Role of pharmacokinetic modeling and simulation in precision dosing of anticancer drugs
Introduction
In drug development, late phase clinical trials often aim to establish uniform dosing, balancing efficacy and toxicity, across the patient population from a limited set of proposed dosage schemesJeny (1). Dosing of anticancer drugs has traditionally been based on body surface area (BSA) under the assumption that there is a relationship between BSA and clearance (CL) or volume of distribution (Vd). However, this relationship is in many instances poor and may therefore not accurately reflect the change in drug exposure seen across the population (2-5), meaning variability in drug exposure may remain high at the established dosage regimen (5). This is particularly true when the drug is dosed in a more diverse patient population in clinical practice, such as: complex drug-drug interactions (DDIs), pediatric patients, and renally/hepatically impaired or other special populations (6). Explicit dosage recommendations are often absent from the drug label for most special populations at the time of approval (7). These factors contribute to variable clinical practices, where clinicians are challenged to make decisions based on experience and the many times limited literature. Patients with multiple comorbidities/co-medication are therefore at risk of suboptimal pharmacotherapy that may lead to unacceptable levels of toxicity or reduced efficacy (6,8,9). Model-informed precision dosing (MIPD) provides a quantitative framework for achieving the accurate dose for the individual patient through statistical and/or mathematical modeling, such as pharmacokinetic (PK) modeling, by accounting for inter-individual variability (IIV), and other factors that lead to variable drug exposure and/or pharmacodynamic (PD) response (10).
Here we examine the current state of PK modeling in dose individualization of anticancer drugs. The comparative analysis presented here was based on a sample of 393 peer-reviewed publications on PK modeling in oncology (see Table S1, in supplementary appendix available online). The dataset should not be considered an exhaustive list of the abundant literature on PK modeling in oncology. Many arguments on precision dosing presented here are part of a much broader discussion on MIPD across therapeutic areas (7,10-12).
The case for MIPD in oncology
The traditional resolution to dose optimization in special populations/DDIs is to carry out dedicated clinical studies. This is however not always feasible in oncology due to patient recruitment issues around dosing drugs in vulnerable populations or patients outside the indicated treatment group (13). Statistical nonlinear-mixed effects (NLME) modeling (population-PK/PD modeling, or pop-PK/PD) aims to describe the IIV in PK parameters using compartmental and increasingly mechanistic models. Physiologically-based PK (PBPK) modeling and simulation (M&S), attribute physiological meaning to PK models by mimicking physiology (inter-compartmental CLs informed by blood flows, volumes based on organ/tissue volumes etc.) in an attempt to better understand the processes that determine drug ADME (absorption, distribution, metabolism and excretion). The combined approach (“middle-out”) accommodates physiological models where model parameters may account for observed IIV in the population sample (see Figure 1) (14). Pop-PK/PD and PBPK M&S have gained increasing acceptance in pharmaceutical research and development (R&D) and by regulatory agencies over the last couple of decades to a point where dedicated clinical trials may be substituted/supplemented by modeling, foremost interpolating the effect of metabolic DDIs (15). It is anticipated that M&S will gain further utility over the coming years as confidence is built in other areas of application, both in pharmaceutical R&D, regulatory submission and clinical practice for dose individualization (7,10,12).
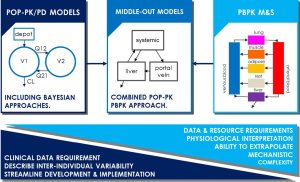
Dose individualization, personalized dosing or precision dosing, may be considered part of the well-recognized paradigm of precision medicine. Precision medicine pursues to personalize prevention, diagnostics and optimal treatment of disease based on individual patient characteristics, e.g., genotyping, renal function and other biomarkers (16). Similarly, precision dosing strives to account for between-patient variability in drug exposure and response to optimize dosing for the individual. This is not a novel idea, carboplatin is the perhaps most famous example in oncology, seeing early adoption of renal function guided dosing (Calvert et al. formula) to reduce the risk of hematological toxicity (17). Similarly, PK-based dose adjustment, using therapeutic drug monitoring (TDM), of 5-fluorouracil (5-FU) was shown to produce superior treatment response and reduced toxicity as compared to BSA-guided dosing alone in metastatic colorectal cancer (18).
Precision dosing in cancer therapy is attractive for several reasons. Many anticancer drugs display narrow therapeutic indices, where suboptimal therapy may lead to severe patient outcomes. Clinical study participant recruitment issues accentuates the difficulty of patient recruitment in dedicated clinical trials for special populations and is perhaps part of the reason (as well as accelerated approvals) why there has been an above average adoption of PBPK M&S for new drug applications (NDAs) in oncology to the U.S. Food and Drug Administration (FDA) (19). The relatively high rate of non-responders in cancer treatment together with high cost of cancer therapies warrants alternative approaches to increasing patient benefit and cost-benefit; this may include more effective use of approaches that maximize treatment outcome, such as PK modeling and MIPD (20,21).
Application of PK modeling in oncology
Model-informed drug discovery and development has become established practice in the pharmaceutical industry over the past decades, where today it is employed across drug development to inform internal and regulatory decisions (15,22). In early discovery and pre-clinical development, modeling is used to inform candidate selection, ADME characterization, translation of exposure and effect and more, this includes pop-PK/PD, PBPK and more mechanistic systems pharmacology/biology approaches. Pop-PK/PD is widely used in clinical development to investigate efficacy, dose selection and dose bridging. PBPK M&S is used clinically for predicting metabolic DDIs, impact of genetic polymorphism, biopharmaceutics effects and extrapolating to special populations (22).
Analysis of peer-reviewed publications using PK modeling in oncology, based on the modeling approach (see Figure 2), showed that a majority of studies employed population-based approaches in their data analysis (75%), a subset of these include traditional pop-PK (45%), pop-PK/PD (14%), Bayesian pop-PK (10%) and semi-mechanistic pop-PK/PD (6%). PBPK M&S accounted for 8% of identified studies. In terms of areas of application of PK modeling (see Figure 3), the most prominent area of application was investigation of covariates (49%) to account for IIV in PK. This was followed by studies investigating dosing issues (22%), including dose finding and practice based dosing issues. The most studied special populations included pediatric patients (13%), hepatic (3%) and renal impairment (2%). Other investigated special populations included: pregnancy, elderly, and more. Other areas of investigation included: toxicity (18%), dose/PK-efficacy studies (response: 8%), metabolite kinetics (8%), metabolic/transporter genotype/phenotype (6%), DDIs (5%), limited sampling strategies (5%) and more.
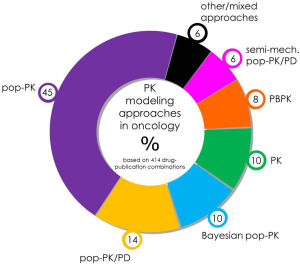
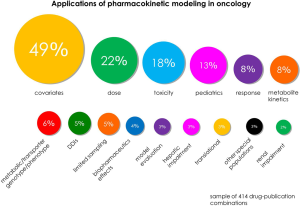
In PBPK M&S, predictions of metabolic DDIs and extrapolation to special populations were the perhaps most prominent area of research. This was consistent with common areas of application seen in regulatory submissions where predictions of DDIs tend to dominate due to more well-established body of evidence to support PBPK, guidelines and regulatory acceptance (15,19). There is in other words wide application of PK modeling to address critical questions in dose individualization of oncology drugs.
Population PKs and covariate analysis to aid precision dosing in oncology
Pop-PK/PD aims to describe the observed IIV in drug exposure and response for a given population sample. The method allows estimation of the population mean (θ) and IIV (η) of PK/PD parameters and the remaining residual, or unexplained, variability (ε). The approach allows interpolation of drug exposure and response over the observed parameter space through identification of covariates (demographics, genetic polymorphism and other pathophysiological variables). For example, covariates can be included as either dichotomous {Eq. [1]} or continuous effects {Eq. [2]} on PK parameters, e.g., (23):
Where mean effect of the nth covariate (θCov,n) and IIV of the jth parameter of the ith individual (ηj,i) on population mean (θj) determines the individual parameter estimate for the jth parameter (θj,i), Covi is the individual observed covariate and Cov the central tendency of the sample population. Significant covariates account for IIV in exposure, meaning that a combined PK-covariate model can forecast individual exposure based on individual biomarker data prior to dosing and refine predictions following sparse PK sampling or TDM. This makes for a powerful tool for precision dosing. Covariate significance can be determined using, e.g., step-wise inclusion based on a predefined statistical criterion (post-hoc ρ-value testing for difference in objective function), or alternative approaches. Pop-PK-covariate modeling has been successfully employed for oncology drugs to individualize dosing, as included in drug labelling (24,25). Further, there are a number of examples of pop-PK-covariate models that have been used to address dosing issues in clinical practice with some success (see section “PK modeling of anticancer drugs in healthcare”).
Figure 4 shows identified PK covariates for anticancer drugs based on the peer-reviewed literature. The most commonly included covariates were bodyweight (50% of drugs in sample set) and other demographic data [sex (28%), BSA (26%) and age (21%)]. Other common covariates included biomarkers related renal function [creatinine CL (19%), serum creatinine (7%) and estimated glomerular filtration rate (5%)], drug-binding plasma proteins [albumin (17%) and α1-acid glycoprotein (AAG, 2%)], cancer type (14%) and concomitant treatment (11%). Other biomarkers of liver function were also reasonably prominent as model covariates, e.g., alanine amino transferase (ALT, 9%), aspartate amino transferase (AST, 6%) and alkaline phosphatase (ALK, 5%) including more. Metabolic genotyping was included for 7% of drugs.
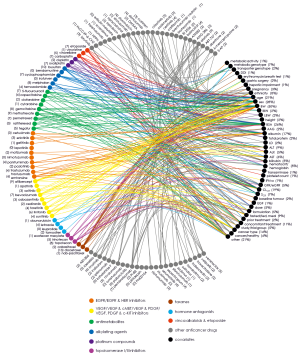
It has been recognized that there is a disparity between the wealth of covariates identified in the literature and the limited number pertaining to the dosage recommendation in the drug labels of oncology drugs (25). Figure 5 shows factors affecting explicit dose recommendations in the FDA label for selected drugs compared to additional identified PK covariates in the literature. There are many potential explanations for this: covariate selection can be biased (insufficient power, collinearity, etc.), not all statistically significant covariates are clinically relevant, covariates may for example be of obscure meaning and have little physiological/pharmacological relevance (25,32). Further, the identification of significant but low-effect covariates may have little clinical implication. Here, cut-off points have been proposed where covariates may be considered clinically relevant if they explain at least 20% to 30% of IIV (25,33). A lack of communication of research between academia and industry may also affect the difference in the adoption of covariates, suggesting some scope for further individualization of dosing of oncology drugs based on disseminated research (25).
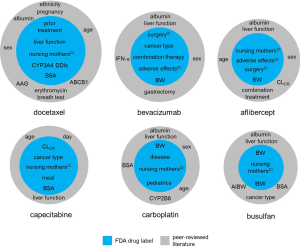
PBPK modeling to inform individualized dosing of anticancer drugs
By assigning physiological meaning to model parameters, PBPK M&S offers a method for quantitative extrapolation of drug exposure from in vitro to in vivo (IVIVE), between species, across populations and for metabolic/transporter DDIs. In oncology, PBPK M&S has been used extensively for the prediction of DDIs, special populations (renal/hepatic impairment and pediatrics) and biopharmaceutics effects (absorption, formulation, food effects). In fact, some of the earliest examples of PBPK M&S in oncology can be traced back to modeling of chemotherapy agents in the 1970s (34).
There are several factors that explain the wide usage of PBPK in oncology: ethical/safety or recruitment issues, many oncology drugs exhibit narrow therapeutic indices and/or pose risks of severe toxicity and may therefore require more consideration for precision dosing, many anticancer drugs are carried forward through accelerated regulatory approval meaning that studies that have not been carried out in timely fashion may be substituted by PBPK M&S (19). Numerous examples of PBPK M&S of oncology drugs exist in the literature, including for: pediatrics (35-37), biopharmaceutics effects (38), renal impairment (39-41), hepatic impairment (42), metabolic phenotypes/genotypes (43,44) and adherence (44) metabolic/transporter DDIs (45-49), with more examples available in FDA drug labels (50). The current view of FDA regarding PBPK-informed dosing, is that sufficient evidence exists to employ verified models for the prediction of metabolic DDIs where the drug is the victim substrate (50). For special populations and biopharmaceutics effects, the jury is still out in the absence of more evidence to support the ability to prospectively and quantitatively predict these effects (50). Here we present selected case examples to illustrate the usefulness of PBPK M&S for dose individualization of anticancer drugs.
Case examples
Ibrutinib—metabolic DDIs
Ibrutinib (Bruton’s tyrosine kinase inhibitor; Imbruvica®) was granted accelerated approval by the FDA in 2013 (51). The drug is given orally and undergoes extensive first-pass metabolism, mainly via CYP3A4 and to a lesser extent by CYP2D6, whilst undergoing minimal renal CL. Ketoconazole inhibited 96% of ibrutinib’s metabolism in human liver microsomes. A PBPK model was developed to evaluate CYP3A4 DDIs in healthy volunteers with ibrutinib as the victim. The model was validated against clinical DDI studies of ibrutinib in the presence of ketoconazole (strong inhibitor) and rifampin (strong inducer). The PBPK model was then used to interpolate DDI effects of mild, moderate and strong CYP3A4 inducers and inhibitors and used to inform dose guidance in the drug label (52,53).
Sonidegib—bridging DDIs to cancer patients
Sonidegib (Odomzo®) is an oral anticancer agent for the treatment of locally advanced basal cell carcinoma. The drug displays low oral bioavailability. In vitro drug metabolism studies and clinical DDI trials in the presence of ketoconazole and rifampin were carried to elucidate the metabolic contribution of sonidegib elimination. Co-administration of ketoconazole led to a 2.25-fold increase in area under the curve (AUC), whereas rifampin produced a 72% reduction in exposure. A PBPK model was developed to bridge the DDI data from healthy volunteers to cancer patients at the ratified dose, to extrapolate to steady state effects and interpolate the effect of moderate/weak CYP3A inhibition/induction. The simulation study showed the effect of the DDIs to be slightly reduced in cancer patients compared to healthy and the interaction magnitude to increase at steady state dosing of sonedegib. The simulated DDI magnitudes and alternative dosing schedules informed dosing recommendations provided in the FDA drug label (54). The study demonstrates the utility of using PBPK M&S to bridge the effect of metabolic DDIs from healthy volunteers to cancer patients.
Alectinib—biopharmaceutics effects
Alectinib [selective anaplastic lymphoma kinase (ALK) inhibitor; Alecenza®] underwent accelerated regulatory approval in 2015 because of likely clinical benefit in treating ALK-positive non-small cell lung cancer (55). The drug is a lipophilic basic (pKa ~6–7) displaying poor solubility and moderate oral bioavailability. As alectinib is given orally the pH-dependent solubility may be indicative of potential impact of biopharmaceutics effects. Parrott and co-workers developed a PBPK model to prospectively evaluate food effects and increased gastric pH with esomeprazole. The model predicted a positive food effect and a lack of impact of co-administration of esomeprazole. This was later confirmed in clinical trials, although the magnitude of the food effect was not accurately predicted, potentially due to excipient effects. The absorption model was further refined following confirmatory clinical studies and used to inform dose recommendations on timing of alectinib administration in relation to food intake. Authors stated that these finds were used to inform drug labeling (38).
Docetaxel—pediatric dose bridging
Docetaxel (taxane anticancer drug) is extensively metabolized by CYP3A4, substrate to the efflux transporter P-glycoprotein (P-gp), and active hepatic uptake transporters OATP1B1 and OATP1B3. The drug exhibits dose limiting toxicity in the form of neutropenia. In a retrospective study, a full PBPK model was developed based on adult data and validated against adult data in presence of ketoconazole. The PBPK model was then scaled to pediatrics in order to establish first dose in children assuming a similar exposure-response to adults with the same indication. A global approach was used where PBPK predictions were fitted using a pop-PK model in order to carry out optimization of sampling times. PBPK predictions of pediatric data gave a reasonable prediction with a 1.4-fold overprediction of CL (37). The study shows proof-of-concept for dose bridging from adults to pediatrics in oncology using PBPK M&S coupled with pop-PK, to inform first-dose-in-children and optimal sampling design.
Impact of physiology of oncology patients on exposure of anticancer drugs
A number of physiological changes have been reported in cancer patients that may impact the PKs of anticancer drugs (such as increased levels of inflammation and altered levels of plasma proteins) (56). With sufficient information, PBPK M&S can facilitate extrapolation of drug exposure to a more clinically relevant oncology population. Cheeti and co-workers developed an oncology PBPK model by altering sex, age, height and weight population distributions, levels of drug-binding plasma proteins (albumin and AAG), and hematocrit to investigate the effect of plasma protein binding on exposure of midazolam (CYP3A probe) and saquinavir (CYP3A probe highly bound to AAG) (57). A similar PBPK model for oncology patients developed by Thai and co-workers was demonstrated to better recover variability in PK profiles and CL of docetaxel compared to when physiology was assumed to remain the same as in healthy (37). In the absence of physiological data, PBPK modeling can also be used to make inferences about physiological parameters based on clinical PK data. Yoshida and co-workers developed a PBPK of irinotecan (topoisomerase I inhibitor) and its metabolites to explore different PK of the drug in cancer patients. Using parameter estimation, the authors could get an indication of the feasible parameter space of irinotecan’s CL pathways in cancer patients (58).
PK modeling of anticancer drugs in healthcare
Lately, there has been much debate how PK modeling can be used to aid precision dosing in clinical practice (10,11,59). In an earlier state of the art paper, we proposed a categorization to describe implementation of MIPD in healthcare based on current practices, these were: real-time implementation in healthcare systems, mechanistic modeling and extrapolation and model-derived dose banding (10).
Real-time implementation in healthcare systems refers to direct implementation of M&S in-line with healthcare, e.g., software tools and integration into electronic health records (EHR). The approach is particularly well-suited for treatments where continuous measurement, such as TDM, is carried out routinely throughout the therapy. Bayesian modeling approaches are particularly well-suited for this approach where feedback-control can be used to update prior parameter estimates and refine individual patient predictions as more data becomes available (10,11). Mechanistic or PBPK models are a powerful tool for allowing extrapolation to for example DDIs or special populations. Despite many examples of application to address dosing in special populations (60), there are few examples of the approach being evaluated in clinical practice (61). This may partly be due to PBPK’s reliance on drug-specific and physiological information and its inferior ability to describe IIV compared to pop-PK. This may however change over the coming years, the generation of new proteomic data (62), emergence of “middle-out” modeling (14), and Bayesian PBPK M&S (63) certainly makes PBPK an increasingly viable approach in precision dosing. Model-derived dose banding refers to the use of PK models to develop dosing strategies based on clinically relevant covariates identified during the data analysis. This is perhaps the most practical approach although it potentially may offer less scope for dose individualization compared to other model-based approaches (64-66). Based on previous experiences, work streams have been proposed for how to develop these model-based approaches from conception to implementation in clinical practice. The proposed necessary steps to prove clinical effectiveness of MIPD include: model development, internal validation—to diagnose model misspecifications, external validation—to test performance against a different but related population sample, prospective clinical evaluation—to test the performance of the model-informed approach compared to standard practice, and an implementation phase—for integration into clinical practice (10,67,68). Here follow examples of PK modeling applied to answer clinically relevant dosing issues in oncology (in addition, see Table 1). These illustrate both utility and concepts for clinical evaluation and practical implementation in a healthcare.
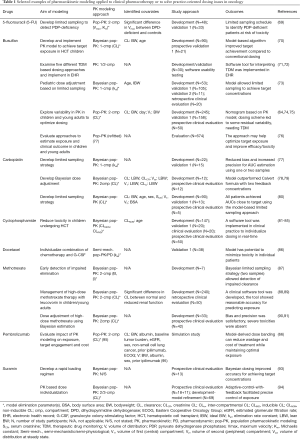
Full table
Case examples
5-FU—metabolic phenotyping
Due to the risk of severe toxicity and a relatively narrow therapeutic index, prediction of toxicity has been widely sought for treatment using thymidylate synthase inhibitor 5-FU (see Table S1, supplementary appendix). The drug undergoes metabolism by dihydropyrimidine dehydrogenase (DPD), where DPD deficiency has been linked to an increased risk for toxicity (95). In a retrospective study, van Kuilenburg and co-workers developed a Bayesian pop-PK model, based on population sample of DPD deficient patients and controls. The model described 5-FU CL using nonlinear Michaelis-Menten PK, where DPD deficient patients displayed a 40% reduction in maximum velocity (Vmax). Using a limited sampling strategy, the DPD phenotype could be determined based on Bayesian estimation of individual Vmax, AUC or terminal half-life to anticipate the risk of toxicity (69). The approach potentially offers advantages to genotyping where misclassification can occur due to discrepancies in genotype and phenotype.
Carboplatin—Bayesian forecasting of drug exposure
Despite being safer than cisplatin, carboplatin displays dose-limiting bone marrow toxicity. Duffull and co-workers developed a bioanalytical method for measuring carboplatin serum concentrations and a Bayesian pop-PK model with covariates lean bodyweight (LBW) and creatinine CL on CL, and LBW on volumes and inter-compartmental CL based on data from 12 ovarian cancer patients (78). The model was tested prospectively against the Calvert et al. formula in an additional 12 patients over two courses. During the first treatment course, the Calvert formula produced less bias compared to the population methods. Following two feedback concentrations the Bayesian method showed superior accuracy for AUC predictions. It was recognized that an updated model may produce better accuracy (79). This was perhaps one of the earliest examples incorporating patient data to update individual priors for prospective predictions (78,79).
Busulfan—strategies for healthcare implementation
Busulfan (alkylating agent) is widely used in combination with cyclophosphamide for conditioning prior to hematopoietic stem cell transplantation. The narrow therapeutic window of busulfan warrants TDM in pediatrics, where up to nine PK samples are taken over the course of a single dose to inform dose adjustments. Hence numerous PK models have been published to address dose individualization of busulfan (see Table S1, supplementary appendix).
Neely and co-workers, developed a nonparametric pop-PK model with age (described using a continuous polynomial function), and ideal body weight as covariates on CL and Vd based on a population sample of 53 pediatric patients. An additional two datasets consisting of a total of 116 pediatric patients were then used for model validation and Bayesian updating of priors. The final model was then incorporated into the BestDoseTM software platform and blindly tested against an additional 20 patients. The final model allowed target concentration achievement with only two blood samples per adjustment (73).
Abdel-Rahman and co-workers carried out a retrospective evaluation to examine the performance of five different TDM approaches (including non-compartmental and compartmental modeling) to estimate the dose of busulfan in pediatric cancer patients and found considerable discrepancies in dose recommendations (71). Due to observed inefficiencies in the workflow for TDM guided dosing at Children’s Mercy Hospital (Kansas City, MO), a clinician-oriented interface was developed around a compartmental model for dose optimization of busulfan based on TDM. The software allowed the use of either a one- or two-compartment model based on a series of quantitative goodness-of-fit criteria implemented within the software. The interface could be accessed through the EHR and was subject to usability testing by healthcare professionals. The research represents a significant step towards bringing MIPD into clinical practice and serves as a proof-of-concept for practical implementation. The authors noted that next steps will focus on quality assurance, predictive performance of the software tool and investigations of model-refinement, including inclusion of covariate effects (72).
Similarly, Long-Boyle and co-workers developed a pop-PK model for busulfan based on retrospective data of 90 pediatric and young adult patients. The final model (CL covariates: actual bodyweight and age) was then implemented in a user-friendly Microsoft Excel-based tool for guiding initial dosing in clinical practice and prospectively evaluated in 21 children. The healthcare tool showed significant improvement in attaining busulfan target concentrations compared to conventional dosing guidelines (70).
As previously mentioned, healthcare implementation of model-based precision dosing may take on different forms; where the previous examples detailed the process of incorporating real-time software tools in healthcare. A more pragmatic approach is the derivation of dose banding based on model optimized dosing regimens. Bartelink and co-workers, developed a two-compartment pop-PK model for busulfan with body weight as a covariate on CL based on 245 pediatric patients. The model was then used to derive a nomogram for dosing busulfan in clinical practice. The model was externally validated against an additional 158 adult and pediatric patients (64,74). A prospective study was carried out to assess the performance of the model-derived nomogram and the added value of TDM was carried out in 50 pediatric patients undergoing hematopoietic stem cell transplantation. The study concluded that following the model-derived dosing, variability was still significant and therefore TDM was still needed to inform dose optimization (75).
Cyclophosphamide—reduced toxicity and healthcare implementation
Cyclophosphamide (alkylating agent) is given in two doses over two consecutive days as myeloablative preparative treatment before hematopoietic stem cell transplantation. The drug is metabolized to carboxyethylphosphoramide mustard (CEPM) amongst others, where the CEPM is linked to liver toxicity and nonrelapse death. A Bayesian pop-PK model was developed for cyclophosphamide and its metabolites. The model was internally validated and incorporated into an open-source code to allow real-time dose adjustments between the two doses. This was a considerable logistical effort considering the time constraint for bioanalysis and model-derived dose recommendation. A clinical trial was carried out to test the performance of the model-based dosing approach. The approach led to an average total dose reduction of around 9% and a reduction in acute liver and kidney injury with similar overall survival (81-84,96). This case illustrates some of the logistic challenges of real-time implementation of MIPD in clinical practice.
Methotrexate—reduced toxicity and healthcare implementation
Methotrexate (antimetabolite) is used in the treatment of a number of cancers. Due to high IIV in exposure and the risk of toxicity in high-dose methotrexate treatments, the drug is routinely subject to TDM. Barrett and co-workers developed a pop-PK model on TDM data from 240 patients at the Children’s Hospital of Philadelphia (Philadelphia, PA), accounting for impaired CL by estimating the probability of a patient belonging to one of the two subgroups. A software dashboard was developed, consisting of a database of patient records, lab data and adverse events management system. The data was then used for Bayesian forecasting of exposure. A user-interface was designed that allowed viewing of TDM data, forecasting of exposure, the potential risk of toxicity and dose guidance (88). A retrospective study was carried out in 50 pediatric and young adult patients to test the ability of the dashboard to predict future toxicity events to allow earlier recommendations of leucovorin rescue therapy. The study concluded that the dashboard gave reasonably accurate predictions (precision of 12.9%, bias of 2.2%) and could have been used to initiate earlier rescue therapy in 16 of the studied patients, seven patients would have received a larger dose of leucovorin and 37 patients would have received the drug less often. The dashboard can support clinicians in monitoring for risk of toxicity and guide decision making of initiation of rescue therapy (89).
Pembrolizumab—minimizing excess drug wastage
Infused anticancer drugs are sold in vials with a set volume of the drug; however, dosing is often based on body size. This leads to excess drug volumes after dosing a patient which many times are being discarded at the cost of healthcare providers or insurers. It is estimated that the total cost incurred by wastage of the top 20 anticancer drugs amounted to USA dollar (USD) 1.8bn in 2016 in the U.S. alone (97). Pembrolizumab (programmed cell death protein 1 ligand antibody) is currently available as 50-mg vial size in the UK at a licensed dose of 2 mg/kg every 3 weeks. Ogungbenro and co-workers proposed a model-based approach to optimize dose banding to maximize target attainment and minimize wastage. Cost analysis showed that the model-derived dosing strategy could save 16% of the cost of drug treatment compared to dosing by bodyweight by reducing discarded excess volumes of the drug, without altering exposure significantly (66). The work provides an example of how modeling can improve cost-benefit of anticancer treatment.
MIPD in oncology: future challenges
It has been almost 50 years since the first model-based dosing strategies for dose individualization were proposed (98,99). PK modeling has come a long way in supporting dose selection and answering clinically relevant questions in oncology and other disease areas (10,11,59). While academic groups, in collaboration with healthcare professionals, are leading the way for model-based approaches to answer bedside dose individualization, pharmaceutical industry and regulatory agencies have made great strides in model-informed dose guidance in the drug label. It is thought that modeling will gain wider application in clinical practice over the coming years as precision medicine realizes its potential. For this to happen, there are a number of challenges that need to be met.
At the moment, there is a lack of clinical effectiveness, patient benefit and health economic evidence to support MIPD in healthcare. This is crucial for wider acceptance of MIPD in clinical care. Too many published modeling efforts are concluded following model development, indicating areas of clinical application. Without rigorous validation and clinical evaluation, these models will not see their full utility. Better coordination between academia, industry, healthcare, patient groups, and funding bodies are warranted to support implementation-based research in healthcare.
A prerequisite for precision dosing is the availability of multiple drug dose formulations. For MIPD to gain greater traction, some adjustments to pharmaceutical R&D would be required, with focus earlier in development of precision dosing. Recently, we illustrated how this could work using the “companion tool” approach (10), where a MIPD tool can be considered following candidate selection and developed alongside the drug. Precision dosing can facilitate the advancement of candidate drug that otherwise would be abandoned and there is therefore, in our opinion, great financial incentives to pursue this approach in pharmaceutical industry.
Oncology is an area with a lot to gain from PK modeling based dose individualization. There are however some specific challenges, such as the lack of exposure-effect/toxicity relationship for many new drugs coming to the market. This is of course a prerequisite for PK modeling to be meaningful. The concentration-effect relationship for monoclonal antibodies is poorly understood, where for many of these the dose-efficacy relationship found in clinical trials is flat (100,101). This suggests that current dosing of monoclonal antibodies may not be optimal from an efficacy-cost perspective. Here lies an optimization challenge that can reap financial benefits for healthcare providers and payers, and in the end aid pharmaceutical industry to improve cost-benefit.
Conclusions
Here we present the current state of PK modeling in precision dosing of anticancer drugs. We have illustrated, using published case examples, some of the potential benefits the approach may bring in terms of prospective dosage guidance for DDIs and in special populations, improved attainment of target drug concentration and reduced risk of toxicity, reducing wastage and scope for improved patient benefit and cost-benefit. While some great progress has been seen in implementation of model-informed dosage guidance in the drug labeling, a collaborative effort from regulators, industry and academia, the uptake has been modest in healthcare. Collaboration between academia, healthcare and industry together with greater financial support for applied research into patient benefit, cost-benefit and clinical effectiveness of model-based dosing approaches is warranted for wider adaption in healthcare.
Supplementary
Role of pharmacokinetic modeling and simulation in precision dosing of anticancer drugs
Methods
A literature search was carried out using PubMed (https://www.ncbi.nlm.nih.gov/pubmed; 1950–March 2017) to identify a sample set of publications including pharmacokinetic (PK) modeling of anticancer drugs. The inclusion criteria consisted of PK modeling of oncology drugs aimed at either predicting human exposure or based on clinical data. Studies based on pre-clinical data only or pharmacodynamic modeling only were excluded during screening of publications. To capture a broad variety of modeling applications in oncology the search terms included: “physiologically based pharmacokinetic”, “pharmacokinetic”, “PBPK”, “PKPD”, and “pharmacodynamic”, and “model”, “modeling”, and “modelling”, and “oncology”, “cancer”, “anti-cancer”, “tumor”, “tumor”, “anti-tumor” or “anti-tumor”, with filtering based on human species. The search yielded 1,749 publications. Additional publications were identified through references in identified publications, review articles and key literature. The final dataset included 393 publications.
The identified set of publications was categorized based on main and secondary study aims, including:
- Toxicity: adverse effects/toxicity;
- Bioequivalence: PK modeling of bioequivalence studies;
- Biopharmaceutics effects: biopharmaceutics effects, other than food and formulation effects;
- Covariates: PK covariates, or otherwise included covariates in the model;
- DDIs: metabolic/transporter drug-drug interactions;
- Dose: investigating multiple doses, optimal dose, or dose individualization;
- Efficacy: drug efficacy/response;
- Ethnicity: differences in PK based on ethnicity;
- Evaluation/validation: assessment or validation of PK model performance;
- Food effects: impact of prandial state and/or meal properties on PK;
- Formulation: impact of formulation on PK;
- Genotype: metabolic/transporter genotype (and α1-acid glycoprotein genotype) effects on PK;
- Surgery: impact of surgery on PK (including gastric and cytoreductive surgery);
- Hepatic impairment: PK in hepatically impaired subjects, assessing impact of hepatic impairment on PK;
- Investigational: main focus to investigate PK of the selected drug;
- Limited: optimal limited sampling strategies;
- Metabolite: metabolite PK;
- Other special populations: PK in other special populations (including: elderly, renal replacement therapy and postmenopausal women);
- Pediatrics: pediatric drug exposure;
- Phenotype: metabolic/transporter phenotype effects on PK;
- Renal impairment: PK in renally impaired subjects, assessing impact of renal impairment on PK;
- Sex: impact of sex on PK.
Where applicable, identified PK covariates were recorded, either including the full covariate model or a summary of covariates and the PK parameter the effect was modeled on. Additional recording of information included: investigated drug, modeling approach used for development and/or application, and study population(s). Data was analyzed through graphical presentation.
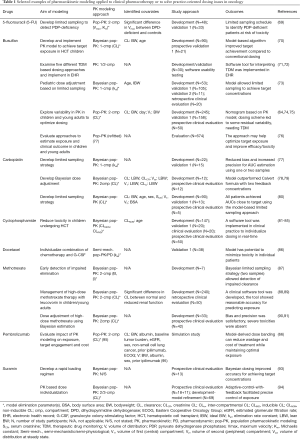
Full table
Acknowledgments
Funding: None.
Footnote
Provenance and Peer Review: This article was commissioned by the Guest Editors (Michael Sorich and Andrew Rowland) for the series “Precision dosing of targeted anticancer drugs” published in Translational Cancer Research. The article has undergone external peer review.
Conflicts of Interest: All authors have completed the ICMJE uniform disclosure form (available at http://dx.doi.org/10.21037/tcr.2017.09.14). The series “Precision dosing of targeted anticancer drugs” was commissioned by the editorial office without any funding or sponsorship. The authors have no other conflicts of interest to declare.
Ethical Statement: The authors are accountable for all aspects of the work in ensuring that questions related to the accuracy or integrity of any part of the work are appropriately investigated and resolved.
Open Access Statement: This is an Open Access article distributed in accordance with the Creative Commons Attribution-NonCommercial-NoDerivs 4.0 International License (CC BY-NC-ND 4.0), which permits the non-commercial replication and distribution of the article with the strict proviso that no changes or edits are made and the original work is properly cited (including links to both the formal publication through the relevant DOI and the license). See: https://creativecommons.org/licenses/by-nc-nd/4.0/.
References
- Orloff J, Douglas F, Pinheiro J, et al. The future of drug development: advancing clinical trial design. Nat Rev Drug Discov 2009;8:949-57. [PubMed]
- Felici A, Verweij J, Sparreboom A. Dosing strategies for anticancer drugs: the good, the bad and body-surface area. Eur J Cancer 2002;38:1677-84. [Crossref] [PubMed]
- Pinkel D. The use of body surface area as a criterion of drug dosage in cancer chemotherapy. Cancer Res 1958;18:853-6. [PubMed]
- Beumer JH, Chu E, Salamone SJ. Body-surface area-based chemotherapy dosing: appropriate in the 21st century? J Clin Oncol 2012;30:3896-7. [Crossref] [PubMed]
- Baker SD, Verweij J, Rowinsky EK, et al. Role of body surface area in dosing of investigational anticancer agents in adults, 1991-2001. J Natl Cancer Inst 2002;94:1883-8. [Crossref] [PubMed]
- Grimsrud KN, Sherwin CM, Constance JE, et al. Special population considerations and regulatory affairs for clinical research. Clin Res Regul Aff 2015;32:47-56. [Crossref] [PubMed]
- Jadhav PR, Cook J, Sinha V, et al. A proposal for scientific framework enabling specific population drug dosing recommendations. J Clin Pharmacol 2015;55:1073-8. [Crossref] [PubMed]
- van der Elst KC, Pereboom M, van den Heuvel ER, et al. Insufficient Fluconazole Exposure in Pediatric Cancer Patients and the Need for Therapeutic Drug Monitoring in Critically Ill Children. Clin Infect Dis 2014;59:1527-33. [Crossref] [PubMed]
- Launay-Vacher V, Janus N, Deray G. Renal insufficiency and cancer treatments. ESMO Open 2016;1:e000091 [Crossref] [PubMed]
- Darwich AS, Ogungbenro K, Vinks AA, et al. Why has model-informed precision dosing not yet become common clinical reality? lessons from the past and a roadmap for the future. Clin Pharmacol Ther 2017;101:646-56. [Crossref] [PubMed]
- Neely M. Scalpels not hammers: The way forward for precision drug prescription. Clin Pharmacol Ther 2017;101:368-72. [Crossref] [PubMed]
- Younis IR, Robert Powell J, Rostami-Hodjegan A, et al. Utility of Model Based Approaches for Informing Dosing Recommendations in Specific Populations: Report from the Public AAPS Workshop. J Clin Pharmacol 2017;57:105-9. [Crossref] [PubMed]
- Wong KM, Capasso A, Eckhardt SG. The changing landscape of phase I trials in oncology. Nat Rev Clin Oncol 2016;13:106-17. [Crossref] [PubMed]
- Tsamandouras N, Rostami-Hodjegan A, Aarons L. Combining the 'bottom up' and 'top down' approaches in pharmacokinetic modelling: fitting PBPK models to observed clinical data. Br J Clin Pharmacol 2015;79:48-55. [Crossref] [PubMed]
- Jamei M. Recent Advances in Development and Application of Physiologically-Based Pharmacokinetic (PBPK) Models: a Transition from Academic Curiosity to Regulatory Acceptance. Curr Pharmacol Rep 2016;2:161-9. [Crossref] [PubMed]
- Collins FS, Varmus H. A new initiative on precision medicine. N Engl J Med 2015;372:793-5. [Crossref] [PubMed]
- Calvert AH, Newell DR, Gumbrell LA, et al. Carboplatin dosage: prospective evaluation of a simple formula based on renal function. J Clin Oncol 1989;7:1748-56. [Crossref] [PubMed]
- Capitain O, Asevoaia A, Boisdron-Celle M, et al. Individual fluorouracil dose adjustment in FOLFOX based on pharmacokinetic follow-up compared with conventional body-area-surface dosing: a phase II, proof-of-concept study. Clin Colorectal Cancer 2012;11:263-7. [Crossref] [PubMed]
- Yoshida K, Budha N, Jin JY. Impact of physiologically based pharmacokinetic models on regulatory reviews and product labels: Frequent utilization in the field of oncology. Clin Pharmacol Ther 2017;101:597-602. [Crossref] [PubMed]
- Hait WN. Anticancer drug development: the grand challenges. Nat Rev Drug Discov 2010;9:253-4. [Crossref] [PubMed]
- Siddiqui M, Rajkumar SV. The High Cost of Cancer Drugs and What We Can Do About It. Mayo Clin Proc 2012;87:935-43. [Crossref] [PubMed]
- EFPIA MID3 Workgroup. Good Practices in Model-Informed Drug Discovery and Development: Practice, Application, and Documentation. CPT Pharmacometrics Syst Pharmacol 2016;5:93-122. [Crossref] [PubMed]
- Mould DR, Upton RN. Basic concepts in population modeling, simulation, and model-based drug development-part 2: introduction to pharmacokinetic modeling methods. CPT Pharmacometrics Syst Pharmacol 2013;2:e38 [Crossref] [PubMed]
- Williams PJ, Ette EI. The role of population pharmacokinetics in drug development in light of the Food and Drug Administration's 'Guidance for Industry: Population pharmacokinetics'. Clin Pharmacokinet 2000;39:385-95. [Crossref] [PubMed]
- Joerger M. Covariate pharmacokinetic model building in oncology and its potential clinical relevance. AAPS J 2012;14:119-32. [Crossref] [PubMed]
- FDA. Highlights of prescribing information (Taxotere®). 2015. Available online: https://www.accessdata.fda.gov/drugsatfda_docs/label/2015/020449s075lbl.pdf
- FDA. Highlights of prescribing information (Avastin®). 2016. Available online: https://www.accessdata.fda.gov/drugsatfda_docs/label/2016/125085s317lbl.pdf
- FDA. Highlights of prescribing information (Zaltrap®). 2016. Available online: https://www.accessdata.fda.gov/drugsatfda_docs/label/2016/125418s039lbl.pdf
- FDA. Highlights of prescribing information (Xeloda®). 2016. Available online: https://www.accessdata.fda.gov/drugsatfda_docs/label/2016/020896s039lbl.pdf
- FDA. Highlights of prescribing information [carboplatin (TEVA Pharmaceuticals USA)]. 2012. Available online: https://www.accessdata.fda.gov/drugsatfda_docs/label/2012/077139Orig1s016lbl.pdf
- FDA. Highlights of prescribing information (Busulfex®). 2016. Available online: https://www.accessdata.fda.gov/drugsatfda_docs/label/2016/020954s016lbl.pdf
- Ribbing J, Jonsson EN. Power, selection bias and predictive performance of the Population Pharmacokinetic Covariate Model. J Pharmacokinet Pharmacodyn 2004;31:109-34. [Crossref] [PubMed]
- Duffull SB, Wright DFB, Winter HR. Interpreting population pharmacokinetic-pharmacodynamic analyses - a clinical viewpoint. Br J Clin Pharmacol 2011;71:807-14. [Crossref] [PubMed]
- Chen HS, Gross JF. Physiologically based pharmacokinetic models for anticancer drugs. Cancer Chemother Pharmacol 1979;2:85-94. [Crossref] [PubMed]
- Walsh C, Bonner JJ, Johnson TN, et al. Development of a physiologically based pharmacokinetic model of actinomycin D in children with cancer. Br J Clin Pharmacol 2016;81:989-98. [Crossref] [PubMed]
- Diestelhorst C, Boos J, McCune JS, et al. Predictive performance of a physiologically based pharmacokinetic model of busulfan in children. Pediatr Hematol Oncol 2014;31:731-42. [Crossref] [PubMed]
- Thai HT, Mazuir F, Cartot-Cotton S, et al. Optimizing pharmacokinetic bridging studies in paediatric oncology using physiologically-based pharmacokinetic modelling: application to docetaxel. Br J Clin Pharmacol 2015;80:534-47. [Crossref] [PubMed]
- Parrott NJ, Yu LJ, Takano R, et al. Physiologically Based Absorption Modeling to Explore the Impact of Food and Gastric pH Changes on the Pharmacokinetics of Alectinib. AAPS J 2016;18:1464-74. [Crossref] [PubMed]
- Tan W, Yamazaki S, Johnson TR, et al. Effects of Renal Function on Crizotinib Pharmacokinetics: Dose Recommendations for Patients with ALK-Positive Non-Small Cell Lung Cancer. Clin Drug Investig 2017;37:363-73. [Crossref] [PubMed]
- Fujita K, Masuo Y, Okumura H, et al. Increased Plasma Concentrations of Unbound SN-38, the Active Metabolite of Irinotecan, in Cancer Patients with Severe Renal Failure. Pharm Res 2016;33:269-82. [Crossref] [PubMed]
- Lu C, Suri A, Shyu WC, et al. Assessment of cytochrome P450-mediated drug-drug interaction potential of orteronel and exposure changes in patients with renal impairment using physiologically based pharmacokinetic modeling and simulation. Biopharm Drug Dispos 2014;35:543-52. [Crossref] [PubMed]
- Emoto C, Fukuda T, Cox S, et al. Development of a Physiologically-Based Pharmacokinetic Model for Sirolimus: Predicting Bioavailability Based on Intestinal CYP3A Content. CPT Pharmacometrics Syst Pharmacol 2013;2:e59 [Crossref] [PubMed]
- Dickschen K, Willmann S, Thelen K, et al. Physiologically Based Pharmacokinetic Modeling of Tamoxifen and its Metabolites in Women of Different CYP2D6 Phenotypes Provides New Insight into the Tamoxifen Mass Balance. Frontiers in Pharmacology 2012;3:92. [Crossref] [PubMed]
- Dickschen KJ, Willmann S, Hempel G, et al. Addressing Adherence Using Genotype-Specific PBPK Modeling-Impact of Drug Holidays on Tamoxifen and Endoxifen Plasma Levels. Front Pharmacol 2017;8:67. [Crossref] [PubMed]
- Jing J, Nelson C, Paik J, et al. Physiologically Based Pharmacokinetic Model of All-trans-Retinoic Acid with Application to Cancer Populations and Drug Interactions. J Pharmacol Exp Ther 2017;361:246-58. [Crossref] [PubMed]
- Glenn KJ, Yu LJ, Reddy MB, et al. Investigating the effect of autoinduction in cynomolgus monkeys of a novel anticancer MDM2 antagonist, idasanutlin, and relevance to humans. Xenobiotica 2016;46:667-76. [Crossref] [PubMed]
- Posada MM, Bacon JA, Schneck KB, et al. Prediction of renal transporter mediated drug-drug interactions for pemetrexed using physiologically based pharmacokinetic modeling. Drug Metab Dispos 2015;43:325-34. [Crossref] [PubMed]
- Yamazaki S, Johnson TR, Smith BJ. Prediction of Drug-Drug Interactions with Crizotinib as the CYP3A Substrate Using a Physiologically Based Pharmacokinetic Model. Drug Metab Dispos 2015;43:1417-29. [Crossref] [PubMed]
- Mao J, Johnson TR, Shen Z, et al. Prediction of crizotinib-midazolam interaction using the Simcyp population-based simulator: comparison of CYP3A time-dependent inhibition between human liver microsomes versus hepatocytes. Drug Metab Dispos 2013;41:343-52. [Crossref] [PubMed]
- Pharmaceutical Science and Clinical Pharmacology Advisory Committee Meeting. March 15, 2017. Available online: https://www.fda.gov/AdvisoryCommittees/Calendar/ucm535513.htm
- National Cancer Institute. FDA Approval for Ibrutinib. November 19, 2013. Available online: https://www.cancer.gov/about-cancer/treatment/drugs/fda-ibrutinib
- Fowler S, Morcos PN, Cleary Y, et al. Progress in Prediction and Interpretation of Clinically Relevant Metabolic Drug-Drug Interactions: a Minireview Illustrating Recent Developments and Current Opportunities. Curr Pharmacol Rep 2017;3:36-49. [Crossref] [PubMed]
- de Zwart L, Snoeys J, De Jong J, et al. Ibrutinib Dosing Strategies Based on Interaction Potential of CYP3A4 Perpetrators Using Physiologically Based Pharmacokinetic Modeling. Clin Pharmacol Ther 2016;100:548-57. [Crossref] [PubMed]
- Einolf HJ, Zhou J, Won C, et al. A Physiologically-Based Pharmacokinetic Modeling Approach To Predict Drug-Drug Interactions of Sonidegib (LDE225) with Perpetrators of CYP3A in Cancer Patients. Drug Metab Dispos 2017;45:361-74. [Crossref] [PubMed]
- Administration USFD. FDA approves new oral therapy to treat ALK-positive lung cancer. December 11, 2015. Available online: https://www.fda.gov/newsevents/newsroom/pressannouncements/ucm476926.htm
- Coutant DE, Kulanthaivel P, Turner PK, et al. Understanding Disease-Drug Interactions in Cancer Patients: Implications for Dosing Within the Therapeutic Window. Clin Pharmacol Ther 2015;98:76-86. [Crossref] [PubMed]
- Cheeti S, Budha NR, Rajan S, et al. A physiologically based pharmacokinetic (PBPK) approach to evaluate pharmacokinetics in patients with cancer. Biopharm Drug Dispos 2013;34:141-54. [Crossref] [PubMed]
- Yoshida K, Maeda K, Kusuhara H, et al. Estimation of feasible solution space using Cluster Newton Method: application to pharmacokinetic analysis of irinotecan with physiologically-based pharmacokinetic models. BMC Syst Biol 2013;7:S3. [Crossref] [PubMed]
- Neely M, Jelliffe R. Practical, individualized dosing: 21st century therapeutics and the clinical pharmacometrician. J Clin Pharmacol 2010;50:842-7. [Crossref] [PubMed]
- Marsousi N, Desmeules JA, Rudaz S, et al. Usefulness of PBPK modeling in incorporation of clinical conditions in personalized medicine. J Pharm Sci 2017;106:2380-91. [Crossref] [PubMed]
- Marsousi N, Samer CF, Fontana P, et al. Coadministration of ticagrelor and ritonavir: Toward prospective dose adjustment to maintain an optimal platelet inhibition using the PBPK approach. Clin Pharmacol Ther 2016;100:295-304. [Crossref] [PubMed]
- Heikkinen AT, Lignet F, Cutler P, et al. The role of quantitative ADME proteomics to support construction of physiologically based pharmacokinetic models for use in small molecule drug development. Proteomics Clinical applications 2015;9:732-44. [Crossref] [PubMed]
- Krauss M, Burghaus R, Lippert J, et al. Using Bayesian-PBPK modeling for assessment of inter-individual variability and subgroup stratification. In Silico Pharmacol 2013;1:6. [Crossref] [PubMed]
- Bartelink IH, van Kesteren C, Boelens JJ, et al. Predictive performance of a busulfan pharmacokinetic model in children and young adults. Ther Drug Monit 2012;34:574-83. [Crossref] [PubMed]
- Krekels EH, Tibboel D, de Wildt SN, et al. Evidence-based morphine dosing for postoperative neonates and infants. Clin Pharmacokinet 2014;53:553-63. [Crossref] [PubMed]
- Ogungbenro K, Patel A, Duncombe R, et al. A rational approach to dose optimisation of pembrolizumab using cost analysis and pharmacokinetic modelling and simulation. J Clin Oncol 2016;34:abstr 9547.
- Ince I, de Wildt SN, Tibboel D, et al. Tailor-made drug treatment for children: creation of an infrastructure for data-sharing and population PK-PD modeling. Drug Discov Today 2009;14:316-20. [Crossref] [PubMed]
- Vinks AA, Derendorf H, Mouton JW. editors. Fundamentals of antimicrobial pharmacokinetics and pharmacodynamics. New York, USA: Springer, 2014.
- van Kuilenburg AB, Häusler P, Schalhorn A, et al. Evaluation of 5-fluorouracil pharmacokinetics in cancer patients with a c.1905+1G>A mutation in DPYD by means of a Bayesian limited sampling strategy. Clin Pharmacokinet 2012;51:163-74. [Crossref] [PubMed]
- Long-Boyle JR, Savic R, Yan S, et al. Population Pharmacokinetics of Busulfan in Pediatric and Young Adult Patients Undergoing Hematopoietic Cell Transplant: A Model-Based Dosing Algorithm for Personalized Therapy and Implementation Into Routine Clinical Use. Ther Drug Monit 2015;37:236-45. [Crossref] [PubMed]
- Abdel-Rahman SM, Casey KL, Garg U, et al. Intravenous busulfan dose individualization - impact of modeling approach on dose recommendation. Pediatr Transplant 2016;20:443-8. [Crossref] [PubMed]
- Abdel-Rahman SM, Breitkreutz ML, Bi C, et al. Design and Testing of an EHR-Integrated, Busulfan Pharmacokinetic Decision Support Tool for the Point-of-Care Clinician. Front Pharmacol 2016;7:65. [Crossref] [PubMed]
- Neely M, Philippe M, Rushing T, et al. Accurately Achieving Target Busulfan Exposure in Children and Adolescents With Very Limited Sampling and the BestDose Software. Ther Drug Monit 2016;38:332-42. [Crossref] [PubMed]
- Bartelink IH, Boelens JJ, Bredius RG, et al. Body weight-dependent pharmacokinetics of busulfan in paediatric haematopoietic stem cell transplantation patients: towards individualized dosing. Clin Pharmacokinet 2012;51:331-45. [Crossref] [PubMed]
- van Reij E, van Maarseveen E, Bartelink I, et al. Prospective Validation of a Busulfan Pharmacokinetic Model in Children: Therapeutic Drug Monitoring Remains of Utmost Importance to Optimize Outcomes of HCT. Biol Blood Marrow Transplant 2014;20:S211-56. [Crossref]
- Bartelink IH, Lalmohamed A, van Reij EM, et al. Busulfan Exposure Predicts Event Free Survival and Toxicity after Hematopoietic Cell Transplantation in Children And Young Adults: A Multicenter Retrospective Cohort Analysis. 42nd Annual Meeting of the European Society for Blood and Marrow Transplantation. 2016; Valencia, Spain. Nature Publishing Group, 2016;51:S14.
- Peng B, Boddy AV, Cole M, et al. Comparison of methods for the estimation of carboplatin pharmacokinetics in paediatric cancer patients. Eur J Cancer 1995;31A:1804-10. [Crossref] [PubMed]
- Duffull SB, Begg EJ, Robinson BA, et al. A sequential Bayesian algorithm for dose individualisation of carboplatin. Cancer Chemother Pharmacol 1997;39:317-26. [Crossref] [PubMed]
- Duffull SB, Robinson BA. Clinical pharmacokinetics and dose optimisation of carboplatin. Clin Pharmacokinet 1997;33:161-83. [Crossref] [PubMed]
- Chatelut E, Pivot X, Otto J, et al. A limited sampling strategy for determining carboplatin AUC and monitoring drug dosage. Eur J Cancer 2000;36:264-9. [Crossref] [PubMed]
- McDonald GB, McCune JS, Batchelder A, et al. Metabolism-based cyclophosphamide dosing for hematopoietic cell transplant. Clin Pharmacol Ther 2005;78:298-308. [Crossref] [PubMed]
- McCune JS, Batchelder A, Guthrie KA, et al. Personalized dosing of cyclophosphamide in the total body irradiation-cyclophosphamide conditioning regimen: a phase II trial in patients with hematologic malignancy. Clin Pharmacol Ther 2009;85:615-22. [Crossref] [PubMed]
- Salinger DH, McCune JS, Ren AG, et al. Real-time dose adjustment of cyclophosphamide in a preparative regimen for hematopoietic cell transplant: a Bayesian pharmacokinetic approach. Clin Cancer Res 2006;12:4888-98. [Crossref] [PubMed]
- Qiu R, Yao A, Vicini P, et al. Diminishing the risk of nonrelapse mortality in hematopoietic stem cell transplantation: Prediction of exposure to the cyclophosphamide metabolite carboxyethylphosphoramide mustard. Clin Pharmacol Ther 2004;76:270-80. [Crossref] [PubMed]
- McCune JS, Salinger DH, Vicini P, et al. Population Pharmacokinetics of Cyclophosphamide and Metabolites in Children With Neuroblastoma: A Report From the Children's Oncology Group. J Clin Pharmacol 2009;49:88-102. [Crossref] [PubMed]
- Vainas O, Ariad S, Amir O, et al. Personalising docetaxel and G-CSF schedules in cancer patients by a clinically validated computational model. Br J Cancer 2012;107:814-22. [Crossref] [PubMed]
- Monjanel-Mouterde S, Lejeune C, Ciccolini J, et al. Bayesian population model of methotrexate to guide dosage adjustments for folate rescue in patients with breast cancer. J Clin Pharm Ther 2002;27:189-95. [Crossref] [PubMed]
- Barrett JS, Mondick JT, Narayan M, et al. Integration of modeling and simulation into hospital-based decision support systems guiding pediatric pharmacotherapy. BMC Med Inform Decis Mak 2008;8:6. [Crossref] [PubMed]
- Dombrowsky E, Jayaraman B, Narayan M, et al. Evaluating performance of a decision support system to improve methotrexate pharmacotherapy in children and young adults with cancer. Ther Drug Monit 2011;33:99-107. [Crossref] [PubMed]
- Pignon T, Lacarelle B, Duffaud F, et al. Pharmacokinetics of high-dose methotrexate in adult osteogenic sarcoma. Cancer Chemother Pharmacol 1994;33:420-4. [Crossref] [PubMed]
- Pignon T, Lacarelle B, Duffaud F, et al. Dosage Adjustment of High-Dose Methotrexate Using Bayesian-Estimation: a Comparative-Study of 2 Different Concentrations at the End of 8-H Infusions. Ther Drug Monit 1995;17:471-8. [Crossref] [PubMed]
- Ahamadi M, Freshwater T, Prohn M, et al. Model-Based Characterization of the Pharmacokinetics of Pembrolizumab: A Humanized Anti-PD-1 Monoclonal Antibody in Advanced Solid Tumors. CPT Pharmacometrics Syst Pharmacol 2017;6:49-57. [Crossref] [PubMed]
- van Rijswijk RE, Vanloenen AC, Wagstaff J, et al. Suramin - Rapid Loading and Weekly Maintenance Regimens for Cancer-Patients. J Clin Oncol 1992;10:1788-94. [Crossref] [PubMed]
- Jodrell DI, Reyno LM, Sridhara R, et al. Suramin: Development of a Population Pharmacokinetic Model and Its Use with Intermittent Short Infusions to Control Plasma Drug Concentration in Patients with Prostate-Cancer. J Clin Oncol 1994;12:166-75. [Crossref] [PubMed]
- van Kuilenburg AB, Haasjes J, Richel DJ, et al. Clinical implications of dihydropyrimidine dehydrogenase (DPD) deficiency in patients with severe 5-fluorouracil-associated toxicity: Identification of new mutations in the DPD gene. Clin Cancer Res 2000;6:4705-12. [PubMed]
- McDonald GB, Slattery JT, Bouvier ME, et al. Cyclophosphamide metabolism, liver toxicity, and mortality following hematopoietic stem cell transplantation. Blood 2003;101:2043-8. [Crossref] [PubMed]
- Bach PB, Conti RM, Muller RJ, et al. Overspending driven by oversized single dose vials of cancer drugs. BMJ 2016;352:i788. [Crossref] [PubMed]
- Sheiner LB. Computer-aided long-term anticoagulation therapy. Comput Biomed Res 1969;2:507-18. [Crossref] [PubMed]
- Jelliffe RW. Administration of digoxin. Dis Chest 1969;56:56-60. [Crossref] [PubMed]
- Center for Drug Evaluation And Research. Clinical pharmacology and biopharmaceutics reviews (nivolumab). Application number: 125554Orig1s000. 2014. Available online: https://www.accessdata.fda.gov/drugsatfda_docs/nda/2014/125554Orig1s000ClinPharmR.pdf
- Center for Drug Evaluation And Research. Clinical pharmacology and biopharmaceutics reviews (pembrolizumab). Application number: 125514Orig1s000. 2014. Available online: https://www.accessdata.fda.gov/drugsatfda_docs/nda/2014/125514Orig1s000ClinPharmR.pdf
- Bellnier DA, Greco WR, Loewen GM, et al. Population pharmacokinetics of the photodynamic therapy agent 2-[1-hexyloxyethyl]-2-devinyl pyropheophorbide-a in cancer patients. Cancer Res 2003;63:1806-13. [PubMed]
- Griffin TW, Bokhari F, Collins J, et al. A Preliminary Pharmacokinetic Study of in-111-Labeled 260f9 Anti-(Breast Cancer) Antibody in Patients. Cancer Immunology Immunotherapy 1989;29:43-50. [Crossref] [PubMed]
- Kolesar J, Brundage RC, Pomplun M, et al. Population pharmacokinetics of 3-aminopyridine-2-carboxaldehyde thiosemicarbazone (Triapine (R)) in cancer patients. Cancer Chemother Pharmacol 2011;67:393-400. [Crossref] [PubMed]
- Pelsor FR, Allen LM, Creaven PJ. Multicompartment pharmacokinetic model of 4'-demethylepipodophyllotoxin 9-(4,6-O-ethylidene-beta-D-glucopyranoside) in humans. J Pharm Sci 1978;67:1106-8. [Crossref] [PubMed]
- Li J, Jameson MB, Baguley BC, et al. Population pharmacokinetic-pharmacodynamic model of the vascular-disrupting agent 5,6-dimethylxanthenone-4-acetic acid in cancer patients. Clinical Cancer Research 2008;14:2102-10. [Crossref] [PubMed]
- Robinson PJ, Bass L, Pond SM, et al. Clinical applicability of current pharmacokinetic models: splanchnic elimination of 5-fluorouracil in cancer patients. J Pharmacokinet Biopharm 1988;16:229-49. [Crossref] [PubMed]
- Wattanatorn W, McLeod HL, Macklon F, et al. Comparison of 5-fluorouracil pharmacokinetics in whole blood, plasma, and red blood cells in patients with colorectal cancer. Pharmacotherapy 1997;17:881-6. [PubMed]
- Bressolle F, Joulia JM, Pinguet F, et al. Circadian rhythm of 5-fluorouracil population pharmacokinetics in patients with metastatic colorectal cancer. Cancer Chemother Pharmacol 1999;44:295-302. [Crossref] [PubMed]
- Gardner SN. Modeling multi-drug chemotherapy: tailoring treatment to individuals. J Theor Biol 2002;214:181-207. [Crossref] [PubMed]
- Di Paolo A, Danesi R, Vannozzi F, et al. Limited sampling model for the analysis of 5-fluorouracil pharmacokinetics in adjuvant chemotherapy for colorectal cancer. Clin Pharmacol Ther 2002;72:627-37. [Crossref] [PubMed]
- Climente-Marti M, Merino-Sanjuan M, Almenar-Cubells D, et al. A Bayesian method for predicting 5-fluorouracil pharmacokinetic parameters following short-term infusion in patients with colorectal cancer. J Pharm Sci 2003;92:1155-65. [Crossref] [PubMed]
- Coustere C, Mentre F, Sommadossi JP, et al. A mathematical model of the kinetics of 5-fluorouracil and its metabolites in cancer patients. Cancer Chemother Pharmacol 1991;28:123-9. [Crossref] [PubMed]
- Sandstrom M, Lindman H, Nygren P, et al. Population analysis of the pharmacokinetics and the haematological toxicity of the fluorouracil-epirubicin-cyclophosphamide regimen in breast cancer patients. Cancer Chemother Pharmacol 2006;58:143-56. [Crossref] [PubMed]
- Sandström M, Freijs A, Larsson R, et al. Lack of relationship between systemic exposure for the component drugs of the fluorouracil, epirubicin, and 4-hydroxycyclophosphamide regimen in breast cancer patients. J Clin Oncol 1996;14:1581-8. [Crossref] [PubMed]
- Woloch C, Di Paolo A, Marouani H, et al. Population Pharmacokinetic Analysis of 5-FU and 5-FDHU in Colorectal Cancer Patients: Search for Biomarkers Associated with Gastro-Intestinal Toxicity. Curr Top Med Chem 2012;12:1713-9. [Crossref] [PubMed]
- Hawwa AF, Collier PS, Millership JS, et al. Population pharmacokinetic and pharmacogenetic analysis of 6-mercaptopurine in paediatric patients with acute lymphoblastic leukaemia. Br J Clin Pharmacol 2008;66:826-37. [Crossref] [PubMed]
- Rubin E, Wood V, Bharti A, et al. A phase I and pharmacokinetic study of a new camptothecin derivative, 9-aminocamptothecin. Clin Cancer Res 1995;1:269-76. [PubMed]
- Chalret du Rieu Q, Fouliard S, White-Koning M, et al. Pharmacokinetic/Pharmacodynamic modeling of abexinostat-induced thrombocytopenia across different patient populations: application for the determination of the maximum tolerated doses in both lymphoma and solid tumour patients. Invest New Drugs 2014;32:985-94. [Crossref] [PubMed]
- Stuyckens K, Saad F, Xu XS, et al. Population pharmacokinetic analysis of abiraterone in chemotherapy-naive and docetaxel-treated patients with metastatic castration-resistant prostate cancer. Clin Pharmacokinet 2014;53:1149-60. [Crossref] [PubMed]
- Mittapalli RK, Nuthalapati S, Shepherd SP, et al. Population pharmacokinetics of ABT-767 in BRCA1 or BRCA2 mutation carriers with advanced solid tumors or in subjects with high grade serous ovarian, primary peritoneal or fallopian tube cancer. Cancer Chemother Pharmacol 2017;79:587-94. [Crossref] [PubMed]
- Sharma S, Mittapalli RK, Holen KD, et al. Population Pharmacokinetics of ABT-806, an Investigational Anti-Epidermal Growth Factor Receptor (EGFR) Monoclonal Antibody, in Advanced Solid Tumor Types Likely to Either Over-Express Wild-Type EGFR or Express Variant III Mutant EGFR. Clin Pharmacokinet 2015;54:1071-81. [Crossref] [PubMed]
- Edwards AY, Skolnik JM, Dombrowsky E, et al. Modeling and simulation approaches to evaluate pharmacokinetic sampling contamination from central venous catheters in pediatric pharmacokinetic studies of actinomycin-D: a report from the children's oncology group. Cancer Chemother Pharmacol 2012;70:83-94. [Crossref] [PubMed]
- Freiwald M, Schmid U, Fleury A, et al. Population pharmacokinetics of afatinib, an irreversible ErbB family blocker, in patients with various solid tumors. Cancer Chemother Pharmacol 2014;73:759-70. [Crossref] [PubMed]
- Thai HT, Veyrat-Follet C, Mentre F, et al. Population pharmacokinetic analysis of free and bound aflibercept in patients with advanced solid tumors. Cancer Chemother Pharmacol 2013;72:167-80. [Crossref] [PubMed]
- Thai HT, Veyrat-Follet C, Vivier N, et al. A mechanism-based model for the population pharmacokinetics of free and bound aflibercept in healthy subjects. Br J Clin Pharmacol 2011;72:402-14. [Crossref] [PubMed]
- Hu ZY, Lu J, Zhao Y. A physiologically based pharmacokinetic model of alvespimycin in mice and extrapolation to rats and humans. Br J Pharmacol 2014;171:2778-89. [Crossref] [PubMed]
- Gupta A, Hussein Z, Hassan R, et al. Population pharmacokinetics and exposure-response relationship of amatuximab, an anti-mesothelin monoclonal antibody, in patients with malignant pleural mesothelioma and its application in dose selection. Cancer Chemother Pharmacol 2016;77:733-43. [Crossref] [PubMed]
- Lack NA, Green B, Dale DC, et al. A pharmacokinetic-pharmacodynamic model for the mobilization of CD34+ hematopoietic progenitor cells by AMD3100. Clin Pharmacol Ther 2005;77:427-36. [Crossref] [PubMed]
- Lu JF, Rasmussen E, Karlan BY, et al. Exposure-response relationship of AMG 386 in combination with weekly paclitaxel in recurrent ovarian cancer and its implication for dose selection. Cancer Chemother Pharmacol 2012;69:1135-44. [Crossref] [PubMed]
- Makino Y, Watanabe M, Makihara RA, et al. Simultaneous optimization of limited sampling points for pharmacokinetic analysis of amrubicin and amrubicinol in cancer patients. Asia Pac J Clin Oncol 2016;12:259-64. [Crossref] [PubMed]
- Noh YH, Ko YJ, Cho SH, et al. Pharmacokinetic comparison of 2 formulations of anastrozole (1 mg) in healthy Korean male volunteers: a randomized, single-dose, 2-period, 2-sequence, crossover study. Clin Ther 2012;34:305-13. [Crossref] [PubMed]
- Kletting P, Kull T, Bunjes D, et al. Radioimmunotherapy with anti-CD66 antibody: improving the biodistribution using a physiologically based pharmacokinetic model. J Nucl Med 2010;51:484-91. [Crossref] [PubMed]
- Kletting P, Maass C, Reske S, et al. Physiologically Based Pharmacokinetic Modeling Is Essential in 90Y-Labeled Anti-CD66 Radioimmunotherapy. PLoS One 2015;10:e0127934 [Crossref] [PubMed]
- Maass C, Kletting P, Bunjes D, et al. Population-Based Modeling Improves Treatment Planning Before (90)Y-Labeled Anti-CD66 Antibody Radioimmunotherapy. Cancer Biother Radiopharm 2015;30:285-90. [Crossref] [PubMed]
- Yu M, Gao Z, Dai X, et al. Population Pharmacokinetic and Covariate Analysis of Apatinib, an Oral Tyrosine Kinase Inhibitor, in Healthy Volunteers and Patients with Solid Tumors. Clin Pharmacokinet 2017;56:65-76. [Crossref] [PubMed]
- Duong JK, Griffin MJ, Hargrave D, et al. A population pharmacokinetic model of AT9283 in adults and children to predict the maximum tolerated dose in children with leukaemia. Br J Clin Pharmacol 2017;83:1713-22. [Crossref] [PubMed]
- Tortorici MA, Cohen EE, Pithavala YK, et al. Pharmacokinetics of single-agent axitinib across multiple solid tumor types. Cancer Chemother Pharmacol 2014;74:1279-89. [Crossref] [PubMed]
- Reilly RM, Kirsh J, Gallinger S, et al. Compartmental analysis of the pharmacokinetics of radioiodinated monoclonal antibody B72.3 in colon cancer patients. Nucl Med Biol 1993;20:57-64. [Crossref] [PubMed]
- Keizer RJ, Zandvliet AS, Beijnen JH, et al. Two-stage model-based design of cancer phase I dose escalation trials: evaluation using the phase I program of barasertib (AZD1152). Invest New Drugs 2012;30:1519-30. [Crossref] [PubMed]
- Peer CJ, Goey AK, Sissung TM, et al. UGT1A1 genotype-dependent dose adjustment of belinostat in patients with advanced cancers using population pharmacokinetic modeling and simulation. J Clin Pharmacol 2016;56:450-60. [Crossref] [PubMed]
- Darwish M, Megason G, Bond M, et al. Population pharmacokinetics and pharmacokinetics/pharmacodynamics of bendamustine in pediatric patients with relapsed/refractory acute leukemia. Curr Med Res Opin 2014;30:2305-15. [Crossref] [PubMed]
- Panoilia E, Schindler E, Samantas E, et al. A pharmacokinetic binding model for bevacizumab and VEGF165 in colorectal cancer patients. Cancer Chemother Pharmacol 2015;75:791-803. [Crossref] [PubMed]
- Caulet M, Lecomte T, Bouche O, et al. Bevacizumab Pharmacokinetics Influence Overall and Progression-Free Survival in Metastatic Colorectal Cancer Patients. Clin Pharmacokinet 2016;55:1381-94. [Crossref] [PubMed]
- Han K, Peyret T, Marchand M, et al. Population pharmacokinetics of bevacizumab in cancer patients with external validation. Cancer Chemother Pharmacol 2016;78:341-51. [Crossref] [PubMed]
- Han K, Peyret T, Quartino A, et al. Bevacizumab dosing strategy in paediatric cancer patients based on population pharmacokinetic analysis with external validation. Br J Clin Pharmacol 2016;81:148-60. [Crossref] [PubMed]
- Han K, Jin J, Maia M, et al. Lower exposure and faster clearance of bevacizumab in gastric cancer and the impact of patient variables: analysis of individual data from AVAGAST phase III trial. AAPS J 2014;16:1056-63. [Crossref] [PubMed]
- Stefanini MO, Wu FT, Mac Gabhann F, et al. Increase of plasma VEGF after intravenous administration of bevacizumab is predicted by a pharmacokinetic model. Cancer Res 2010;70:9886-94. [Crossref] [PubMed]
- Soto E, Staab A, Tillmann C, et al. Semi-mechanistic population pharmacokinetic/pharmacodynamic model for neutropenia following therapy with the Plk-1 inhibitor BI 2536 and its application in clinical development. Cancer Chemother Pharmacol 2010;66:785-95. [Crossref] [PubMed]
- Soto E, Staab A, Doege C, et al. Comparison of different semi-mechanistic models for chemotherapy-related neutropenia: application to BI 2536 a Plk-1 inhibitor. Cancer Chemother Pharmacol 2011;68:1517-27. [Crossref] [PubMed]
- Titze MI, Schaaf O, Hofmann MH, et al. An allometric pharmacokinetic/pharmacodynamics model for BI 893923, a novel IGF-1 receptor inhibitor. Cancer Chemother Pharmacol 2017;79:545-58. [Crossref] [PubMed]
- Hanley MJ, Mould DR, Taylor TJ, et al. Population Pharmacokinetic Analysis of Bortezomib in Pediatric Leukemia Patients: Model-Based Support for Body Surface Area-Based Dosing Over the 2- to 16-Year Age Range. J Clin Pharmacol 2017;57:1183-93. [Crossref] [PubMed]
- Li H, Han TH, Hunder NN, et al. Population Pharmacokinetics of Brentuximab Vedotin in Patients With CD30-Expressing Hematologic Malignancies. J Clin Pharmacol 2017;57:1148-58. [Crossref] [PubMed]
- McCune JS, Bemer MJ, Barrett JS, et al. Busulfan in infant to adult hematopoietic cell transplant recipients: a population pharmacokinetic model for initial and Bayesian dose personalization. Clin Cancer Res 2014;20:754-63. [Crossref] [PubMed]
- Booth BP, Rahman A, Dagher R, et al. Population pharmacokinetic-based dosing of intravenous busulfan in pediatric patients. J Clin Pharmacol 2007;47:101-11. [Crossref] [PubMed]
- Hassan M, Fasth A, Gerritsen B, et al. Busulphan kinetics and limited sampling model in children with leukemia and inherited disorders. Bone Marrow Transplant 1996;18:843-50. [PubMed]
- Nguyen L, Leger F, Lennon S, et al. Intravenous busulfan in adults prior to haematopoietic stem cell transplantation: a population pharmacokinetic study. Cancer Chemother Pharmacol 2006;57:191-8. [Crossref] [PubMed]
- Nakamura H, Sato T, Okada K, et al. Population pharmacokinetics of oral busulfan in young Japanese children before hematopoietic stem cell transplantation. Ther Drug Monit 2008;30:75-83. [Crossref] [PubMed]
- Trame MN, Bergstrand M, Karlsson MO, et al. Population pharmacokinetics of busulfan in children: increased evidence for body surface area and allometric body weight dosing of busulfan in children. Clin Cancer Res 2011;17:6867-77. [Crossref] [PubMed]
- de Castro FA, Piana C, Simoes BP, et al. Busulfan dosing algorithm and sampling strategy in stem cell transplantation patients. Br J Clin Pharmacol 2015;80:618-29. [Crossref] [PubMed]
- Zao JH, Schechter T, Liu WJ, et al. Performance of Busulfan Dosing Guidelines for Pediatric Hematopoietic Stem Cell Transplant Conditioning. Biol Blood Marrow Transplant 2015;21:1471-8. [Crossref] [PubMed]
- De Buck SS, Jakab A, Boehm M, et al. Population pharmacokinetics and pharmacodynamics of BYL719, a phosphoinositide 3-kinase antagonist, in adult patients with advanced solid malignancies. Br J Clin Pharmacol 2014;78:543-55. [Crossref] [PubMed]
- Ferron GM, Dai Y, Semiond D. Population pharmacokinetics of cabazitaxel in patients with advanced solid tumors. Cancer Chemother Pharmacol 2013;71:681-92. [Crossref] [PubMed]
- Miles D, Jumbe NL, Lacy S, et al. Population Pharmacokinetic Model of Cabozantinib in Patients with Medullary Thyroid Carcinoma and Its Application to an Exposure-Response Analysis. Clin Pharmacokinet 2016;55:93-105. [Crossref] [PubMed]
- Miles DR, Wada DR, Jumbe NL, et al. Population pharmacokinetic/pharmacodynamic modeling of tumor growth kinetics in medullary thyroid cancer patients receiving cabozantinib. Anticancer Drugs 2016;27:328-41. [Crossref] [PubMed]
- Daher Abdi Z, Lavau-Denes S, Premaud A, et al. Pharmacokinetics and exposure-effect relationships of capecitabine in elderly patients with breast or colorectal cancer. Cancer Chemother Pharmacol 2014;73:1285-93. [Crossref] [PubMed]
- Urien S, Rezai K, Lokiec F. Pharmacokinetic modelling of 5-FU production from capecitabine - A population study in 40 adult patients with metastatic cancer. J Pharmacokinet Pharmacodyn 2005;32:817-33. [Crossref] [PubMed]
- Gieschke R, Reigner B, Blesch KS, et al. Population pharmacokinetic analysis of the major metabolites of capecitabine. J Pharmacokinet Pharmacodyn 2002;29:25-47. [Crossref] [PubMed]
- Gieschke R, Burger HU, Reigner B, et al. Population pharmacokinetics and concentration-effect relationships of capecitabine metabolites in colorectal cancer patients. Br J Clin Pharmacol 2003;55:252-63. [Crossref] [PubMed]
- Merino-Sanjuán M, Monteiro JF, Porta-Oltra B, et al. Effect of age on systemic exposure and haematological toxicity of carboplatin in advanced non-small cell lung cancer patients. Basic Clin Pharmacol Toxicol 2011;109:457-64. [Crossref] [PubMed]
- Shen MY, Schilder RJ, Obasaju C, et al. Population pharmacokinetic and limited sampling models for carboplatin administered in high-dose combination regimens with peripheral blood stem cell support. Cancer Chemother Pharmacol 2002;50:243-50. [Crossref] [PubMed]
- Kloft C, Siegert W, Jaehde U. Individualised dosing strategy for high-dose carboplatin in patients with germ cell cancer. Br J Cancer 2003;89:787-94. [Crossref] [PubMed]
- Ekhart C, de Jonge ME, Huitema AD, et al. Flat dosing of carboplatin is justified in adult patients with normal renal function. Clin Cancer Res 2006;12:6502-8. [Crossref] [PubMed]
- Lindauer A, Eickhoff C, Kloft C, et al. Population pharmacokinetics of high-dose carboplatin in children and adults. Ther Drug Monit 2010;32:159-68. [PubMed]
- Guillet P, Monjanel S, Nicoara A, et al. A Bayesian dosing method for carboplatin given by continuous infusion for 120 h. Cancer Chemother Pharmacol 1997;40:143-9. [Crossref] [PubMed]
- Ou Y, Doshi S, Nguyen A, et al. Population Pharmacokinetics and Exposure-Response Relationship of Carfilzomib in Patients With Multiple Myeloma. J Clin Pharmacol 2017;57:663-77. [Crossref] [PubMed]
- Fetterly GJ, Aras U, Meholick PD, et al. Utilizing pharmacokinetics/pharmacodynamics modeling to simultaneously examine free CCL2, total CCL2 and carlumab (CNTO 888) concentration time data. J Clin Pharmacol 2013;53:1020-7. [Crossref] [PubMed]
- Davda JP, Jain M, Batra SK, et al. A physiologically based pharmacokinetic (PBPK) model to characterize and predict the disposition of monoclonal antibody CC49 and its single chain Fv constructs. Int Immunopharmacol 2008;8:401-13. [Crossref] [PubMed]
- Li J, Al-Huniti N, Henningsson A, et al. Population pharmacokinetic and exposure simulation analysis for cediranib (AZD2171) in pooled Phase I/II studies in patients with cancer. Br J Clin Pharmacol 2017;83:1723-33. [Crossref] [PubMed]
- Hong Y, Passos VQ, Huang PH, et al. Population Pharmacokinetics of Ceritinib in Adult Patients With Tumors Characterized by Genetic Abnormalities in Anaplastic Lymphoma Kinase. J Clin Pharmacol 2017;57:652-62. [Crossref] [PubMed]
- Azzopardi N, Lecomte T, Ternant D, et al. Cetuximab pharmacokinetics influences progression-free survival of metastatic colorectal cancer patients. Clin Cancer Res 2011;17:6329-37. [Crossref] [PubMed]
- Pointreau Y, Azzopardi N, Ternant D, et al. Cetuximab Pharmacokinetics Influences Overall Survival in Patients With Head and Neck Cancer. Therapeutic Drug Monitoring 2016;38:567-72. [PubMed]
- Miller VA, Rigas JR, Tong WP, et al. Phase II trial of chloroquinoxaline sulfonamide (CQS) in patients with stage III and IV non-small-cell lung cancer. Cancer Chemother Pharmacol 1997;40:415-8. [Crossref] [PubMed]
- Zühlsdorf M, Bhattaram VA, Campioni M, et al. Population pharmacokinetics of cilengitide in adult and pediatric cancer patients from a nonlinear mixed-effects analysis. J Clin Pharmacol 2014;54:1391-9. [Crossref] [PubMed]
- de Jongh FE, Gallo JM, Shen MY, et al. Population pharmacokinetics of cisplatin in adult cancer patients. Cancer Chemother Pharmacol 2004;54:105-12. [Crossref] [PubMed]
- Reece PA, Stafford I, Davy M, et al. Disposition of unchanged cisplatin in patients with ovarian cancer. Clin Pharmacol Ther 1987;42:320-5. [Crossref] [PubMed]
- Nagai N, Ogata H, Wada Y, et al. Population pharmacokinetics and pharmacodynamics of cisplatin in patients with cancer: analysis with the NONMEM program. J Clin Pharmacol 1998;38:1025-34. [Crossref] [PubMed]
- Salas S, Mercier C, Ciccolini J, et al. Therapeutic drug monitoring for dose individualization of Cisplatin in testicular cancer patients based upon total platinum measurement in plasma. Ther Drug Monit 2006;28:532-9. [Crossref] [PubMed]
- Hanada K, Nishijima K, Ogata H, et al. Population pharmacokinetic analysis of cisplatin and its metabolites in cancer patients: possible misinterpretation of covariates for pharmacokinetic parameters calculated from the concentrations of unchanged cisplatin, ultrafiltered platinum and total platinum. Jpn J Clin Oncol 2001;31:179-84. [Crossref] [PubMed]
- Griffiths H, Shelley MD, Fish RG. A modified pharmacokinetic model for platinum disposition in ovarian cancer patients receiving cisplatin. Eur J Clin Pharmacol 1987;33:67-72. [Crossref] [PubMed]
- Fracasso ME, Apostoli P, Benoni G, et al. Kinetics of Platinum in Cancer-Patients Treated with Cisplatin at Different Doses. Drugs Exp Clin Res 1987;13:367-72. [PubMed]
- Leone R, Benoni G, Apostoli P, et al. Two-cycle cisplatin kinetics in patients with ovarian and mammary cancer. Ther Drug Monit 1987;9:374-7. [Crossref] [PubMed]
- Bonetti A, Franceschi T, Apostoli P, et al. Cisplatin Pharmacokinetics in Elderly Patients. Ther Drug Monit 1994;16:477-82. [Crossref] [PubMed]
- Panteix G, Beaujard A, Garbit F, et al. Population pharmacokinetics of cisplatin in patients with advanced ovarian cancer during intraperitoneal hyperthermia chemotherapy. Anticancer Res 2002;22:1329-36. [PubMed]
- Joerger M, Burgers JA, Baas P, et al. Gene polymorphisms, pharmacokinetics, and hematological toxicity in advanced non-small-cell lung cancer patients receiving cisplatin/gemcitabine. Cancer Chemother Pharmacol 2012;69:25-33. [Crossref] [PubMed]
- Evans WE, Crom WR, Tsiatis A, et al. Pharmacokinetic modeling of cisplatin disposition in children and adolescents with cancer. Cancer Chemother Pharmacol 1982;10:22-6. [Crossref] [PubMed]
- Wu H, Ramanathan RK, Zamboni BA, et al. Mechanism-based model characterizing bidirectional interaction between PEGylated liposomal CKD-602 (S-CKD602) and monocytes in cancer patients. Int J Nanomedicine 2012;7:5555-64. [Crossref] [PubMed]
- Bonate PL, Craig A, Gaynon P, et al. Population pharmacokinetics of clofarabine, a second-generation nucleoside analog, in pediatric patients with acute leukemia. J Clin Pharmacol 2004;44:1309-22. [Crossref] [PubMed]
- Han K, Jin JY, Marchand M, et al. Population pharmacokinetics and dosing implications for cobimetinib in patients with solid tumors. Cancer Chemother Pharmacol 2015;76:917-24. [Crossref] [PubMed]
- Yamazaki S, Vicini P, Shen Z, et al. Pharmacokinetic/pharmacodynamic modeling of crizotinib for anaplastic lymphoma kinase inhibition and antitumor efficacy in human tumor xenograft mouse models. J Pharmacol Exp Ther 2012;340:549-57. [Crossref] [PubMed]
- Wang E, Nickens DJ, Bello A, et al. Clinical Implications of the Pharmacokinetics of Crizotinib in Populations of Patients with Non-Small Cell Lung Cancer. Clin Cancer Res 2016;22:5722-8. [Crossref] [PubMed]
- Edwards AY, Elgart A, Farrell C, et al. A population pharmacokinetic meta-analysis of custirsen, an antisense oligonucleotide, in oncology patients and healthy subjects. Br J Clin Pharmacol 2017;83:1932-43. [Crossref] [PubMed]
- Xie HJ, Griskevicius L, Stahle L, et al. Pharmacogenetics of cyclophosphamide in patients with hematological malignancies. Eur J Pharm Sci 2006;27:54-61. [Crossref] [PubMed]
- Chen TL, Passos-Coelho JL, Noe DA, et al. Nonlinear pharmacokinetics of cyclophosphamide in patients with metastatic breast cancer receiving high-dose chemotherapy followed by autologous bone marrow transplantation. Cancer Research 1995;55:810-6. [PubMed]
- Hassan M, Svensson US, Ljungman P, et al. A mechanism-based pharmacokinetic-enzyme model for cyclophosphamide autoinduction in breast cancer patients. Br J Clin Pharmacol 1999;48:669-77. [Crossref] [PubMed]
- Huitema AD, Mathot RA, Tibben MM, et al. A mechanism-based pharmacokinetic model for the cytochrome P450 drug-drug interaction between cyclophosphamide and thioTEPA and the autoinduction of cyclophosphamide. J Pharmacokinet Pharmacodyn 2001;28:211-30. [Crossref] [PubMed]
- Krogh-Madsen M, Bender B, Jensen MK, et al. Population pharmacokinetics of cytarabine, etoposide, and daunorubicin in the treatment for acute myeloid leukemia. Cancer Chemother Pharmacol 2012;69:1155-63. [Crossref] [PubMed]
- Hempel G, Relling MV, de Rossi G, et al. Pharmacokinetics of daunorubicin and daunorubicinol in infants with leukemia treated in the interfant 99 protocol. Pediatr Blood Cancer 2010;54:355-60. [Crossref] [PubMed]
- Tornoe CW, Agerso H, Nielsen HA, et al. Pharmacokinetic/pharmacodynamic modelling of GnRH antagonist degarelix: a comparison of the non-linear mixed-effects programs NONMEM and NLME. J Pharmacokinet Pharmacodyn 2004;31:441-61. [Crossref] [PubMed]
- Tornoe CW, Agerso H, Nielsen HA, et al. Population pharmacokinetic modeling of a subcutaneous depot for GnRH antagonist degarelix. Pharm Res 2004;21:574-84. [Crossref] [PubMed]
- Perez Ruixo JJ, Doshi S, Sohn W, et al. Quantitative pharmacology of denosumab in patients with bone metastases from solid tumors. J Clin Pharmacol 2015;55:S85-92. [Crossref] [PubMed]
- Gibiansky L, Sutjandra L, Doshi S, et al. Population pharmacokinetic analysis of denosumab in patients with bone metastases from solid tumours. Clin Pharmacokinet 2012;51:247-60. [Crossref] [PubMed]
- Soto E, Keizer RJ, Troconiz IF, et al. Predictive ability of a semi-mechanistic model for neutropenia in the development of novel anti-cancer agents: two case studies. Invest New Drugs 2011;29:984-95. [Crossref] [PubMed]
- Marachelian A, Desai A, Balis F, et al. Comparative pharmacokinetics, safety, and tolerability of two sources of ch14.18 in pediatric patients with high-risk neuroblastoma following myeloablative therapy. Cancer Chemother Pharmacol 2016;77:405-12. [Crossref] [PubMed]
- Onoue H, Yano I, Tanaka A, et al. Significant effect of age on docetaxel pharmacokinetics in Japanese female breast cancer patients by using the population modeling approach. Eur J Clin Pharmacol 2016;72:703-10. [Crossref] [PubMed]
- van Hasselt JG, van Calsteren K, Heyns L, et al. Optimizing anticancer drug treatment in pregnant cancer patients: pharmacokinetic analysis of gestation-induced changes for doxorubicin, epirubicin, docetaxel and paclitaxel. Ann Oncol 2014;25:2059-65. [Crossref] [PubMed]
- Hooker AC, Ten Tije AJ, Carducci MA, et al. Population pharmacokinetic model for docetaxel in patients with varying degrees of liver function: Incorporating cytochrome P450 3A activity measurements. Clin Pharmacol Ther 2008;84:111-8. [Crossref] [PubMed]
- Lewis LD, Miller AA, Rosner GL, et al. A comparison of the pharmacokinetics and pharmacodynamics of docetaxel between African-American and Caucasian cancer patients: CALGB 9871. Clin Cancer Res 2007;13:3302-11. [Crossref] [PubMed]
- Fukae M, Shiraishi Y, Hirota T, et al. Population pharmacokinetic-pharmacodynamic modeling and model-based prediction of docetaxel-induced neutropenia in Japanese patients with non-small cell lung cancer. Cancer Chemother Pharmacol 2016;78:1013-23. [Crossref] [PubMed]
- Bruno R, Vivier N, Veyrat-Follet C, et al. Population pharmacokinetics and pharmacokinetic-pharmacodynamic relationships for docetaxel. Invest New Drugs 2001;19:163-9. [Crossref] [PubMed]
- Slaviero KA, Clarke SJ, McLachlan AJ, et al. Population pharmacokinetics of weekly docetaxel in patients with advanced cancer. Br J Clin Pharmacol 2004;57:44-53. [Crossref] [PubMed]
- Kloft C, Wallin J, Henningsson A, et al. Population pharmacokinetic-pharmacodynamic model for neutropenia with patient subgroup identification: comparison across anticancer drugs. Clin Cancer Res 2006;12:5481-90. [Crossref] [PubMed]
- Ozawa K, Minami H, Sato H. Population pharmacokinetic and pharmacodynamic analysis for time courses of docetaxel-induced neutropenia in Japanese cancer patients. Cancer Sci 2007;98:1985-92. [Crossref] [PubMed]
- Minami H, Kawada K, Sasaki Y, et al. Population pharmacokinetics of docetaxel in patients with hepatic dysfunction treated in an oncology practice. Cancer Sci 2009;100:144-9. [Crossref] [PubMed]
- Koolen SL, Oostendorp RL, Beijnen JH, et al. Population pharmacokinetics of intravenously and orally administered docetaxel with or without co-administration of ritonavir in patients with advanced cancer. Br J Clin Pharmacol 2010;69:465-74. [Crossref] [PubMed]
- Minami H, Ohe Y, Niho S, et al. Comparison of pharmacokinetics and pharmacodynamics of docetaxel and Cisplatin in elderly and non-elderly patients: why is toxicity increased in elderly patients? J Clin Oncol 2004;22:2901-8. [Crossref] [PubMed]
- Meille C, Barbolosi D, Ciccolini J, et al. Revisiting Dosing Regimen Using Pharmacokinetic/Pharmacodynamic Mathematical Modeling: Densification and Intensification of Combination Cancer Therapy. Clin Pharmacokinet 2016;55:1015-25. [Crossref] [PubMed]
- Bruno R, Vivier N, Vergniol JC, et al. A population pharmacokinetic model for docetaxel (Taxotere): model building and validation. J Pharmacokinet Biopharm 1996;24:153-72. [Crossref] [PubMed]
- Bosch TM, Huitema AD, Doodeman VD, et al. Pharmacogenetic screening of CYP3A and ABCB1 in relation to population pharmacokinetics of docetaxel. Clin Cancer Res 2006;12:5786-93. [Crossref] [PubMed]
- Launay-Iliadis MC, Bruno R, Cosson V, et al. Population pharmacokinetics of docetaxel during phase I studies using nonlinear mixed-effect modeling and nonparametric maximum-likelihood estimation. Cancer Chemother Pharmacol 1995;37:47-54. [Crossref] [PubMed]
- Kletting P, Muller B, Erentok B, et al. Differences in predicted and actually absorbed doses in peptide receptor radionuclide therapy. Med Phys 2012;39:5708-17. [Crossref] [PubMed]
- Joerger M, Huitema ADR, Richel DJ, et al. Population pharmacokinetics and pharmacodynamics of doxorubicin and cyclophosphamide in breast cancer patients: A study by the EORTC-PAMM-NDDG. Clin Pharmacokinet 2007;46:1051-68. [Crossref] [PubMed]
- Voller S, Boos J, Krischke M, et al. Age-Dependent Pharmacokinetics of Doxorubicin in Children with Cancer. Clin Pharmacokinet 2015;54:1139-49. [Crossref] [PubMed]
- Frenay M, Milano G, Renee N, et al. Pharmacokinetics of weekly low dose doxorubicin. Eur J Cancer Clin Oncol 1989;25:191-5. [Crossref] [PubMed]
- Thompson PA, Rosner GL, Matthay KK, et al. Impact of body composition on pharmacokinetics of doxorubicin in children: a Glaser Pediatric Research Network study. Cancer Chemother Pharmacol 2009;64:243-51. [Crossref] [PubMed]
- Barpe DR, Rosa DD, Froehlich PE. Pharmacokinetic evaluation of doxorubicin plasma levels in normal and overweight patients with breast cancer and simulation of dose adjustment by different indexes of body mass. Eur J Pharm Sci 2010;41:458-63. [Crossref] [PubMed]
- Wong AL, Seng KY, Ong EM, et al. Body fat composition impacts the hematologic toxicities and pharmacokinetics of doxorubicin in Asian breast cancer patients. Breast Cancer Res Treat 2014;144:143-52. [Crossref] [PubMed]
- Kunarajah K, Hennig S, Norris RLG, et al. Population pharmacokinetic modelling of doxorubicin and doxorubicinol in children with cancer: is there a relationship with cardiac troponin profiles? Cancer Chemother Pharmacol 2017;80:15-25. [Crossref] [PubMed]
- Xu L, Wang W, Sheng YC, et al. Pharmacokinetics and its relation to toxicity of pegylated-liposomal doxorubicin in Chinese patients with breast tumours. J Clin Pharm Ther 2010;35:593-601. [Crossref] [PubMed]
- Liang S, Brundage RC, Jacobson PA, et al. Pharmacokinetic-pharmacodynamic modelling of acute N-terminal pro B-type natriuretic peptide after doxorubicin infusion in breast cancer. Br J Clin Pharmacol 2016;82:773-83. [Crossref] [PubMed]
- Rahman A, Treat J, Roh JK, et al. A Phase-I Clinical-Trial and Pharmacokinetic Evaluation of Liposome-Encapsulated Doxorubicin. J Clin Oncol 1990;8:1093-100. [Crossref] [PubMed]
- Harris PA, Gross JF. Preliminary Pharmacokinetic Model for Adriamycin (Nsc-123127). Cancer Chemother Rep 1975;59:819-25. [PubMed]
- Chan KK, Cohen JL, Gross JF, et al. Prediction of adriamycin disposition in cancer patients using a physiologic, pharmacokinetic model. Cancer Treat Rep 1978;62:1161-71. [PubMed]
- Keizer RJ, Gupta A, Mac Gillavry MR, et al. A model of hypertension and proteinuria in cancer patients treated with the anti-angiogenic drug E7080. J Pharmacokinet Pharmacodyn 2010;37:347-63. [Crossref] [PubMed]
- Keizer RJ, Zamacona MK, Jansen M, et al. Application of population pharmacokinetic modeling in early clinical development of the anticancer agent E7820. Invest New Drugs 2009;27:140-52. [Crossref] [PubMed]
- Gibiansky L, Passey C, Roy A, et al. Model-based pharmacokinetic analysis of elotuzumab in patients with relapsed/refractory multiple myeloma. J Pharmacokinet Pharmacodyn 2016;43:243-57. [Crossref] [PubMed]
- Narayanan R, Hoffmann M, Kumar G, et al. Application of a "Fit for Purpose" PBPK Model to Investigate the CYP3A4 Induction Potential of Enzalutamide. Drug Metab Lett 2016;10:172-9. [Crossref] [PubMed]
- Shi JG, Bowman KJ, Chen X, et al. Population Pharmacokinetic and Pharmacodynamic Modeling of Epacadostat in Patients With Advanced Solid Malignancies. J Clin Pharmacol 2017;57:720-9. [Crossref] [PubMed]
- Jakobsen P, Steiness E, Bastholt L, et al. Multiple-Dose Pharmacokinetics of Epirubicin at 4 Different Dose Levels - Studies in Patients with Metastatic Breast-Cancer. Cancer Chemotherapy and Pharmacology 1991;28:63-8. [Crossref] [PubMed]
- van Hasselt JG, Gupta A, Hussein Z, et al. Population pharmacokinetic-pharmacodynamic analysis for eribulin mesilate-associated neutropenia. Br J Clin Pharmacol 2013;76:412-24. [Crossref] [PubMed]
- Foo J, Chmielecki J, Pao W, et al. Effects of pharmacokinetic processes and varied dosing schedules on the dynamics of acquired resistance to erlotinib in EGFR-mutant lung cancer. J Thorac Oncol 2012;7:1583-93. [Crossref] [PubMed]
- Lu JF, Eppler SM, Wolf J, et al. Clinical pharmacokinetics of erlotinib in patients with solid tumors and exposure-safety relationship in patients with non-small cell lung cancer. Clin Pharmacol Ther 2006;80:136-45. [Crossref] [PubMed]
- Sassen SD, Mathot RA, Pieters R, et al. Population pharmacokinetics of intravenous Erwinia asparaginase in pediatric acute lymphoblastic leukemia patients. Haematologica 2017;102:552-61. [Crossref] [PubMed]
- You B, Salles G, Bachy E, et al. Etoposide pharmacokinetics impact the outcomes of lymphoma patients treated with BEAM regimen and ASCT: a multicenter study of the LYmphoma Study Association (LYSA). Cancer Chemother Pharmacol 2015;76:939-48. [Crossref] [PubMed]
- Evans WE, Sinkule JA, Crom WR, et al. Pharmacokinetics of Teniposide (VM26) and etoposide (VP16-213) in children with cancer. Cancer Chemother Pharmacol 1982;7:147-50. [Crossref] [PubMed]
- Tranchand B, Amsellem C, Chatelut E, et al. A limited-sampling strategy for estimation of etoposide pharmacokinetics in cancer patients. Cancer Chemotherapy and Pharmacology 1999;43:316-22. [Crossref] [PubMed]
- Toffoli G, Corona G, Sorio R, et al. Population pharmacokinetics and pharmacodynamics of oral etoposide. Br J Clin Pharmacol 2001;52:511-9. [Crossref] [PubMed]
- You B, Tranchand B, Girard P, et al. Etoposide pharmacokinetics and survival in patients with small cell lung cancer: a multicentre study. Lung Cancer 2008;62:261-72. [Crossref] [PubMed]
- Millward MJ, Newell DR, Yuen K, et al. Pharmacokinetics and pharmacodynamics of prolonged oral etoposide in women with metastatic breast cancer. Cancer Chemother Pharmacol 1995;37:161-7. [Crossref] [PubMed]
- Kersting G, Willmann S, Wurthwein G, et al. Physiologically based pharmacokinetic modelling of high- and low-dose etoposide: from adults to children. Cancer Chemother Pharmacol 2012;69:397-405. [Crossref] [PubMed]
- van Erp NP, van Herpen CM, de Wit D, et al. A Semi-Physiological Population Model to Quantify the Effect of Hematocrit on Everolimus Pharmacokinetics and Pharmacodynamics in Cancer Patients. Clin Pharmacokinet 2016;55:1447-56. [Crossref] [PubMed]
- de Wit D, Schneider TC, Moes DJ, et al. Everolimus pharmacokinetics and its exposure-toxicity relationship in patients with thyroid cancer. Cancer Chemother Pharmacol 2016;78:63-71. [Crossref] [PubMed]
- Tanaka C, O'Reilly T, Kovarik JM, et al. Identifying optimal biologic doses of everolimus (RAD001) in patients with cancer based on the modeling of preclinical and clinical pharmacokinetic and pharmacodynamic data. J Clin Oncol 2008;26:1596-602. [Crossref] [PubMed]
- Saba NF, Hurwitz SJ, Magliocca K, et al. Phase 1 and pharmacokinetic study of everolimus in combination with cetuximab and carboplatin for recurrent/metastatic squamous cell carcinoma of the head and neck. Cancer 2014;120:3940-51. [Crossref] [PubMed]
- Ajani JA, Takimoto C, Becerra CR, et al. A phase II clinical and pharmacokinetic study of intravenous exatecan mesylate (DX-8951f) in patients with untreated metastatic gastric cancer. Invest New Drugs 2005;23:479-84. [Crossref] [PubMed]
- Farrell C, Schweizer C, Wustner J, et al. Population pharmacokinetics of farletuzumab, a humanized monoclonal antibody against folate receptor alpha, in epithelial ovarian cancer. Cancer Chemother Pharmacol 2012;70:727-34. [Crossref] [PubMed]
- Singh RP, Patel B, Kallender H, et al. Population pharmacokinetics modeling and analysis of foretinib in adult patients with advanced solid tumors. J Clin Pharmacol 2015;55:1184-92. [Crossref] [PubMed]
- Urien S, Fumoleau P, Campone M, et al. Modelling of ftorafur and 5-fluorouracil pharmacokinetics following oral UFT administration. A population study in 30 patients with advanced breast cancer. Cancer Chemother Pharmacol 2003;52:99-107. [Crossref] [PubMed]
- Robertson JF, Erikstein B, Osborne KC, et al. Pharmacokinetic profile of intramuscular fulvestrant in advanced breast cancer. Clin Pharmacokinet 2004;43:529-38. [Crossref] [PubMed]
- McCormack P, Sapunar F. Pharmacokinetic profile of the fulvestrant loading dose regimen in postmenopausal women with hormone receptor-positive advanced breast cancer. Clinical Breast Cancer 2008;8:347-51. [Crossref] [PubMed]
- Wong H, Gould SE, Budha N, et al. Learning and confirming with preclinical studies: modeling and simulation in the discovery of GDC-0917, an inhibitor of apoptosis proteins antagonist. Drug Metab Dispos 2013;41:2104-13. [Crossref] [PubMed]
- Salphati L, Pang J, Plise EG, et al. Preclinical assessment of the absorption and disposition of the phosphatidylinositol 3-kinase/mammalian target of rapamycin inhibitor GDC-0980 and prediction of its pharmacokinetics and efficacy in human. Drug Metab Dispos 2012;40:1785-96. [Crossref] [PubMed]
- Li J, Karlsson MO, Brahmer J, et al. CYP3A phenotyping approach to predict systemic exposure to EGFR tyrosine kinase inhibitors. J Natl Cancer Inst 2006;98:1714-23. [Crossref] [PubMed]
- Bi Y, Deng J, Murry DJ, et al. A Whole-Body Physiologically Based Pharmacokinetic Model of Gefitinib in Mice and Scale-Up to Humans. AAPS J 2016;18:228-38. [Crossref] [PubMed]
- Leijen S, Veltkamp SA, Huitema AD, et al. Phase I dose-escalation study and population pharmacokinetic analysis of fixed dose rate gemcitabine plus carboplatin as second-line therapy in patients with ovarian cancer. Gynecol Oncol 2013;130:511-7. [Crossref] [PubMed]
- Jiang XM, Galettis P, Links M, et al. Population pharmacokinetics of gemcitabine and its metabolite in patients with cancer: effect of oxaliplatin and infusion rate. Br J Clin Pharmacol 2008;65:326-33. [Crossref] [PubMed]
- Masumori N, Kunishima Y, Hirobe M, et al. Measurement of plasma concentration of gemcitabine and its metabolite dFdU in hemodialysis patients with advanced urothelial cancer. Jpn J Clin Oncol 2008;38:182-5. [Crossref] [PubMed]
- Sugiyama E, Kaniwa N, Kim SR, et al. Population Pharmacokinetics of Gemcitabine and Its Metabolite in Japanese Cancer Patients Impact of Genetic Polymorphisms. Clin Pharmacokinet 2010;49:549-58. [Crossref] [PubMed]
- Tham LS, Wang LZ, Soo RA, et al. A pharmacodynamic model for the time course of tumor shrinkage by gemcitabine plus carboplatin in non-small cell lung cancer patients. Clin Cancer Res 2008;14:4213-8. [Crossref] [PubMed]
- Fang L, Holford NH, Hinkle G, et al. Population pharmacokinetics of humanized monoclonal antibody HuCC49deltaCH2 and murine antibody CC49 in colorectal cancer patients. J Clin Pharmacol 2007;47:227-37. [Crossref] [PubMed]
- Tracewell WG, Trump DL, Vaughan WP, et al. Population pharmacokinetics of hydroxyurea in cancer patients. Cancer Chemother Pharmacol 1995;35:417-22. [Crossref] [PubMed]
- Albrecht CF, Kruger PB, Smit BJ, et al. The Pharmacokinetic Behavior of Hypoxoside Taken Orally by Patients with Lung-Cancer in a Phase-I Trial. S Afr Med J 1995;85:861-5. [PubMed]
- Marostica E, Sukbuntherng J, Loury D, et al. Population pharmacokinetic model of ibrutinib, a Bruton tyrosine kinase inhibitor, in patients with B cell malignancies. Cancer Chemother Pharmacol 2015;75:111-21. [Crossref] [PubMed]
- Reid JM, Pendergrass TW, Krailo MD, et al. Plasma pharmacokinetics and cerebrospinal fluid concentrations of idarubicin and idarubicinol in pediatric leukemia patients: a Childrens Cancer Study Group report. Cancer Res 1990;50:6525-8. [PubMed]
- Jin F, Gao Y, Zhou H, et al. Population pharmacokinetic modeling of idelalisib, a novel PI3Kdelta inhibitor, in healthy subjects and patients with hematologic malignancies. Cancer Chemother Pharmacol 2016;77:89-98. [Crossref] [PubMed]
- Kerbusch T, vanPutten JW, Groen HJ, et al. Population pharmacokinetics of ifosfamide and its 2- and 3-dechloroethylated and 4-hydroxylated metabolites in resistant small-cell lung cancer patients. Cancer Chemother Pharmacol 2001;48:53-61. [Crossref] [PubMed]
- Gotta V, Widmer N, Montemurro M, et al. Therapeutic drug monitoring of imatinib: Bayesian and alternative methods to predict trough levels. Clin Pharmacokinet 2012;51:187-201. [Crossref] [PubMed]
- Widmer N, Decosterd LA, Csajka C, et al. Population pharmacokinetics of imatinib and the role of alpha-acid glycoprotein. Br J Clin Pharmacol 2006;62:97-112. [Crossref] [PubMed]
- van Erp N, Gelderblom H, van Glabbeke M, et al. Effect of cigarette smoking on imatinib in patients in the soft tissue and bone sarcoma group of the EORTC. Clin Cancer Res 2008;14:8308-13. [Crossref] [PubMed]
- Treiber G, Wex T, Schleyer E, et al. Imatinib for hepatocellular cancer - Focus on pharmacokinetic/pharmacodynamic modelling and liver function. Cancer Lett 2008;260:146-54. [Crossref] [PubMed]
- Petain A, Kattygnarath D, Azard J, et al. Population pharmacokinetics and pharmacogenetics of imatinib in children and adults. Clin Cancer Res 2008;14:7102-9. [Crossref] [PubMed]
- Schmidli H, Peng B, Riviere GJ, et al. Population pharmacokinetics of imatinib mesylate in patients with chronic-phase chronic myeloid leukaemia: results of a phase III study. Br J Clin Pharmacol 2005;60:35-44. [Crossref] [PubMed]
- Delbaldo C, Chatelut E, Re M, et al. Pharmacokinetic-pharmacodynamic relationships of imatinib and its main metabolite in patients with advanced gastrointestinal stromal tumors. Clin Cancer Res 2006;12:6073-8. [Crossref] [PubMed]
- Menon-Andersen D, Mondick JT, Jayaraman B, et al. Population pharmacokinetics of imatinib mesylate and its metabolite in children and young adults. Cancer Chemother Pharmacol 2009;63:229-38. [Crossref] [PubMed]
- Ames MM, Miser JS, Smithson WA, et al. Pharmacokinetic Study of Indicine N-Oxide in Pediatric Cancer-Patients. Cancer Chemother Pharmacol 1982;10:43-6. [Crossref] [PubMed]
- Zandvliet AS, Schellens JH, Copalu W, et al. A semi-physiological population pharmacokinetic model describing the non-linear disposition of indisulam. J Pharmacokinet Pharmacodyn 2006;33:543-70. [Crossref] [PubMed]
- Zandvliet AS, Huitema AD, Copalu W, et al. CYP2C9 and CYP2C19 polymorphic forms are related to increased indisulam exposure and higher risk of severe hematologic toxicity. Clin Cancer Res 2007;13:2970-6. [Crossref] [PubMed]
- Garcia-Garcia I, Hernandez-Gonzalez I, Diaz-Machado A, et al. Pharmacokinetic and pharmacodynamic characterization of a novel formulation containing co-formulated interferons alpha-2b and gamma in healthy male volunteers. BMC Pharmacol Toxicol 2016;17:58. [Crossref] [PubMed]
- Pahlka RB, Sonnad JR. The effects of dialysis on 131I kinetics and dosimetry in thyroid cancer patients--a pharmacokinetic model. Health Physics 2006;91:227-37. [Crossref] [PubMed]
- Feng Y, Masson E, Dai D, et al. Model-based clinical pharmacology profiling of ipilimumab in patients with advanced melanoma. Br J Clin Pharmacol 2014;78:106-17. [Crossref] [PubMed]
- de Jong FA, Mathijssen RH, Xie R, et al. Flat-fixed dosing of irinotecan: Influence on pharmacokinetic and pharmacodynamic variability. Clin Cancer Res 2004;10:4068-71. [Crossref] [PubMed]
- Xie R, Mathijssen RH, Sparreboom A, et al. Clinical pharmacokinetics of irinotecan and its metabolites: A population analysis. J Clin Oncol 2002;20:3293-301. [Crossref] [PubMed]
- Toshimoto K, Tomaru A, Hosokawa M, et al. Virtual Clinical Studies to Examine the Probability Distribution of the AUC at Target Tissues Using Physiologically-Based Pharmacokinetic Modeling: Application to Analyses of the Effect of Genetic Polymorphism of Enzymes and Transporters on Irinotecan Induced Side Effects. Pharm Res 2017;34:1584-1600. [Crossref] [PubMed]
- Ahn BJ, Choi MK, Park YS, et al. Population pharmacokinetics of CPT-11 (irinotecan) in gastric cancer patients with peritoneal seeding after its intraperitoneal administration. Eur J Clin Pharmacol 2010;66:1235-45. [Crossref] [PubMed]
- Nakashima H, Lieberman R, Karato A, et al. Efficient Sampling Strategies for Forecasting Pharmacokinetic Parameters of Irinotecan (Cpt-11) - Implication for Area under the Concentration-Time Curve Monitoring. Ther Drug Monit 1995;17:221-9. [Crossref] [PubMed]
- Poujol S, Pinguet F, Ychou M, et al. A limited sampling strategy to estimate the pharmacokinetic parameters of irinotecan and its active metabolite, SN-38, in patients with metastatic digestive cancer receiving the FOLFIRI regimen. Oncology Reports 2007;18:1613-21. [PubMed]
- Thompson PA, Gupta M, Rosner GL, et al. Pharmacokinetics of irinotecan and its metabolites in pediatric cancer patients: a report from the children's oncology group. Cancer Chemother Pharmacol 2008;62:1027-37. [Crossref] [PubMed]
- Berg AK, Buckner JC, Galanis E, et al. Quantification of the impact of enzyme-inducing antiepileptic drugs on irinotecan pharmacokinetics and SN-38 exposure. J Clin Pharmacol 2015;55:1303-12. [Crossref] [PubMed]
- Urien S, Alexandre J, Raymond E, et al. Phase I population pharmacokinetics of irofulven. Anticancer Drugs 2003;14:353-8. [Crossref] [PubMed]
- Parra-Guillen ZP, Cendros Carreras JM, Peraire C, et al. Population pharmacokinetic modelling of irosustat in postmenopausal women with oestrogen-receptor positive breast cancer incorporating non-linear red blood cell uptake. Pharm Res 2015;32:1493-504. [Crossref] [PubMed]
- Gupta N, Huh Y, Hutmacher MM, et al. Integrated nonclinical and clinical risk assessment of the investigational proteasome inhibitor ixazomib on the QTc interval in cancer patients. Cancer Chemother Pharmacol 2015;76:507-16. [Crossref] [PubMed]
- Miguel-Lillo B, Valenzuela B, Peris-Ribera JE, et al. Population pharmacokinetics of kahalalide F in advanced cancer patients. Cancer Chemother Pharmacol 2015;76:365-74. [Crossref] [PubMed]
- Hudachek SF, Gustafson DL. Physiologically based pharmacokinetic model of lapatinib developed in mice and scaled to humans. J Pharmacokinet Pharmacodyn 2013;40:157-76. [Crossref] [PubMed]
- Rezai K, Urien S, Isambert N, et al. Pharmacokinetic evaluation of the vinorelbine-lapatinib combination in the treatment of breast cancer patients. Cancer Chemother Pharmacol 2011;68:1529-36. [Crossref] [PubMed]
- Gupta A, Jarzab B, Capdevila J, et al. Population pharmacokinetic analysis of lenvatinib in healthy subjects and patients with cancer. Br J Clin Pharmacol 2016;81:1124-33. [Crossref] [PubMed]
- Tanii H, Shitara Y, Horie T. Population pharmacokinetic analysis of letrozole in Japanese postmenopausal women. Eur J Clin Pharmacol 2011;67:1017-25. [Crossref] [PubMed]
- Lim CN, Salem AH. A semi-mechanistic integrated pharmacokinetic/pharmacodynamic model of the testosterone effects of the gonadotropin-releasing hormone agonist leuprolide in prostate cancer patients. Clin Pharmacokinet 2015;54:963-73. [Crossref] [PubMed]
- Salem AH, Koenig D, Carlson D. Pooled population pharmacokinetic analysis of phase I, II and III studies of linifanib in cancer patients. Clin Pharmacokinet 2014;53:347-59. [Crossref] [PubMed]
- Chiu YL, Carlson DM, Pradhan RS, et al. Exposure-response (safety) analysis to identify linifanib dose for a Phase III study in patients with hepatocellular carcinoma. Clin Ther 2013;35:1770-7. [Crossref] [PubMed]
- Koizumi K, DeNardo GL, DeNardo SJ, et al. Multicompartmental analysis of the kinetics of radioiodinated monoclonal antibody in patients with cancer. J Nucl Med 1986;27:1243-54. [PubMed]
- Kuester K, Kovar A, Lupfert C, et al. Refinement of the population pharmacokinetic model for the monoclonal antibody matuzumab: external model evaluation and simulations. Clin Pharmacokinet 2009;48:477-87. [Crossref] [PubMed]
- Kuester K, Kovar A, Lupfert C, et al. Population pharmacokinetic data analysis of three phase I studies of matuzumab, a humanised anti-EGFR monoclonal antibody in clinical cancer development. Br J Cancer 2008;98:900-6. [Crossref] [PubMed]
- Mougenot P, Pinguet F, Fabbro M, et al. Population pharmacokinetics of melphalan, infused over a 24-hour period, in patients with advanced malignancies. Cancer Chemother Pharmacol 2004;53:503-12. [Crossref] [PubMed]
- Smith DC, Jodrell DI, Egorin MJ, et al. Phase II trial and pharmacokinetic assessment of intravenous melphalan in patients with advanced prostate cancer. Cancer Chemother Pharmacol 1993;31:363-8. [Crossref] [PubMed]
- Nath CE, Shaw PJ, Montgomery K, et al. Population pharmacokinetics of melphalan in paediatric blood or marrow transplant recipients. Br J Clin Pharmacol 2007;64:151-64. [Crossref] [PubMed]
- Nath CE, Shaw PJ, Trotman J, et al. Population pharmacokinetics of melphalan in patients with multiple myeloma undergoing high dose therapy. Br J Clin Pharmacol 2010;69:484-97. [Crossref] [PubMed]
- Nath CE, Shaw PJ, Montgomery K, et al. Melphalan pharmacokinetics in children with malignant disease: influence of body weight, renal function, carboplatin therapy and total body irradiation. Br J Clin Pharmacol 2005;59:314-24. [Crossref] [PubMed]
- Piergies AA, Benson AB 3rd. Pharmacokinetics and acute cardiovascular effects of menogaril in patients with metastatic tumors. Int J Clin Pharmacol Ther 1995;33:63-9. [PubMed]
- Johansson AM, Hill N, Perisoglou M, et al. A population pharmacokinetic/pharmacodynamic model of methotrexate and mucositis scores in osteosarcoma. Ther Drug Monit 2011;33:711-8. [Crossref] [PubMed]
- Nader A, Zahran N, Alshammaa A, et al. Population Pharmacokinetics of Intravenous Methotrexate in Patients with Hematological Malignancies: Utilization of Routine Clinical Monitoring Parameters. Eur J Drug Metab Pharmacokinet 2017;42:221-8. [Crossref] [PubMed]
- Dupuis C, Mercier C, Yang C, et al. High-dose methotrexate in adults with osteosarcoma: a population pharmacokinetics study and validation of a new limited sampling strategy. Anticancer Drugs 2008;19:267-73. [Crossref] [PubMed]
- Nagulu M, Kiran VU, Nalini Y, et al. Population pharmacokinetics of methotrexate in Indian cancer patients. Asian Pac J Cancer Prev 2010;11:403-7. [PubMed]
- Joerger M, Huitema AD, van den Bongard HJ, et al. Determinants of the elimination of methotrexate and 7-hydroxy-methotrexate following high-dose infusional therapy to cancer patients. Br J Clin Pharmacol 2006;62:71-80. [Crossref] [PubMed]
- Rump AF, Botvinik-Helling S, Theisohn M, et al. Pharmacokinetics of intraarterial mitomycin C in the chemoembolisation treatment of liver metastases. Gen Pharmacol 1996;27:669-71. [Crossref] [PubMed]
- Richard B, Launay-Iliadis MC, Iliadis A, et al. Pharmacokinetics of mitoxantrone in cancer patients treated by high-dose chemotherapy and autologous bone marrow transplantation. Br J Cancer 1992;65:399-404. [Crossref] [PubMed]
- Xin Y, Li J, Wu J, et al. Pharmacokinetic and pharmacodynamic analysis of circulating biomarkers of anti-NRP1, a novel antiangiogenesis agent, in two phase I trials in patients with advanced solid tumors. Clin Cancer Res 2012;18:6040-8. [Crossref] [PubMed]
- Claret L, Lu JF, Sun YN, et al. Development of a modeling framework to simulate efficacy endpoints for motesanib in patients with thyroid cancer. Cancer Chemother Pharmacol 2010;66:1141-9. [Crossref] [PubMed]
- Lu JF, Claret L, Sutjandra L, et al. Population pharmacokinetic/pharmacodynamic modeling for the time course of tumor shrinkage by motesanib in thyroid cancer patients. Cancer Chemother Pharmacol 2010;66:1151-8. [Crossref] [PubMed]
- Gosselin NH, Mouksassi MS, Lu JF, et al. Population pharmacokinetic modeling of motesanib and its active metabolite, M4, in cancer patients. Clin Pharmacol Drug Dev 2015;4:463-72. [Crossref] [PubMed]
- Chen N, Brachmann C, Liu X, et al. Albumin-bound nanoparticle (nab) paclitaxel exhibits enhanced paclitaxel tissue distribution and tumor penetration. Cancer Chemother Pharmacol 2015;76:699-712. [Crossref] [PubMed]
- Chen N, Li Y, Ye Y, et al. Pharmacokinetics and pharmacodynamics of nab-paclitaxel in patients with solid tumors: disposition kinetics and pharmacology distinct from solvent-based paclitaxel. J Clin Pharmacol 2014;54:1097-107. [Crossref] [PubMed]
- Kaefer A, Yang J, Noertersheuser P, et al. Mechanism-based pharmacokinetic/pharmacodynamic meta-analysis of navitoclax (ABT-263) induced thrombocytopenia. Cancer Chemother Pharmacol 2014;74:593-602. [Crossref] [PubMed]
- Rodriguez-Vera L, Ramos-Suzarte M, Fernandez-Sanchez E, et al. Semimechanistic model to characterize nonlinear pharmacokinetics of nimotuzumab in patients with advanced breast cancer. J Clin Pharmacol 2015;55:888-98. [Crossref] [PubMed]
- Bajaj G, Wang X, Agrawal S, et al. Model-Based Population Pharmacokinetic Analysis of Nivolumab in Patients With Solid Tumors. CPT Pharmacometrics Syst Pharmacol 2017;6:58-66. [Crossref] [PubMed]
- Neville K, Blaney S, Bernstein M, et al. Pharmacokinetics of O(6)-benzylguanine in pediatric patients with central nervous system tumors: a pediatric oncology group study. Clin Cancer Res 2004;10:5072-5. [Crossref] [PubMed]
- Xin Y, Jin D, Eppler S, et al. Population pharmacokinetic analysis from phase I and phase II studies of the humanized monovalent antibody, onartuzumab (MetMAb), in patients with advanced solid tumors. J Clin Pharmacol 2013;53:1103-11. [PubMed]
- Brown K, Comisar C, Witjes H, et al. Population pharmacokinetics and exposure-response of osimertinib in patients with non-small cell lung cancer. Br J Clin Pharmacol 2017;83:1216-26. [Crossref] [PubMed]
- Chalret du Rieu Q, White-Koning M, Picaud L, et al. Population pharmacokinetics of peritoneal, plasma ultrafiltrated and protein-bound oxaliplatin concentrations in patients with disseminated peritoneal cancer after intraperitoneal hyperthermic chemoperfusion of oxaliplatin following cytoreductive surgery: correlation between oxaliplatin exposure and thrombocytopenia. Cancer Chemother Pharmacol 2014;74:571-82. [Crossref] [PubMed]
- Bastian G, Barrail A, Urien S. Population pharmacokinetics of oxaliplatin in patients with metastatic cancer. Anticancer Drugs 2003;14:817-24. [Crossref] [PubMed]
- Nikanjam M, Stewart CF, Takimoto CH, et al. Population pharmacokinetic analysis of oxaliplatin in adults and children identifies important covariates for dosing. Cancer Chemother Pharmacol 2015;75:495-503. [Crossref] [PubMed]
- Delord JP, Umlil A, Guimbaud R, et al. Population pharmacokinetics of oxaliplatin. Cancer Chemother Pharmacol 2003;51:127-31. [PubMed]
- Valenzuela B, Nalda-Molina R, Bretcha-Boix P, et al. Pharmacokinetic and pharmacodynamic analysis of hyperthermic intraperitoneal oxaliplatin-induced neutropenia in subjects with peritoneal carcinomatosis. AAPS J 2011;13:72-82. [Crossref] [PubMed]
- Kho Y, Jansman FG, Prins NH, et al. Population pharmacokinetics of oxaliplatin (85 mg/m2) in combination with 5-fluorouracil in patients with advanced colorectal cancer. Ther Drug Monit 2006;28:206-11. [Crossref] [PubMed]
- Lim HS, Bae KS, Jung JA, et al. Predicting the efficacy of an oral paclitaxel formulation (DHP107) through modeling and simulation. Clin Ther 2015;37:402-17. [Crossref] [PubMed]
- Bergmann TK, Brasch-Andersen C, Green H, et al. Impact of CYP2C8*3 on paclitaxel clearance: a population pharmacokinetic and pharmacogenomic study in 93 patients with ovarian cancer. Pharmacogenomics J 2011;11:113-20. [Crossref] [PubMed]
- Joerger M, Huitema AD, Huizing MT, et al. Safety and pharmacology of paclitaxel in patients with impaired liver function: a population pharmacokinetic-pharmacodynamic study. Br J Clin Pharmacol 2007;64:622-33. [Crossref] [PubMed]
- Joerger M, Huitema AD, van den Bongard DH, et al. Quantitative effect of gender, age, liver function, and body size on the population pharmacokinetics of paclitaxel in patients with solid tumors. Clin Cancer Res 2006;12:2150-7. [Crossref] [PubMed]
- Henningsson A, Marsh S, Loos WJ, et al. Association of CYP2C8, CYP3A4, CYP3A5, and ABCB1 polymorphisms with the pharmacokinetics of paclitaxel. Clin Cancer Res 2005;11:8097-104. [Crossref] [PubMed]
- Henningsson A, Sparreboom A, Sandstrom M, et al. Population pharmacokinetic modelling of unbound and total plasma concentrations of paclitaxel in cancer patients. Eur J Cancer 2003;39:1105-14. [Crossref] [PubMed]
- van Zuylen L, Karlsson MO, Verweij J, et al. Pharmacokinetic modeling of paclitaxel encapsulation in Cremophor EL micelles. Cancer Chemother Pharmacol 2001;47:309-18. [Crossref] [PubMed]
- Karlsson MO, Molnar V, Freijs A, et al. Pharmacokinetic models for the saturable distribution of paclitaxel. Drug Metabolism and Disposition 1999;27:1220-3. [PubMed]
- Karlsson MO, Molnar V, Bergh J, et al. A general model for time-dissociated pharmacokinetic-pharmacodynamic relationship exemplified by paclitaxel myelosuppression. Clin Pharmacol Ther 1998;63:11-25. [Crossref] [PubMed]
- Mross K, Hollander N, Hauns B, et al. The pharmacokinetics of a 1-h paclitaxel infusion. Cancer Chemother Pharmacol 2000;45:463-70. [Crossref] [PubMed]
- Ye M, Zhu Z, Fu Q, et al. Nonlinear pharmacokinetics of paclitaxel in ovarian cancer patients. Acta Pharmacol Sin 2000;21:596-9. [PubMed]
- Bulitta JB, Zhao P, Arnold RD, et al. Mechanistic population pharmacokinetics of total and unbound paclitaxel for a new nanodroplet formulation versus Taxol in cancer patients. Cancer Chemother Pharmacol 2009;63:1049-63. [Crossref] [PubMed]
- Fetterly GJ, Grasela TH, Sherman JW, et al. Pharmacokinetic/pharmacodynamic modeling and simulation of neutropenia during phase I development of liposome-entrapped paclitaxel. Clin Cancer Res 2008;14:5856-63. [Crossref] [PubMed]
- Joerger M, Huitema AD, Richel DJ, et al. Population pharmacokinetics and pharmacodynamics of paclitaxel and carboplatin in ovarian cancer patients: a study by the European organization for research and treatment of cancer-pharmacology and molecular mechanisms group and new drug development group. Clin Cancer Res 2007;13:6410-8. [Crossref] [PubMed]
- Henningsson A, Karlsson MO, Viganò L, et al. Mechanism-based pharmacokinetic model for paclitaxel. J Clin Oncol 2001;19:4065-73. [Crossref] [PubMed]
- Gianni L, Kearns CM, Giani A, et al. Nonlinear Pharmacokinetics and Metabolism of Paclitaxel and Its Pharmacokinetic/Pharmacodynamic Relationships in Humans. J Clin Oncol 1995;13:180-90. [Crossref] [PubMed]
- Sun W, O'Dwyer PJ, Finn RS, et al. Characterization of Neutropenia in Advanced Cancer Patients Following Palbociclib Treatment Using a Population Pharmacokinetic-Pharmacodynamic Modeling and Simulation Approach. J Clin Pharmacol 2017;57:1159-73. [Crossref] [PubMed]
- Ma PM, Yang BB, Wang YM, et al. Population Pharmacokinetic Analysis of Panitumumab in Patients With Advanced Solid Tumors. J Clin Pharmacol 2009;49:1142-56. [Crossref] [PubMed]
- Yoshiba S, Jansen M, Matsushima N, et al. Population pharmacokinetic analysis of patritumab, a HER3 inhibitor, in subjects with advanced non-small cell lung cancer (NSCLC) or solid tumors. Cancer Chemother Pharmacol 2016;77:987-96. [Crossref] [PubMed]
- Xia B, Heimbach T, Lin TH, et al. Novel physiologically based pharmacokinetic modeling of patupilone for human pharmacokinetic predictions. Cancer Chemother Pharmacol 2012;69:1567-82. [Crossref] [PubMed]
- Suttle AB, de Souza P, Arumugham T. Bayesian methods for pharmacokinetic/pharmacodynamic modeling of pazopanib-induced increases in blood pressure and transaminases. J Clin Pharmacol 2013;53:377-84. [Crossref] [PubMed]
- Yu H, van Erp N, Bins S, et al. Development of a Pharmacokinetic Model to Describe the Complex Pharmacokinetics of Pazopanib in Cancer Patients. Clin Pharmacokinet 2017;56:293-303. [Crossref] [PubMed]
- Latz JE, Karlsson MO, Rusthoven JJ, et al. A semimechanistic-physiologic population pharmacokinetic/pharmacodynamic model for neutropenia following pemetrexed therapy. Cancer Chemother Pharmacol 2006;57:412-26. [Crossref] [PubMed]
- Latz JE, Chaudhary A, Ghosh A, et al. Population pharmacokinetic analysis of ten phase II clinical trials of pemetrexed in cancer patients. Cancer Chemother Pharmacol 2006;57:401-11. [Crossref] [PubMed]
- Ouellet D, Periclou AP, Johnson RD, et al. Population pharmacokinetics of pemetrexed disodium (ALIMTA) in patients with cancer. Cancer Chemother Pharmacol 2000;46:227-34. [Crossref] [PubMed]
- Ng CM, Lum BL, Gimenez V, et al. Rationale for fixed dosing of pertuzumab in cancer patients based on population pharmacokinetic analysis. Pharm Res 2006;23:1275-84. [Crossref] [PubMed]
- Quartino AL, Li H, Jin JY, et al. Pharmacokinetic and exposure-response analyses of pertuzumab in combination with trastuzumab and docetaxel during neoadjuvant treatment of HER2+ early breast cancer. Cancer Chemother Pharmacol 2017;79:353-61. [Crossref] [PubMed]
- Thompson P, Balis F, Serabe BM, et al. Pharmacokinetics of phenylacetate administered as a 30-min infusion in children with refractory cancer. Cancer Chemother Pharmacol 2003;52:417-23. [Crossref] [PubMed]
- Hudachek SF, Eckhardt SG, Hicks B, et al. Population pharmacokinetic model of PI-88, a heparanase inhibitor. Cancer Chemother Pharmacol 2010;65:743-53. [Crossref] [PubMed]
- Nalda-Molina R, Valenzuela B, Ramon-Lopez A, et al. Population pharmacokinetics meta-analysis of plitidepsin (Aplidin) in cancer subjects. Cancer Chemother Pharmacol 2009;64:97-108. [Crossref] [PubMed]
- González-Sales M, Valenzuela B, Pérez-Ruixo C, et al. Population pharmacokinetic-pharmacodynamic analysis of neutropenia in cancer patients receiving PM00104 (Zalypsis Clin Pharmacokinet 2012;51:751-64. [Crossref] [PubMed]
- Henriksson P, Carlstrom K, Pousette A, et al. Time for revival of estrogens in the treatment of advanced prostatic carcinoma? Pharmacokinetics, and endocrine and clinical effects, of a parenteral estrogen regimen. Prostate 1999;40:76-82. [Crossref] [PubMed]
- Bellnier DA, Greco WR, Loewen GM, et al. Clinical pharmacokinetics of the PDT photosensitizers porfimer sodium (Photofrin), 2-[1-hexyloxyethyl]-2-devinyl pyropheophorbide-a (Photochlor) and 5-ALA-induced protoporphyrin IX. Lasers Surg Med 2006;38:439-44. [Crossref] [PubMed]
- Noh YH, Lim HS, Jung JA, et al. Population pharmacokinetics of HM781-36 (poziotinib), pan-human EGF receptor (HER) inhibitor, and its two metabolites in patients with advanced solid malignancies. Cancer Chemother Pharmacol 2015;75:97-109. [Crossref] [PubMed]
- Xiang H, Reyes AE 2nd, Eppler S, et al. Death receptor 5 agonistic antibody PRO95780: preclinical pharmacokinetics and concentration-effect relationship support clinical dose and regimen selection. Cancer Chemother Pharmacol 2013;72:405-15. [Crossref] [PubMed]
- Vogelzang NJ, Mick R, Janisch L, et al. Phase I and pharmacokinetic study of a new antineoplastic agent: pyrazine diazohydroxide (NSC 361456). Cancer Res 1994;54:114-9. [PubMed]
- Blair EY, Rivory LP, Clarke SJ, et al. Population pharmacokinetics of raltitrexed in patients with advanced solid tumours. Br J Clin Pharmacol 2004;57:416-26. [Crossref] [PubMed]
- Horton TM, Blaney SM, Langevin AM, et al. Phase I trial and pharmacokinetic study of raltitrexed in children with recurrent or refractory leukemia: a pediatric oncology group study. Clin Cancer Res 2005;11:1884-9. [Crossref] [PubMed]
- Paz-Ares LG, Gomez-Roca C, Delord JP, et al. Phase I pharmacokinetic and pharmacodynamic dose-escalation study of RG7160 (GA201), the first glycoengineered monoclonal antibody against the epidermal growth factor receptor, in patients with advanced solid tumors. J Clin Oncol 2011;29:3783-90. [Crossref] [PubMed]
- Zhu M, Doshi S, Gisleskog PO, et al. Population pharmacokinetics of rilotumumab, a fully human monoclonal antibody against hepatocyte growth factor, in cancer patients. J Pharm Sci 2014;103:328-36. [Crossref] [PubMed]
- Tout M, Casasnovas O, Meignan M, et al. Rituximab exposure is influenced by baseline metabolic tumor volume and predicts outcome of DLBCL patients: a Lymphoma Study Association report. Blood 2017;129:2616-23. [Crossref] [PubMed]
- Wang K, Stark FS, Schlothauer T, et al. An apparent clinical pharmacokinetic drug-drug interaction between bevacizumab and the anti-placental growth factor monoclonal antibody RO5323441 via a target-trapping mechanism. Cancer Chemother Pharmacol 2017;79:661-71. [Crossref] [PubMed]
- Patel YT, Daryani VM, Patel P, et al. Population Pharmacokinetics of Selumetinib and Its Metabolite N-desmethyl-selumetinib in Adult Patients With Advanced Solid Tumors and Children With Low-Grade Gliomas. CPT Pharmacometrics Syst Pharmacol 2017;6:305-14. [Crossref] [PubMed]
- Aoyama Y, Kaibara A, Takada A, et al. Population pharmacokinetic modeling of sepantronium bromide (YM155), a small molecule survivin suppressant, in patients with non-small cell lung cancer, hormone refractory prostate cancer, or unresectable stage III or IV melanoma. Invest New Drugs 2013;31:443-51. [Crossref] [PubMed]
- Kloft C, Graefe EU, Tanswell P, et al. Population pharmacokinetics of sibrotuzumab, a novel therapeutic monoclonal antibody, in cancer patients. Invest New Drugs 2004;22:39-52. [Crossref] [PubMed]
- Wu K, Cohen EE, House LK, et al. Nonlinear population pharmacokinetics of sirolimus in patients with advanced cancer. CPT Pharmacometrics Syst Pharmacol 2012;1:e17 [Crossref] [PubMed]
- Berg AK, Mandrekar SJ, Ziegler KL, et al. Population pharmacokinetic model for cancer chemoprevention with sulindac in healthy subjects. J Clin Pharmacol 2013;53:403-12. [Crossref] [PubMed]
- Houk BE, Bello CL, Poland B, et al. Relationship between exposure to sunitinib and efficacy and tolerability endpoints in patients with cancer: results of a pharmacokinetic/pharmacodynamic meta-analysis. Cancer Chemother Pharmacol 2010;66:357-71. [Crossref] [PubMed]
- Yu H, Steeghs N, Kloth JS, et al. Integrated semi-physiological pharmacokinetic model for both sunitinib and its active metabolite SU12662. Br J Clin Pharmacol 2015;79:809-19. [Crossref] [PubMed]
- Houk BE, Bello CL, Kang D, et al. A population pharmacokinetic meta-analysis of sunitinib malate (SU11248) and its primary metabolite (SU12662) in healthy volunteers and oncology patients. Clin Cancer Res 2009;15:2497-506. [Crossref] [PubMed]
- Lindauer A, Di Gion P, Kanefendt F, et al. Pharmacokinetic/pharmacodynamic modeling of biomarker response to sunitinib in healthy volunteers. Clin Pharmacol Ther 2010;87:601-8. [Crossref] [PubMed]
- ter Heine R, Binkhorst L, de Graan AJ, et al. Population pharmacokinetic modelling to assess the impact of CYP2D6 and CYP3A metabolic phenotypes on the pharmacokinetics of tamoxifen and endoxifen. Br J Clin Pharmacol 2014;78:572-86. [Crossref] [PubMed]
- Lim HS, Ryu KW, Lee JH, et al. Postgastrectomy pharmacokinetic changes of S-1 in patients with localized advanced gastric cancer. J Clin Pharmacol 2015;55:926-35. [Crossref] [PubMed]
- Comets E, Ikeda K, Hoff P, et al. Comparison of the pharmacokinetics of S-1, an oral anticancer agent, in Western and Japanese patients. J Pharmacokinet Pharmacodyn 2003;30:257-83. [Crossref] [PubMed]
- Hirose T, Fujita K, Nishimura K, et al. Pharmacokinetics of S-1 and CYP2A6 genotype in Japanese patients with advanced cancer. Oncol Rep 2010;24:529-36. [Crossref] [PubMed]
- Jen JF, Cutler DL, Pai SM, et al. Population pharmacokinetics of temozolomide in cancer patients. Pharm Res 2000;17:1284-9. [Crossref] [PubMed]
- Panetta JC, Kirstein MN, Gajjar A, et al. Population pharmacokinetics of temozolomide and metabolites in infants and children with primary central nervous system tumors. Cancer Chemother Pharmacol 2003;52:435-41. [Crossref] [PubMed]
- Boni JP, Leister C, Bender G, et al. Population pharmacokinetics of CCI-779: correlations to safety and pharmacogenomic responses in patients with advanced renal cancer. Clin Pharmacol Ther 2005;77:76-89. [Crossref] [PubMed]
- Mizuno T, Fukuda T, Christians U, et al. Population pharmacokinetics of temsirolimus and sirolimus in children with recurrent solid tumours: a report from the Children's Oncology Group. Br J Clin Pharmacol 2017;83:1097-1107. [Crossref] [PubMed]
- Kitchen BJ, Balis FM, Poplack DG, et al. A pediatric phase I trial and pharmacokinetic study of thioguanine administered by continuous i.v. infusion. Clin Cancer Res 1997;3:713-7. [PubMed]
- Schaiquevich P, Panetta JC, Iacono LC, et al. Population pharmacokinetic analysis of topotecan in pediatric cancer patients. Clin Cancer Res 2007;13:6703-11. [Crossref] [PubMed]
- Montazeri A, Boucaud M, Lokiec F, et al. Population pharmacokinetics of topotecan: intraindividual variability in total drug. Cancer Chemother Pharmacol 2000;46:375-81. [Crossref] [PubMed]
- Zamboni WC, D'Argenio DZ, Stewart CF, et al. Pharmacodynamic model of topotecan-induced time course of neutropenia. Clin Cancer Res 2001;7:2301-8. [PubMed]
- Mould DR, Holford NH, Schellens JH, et al. Population pharmacokinetic and adverse event analysis of topotecan in patients with solid tumors. Clin Pharmacol Ther 2002;71:334-48. [Crossref] [PubMed]
- Klein CE, Kastrissios H, Miller AA, et al. Pharmacokinetics, pharmacodynamics and adherence to oral topotecan in myelodysplastic syndromes: a Cancer and Leukemia Group B study. Cancer Chemother Pharmacol 2006;57:199-206. [Crossref] [PubMed]
- Boucaud M, Pinguet F, Culine S, et al. Modeling plasma and saliva topotecan concentration time course using a population approach. Oncol Res 2003;13:211-9. [PubMed]
- Montazeri A, Culine S, Laguerre B, et al. Individual adaptive dosing of topotecan in ovarian cancer. Clin Cancer Res 2002;8:394-9. [PubMed]
- Hing J, Perea-Ruixo J, Stuyckens K, et al. Mechanism-based pharmacokinetic/pharmacodynamic meta-analysis of trabectedin (ET-743, Yondelis) induced neutropenia. Clin Pharmacol Ther 2008;83:130-43. [Crossref] [PubMed]
- Perez-Ruixo JJ, Zannikos P, Hirankarn S, et al. Population pharmacokinetic meta-analysis of trabectedin (ET-743, Yondelis) in cancer patients. Clin Pharmacokinet 2007;46:867-84. [Crossref] [PubMed]
- Fetterly GJ, Owen JS, Stuyckens K, et al. Semimechanistic pharmacokinetic/pharmacodynamic model for hepatoprotective effect of dexamethasone on transient transaminitis after trabectedin (ET-743) treatment. Cancer Chemother Pharmacol 2008;62:135-47. [Crossref] [PubMed]
- Quartino AL, Hillenbach C, Li J, et al. Population pharmacokinetic and exposure-response analysis for trastuzumab administered using a subcutaneous "manual syringe" injection or intravenously in women with HER2-positive early breast cancer. Cancer Chemother Pharmacol 2016;77:77-88. [Crossref] [PubMed]
- Eppler S, Gordon MS, Redfern CH, et al. Lack of a pharmacokinetic interaction between trastuzumab and carboplatin in the presence of docetaxel: results from a phase Ib study in patients with HER2-positive metastatic or locally advanced inoperable solid tumors. Anticancer Drugs 2015;26:448-55. [Crossref] [PubMed]
- van Hasselt JG, Boekhout AH, Beijnen JH, et al. Population Pharmacokinetic-Pharmacodynamic Analysis of Trastuzumab-Associated Cardiotoxicity. Clin Pharmacol Ther 2011;90:126-32. [Crossref] [PubMed]
- Bruno R, Washington CB, Lu JF, et al. Population pharmacokinetics of trastuzumab in patients with HER2+ metastatic breast cancer. Cancer Chemother Pharmacol 2005;56:361-9. [Crossref] [PubMed]
- Bender BC, Schaedeli-Stark F, Koch R, et al. A population pharmacokinetic/pharmacodynamic model of thrombocytopenia characterizing the effect of trastuzumab emtansine (T-DM1) on platelet counts in patients with HER2-positive metastatic breast cancer. Cancer Chemother Pharmacol 2012;70:591-601. [Crossref] [PubMed]
- Lu D, Girish S, Gao Y, et al. Population pharmacokinetics of trastuzumab emtansine (T-DM1), a HER2-targeted antibody-drug conjugate, in patients with HER2-positive metastatic breast cancer: clinical implications of the effect of covariates. Cancer Chemother Pharmacol 2014;74:399-410. [Crossref] [PubMed]
- Chudasama VL, Schaedeli Stark F, Harrold JM, et al. Semi-mechanistic population pharmacokinetic model of multivalent trastuzumab emtansine in patients with metastatic breast cancer. Clin Pharmacol Ther 2012;92:520-7. [Crossref] [PubMed]
- Li C, Wang B, Lu D, et al. Ethnic sensitivity assessment of the antibody-drug conjugate trastuzumab emtansine (T-DM1) in patients with HER2-positive locally advanced or metastatic breast cancer. Cancer Chemother Pharmacol 2016;78:547-58. [Crossref] [PubMed]
- Singh AP, Shah DK. Application of a PK-PD Modeling and Simulation-Based Strategy for Clinical Translation of Antibody-Drug Conjugates: a Case Study with Trastuzumab Emtansine (T-DM1). AAPS J 2017;19:1054-70. [Crossref] [PubMed]
- Lu D, Joshi A, Wang B, et al. An integrated multiple-analyte pharmacokinetic model to characterize trastuzumab emtansine (T-DM1) clearance pathways and to evaluate reduced pharmacokinetic sampling in patients with HER2-positive metastatic breast cancer. Clin Pharmacokinet 2013;52:657-72. [Crossref] [PubMed]
- Tornoe CW, Agerso H, Senderovitz T, et al. Population pharmacokinetic/pharmacodynamic (PK/PD) modelling of the hypothalamic-pituitary-gonadal axis following treatment with GnRH analogues. Br J Clin Pharmacol 2007;63:648-64. [Crossref] [PubMed]
- Romero E, Velez de Mendizabal N, Cendros JM, et al. Pharmacokinetic/pharmacodynamic model of the testosterone effects of triptorelin administered in sustained release formulations in patients with prostate cancer. J Pharmacol Exp Ther 2012;342:788-98. [Crossref] [PubMed]
- Lee CK, Rowinsky EK, Li J, et al. Population pharmacokinetics of troxacitabine, a novel dioxolane nucleoside analogue. Clin Cancer Res 2006;12:2158-65. [Crossref] [PubMed]
- Ng CM, Patnaik A, Beeram M, et al. Mechanism-based pharmacokinetic/pharmacodynamic model for troxacitabine-induced neutropenia in cancer patients. Cancer Chemother Pharmacol 2011;67:985-94. [Crossref] [PubMed]
- Niu J, Scheuerell C, Mehrotra S, et al. Parent-Metabolite Pharmacokinetic Modeling and Pharmacodynamics of Veliparib (ABT-888), a PARP Inhibitor, in Patients With BRCA 1/2-Mutated Cancer or PARP-Sensitive Tumor Types. J Clin Pharmacol 2017;57:977-87. [Crossref] [PubMed]
- Jones AK, Freise KJ, Agarwal SK, et al. Clinical Predictors of Venetoclax Pharmacokinetics in Chronic Lymphocytic Leukemia and Non-Hodgkin's Lymphoma Patients: a Pooled Population Pharmacokinetic Analysis. AAPS J 2016;18:1192-202. [Crossref] [PubMed]
- Moore AS, Norris R, Price G, et al. Vincristine pharmacodynamics and pharmacogenetics in children with cancer: a limited-sampling, population modelling approach. J Paediatr Child Health 2011;47:875-82. [Crossref] [PubMed]
- de Graaf SS, Bloemhof H, Vendrig DE, et al. Vincristine disposition in children with acute lymphoblastic leukemia. Med Pediatr Oncol 1995;24:235-40. [Crossref] [PubMed]
- Gidding CE, Meeuwsen-de Boer GJ, Koopmans P, et al. Vincristine pharmacokinetics after repetitive dosing in children. Cancer Chemother Pharmacol 1999;44:203-9. [Crossref] [PubMed]
- Sethi VS, Jackson DV Jr, White DR, et al. Pharmacokinetics of vincristine sulfate in adult cancer patients. Cancer Res 1981;41:3551-5. [PubMed]
- Guilhaumou R, Simon N, Quaranta S, et al. Population pharmacokinetics and pharmacogenetics of vincristine in paediatric patients treated for solid tumour diseases. Cancer Chemother Pharmacol 2011;68:1191-8. [Crossref] [PubMed]
- Wong M, Balleine RL, Blair EY, et al. Predictors of vinorelbine pharmacokinetics and pharmacodynamics in patients with cancer. J Clin Oncol 2006;24:2448-55. [Crossref] [PubMed]
- Deporte-Fety R, Simon N, Fumoleau P, et al. Population pharmacokinetics of short intravenous vinorelbine infusions in patients with metastatic breast cancer. Cancer Chemother Pharmacol 2004;53:233-8. [Crossref] [PubMed]
- Puozzo C, Gridelli C. Non-small-cell lung cancer in elderly patients: influence of age on vinorelbine oral pharmacokinetics. Clin Lung Cancer 2004;5:237-42. [Crossref] [PubMed]
- Gauvin A, Pinguet F, Culine S, et al. Bayesian estimate of vinorelbine pharmacokinetic parameters in elderly patients with advanced metastatic cancer. Clin Cancer Res 2000;6:2690-5. [PubMed]
- Sabot C, Marquet P, Debord J, et al. Bayesian pharmacokinetic estimation of vinorelbine in non-small-cell lung cancer patients. Eur J Clin Pharmacol 1998;54:171-5. [Crossref] [PubMed]
- Gauvin A, Pinguet F, Culine S, et al. A limited-sampling strategy to estimate individual pharmacokinetic parameters of vinorelbine in elderly patients with advanced metastatic cancer. Anticancer Drugs 2002;13:473-80. [Crossref] [PubMed]
- Taylor CW, Salmon SE, Satterlee WG, et al. A phase I and pharmacokinetic study of intravenous vinzolidine. Invest New Drugs 1990;8:S51-7. [Crossref] [PubMed]