Taking one step backward to take two steps forward: the importance of breast tumor phenotype in MRI-based prediction of response
Introduction
Neoadjuvant chemotherapy (NAC) is currently among the mainstem of the treatment of locally advanced breast cancer, with the purpose to achieve tumor volume reduction and allowing conservative breast surgery (1,2). Overall, disease- and lesion-tailored therapeutic strategies are increasingly diffused in oncological practice since they tend to determine better rates of pathological complete response (pCR) after NAC (3). As well, pCR is an accepted predictor of better disease-free and overall survival rates (4). On the other hand, the effectiveness of NAC increases the number of patients receiving surgery with smaller residual tumors; this represents a diagnostic challenge for the radiologist.
An association has been traditionally demonstrated between tumor histology (i.e., ductal invasive vs. lobular invasive), responsiveness to therapy and late survival. More recently, the role of lesion phenotype has gained increased attention, in predicting biological behavior. In particular, analyzed patterns include the presence of hormone receptors (HRs) such as: HR+/human epidermal growth factor-2 (HER2)− (so-called luminal tumors); the HR−/HER2+ (so-called HER2 positive tumors); the HR−/HER2− (so-called triple-negative tumors); the HR+/HER2+ lesions (so-called hybrid tumors).
Magnetic resonance imaging (MRI) plays an important role in the assessment of response to NAC and, therefore, to tailor the subsequent management strategy. A large dedicated meta-analysis of 2,050 patients from 44 studies underlined that MRI has good accuracy for the detection of pCR after NAC [area under the curve (AUC) =0.88], greater than the accuracy of mammography (P=0.02) and that of clinical examination alone. Nevertheless, the accuracy of tumor phenotype in predicting pCR has been poorly assessed (5). In a previous work conducted on a monocentric series of 225 patients, we suggested that tumor phenotype evidenced through breast biopsy influences the diagnostic performance of MRI: in particular, the triple negative and HER2+ subgroups showed adequate receiver operating characteristic curve (ROC) AUCs for detection of pCR (0.766 and 0.813, respectively) as opposed to the luminal and hybrid tumors (0.588 and 0.567, respectively) (6,7). To this subject, Lindberg and coworkers recently published a literature review of 15 investigations published in the 2010–2014 period; they concluded for too weak evidence to definitely support the idea of differential MRI diagnostic capabilities according to tumor subtypes (8). Nonetheless, the time span of papers included suggests the need of an updated review of the evidence on this subject.
The results of pathology may be integrated by radiological parameters such as the value of the apparent diffusion coefficient (ADC). One review questioned the value of pre-treatment ADC for the discrimination between responders and non-responders (9). This review was nonetheless based on underpowered investigations and, more importantly, it did not take into consideration stratification by phenotypes. We previously confirmed this concept in the overall population of patients with locally advanced breast cancer, but at the same time suggested that the triple-negative and HER2+ tumors are characterized by ameliorated diagnostic performance through ADC calculation (7).
Our purpose is to revise current literature about the relationship between tumor subtypes and diagnostic performance of MRI monitoring tumor response to NAC. We also discuss the potential role of ADC measurement in assessing tumor response. Data are expected to be of help in the decision-making process for the increasing number of patients undergoing NAC for breast cancer.
Methods
In July 2017, we conducted a literature search in the PubMed National Center for Biotechnology Information, National Institutes of Health database (MEDLINE). The search was limited to abstracts published between January 2000 and June 2017. The abstracts had to be written in English, and the keywords entered for searching under the categories “search word” and “subject heading” included: neoadjuvant chemotherapy; breast cancer; MRI; breast tumor phenotypes; complete response; apparent diffusion coefficient; diffusion-weighted imaging (DWI). Data that have not undergone peer review and proprietary studies were excluded. Studies had to include data about diagnostic performance of MRI for either the pre-treatment prediction of pCR to NAC, or the detection of pCR. Although the focus of the review concerns the impact of tumor phenotypes on the diagnostic performance of phenotypes, studies involving no stratification according to phenotypes were also noted and discussed. We included investigations with variable definitions of complete response as the outcome variable, since our purpose was to address the impact of tumor phenotype on MRI diagnostic performance. To the purposes of the present investigation, ‘MRI’ or ‘MRI examination’ identifies the MRI study performed after the conclusion of NAC, otherwise differently indicated.
Rationale for investigation of tumor phenotypes in MRI
A large body of literature exists on the accuracy and diagnostic performance indexes for MRI in the prediction of pCR, based on morphological factors [i.e., RECIST classification (10)]. In a cumulative evaluation of the evidence, the accuracy of MRI in this context is reported to be 0.88 (5). Beyond imaging-based elements, the same systematic review indicated significantly variable relative diagnostic odds ratios (RDORs) for MRI depending on several factors, some of which are available at the time of MRI examination (such as patient’s age (RDOR =0.58 for patients aged >50), tumor stage [RDOR =0.79 for stage I or II), type of chemotherapy protocol (RDOR =2.02 for anthracycline + taxane protocols), time between therapy and MRI (RDOR =0.99 for one-day increase), biological features of the disease such as histology (RDOR =0.97 for 10% increase in IDC) and phenotype (RDOR =1.13 for HER2 expression >30% and RDOR =1.21 for each 10% increase in ER expression)]. Conversely, other factors are not available at the moment of MRI (presence vs. absence of in situ disease, scattered cells within the tumor bed). Essentially, it is underlined that a number of factors significantly affecting the effectiveness of MRI in identifying response to NAC can become available only later, after final histopathologic analysis. Performance reduction because of false-positive results may be due to inflammatory and reactive tissue changes associated with NAC. Although the above-referenced meta-analysis represent a reference work in the field, it included papers published until 2010, which explains its limited capability to clarify issues associated with phenotypes.
Differential behavior associated with opposite biological subtypes of breast cancer can probably help in the stratification of the likelihood of elements affecting the diagnostic performance of MRI: at the current state of knowledge, stratification by tumor phenotypes is the best available option to achieve such goal. Phenotypes are known at the time of post-NAC MRI examination, since this information is required to guide NAC regimen and is obtained through breast biopsy during the initial diagnostic phase. Hence, several studies have addressed the differential diagnostic characteristics of MRI depending on phenotypes.
Tumor phenotype and MRI
The evaluation of literature on performance of MRI post- according to tumor phenotypes (Table 1) needs to take into account variable definitions of pCR. In fact, most papers adopt absence of invasive disease in the tumor bed as definition of pCR, one adopts absence of both invasive and in situ components to define pCR, others require also negativity of axillary lymph nodes to define pCR). Additionally, diagnostic performance of MRI is in several cases presented as Sensitivity/Specificity/Accuracy rates, while in others methods based on size concordance between MRI and pathology, others report ROC AUC values. Despite recognized immunopathological tests (positivity/negativity of HRs and HER2), various modalities of tumor phenotype definition exist (Table 1), ranging between two and four classes. Despite these challenges, it is possible to enlighten a global concordance between the available evidence.
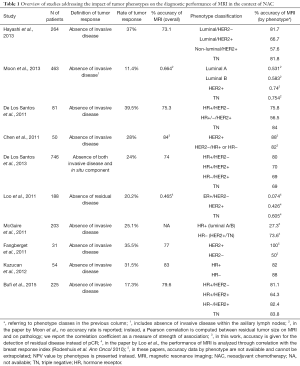
Full table
Accuracy for detection of pCR in a non-stratified population is globally concordant among papers, ranging between 73.1% and 83%. After stratification by phenotypes, accuracy (or other measurement of diagnostic performance) tend to be better among the TN and in HER2+ tumors not expressing HRs (Figures 1,2). McGuire et al. (11) employed a two-class stratification (TN and HER2+ vs. all HR+ lesions) and observed a marked difference in accuracy (73.6% vs. 27.3%) in a large single-center series of 203 cases. Of note, data in this study were obtained from a prospectively collected registry. Such conclusion is also obtained from investigations based on rather different methods of estimation of response to NAC: the breast response index (BRI), initially proposed by Rodenhuis et al. (12) and consisting in a score based on several MRI variables to describe response, showed an excellent correlation within TN and HER2+ tumors, while no significant correlation was evidenced for HR-expressing diseases (13). The Authors underlined inaccuracy of MRI for ER-expressing tumors, with the latter as the main biological factor associated with decreased performance. The so-called hybrid subgroup (expressing both HER2 and ER) is not reported independently in the study by Loo et al., but, interestingly, it shows the worse diagnostic performance for MRI among the four phenotype subgroups defined in our previous investigation [accuracy =64.3% (7)]. This element, beyond the potential consequences for management protocols, recalls the close relationship between biological behavior and radiological MRI-based diagnostic capabilities as the expression of tissue characteristics. Lesser powered investigations failed to disclose a significant difference, in terms of diagnostic accuracy, between HR+ and HR− tumors: nonetheless, in a more detailed analysis they also evidenced greater negative predictive value (NPV) among HR- tumors (56% vs. 88% in HR− lesions) (14). This aspect recurs in other investigations, such as in that by De Los Santos et al. (15). In this robust multicentre study (746 patients from 8 institutions) the NPV for detection of pCR was greatest among TN and HER2+ lesions (60% and 62%, respectively), i.e., the HR− tumors. Instead, HR+/HER2+ and HR+/HER2− lesions had their diagnostic performance hampered mainly by limited NPV (42% and 33%, respectively). It could be surprising to note that this very large study fails to identify significantly different diagnostic accuracy between HR− and HR+ tumors. Such apparent discrepancy can probably be explained by the absence of in situ component being used in this study as a criterion to define pCR, with consequent increase of false negative results with respect to the remaining papers in Table 1. This issue will be addressed again in the following paragraph about diffusion imaging. As well, the latter paper essentially confirms single-center findings by the same group obtained from a smaller cohort (16), although the single-center data are difficultly comparable to the multicentre database due to lack of stratification of HER2+ tumors into those expressing or not HRs. As such, it seems that stratification by HER2+ expression can provide useful insights. Although their study is underpowered (31 cases), Fangberget et al. (17) indicates 100% NPV among HER2+ lesions vs. 50% in HER2− cases.
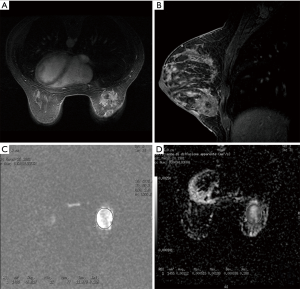
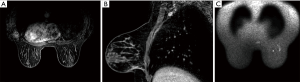
Nonetheless, the above study categorized patients according to HER2+ positivity only, and both subgroups included a proportion of HR-expressing lesions. Such consideration might explain the limited difference in accuracy between HER2+ vs. HER2− cases (88% vs. 82%) in the study by Chen et al. (18). In our opinion, these data suggest that HR status also impacts in a non-negligible way the performance of MRI: performance is therefore the result of the interplay of both HER2 and HR expression. In fact, we previously suggested that HR positivity prevails over HER2+ positivity in determining worse diagnostic performance (ROC AUC reportedly being 0.9 in TN lesions, 0.826 in HER2+/HR−, 0.693 in HER2−/HR+, and 0.611 in HER2+/HR+) (6). Concordantly, Moon et al. (19) identify the HR expression status as a major determinant of MRI performance. They provide no accuracy rate but correlation coefficient of residual tumor size between MRI and pathology findings instead. Despite that, correlation coefficient was lower and similar for HER2+/HR+ and HER−/HR+ (0.531 and 0.583) than for HER2+/HR− and TN (0.74 and 0.754), suggesting improved diagnostic capabilities in the latter two subgroups. Such finding is confirmed by multivariate analysis. Only one paper suggests lower accuracy in HER2+ tumors than in HR+ cases, while confirming greatest accuracy among TN lesions (81.8%) (20). They also indicate that better performance in the TN subgroup is associated with optimized Positive Predictive Value rather than NPV. Remarkably, a recent work strongly underlined the different behavior among TN and HR−/HER2+ tumors vs. HR+ cases, in the term that absence of late enhancement in these cases strongly associates with pCR, the AUC of MRI in these settings being 0.88 (21).
Potential role of the ADC variation in phenotype subgroups
In the previous section, we have addressed the strengths and pitfalls of post-NAC MRI in the prediction of pCR according to tumor phenotype. Overall, there is concordance about the relative diagnostic weakness of MRI in the subgroups expressing HRs. Such considerations are based on the morphological MRI criteria (RECIST classification) (10). It can be hypothesized that additional information can be yielded in this specific context through the use of DWI and calculation of the ADC. While the largest part of the literature focused on the evaluation of ADC difference throughout NAC as an additional measure to predict response (22-24), only one investigation addressed the ADC variation after NAC as a measure of prediction of pCR stratified by tumor phenotype (6). Herein, the employment of an ADC variation-based method to predict pCR was not associated with diagnostic improvement vs. the RECIST criteria in the TN and HR-/HER2+ cases (ROC AUC =0.8 and 0.833, respectively, vs. 0.9 and 0.826 for morphological MRI). Conversely, ROC AUCs for the ADC variation-based method in the HR+/HER2− (luminal) and HR+/HER2+ (so-called hybrid) were 0.787 and 0.722, respectively, both reaching statistical significance. In the same subgroups, ROC AUCs for the RECIST method were 0.693 and 0.611, reaching no or little statistical significance. This finding suggests a potential for filling this diagnostic gap through the coupling of morphological MRI and DWI; the radiologist might therefore adapt his/her judgement on the basis of the known tumor phenotype. Another group proposed the calculation of the ADC ratio (mean post-treatment ADC/mean pretreatment ADC), which was reported to be significantly increased in cases with pCR (21). The Authors supported the idea that the combination of these criteria (i.e., molecular subtype of the disease, morphological MRI information and ADC ratio) yields an optimal diagnostic performance for pCR (ROC AUC =0.92).
The information provided by DWI are based on the biological structure of the lesion, and under one point of view, it represents the closest MRI parameter to pathology- derived data. In such perspective, one can speculate that DWI might improve the diagnostic performance mainly by increased detection of in situ residual disease. In fact, studies including the absence of in situ component to define pCR (in addition to absence of invasive disease) tend to show better accuracy for morphological MRI in HR+/HER2− vs. HR-/HER2+ and TN lesions (15). Further investigations are required to confirm the potential role of post-NAC ADC to improve predictability of pCR in selected phenotype subgroups, and whether the residual in situ component after NAC behaves differently in terms of reduction of accuracy according to phenotypes.
ADC value before NAC and prediction of pCR
It has been already pointed out that the mean ADC value measured before NAC significantly varies according to phenotypes; in particular, it is greater among the HR− and HR−/HER2+ tumors compared to other subgroups, which implies a correlation between such parameter and the biological characteristics of individual lesions (25). A previous review work concluded for insufficient evidence on the effectiveness of pre-NAC ADC in the prediction of pCR; yet, such review was limited by the inclusion of several underpowered studies and by conflicting definitions of response to therapy (9). Li and coworkers defined the response to therapy as based on the clinical evaluation findings (26) while Park and coworkers based their definition of response on the RECIST criteria (27); despite these differences, both reported that pre-NAC average ADC associated with sensitivity to chemotherapy and to the responder status. Another study was concordant (23). Yet, they did not perform any analysis by tumor phenotypes. Indeed, when the analysis is limited to the overall population of breast cancer patients undergoing NAC, the majority of the studies are concordant in denying any association between pre-treatment ADC and response to treatment (7,17,28,29).
On the other hand, when the analysis moves into subgroups by phenotypes, a different scenario appears. In 108 patients, Richard and coworkers evidenced that in TN tumors, average pre-treatment ADC value was significantly lower in cases who were going to show complete response (P=0.047) (29). Interestingly, in the HR−/HER2+ subpopulation, a trend towards lower mean pre-NAC ADC was observed; still, significance was not attained (P=0.14) probably due to insufficient sample size. Concordantly, no meaningful difference emerged in mean ADC for the HR+/HER2+ and HR+/HER2− subpopulation, which also tended to show greater average ADC value among responders. In a larger population (N=225) previously analyzed by our group (7), we disclosed not only significantly lower mean pre-treatment ADC among responders in the TN and HR−/HER2+ cohorts, but also adequate and statistically meaningful ROC AUCs for the same subgroups (AUC =0.766 and P=0.013 for the TN; AUC =0.813 and P=0.03 for the HR−/HER2+). Inadequate diagnostic performance was observed for the HR+/HER2− and HR+/HER2+ cases. Again, the biological features of the lesion seem to deeply impact the diagnostic performance of MRI.
Conclusions
We have addressed the current literature illustrating the impact of tumor phenotype on the diagnostic performance of MRI. Given a global concordance of results, we believe that the available evidence supports the idea of adapting the diagnostic strategy according to tumor phenotype. In such perspective, knowledge of phenotype may guide the interpretation of imaging and should be declined according to the concerned diagnostic step. Specifically, in pre-NAC MRI calculation of the ADC value of the lesion may help in the prediction of pCR only in the TN and HR-HER2+ lesions. In post-NAC MRI, the morphological criteria are most accurate in TN and HR−/HER2+ lesions, where ADC calculation does not seem to offer a significant advantage. On the contrary, in the same post-NAC MRI, the coupling of morphological aspects (RECIST) with a criterion based on ADC value variation across NAC in the HR+ subgroups (i.e., HR+/HER2− and HR+/HER2+), might fill the diagnostic gap associated with the use of RECIST alone. Generally speaking, the expression of HER2 seem to facilitate the diagnostic capabilities of MRI; yet, the expression of HRs seems to play a stronger disadvantageous effect on accuracy. In fact, the HR+/HER2+ lesions behave, with respect to diagnostic performance of MRI, more closely to HR+/HER2− lesions than to HR−/HER2+ cases. Phenotype-specific interpretation of MRI-derived information might represent a further step to personalize the knowledge of cancer of individual patients, and to tailor the therapeutic management.
In conclusion, the radiologist should keep in mind the tumor phenotype at different stages (diagnosis and evaluation of response to treatment). Knowledge of disease should therefore precede any technological advancement.
Acknowledgments
Funding: None.
Footnote
Provenance and Peer Review: This article was commissioned by the editorial office, Translational Cancer Research for the series “Update of Current Evidences in Breast Cancer Multidisciplinary Management”. The article has undergone external peer review.
Conflicts of Interest: All authors have completed the ICMJE uniform disclosure form (available at http://dx.doi.org/10.21037/tcr.2017.09.46). The series “Update of Current Evidences in Breast Cancer Multidisciplinary Management” was commissioned by the editorial office without any funding or sponsorship. GF served as the unpaid Guest Editor of the series and serves as an unpaid editorial board member of Translational Cancer Research from Nov 2016 to Dec 2018. The authors have no other conflicts of interest to declare.
Ethical Statement: The authors are accountable for all aspects of the work in ensuring that questions related to the accuracy or integrity of any part of the work are appropriately investigated and resolved.
Open Access Statement: This is an Open Access article distributed in accordance with the Creative Commons Attribution-NonCommercial-NoDerivs 4.0 International License (CC BY-NC-ND 4.0), which permits the non-commercial replication and distribution of the article with the strict proviso that no changes or edits are made and the original work is properly cited (including links to both the formal publication through the relevant DOI and the license). See: https://creativecommons.org/licenses/by-nc-nd/4.0/.
References
- Davidson NE, Morrow M. Sometimes a great notion--an assessment of neoadjuvant systemic therapy for breast cancer. J Natl Cancer Inst 2005;97:159-61. [Crossref] [PubMed]
- Gralow J. Evolving role of bisphosphonates in women undergoing treatment for localized and advanced breast cancer. Clin Breast Cancer 2005;5:S54-62. [Crossref] [PubMed]
- Mathew J, Asgeirsson KS, Cheung KL, et al. Neoadjuvant chemotherapy for locally advanced breast cancer: a review of the literature and future directions. Eur J Surg Oncol 2009;35:113-22. [Crossref] [PubMed]
- Rastogi P, Anderson SJ, Bear HD, et al. Preoperative chemotherapy: updates of National Surgical Adjuvant Breast and Bowel Project Protocols B-18 and B- 27. J Clin Oncol 2008;26:778-85. [Crossref] [PubMed]
- Marinovich ML, Houssami N, Macaskill P, et al. Meta-analysis of magnetic resonance imaging in detecting residual breast cancer after neoadjuvant therapy. J Natl Cancer Inst 2013;105:321-33. [Crossref] [PubMed]
- Bufi E, Belli P, Di Matteo M, et al. Effect of breast cancer phenotype on diagnostic performance of MRI in the prediction to response to neoadjuvant treatment. Eur J Radiol 2014;83:1631-8. [Crossref] [PubMed]
- Bufi E, Belli P, Costantini M, et al. Role of the Apparent Diffusion Coefficient in the Prediction of Response to Neoadjuvant Chemotherapy in Patients With Locally Advanced Breast Cancer. Clin Breast Cancer 2015;15:370-80. [Crossref] [PubMed]
- Lindenberg MA, Miquel-Cases A, Retèl VP, et al. Imaging performance in guiding response to neoadjuvant therapy according to breast cancer subtypes: A systematic literature review. Crit Rev Oncol Hematol 2017;112:198-207. [Crossref] [PubMed]
- Prevos R, Smidt ML, Tjan-Heijnen VC, et al. Pre-treatment differences and early response monitoring of neoadjuvant chemotherapy in breast cancer patients using magnetic resonance imaging: a systematic review. Eur Radiol 2012;22:2607-16. [Crossref] [PubMed]
- van Persijn van Meerten EL, Gelderblom H, Bloem JL. RECIST revised: implications for the radiologist. A review article on the modified RECIST guideline. Eur Radiol 2010;20:1456-67. [Crossref] [PubMed]
- McGuire KP, Toro-Burguete J, Dang H, et al. MRI staging after neoadjuvant chemotherapy for breast cancer: does tumor biology affect accuracy? Ann Surg Oncol 2011;18:3149-54. [Crossref] [PubMed]
- Rodenhuis S, Mandjes IA, Wesseling J, et al. A simple system for grading the response of breast cancer to neoadjuvant chemotherapy. Ann Oncol 2010;21:481-7. [Crossref] [PubMed]
- Loo CE, Straver ME, Rodenhuis S, et al. Magnetic resonance imaging response monitoring of breast cancer during neoadjuvant chemotherapy: relevance of breast cancer subtype. J Clin Oncol 2011;29:660-6. [Crossref] [PubMed]
- Kuzucan A, Chen JH, Bahri S, et al. Diagnostic performance of magnetic resonance imaging for assessing tumor response in patients with HER2- negative breast cancer receiving neoadjuvant chemotherapy is associated with molecular biomarker profile. Clin Breast Cancer 2012;12:110-8. [Crossref] [PubMed]
- De Los Santos JF, Cantor A, Amos KD, et al. Magnetic resonance imaging as a predictor of pathologic response in patients treated with neoadjuvant systemic treatment for operable breast cancer. Translational Breast Cancer Research Consortium trial 017. Cancer 2013;119:1776-83. [Crossref] [PubMed]
- De Los Santos J, Bernreuter W, Keene K, et al. Accuracy of breast magnetic resonance imaging in predicting pathologic response in patients treated with neoadjuvant chemotherapy. Clin Breast Cancer 2011;11:312-9. [Crossref] [PubMed]
- Fangberget A, Nilsen LB, Hole KH, et al. Neoadjuvant chemotherapy in breast cancer-response evaluation and prediction of response to treatment using dynamic contrast-enhanced and diffusion-weighted MR imaging. Eur Radiol 2011;21:1188-99. [Crossref] [PubMed]
- Chen JH, Bahri S, Mehta RS, et al. Breast cancer: evaluation of response to neoadjuvant chemotherapy with 3.0-T MR imaging. Radiology 2011;261:735-43. [Crossref] [PubMed]
- Moon HG, Han W, Ahn SK, et al. Breast cancer molecular phenotype and the use of HER2-targeted agents influence the accuracy of breast MRI after neoadjuvant chemotherapy. Ann Surg 2013;257:133-7. [Crossref] [PubMed]
- Hayashi Y, Takei H, Nozu S, et al. Analysis of complete response by MRI following neoadjuvant chemotherapy predicts pathological tumor responses differently for molecular subtypes of breast cancer. Oncol Lett 2013;5:83-9. [Crossref] [PubMed]
- Santamaría G, Bargalló X, Fernández PL, et al. Neoadjuvant Systemic Therapy in Breast Cancer: Association of Contrast-enhanced MR Imaging Findings, Diffusion-weighted Imaging Findings, and Tumor Subtype with Tumor Response. Radiology 2017;283:663-72. [Crossref] [PubMed]
- Belli P, Costantini M, Ierardi C, et al. Diffusion-weighted imaging in evaluating the response to neoadjuvant breast cancer treatment. Breast J 2011;17:610-9. [Crossref] [PubMed]
- Iacconi C, Giannelli M, Marini C, et al. The role of mean diffusivity (MD) as a predictive index of the response to chemotherapy in locally advanced breast cancer: a preliminary study. Eur Radiol 2010;20:303-8. [Crossref] [PubMed]
- Li X, Dawant BM, Welch EB, et al. A nonrigid registration algorithm for longitudinal breast MR images and the analysis of breast tumor response. Magn Reson Imaging 2009;27:1258-70. [Crossref] [PubMed]
- Martincich L, Deantoni V, Bertotto I, et al. Correlations between diffusion- weighted imaging and breast cancer biomarkers. Eur Radiol 2012;22:1519-28. [Crossref] [PubMed]
- Li XR, Cheng L, Liu M, et al. DW-MRI ADC values can predict treatment response in patients with locally advanced breast cancer undergoing neoadjuvant chemotherapy. Med Oncol 2012;29:425-31. [Crossref] [PubMed]
- Park SH, Moon WK, Cho N, et al. Diffusion-weighted MR imaging: pretreatment prediction of response to neoadjuvant chemotherapy in patients with breast cancer. Radiology 2010;257:56-63. [Crossref] [PubMed]
- Woodhams R, Kakita S, Hata H, et al. Identification of residual breast carcinoma following neoadjuvant chemotherapy: diffusion-weighted imaging-- comparison with contrast-enhanced MR imaging and pathologic findings. Radiology 2010;254:357-66. [Crossref] [PubMed]
- Richard R, Thomassin I, Chapellier M, et al. Diffusion-weighted MRI in pretreatment prediction of response to neoadjuvant chemotherapy in patients with breast cancer. Eur Radiol 2013;23:2420-31. [Crossref] [PubMed]