p53 and mTOR GAs compared to first-line patients. Thus, ctDNA may be a useful tool for detecting resistance to therapy and guiding treatment. Ball et al. performed targeted gene sequencing on four tumor specimens from patients with locally advanced or mRCC to identify known RCC mutations (43). They found one RCC-related gene mutation (VHL, BAP1, PBRM1, or NF) in each tumor (43). Next, they preoperatively collected and analyzed ctDNA targeted towards each patient’s specific mutation. PCR was able to detect 1 of 4 of the mutations (the VHL mutation in a patient with mRCC). Importantly, ctDNA levels decreased to an undetectable level post-nephrectomy while on systemic therapy and then increased again with disease progression. This study suggests ctDNA may serve as a biomarker for tumor burden and response to therapy.
While the above studies have identified and validated various biomarkers predictive of treatment outcome in RCC, all are retrospective in nature. Therefore, it is vitally important that promising candidate biomarkers be prospectively studied in order to understand their characteristics prior to routine clinical use.
Tissue biomarkers
Histologically, classification of renal tumors is based on morphological, immunohistochemical and chromosomal characteristics. While the majority of renal tumors can be subtyped by morphology alone, immunohistochemical stains may provide supplemental diagnostic information. In addition, many renal tumors have specific chromosomal aberrations, contributing to several new classifications.
Immunohistochemistry
The normal renal parenchyma and most renal neoplasms express PAX8 and PAX2 transcription factors. Therefore these are useful markers to aid in identifying metastatic lesions as renal in origin. PAX2 is less sensitive than PAX8, though it is more specific. Both can be negative in high grade tumors, and they are negative in angiomyolipoma (44). Another marker for renal tumors is RCC-marker, though it is less specific and less sensitive than PAX8 or PAX2 and is consequently now of limited utility.
Some of the most common types of RCC are positive for vimentin, a stain that is usually known more as a mesenchymal marker. Despite not being a mesenchymal lesion, ccRCC and pRCC are a few of carcinomas that express both cytokeratins and vimentin. It is particularly useful to differentiate these RCCs from chRCC and oncocytomas, which usually stain negative (44-46).
CK7 is a low molecular weight cytokeratin that stains cytoplasm and is useful for differentiation of multiple types of RCC. One use is to differentiate pRCC from ccRCC. In pRCC, type 1 shows diffuse CK7 staining, while type 2 stains less diffusely. On the other hand, ccRCC is generally negative for CK7. A more recently recognized entity, clear cell papillary RCC (ccpRCC) stains diffusely with CK7 (Figure 2) (47). chRCC and oncocytomas can both express CK7, but in different staining patterns. In chRCC it is strongly and diffusely positive, while in oncocytoma it only shows focal positivity or no staining at all (44,46,48). In addition, another stain that can be used to differentiate between chRCC and oncocytoma is CD117 (also known as c-kit), which is a stem cell factor receptor. In chRCC, CD117 shows a membranous staining pattern, while in oncocytoma it shows a strong cytoplasmic staining pattern (44). Hale’s colloidal iron is a mucin stain that stains hemosiderin. In chRCC it shows strong and diffuse staining, while in oncocytoma, it is largely negative, with weak, focal granular staining. Due to the possible staining of some oncocytomas, as well as the technical difficulty of executing the stain, Hale’s colloidal iron is usually performed in conjunction with other stains (45,49).
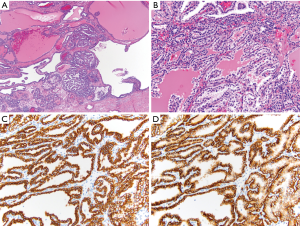
While in general other cytokeratin stains are of limited utility in renal tumors, a recent study has shown 34βE12 to be useful for identifying ccpRCC. Its best use is in cases to distinguish it from ccRCC, particularly when the morphology is equivocal (50). Another more recent immunohistochemical stain, cathepsin K, has been shown to be a useful marker for angiomyolipoma and translocation RCC. It is a cysteine protease and plays an important part in the function of microphthalmia-associated transcription factor (MITF) transcription, and is often expressed in perivascular epithelioid cell tumors (PEComas). Due to its role in MITF transcription, it also shows positivity in translocation RCCs, as translocation RCCs involves the TFE3 or TFEB genes, which are members of the MITF family (44,46,51,52).
Stains to help identify angiomyolipomas include HMB45 and melan-A, which are markers used most frequently to identify melanomas (46,53,54). These markers are also positive in translocation RCCs (46,55). α SMA and desmin are muscle markers that will stain positive for the muscle-differentiating portion of angiomyolipomas (45). P53 is a tumor suppressor gene that shows positivity in epithelioid angiomyolipoma. It may stain in classic angiolipoma, but it stains much stronger in epithelioid angiolipoma (56).
Other immunohistochemical markers that can be useful in differential diagnosis of renal tumors, usually used in conjunction with other stains, include CA-IX, α-methylacyl coenzyme A racemase (AMACR), CD10 and epithelial membrane antigen (EMA). CA-IX is a transmembrane protein that can identify ccRCC when the morphology is not obvious. It is positive in ccRCC and typically negative in all other RCC types (46). In ccpRCC, there is characteristic diffuse “cup shaped” pattern of basolateral membranous staining, with lack of luminal staining (Figure 2) (57). AMACR is a mitochondrial enzyme that is normally expressed in proximal renal tubules. It is helpful in identifying pRCC and ccpRCC, as it is positive in these two entities, and typically negative in other RCC subtypes (44,58). CD10 is a cell-surface glycoprotein that is helpful in identifying ccRCC and pRCC. In ccRCC, it shows diffuse membranous staining, while in PRCC, it shows patchy luminal staining. CD10 is also positive for Xp11.2 translocation RCC but negative for all other RCC histologies (44,58). EMA, also known as MUC1, stains either membranous or cytoplasmic, and is helpful in differentiating ccRCC, pRCC, chRCC, and oncocytoma by its staining patterns. In both ccPRCC and pRCC, it has a membranous staining pattern, while in chRCC and oncocytoma it stains diffusely cytoplasmic (45).
Molecular and genetic
While the majority of RCCs are sporadic, some are associated with hereditary conditions or genetic syndromes. The most common RCC, ccRCC usually involves the VHL gene. Patients with VHL syndrome present with bilateral multifocal ccRCC as well as central nervous system, adrenal, pancreatic, ocular, aural, and epididymal manifestations (59). Other RCC associated mutations include PBRM1, SETD2, KDM5C, PTEN, BAP1, MTOR, and TP53. By cytogenetics, VHL tumors commonly show loss of heterozygosity (LOH) of chromosome 3p, and biallelic inactivation of VHL gene (3p25), as well as gain of 5q22, loss of 6q, 8p, 9p and 14q (58,60,61). A more recent entity, multilocular cystic renal neoplasm of low malignant potential has also been found to be associated with 3p deletion and in some cases, VHL mutations (62,63).
The two types of papillary RCC have been shown to have different genetic abnormalities, and can be associated with different hereditary syndromes (2). Papillary type I is associated with MET alterations, and cytogenetics shows frequent gains in chromosome 7p and 17p, loss of chromosome Y, and variable gains in chromosomes 3q, 8p, 12q, 16q and 20q (48,59). Papillary type II is more heterogeneous and may be associated with silencing of CDKN2A, mutations in SETD2 and NRF2-ARE pathways, TFE3 fusions, as well as some CpG island methylator phenotypes. Cytogenetics shows allelic imbalance of one or more chromosomes, including 1p, 3p, 5, 6, 8, 9p, 10, 11, 15, 18 and 22 (48,54,58,60,61,64,65). Patients with hereditary papillary renal cancer (HPRC) develop bilateral multifocal type I tumors, while those with HLRCC, which is caused by a germline mutation of the fumarate hydratase gene, develop papillary type II tumors that characteristically have eosinophilic cytoplasm and large inclusion-like nucleoli (66,67).
Distinctly different from pRCC, ccpRCC lacks gain of chromosome 7 or loss of chromosome Y. In addition, it is also different from ccRCC, lacking 3p chromosome anomalies. To date, no clinically significant gene mutations have been identified (52,54,58,60,68,69).
TFE3 and TFEB of the MiT family transcriptions factors are associated with Xp11.2 and t(6;11)(p21;q12) translocation RCCs, respectively. The more common translocation involving Xp11.2 results in TFE3 gene fusions, while the less common translocation involving translocation from 6p to 11q12, results in MALAT1-TFEB gene fusions (51,52,55,70).
chRCC grows slowly in vitro, and because of this there are fewer cytogenetic reports compared to other RCC subtypes. Significant mutations have been found in TP53 and PTEN. Other relevant genes that have shown mutation infrequently include mTOR, NRAS, and TSC1 and 2 (71). The most common findings include low chromosome numbers, most often with losses of chromosomes 1, 2, 6, 10, 13, 17 and/or 21. Less frequently, loss of chromosomes 3, 5, 8, 9, 11, 18 and 21 may be seen (45,71-73). Oncocytoma is frequently associated with CCND1 mutations, and most commonly shows complete or partial loss of chromosome 1. Other common changes include loss of Y, monosomy 14, and trisomy 7. However, approximately 50% of oncocytomas may show no chromosomal abnormalities (74-76). Multiple chRCCs, oncocytomas, hybrid tumors and/or oncocytosis are seen in Birt-Hogg-Dube (BHD) syndrome, caused by a mutation in the folliculin gene (77).
Conclusions
Healthcare providers are faced with a rising incidence of renal masses, likely due to an increase in the use of cross-sectional imaging resulting in incidentally found kidney tumors (78). Given the heterogeneity of renal mass biology, developing reliable means of determining diagnosis, optimal therapy, and prognosis are critical. Biomarkers can help achieve these goals.
Imaging biomarkers include PET/CT with various radiotracers, multi-parametric MRI, and radiomics, and play a role in the assessment of tumor subtypes as well as response to treatment. Serum biomarkers have prognostic benefit primarily in the mRCC setting and may help us realize the promise of precision oncology. Tissue biomarkers may be categorized as immunohistochemical or molecular. The former employs various stains as a diagnostic adjunct, while molecular biomarkers analyze chromosomal aberrations to determine diagnosis and potentially inform treatment. Prospective clinical trials are needed to provide validation of novel biomarkers to ensure they are well validated before introduced into clinical practice. Future directions include the development of a pan-omic approach (radiomics, genomics, proteomics, etc.), rather than relying on individual approaches, which would allow for a detailed system-level analysis (79). This combinatorial approach to systems biology offers the potential of enhanced clinical decision-making support, which would be a benefit to patients and providers alike.
Acknowledgments
Funding: This work is supported by a grant from the National Cancer Institute (P30CA072720).
Footnote
Conflicts of Interest: All authors have completed the ICMJE uniform disclosure form (available at http://dx.doi.org/10.21037/tcr.2017.05.19). EAS serves as an unpaid editorial board member of Translational Cancer Research from Sep 2016 to Dec 2018. The other authors have no conflicts of interest to declare.
Ethical Statement: The authors are accountable for all aspects of the work in ensuring that questions related to the accuracy or integrity of any part of the work are appropriately investigated and resolved.
Open Access Statement: This is an Open Access article distributed in accordance with the Creative Commons Attribution-NonCommercial-NoDerivs 4.0 International License (CC BY-NC-ND 4.0), which permits the non-commercial replication and distribution of the article with the strict proviso that no changes or edits are made and the original work is properly cited (including links to both the formal publication through the relevant DOI and the license). See: https://creativecommons.org/licenses/by-nc-nd/4.0/.
References
- Siegel RL, Miller KD, Jemal A. Cancer Statistics, 2017. CA Cancer J Clin 2017;67:7-30. [Crossref] [PubMed]
- Barrisford GW, Singer EA, Rosner IL, et al. Familial renal cancer: molecular genetics and surgical management. Int J Surg Oncol 2011;2011:658767
- Strimbu K, Tavel JA. What are biomarkers? Curr Opin HIV AIDS 2010;5:463-6. [Crossref] [PubMed]
- Modi PK, Farber NJ, Singer EA. Precision Oncology: Identifying Predictive Biomarkers for the Treatment of Metastatic Renal Cell Carcinoma. Transl Cancer Res 2016;5:S76-S80. [Crossref] [PubMed]
- Finelli A, Ismaila N, Bro B, et al. Management of Small Renal Masses: American Society of Clinical Oncology Clinical Practice Guideline. J Clin Oncol 2017;35:668-80. [Crossref] [PubMed]
- Krajewski KM, Shinagare AB. Novel imaging in renal cell carcinoma. Curr Opin Urol 2016;26:388-95. [Crossref] [PubMed]
- Gofrit ON, Orevi M. Diagnostic Challenges of Kidney Cancer: A Systematic Review of the Role of Positron Emission Tomography-Computerized Tomography. J Urol 2016;196:648-57. [Crossref] [PubMed]
- Nakaigawa N, Kondo K, Tateishi U, et al. FDG PET/CT as a prognostic biomarker in the era of molecular-targeting therapies: max SUVmax predicts survival of patients with advanced renal cell carcinoma. BMC Cancer 2016;16:67. [Crossref] [PubMed]
- Kayani I, Avril N, Bomanji J, et al. Sequential FDG-PET/CT as a biomarker of response to Sunitinib in metastatic clear cell renal cancer. Clin Cancer Res 2011;17:6021-8. [Crossref] [PubMed]
- Caldarella C, Muoio B, Isgro MA, et al. The role of fluorine-18-fluorodeoxyglucose positron emission tomography in evaluating the response to tyrosine-kinase inhibitors in patients with metastatic primary renal cell carcinoma. Radiol Oncol 2014;48:219-27. [Crossref] [PubMed]
- Shuch B, Linehan WM, Srinivasan R. Aerobic glycolysis: a novel target in kidney cancer. Expert Rev Anticancer Ther 2013;13:711-9. [Crossref] [PubMed]
- Divgi CR, Uzzo RG, Gatsonis C, et al. Positron emission tomography/computed tomography identification of clear cell renal cell carcinoma: results from the REDECT trial. J Clin Oncol 2013;31:187-94. [Crossref] [PubMed]
- Farber NJ, Wu Y, Zou L, et al. Challenges in RCC Imaging: Renal Insufficiency, Post-Operative Surveillance, and the Role of Radiomics. Kidney Cancer J 2015;13:84-90. [PubMed]
- Turkbey B, Lindenberg ML, Adler S, et al. PET/CT imaging of renal cell carcinoma with (18)F-VM4-037: a phase II pilot study. Abdom Radiol (NY) 2016;41:109-18. [Crossref] [PubMed]
- Rowe SP, Gorin MA, Hammers HJ, et al. Imaging of metastatic clear cell renal cell carcinoma with PSMA-targeted (1)(8)F-DCFPyL PET/CT. Ann Nucl Med 2015;29:877-82. [Crossref] [PubMed]
- Rowe SP, Gorin MA, Hammers HJ, et al. Detection of 18F-FDG PET/CT Occult Lesions With 18F-DCFPyL PET/CT in a Patient With Metastatic Renal Cell Carcinoma. Clin Nucl Med 2016;41:83-5. [Crossref] [PubMed]
- Gorin MA, Rowe SP, Baras AS, et al. Prospective Evaluation of (99m)Tc-sestamibi SPECT/CT for the Diagnosis of Renal Oncocytomas and Hybrid Oncocytic/Chromophobe Tumors. Eur Urol 2016;69:413-6. [Crossref] [PubMed]
- Wu Y, Kwon YS, Labib M, et al. Magnetic Resonance Imaging as a Biomarker for Renal Cell Carcinoma. Dis Markers 2015;2015:648495
- Lanzman RS, Robson PM, Sun MR, et al. Arterial spin-labeling MR imaging of renal masses: correlation with histopathologic findings. Radiology 2012;265:799-808. [Crossref] [PubMed]
- de Bazelaire C, Alsop DC, George D, et al. Magnetic resonance imaging-measured blood flow change after antiangiogenic therapy with PTK787/ZK 222584 correlates with clinical outcome in metastatic renal cell carcinoma. Clin Cancer Res 2008;14:5548-54. [Crossref] [PubMed]
- Kang SK, Zhang A, Pandharipande PV, et al. DWI for Renal Mass Characterization: Systematic Review and Meta-Analysis of Diagnostic Test Performance. AJR Am J Roentgenol 2015;205:317-24. [Crossref] [PubMed]
- Gaing B, Sigmund EE, Huang WC, et al. Subtype differentiation of renal tumors using voxel-based histogram analysis of intravoxel incoherent motion parameters. Invest Radiol 2015;50:144-52. [Crossref] [PubMed]
- Gillies RJ, Kinahan PE, Hricak H. Radiomics: Images Are More than Pictures, They Are Data. Radiology 2016;278:563-77. [Crossref] [PubMed]
- Shinagare AB, Vikram R, Jaffe C, et al. Radiogenomics of clear cell renal cell carcinoma: preliminary findings of The Cancer Genome Atlas-Renal Cell Carcinoma (TCGA-RCC) Imaging Research Group. Abdom Imaging 2015;40:1684-92. [Crossref] [PubMed]
- Escudier B, Eisen T, Stadler WM, et al. Sorafenib for treatment of renal cell carcinoma: Final efficacy and safety results of the phase III treatment approaches in renal cancer global evaluation trial. J Clin Oncol 2009;27:3312-8. [Crossref] [PubMed]
- Peña C, Lathia C, Shan M, et al. Biomarkers predicting outcome in patients with advanced renal cell carcinoma: Results from sorafenib phase III Treatment Approaches in Renal Cancer Global Evaluation Trial. Clin Cancer Res 2010;16:4853-63. [Crossref] [PubMed]
- Zurita AJ, Jonasch E, Wang X, et al. A cytokine and angiogenic factor (CAF) analysis in plasma for selection of sorafenib therapy in patients with metastatic renal cell carcinoma. Ann Oncol 2012;23:46-52. [Crossref] [PubMed]
- Hegde PS, Jubb AM, Chen D, et al. Predictive impact of circulating vascular endothelial growth factor in four phase III trials evaluating bevacizumab. Clin Cancer Res 2013;19:929-37. [Crossref] [PubMed]
- Tran HT, Liu Y, Zurita AJ, et al. Prognostic or predictive plasma cytokines and angiogenic factors for patients treated with pazopanib for metastatic renal-cell cancer: a retrospective analysis of phase 2 and phase 3 trials. Lancet Oncol 2012;13:827-37. [Crossref] [PubMed]
- Fitzgerald JP, Nayak B, Shanmugasundaram K, et al. Nox4 mediates renal cell carcinoma cell invasion through hypoxia-induced interleukin 6- and 8- production. PLoS One 2012;7:e30712 [Crossref] [PubMed]
- Funakoshi T, Lee CH, Hsieh JJ. A systematic review of predictive and prognostic biomarkers for VEGF-targeted therapy in renal cell carcinoma. Cancer Treat Rev 2014;40:533-47. [Crossref] [PubMed]
- Armstrong AJ, George DJ, Halabi S. Serum lactate dehydrogenase predicts for overall survival benefit in patients with metastatic renal cell carcinoma treated with inhibition of mammalian target of rapamycin. J Clin Oncol 2012;30:3402-7. [Crossref] [PubMed]
- Voss MH, Chen D, Marker M, et al. Circulating biomarkers and outcome from a randomised phase II trial of sunitinib vs everolimus for patients with metastatic renal cell carcinoma. Br J Cancer 2016;114:642-9. [Crossref] [PubMed]
- Motzer RJ, Barrios CH, Kim TM, et al. Phase II randomized trial comparing sequential first-line everolimus and second-line sunitinib versus first-line sunitinib and second-line everolimus in patients with metastatic renal cell carcinoma. J Clin Oncol 2014;32:2765-72. [Crossref] [PubMed]
- Heng DY, Xie W, Regan MM, et al. Prognostic factors for overall survival in patients with metastatic renal cell carcinoma treated with vascular endothelial growth factor-targeted agents: results from a large, multicenter study. J Clin Oncol 2009;27:5794-9. [Crossref] [PubMed]
- Motzer RJ, Mazumdar M, Bacik J, et al. Survival and prognostic stratification of 670 patients with advanced renal cell carcinoma. J Clin Oncol 1999;17:2530-40. [Crossref] [PubMed]
- Boissier R, Campagna J, Branger N, et al. The prognostic value of the neutrophil-lymphocyte ratio in renal oncology: A review. Urol Oncol 2017;35:135-41. [Crossref] [PubMed]
- Ohno Y, Nakashima J, Ohori M, et al. Clinical variables for predicting metastatic renal cell carcinoma patients who might not benefit from cytoreductive nephrectomy: neutrophil-to-lymphocyte ratio and performance status. Int J Clin Oncol 2014;19:139-45. [Crossref] [PubMed]
- Dalpiaz O, Luef T, Seles M, et al. Critical evaluation of the potential prognostic value of the pretreatment-derived neutrophil-lymphocyte ratio under consideration of C-reactive protein levels in clear cell renal cell carcinoma. Br J Cancer 2017;116:85-90. [Crossref] [PubMed]
- Rausch S, Kruck S, Walter K, et al. Metastasectomy for metastatic renal cell carcinoma in the era of modern systemic treatment: C-reactive protein is an independent predictor of overall survival. Int J Urol 2016;23:916-21. [Crossref] [PubMed]
- Gatto F, Maruzzo M, Magro C, et al. Prognostic Value of Plasma and Urine Glycosaminoglycan Scores in Clear Cell Renal Cell Carcinoma. Front Oncol 2016;6:253. [Crossref] [PubMed]
- Pal SK, Sonpavde G, Agarwal N, et al. Evolution of circulating tumor DNA (ctDNA) profile from first-line (1L) to second-line (2L) therapy in metastatic renal cell carcinoma (mRCC). J Clin Oncol 2017;35. abstract 434.
- Ball MW, Gorin MA, Guner G, et al. Circulating Tumor DNA as a Marker of Therapeutic Response in Patients With Renal Cell Carcinoma: A Pilot Study. Clin Genitourin Cancer 2016;14:e515-e520. [Crossref] [PubMed]
- Shen SS, Truong LD, Scarpelli M, et al. Role of immunohistochemistry in diagnosing renal neoplasms: when is it really useful? Arch Pathol Lab Med 2012;136:410-7. [Crossref] [PubMed]
- Tan PH, Cheng L, Rioux-Leclercq N, et al. Renal tumors: diagnostic and prognostic biomarkers. Am J Surg Pathol 2013;37:1518-31. [Crossref] [PubMed]
- Kryvenko ON, Jorda M, Argani P, et al. Diagnostic approach to eosinophilic renal neoplasms. Arch Pathol Lab Med 2014;138:1531-41. [Crossref] [PubMed]
- Liddell H, Mare A, Heywood S, et al. Clear cell papillary renal cell carcinoma: a potential mimic of conventional clear cell renal carcinoma on core biopsy. Case Rep Urol 2015;2015:423908
- Fernandes DS, Lopes JM. Pathology, therapy and prognosis of papillary renal carcinoma. Future Oncol 2015;11:121-32. [Crossref] [PubMed]
- Tickoo SK, Amin MB, Zarbo RJ. Colloidal iron staining in renal epithelial neoplasms, including chromophobe renal cell carcinoma: emphasis on technique and patterns of staining. Am J Surg Pathol 1998;22:419-24. [Crossref] [PubMed]
- Martignoni G, Brunelli M, Segala D, et al. Validation of 34betaE12 immunoexpression in clear cell papillary renal cell carcinoma as a sensitive biomarker. Pathology 2017;49:10-8. [Crossref] [PubMed]
- Martignoni G, Bonetti F, Chilosi M, et al. Cathepsin K expression in the spectrum of perivascular epithelioid cell (PEC) lesions of the kidney. Mod Pathol 2012;25:100-11. [Crossref] [PubMed]
- Rao Q, Xia QY, Cheng L, et al. Molecular genetics and immunohistochemistry characterization of uncommon and recently described renal cell carcinomas. Chin J Cancer Res 2016;28:29-49. [PubMed]
- Lei JH, Liu LR, Wei Q, et al. A Four-Year Follow-up Study of Renal Epithelioid Angiomyolipoma: A Multi-Center Experience and Literature Review. Sci Rep 2015;5:10030. [Crossref] [PubMed]
- Srigley JR, Delahunt B, Eble JN, et al. The International Society of Urological Pathology (ISUP) Vancouver Classification of Renal Neoplasia. Am J Surg Pathol 2013;37:1469-89. [Crossref] [PubMed]
- Smith NE, Illei PB, Allaf M, et al. t(6;11) renal cell carcinoma (RCC): expanded immunohistochemical profile emphasizing novel RCC markers and report of 10 new genetically confirmed cases. Am J Surg Pathol 2014;38:604-14. [Crossref] [PubMed]
- Li W, Guo L, Bi X, et al. Immunohistochemistry of p53 and Ki-67 and p53 mutation analysis in renal epithelioid angiomyolipoma. Int J Clin Exp Pathol 2015;8:9446-51. [PubMed]
- Al-Ahmadie HA, Alden D, Qin LX, et al. Carbonic anhydrase IX expression in clear cell renal cell carcinoma: an immunohistochemical study comparing 2 antibodies. Am J Surg Pathol 2008;32:377-82. [Crossref] [PubMed]
- Kuroda N, Tanaka A, Ohe C, et al. Recent advances of immunohistochemistry for diagnosis of renal tumors. Pathol Int 2013;63:381-90. [Crossref] [PubMed]
- Meloni-Ehrig AM. Renal cancer: cytogenetic and molecular genetic aspects. Am J Med Genet 2002;115:164-72. [Crossref] [PubMed]
- Lopez-Beltran A, Cheng L, Vidal A, et al. Pathology of renal cell carcinoma: an update. Anal Quant Cytopathol Histpathol 2013;35:61-76. [PubMed]
- . Cancer Genome Atlas Research N. Comprehensive molecular characterization of clear cell renal cell carcinoma. Nature 2013;499:43-9. [Crossref] [PubMed]
- Halat S, Eble JN, Grignon DJ, et al. Multilocular cystic renal cell carcinoma is a subtype of clear cell renal cell carcinoma. Mod Pathol 2010;23:931-6. [Crossref] [PubMed]
- von Teichman A, Comperat E, Behnke S, et al. VHL mutations and dysregulation of pVHL- and PTEN-controlled pathways in multilocular cystic renal cell carcinoma. Mod Pathol 2011;24:571-8. [Crossref] [PubMed]
- Modi PK, Singer EA. Improving our understanding of papillary renal cell carcinoma with integrative genomic analysis. Ann Transl Med 2016;4:143. [Crossref] [PubMed]
- Cancer Genome Atlas Research N. Comprehensive Molecular Characterization of Papillary Renal-Cell Carcinoma. N Engl J Med 2016;374:135-45. [Crossref] [PubMed]
- Przybycin CG, Magi-Galluzzi C, McKenney JK. Hereditary syndromes with associated renal neoplasia: a practical guide to histologic recognition in renal tumor resection specimens. Adv Anat Pathol 2013;20:245-63. [Crossref] [PubMed]
- Merino MJ, Torres-Cabala C, Pinto P, et al. The morphologic spectrum of kidney tumors in hereditary leiomyomatosis and renal cell carcinoma (HLRCC) syndrome. Am J Surg Pathol 2007;31:1578-85. [Crossref] [PubMed]
- Lin X. Cytomorphology of clear cell papillary renal cell carcinoma. Cancer 2017;125:48-54. [PubMed]
- Raspollini MR, Castiglione F, Cheng L, et al. Genetic mutations in accordance with a low malignant potential tumour are not demonstrated in clear cell papillary renal cell carcinoma. J Clin Pathol 2016;69:547-50. [Crossref] [PubMed]
- Zheng G, Martignoni G, Antonescu C, et al. A broad survey of cathepsin K immunoreactivity in human neoplasms. Am J Clin Pathol 2013;139:151-9. [Crossref] [PubMed]
- Davis CF, Ricketts CJ, Wang M, et al. The somatic genomic landscape of chromophobe renal cell carcinoma. Cancer Cell 2014;26:319-30. [Crossref] [PubMed]
- Ng KL, Morais C, Bernard A, et al. A systematic review and meta-analysis of immunohistochemical biomarkers that differentiate chromophobe renal cell carcinoma from renal oncocytoma. J Clin Pathol 2016;69:661-71. [Crossref] [PubMed]
- Foix MP, Dunatov A, Martinek P, et al. Morphological, immunohistochemical, and chromosomal analysis of multicystic chromophobe renal cell carcinoma, an architecturally unusual challenging variant. Virchows Arch 2016; [Crossref] [PubMed]
- Joshi S, Tolkunov D, Aviv H, et al. The Genomic Landscape of Renal Oncocytoma Identifies a Metabolic Barrier to Tumorigenesis. Cell Rep 2015;13:1895-908. [Crossref] [PubMed]
- Al-Saleem T, Balsara BR, Liu Z, et al. Renal oncocytoma with loss of chromosomes Y and 1 evolving to papillary carcinoma in connection with gain of chromosome 7. Coincidence or progression? Cancer Genet Cytogenet 2005;163:81-5. [Crossref] [PubMed]
- Brunelli M, Delahunt B, Gobbo S, et al. Diagnostic usefulness of fluorescent cytogenetics in differentiating chromophobe renal cell carcinoma from renal oncocytoma: a validation study combining metaphase and interphase analyses. Am J Clin Pathol 2010;133:116-26. [Crossref] [PubMed]
- Pavlovich CP, Walther MM, Eyler RA, et al. Renal tumors in the Birt-Hogg-Dube syndrome. Am J Surg Pathol 2002;26:1542-52. [Crossref] [PubMed]
- Hollingsworth JM, Miller DC, Daignault S, et al. Rising incidence of small renal masses: a need to reassess treatment effect. J Natl Cancer Inst 2006;98:1331-4. [Crossref] [PubMed]
- Tan VM, Cheng LC, Drake JM. Complementing genomics and transcriptomics: Phosphoproteomics illuminates systems biology in prostate cancer. Mol Cell Oncol 2016;3:e1246075 [Crossref] [PubMed]