Quantitative assessment of liver fibrosis and its stage in a rabbit model by using intravoxel incoherent motion diffusion-weighted imaging at a 3T magnetic resonance system
Introduction
Chronic liver fibrosis is the common clinically progressive liver disease. Under the long-term pathogenic factors to the liver, the imbalance between the generation and degradation of collagen fiber will come about. As a result, collagen fiber diffusely deposits in the liver and appears as a series of pathological and physiological changes (1,2). It is of great importance to detect and stage liver fibrosis for selecting appropriate clinical therapy and monitoring patient’s response to the intervention, because liver fibrosis is a progressive disease and patients with stages 2–4 have the potential to keep a well liver function by therapeutic approaches whereas patients with early stage (stage ≤1) should not necessarily receive clinical interventions but get rid of the causes and be monitored (3). To date, liver biopsy is still the gold standard for detecting and staging liver fibrosis. Based the METAVIR classification system as shown on pathology, the liver fibrosis has been divided into four stages from F1 to F4 (4). However, biopsy has several disadvantages, such as invasiveness, patient discomfort, complications, sampling errors, and so forth. Because of invasive nature of biopsy, a noninvasive and repeatable quantitative examination technology is demanded to estimate liver fibrosis progression (5,6).
There are several noninvasive methods for diagnosing or staging liver fibrosis, including ultrasonography, computed tomography, magnetic resonance imaging (MRI) and serological tests (7-10). Among these methods, MRI has the greatest potential to diagnose liver fibrosis since it has a variety of advantages such as good spatial or temporal resolution without radiation and injury, good repeatability of measurement, functional imaging and so forth. Until now, a number of MR techniques, such as T1 rho imaging, dynamic contrast enhanced MR imaging, MR elastography and diffusion weighted imaging (DWI), have been used to assess liver fibrosis (11-23). As a functional imaging, DWI could be considered as a significant and widely-used technology to accurately detect the water molecular motion in liver using the apparent diffusion coefficient (ADC). According to a research from Li et al. (23), the ADC value in fibrotic liver is lower than in healthy liver and decreases as the fibrosis progressing from stage F1 to F4. Some other studies suggested that the reason for decreasing ADC value may be due to the destruction of liver micro-circulation, and consequently, the hepatic perfusion decrease (24). In the early 1980s, Le Bihan et al. (25) firstly put forward to intravoxel incoherent motion (IVIM), they reported IVIM could simultaneously receive the changes of diffusion and perfusion by measuring D value (the true diffusion coefficient), D* value (the pseudo-diffusion coefficient) and f value (the perfusion fraction). Unlike ADC which is characteristic to reflect the combined effects of perfusion and diffusion within liver fibrosis, the major advantage of IVIM-DWI is that it allows the acquisition of diffusion and perfusion parameters at the same time, and can separately provide both measurements within corresponding liver disorder without the requirement for a further coregistration process (24,25). Among these previous literatures, D, D* and f varied widely in different liver fibrosis models, which leaded to the discrepancies of IVIM results in the published studies (26-30). As reported (31), IVIM technique has not yet been able to detect early stage of liver fibrosis. Therefore, this study aimed at utilizing IVIM-DWI to dynamically monitor the liver fibrosis in a rabbit model, and to investigate which IVIM derived parameter was the optimal index for the detection and stage of this disease.
Methods
Animal preparation and histopathologic analysis
The Institutional Committee for Animal Care at our institution approved all the experimental protocols in this study. The animals were housed and provided by our animal laboratory.
Sixty-four mature New Zealand white rabbits (39 females, 25 males), weighing 2.0–3.0 kg, were enrolled into this study and randomly divided into 2 groups. Eight and fifty-six rabbits served as normal control group and experimental group, respectively. The 56 animals in the experimental group were further randomly equally divided into 4 subgroups corresponding to the follow-up weekend after modeling liver fibrosis, and each subgroup contained 14 rabbits. As is known to all, carbon tetrachloride (CCl4) is the most widely used toxic drug for modeling liver fibrosis, and therefore, we choose CCl4 for this modeling this process. The experimental rabbits were induced with pure CCl4 by intra-peritoneal injection at 0.1 mL/kg twice a week for 12 weeks (32). The rabbits from normal control group were dealt with saline by same dose and path. To prevent the chemical peritoneal adhesion resulting from the intra-peritoneal injection, the rabbits would be treated with antibiotic when necessary. In the process of modeling liver fibrosis, daily evaluation of rabbit spiritual status was performed to ensure the health of animals.
On the 6th, 8th, 10th, and 12th weekends after modeling liver fibrosis, we randomly chose a subgroup of experimental group and two rabbits from control group to undergo upper abdomen MR examination with respiratory anesthesia during the entire study. Each chosen subject was required fasting for 8 hours before the examination. The respiratory anesthesia was induced by isoflurane with oxygen flow rate at 3 L/min and drug concentration of 4%, and then maintained with oxygen flow rate at 1.5 L/min and drug concentration of 2%. Before each MR examination, we suspended this modeling process for 3 days. To avoid the impact of respiration, abdominal belt was used to minimize MR image artifacts resulting from abdominal breathing mobility (33).
After the MR scanning was performed, this selected subgroup of experimental rabbits and two rabbits from control group were euthanized by air injection into the auricular vein, and rabbit livers were subsequently harvested for histological evaluation. We randomly cut three slices from each liver with size of 2×15×15 mm3. Liver specimens were fixed in 10% buffered formalin for 24–48 hours and paraffin embedded. Masson trichrome staining was used to identify liver fibrosis (34). Two experienced pathologists with 10- and 25-year experience in hepatic pathology, who were blinded to the MR data, worked in consensus to score the pathological specimens by referring to the METAVIR classification system as follows: F0, no fibrosis; F1, portal fibrosis without septa; F2, portal fibrosis with a few septa; F3, septal fibrosis; and F4, cirrhosis.
MRI examination
The MR examination was performed on a 3T scanner (Discovery MR 750; GE Medical Systems) with an eight-channel coil. All rabbits underwent MR examination under the respiratory anesthesia. The MRI sequences included T2-weighted axial fat-suppressed sequence (Figure 1A), T1-weighted axial LAVA-Flex mask, and axial IVIM sequence with eight b values of 0, 10, 20, 50, 100, 200, 800 and 1,000 s/mm2 for DWI. The IVIM acquisitions were based on a single-shot echo-planar imaging fat-suppressed sequence. The IVIM scanning parameters were listed as follows: repetition time (TR), 1,500 ms; echo time (TE), 63 ms; field of view (FOV), 16 cm × 16 cm; matrix, 128×128; section thickness, 3 mm; slice gap, 1 mm; number of signals excitations, 1; and flip angle, 90°. The parameters for the IVIM scanning were referred to the previous published article (34).
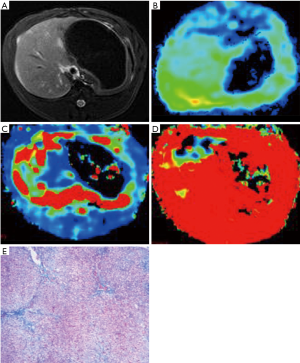
Image analysis
The original IVIM images were loaded to a post-processing workstation (GE Advanced Workstation v.4.4-09) and were analyzed with a standard software package (MADC) independently by two radiologists (the first author who had 2-year experience in radiology and the corresponding author who had 19-year experience in abdominal radiology) blinded to the animals’ pathological outcomes. Automatic pixel-wise analysis on the GE workstation was used to obtain color-coded IVIM derived parameter (D, D* and f) maps. These maps were generated according to the following equation:
Where S0 was the signal intensity for b value of 0 s/mm2, Sb was the signal intensity at the given b value, f was the perfusion fraction linked to microcirculation, D was the diffusion coefficient reflecting pure molecular diffusion, and D* was the pseudo diffusion coefficient reflecting the perfusion-related diffusion. In this GE workstation, an asymptotic fitting method was used to quantify IVIM derived parameters. Since the capillary blood flow rate is much faster than that of water diffusion, the effect of D* on the signal decay can be neglected when b value was more than 200 s/mm2. Hence, the Eq. [1] could be simplified as a linear fitting equation:
In the Eq. [2], D can be obtained by using only b values greater than 200 s/mm2 when the D value was determined, and a nonlinear regression algorithm based on equation (1) was used to calculate f and D* values.
In each liver, three freehand regions of interests (ROI, 30–50 mm2 for each) were drawn on one sectional maximal original IVIM image to perform the IVIM measurement. The previous measurement was performed on three representative sections of each liver. Therefore, there were 9 ROIs of each liver for the IVIM calculation. During the original IVIM data analysis, the same ROIs were automatically copied to the color coded maps of D, D* and f (Figure 1B-D) at the same level, avoiding areas of artifact, vessels and bile ducts by comparing with T2-weighted images. To reduce measurements’ bias, the two observers were taught to follow same rules in data analysis.
In this research, we randomly choose the IVIM data on the 10th weekend measured independently by the previous two radiologists to statistically assess the intra- and inter-observer variability. Each parameter was measured repeatedly 2 weeks later. The two measurements of the first author were used to assess the intra-observer variability, and the first measurements of the first author and the corresponding author were used to evaluate the inter-observer variability. The coefficient of variation (CV) was used to determine the precision of the measurements. An averaged CV was expressed as the resultant precision. When the averaged CV was less than 10%, intra and inter-observer variability of the IVIM parameters were considered small, and the results were considered to be reliable. The mean value of first measurements across the two observers was regarded as the final value (23). If the percentage of the averaged CV was more than 10%, the mean value of the four measurements was used as the final estimate.
Statistical analysis
The statistical analysis was performed on SPSS package version 13.0. A P value less than 0.05 was considered to be significantly different. Descriptive statistics for IVIM derived parameters were expressed as mean ± standard deviation (SD). Kruskal-Wallis test and One-way ANOVA analysis were performed to compare the three parameters among the METAVIR classifications of liver fibrosis. The correlation between the METAVIR stage of liver fibrosis and each IVIM derived parameter was performed by using Spearman’s rank correlation coefficient. Receiver operating characteristic (ROC) curve and the area under the curve (AUC) were subsequently generated to assess the diagnostic value of IVIM derived parameter for differentiating normal liver from cirrhotic liver, or between METAVIR stages.
Results
The animal models and histology findings
In the study, totally ten rabbits in the experimental group died in the process of modeling. No rabbits died in the control group. Among the dead animals in the experimental group, three rabbits died from causes irrelevant to the experimental protocol, the others died from toxic reaction. One rabbit in the experimental group did not be successfully modeled. Consequently, 45 rabbits were successfully modeled (Table 1). In the modeling process, rabbits in the experimental group showed a decreasing trend of weight from the early period of modeling to the middle period and to late period, and the weight decreased to a steady condition. The modeling outcomes of liver fibrosis were confirmed by pathology and staged by referring to the METAVIR classification system (Figures 1E,2).
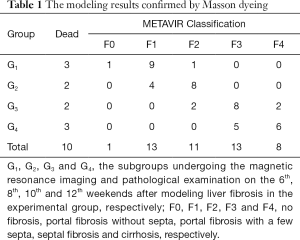
Full table
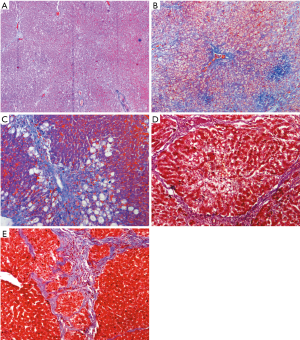
Inter and intra-observer variability of the liver IVIM derived parameters
Based on the liver IVIM derived parameters obtained from randomly chosen rabbits on the 10th weekend after modeling liver fibrosis, the mean CVs in the inter-observer measurement were 6.2% for D (range, 3.3–9.8%), 10.6% for D* (range, 4.5–18.6%) and 11% for f (range, 5.4–19.3%) for the two authors’ first measurements. And the mean CVs in the intra-observer were 6.6% for D (range, 3.3–10.6%), 10.9% for D* (range, 4.5–19.4%), and 11.6% for f (range, 5.4–19.6%) for the first author’s repeated measurements. In this study, only the CVs for inter- and intra-observer variability from D value were less than 10%. We chose the mean value of the two authors’ first measurements as the final estimate. The CVs for the intra- and inter-observer variability from D* and f values were more than 10%, the mean values of the two authors’ four measurements were regarded as the final estimate.
IVIM derived parameters corresponding to liver fibrosis stage
The IVIM derived parameters of normal liver and liver fibrosis stage were described in Table 2 and Figure 3. In this cohort, we found a decreasing trend in D, D* and f over the progression from normal liver to liver fibrosis. But according to Kruskal-Wallis test, only D was significantly different from normal liver to fibrotic liver (P=0.001), while D* or f could not discriminate normal and fibrotic liver (P=0.99 or 0.592, respectively). Spearman’s rank correlation coefficient showed a significant inverse correlation between D value and the stage of fibrosis (r=−0.605, P<0.001). According to One-Way ANOVA analysis, D could distinguish between stages F0 and F3 or F4 (both P<0.05). The ROC showed the AUC of D value to differentiate between stage F0 and F3–4 was 0.937, and the sensitivity, specificity or cut-off value were 0.667, 1 or 0.999×10-3 mm2/s, respectively.

Full table
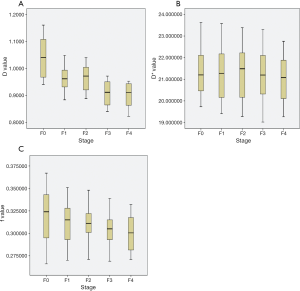
Discussion
The clinical therapy of liver fibrosis is performed according to the stages. The early stage of fibrosis could be completely reversed under the clinical intervention, which implies that the management of early liver fibrosis should concentrate on preventing the progression of this disease and avoiding clinical complications. To perform an appropriate treatment, it is very essential to stage liver fibrosis. Till now there were no noninvasive and accurate methods to stage early liver fibrosis. In our study, we aim to determine the associations of liver IVIM derived parameters and stages of liver fibrosis to explore how any previous IVIM derived parameter could help quantitatively stage this disease. As shown in our study, only D value had the potential to quantitatively monitor and stage liver fibrosis among all IVIM derived parameters.
In our research, we found that the D value trended to decrease along with liver fibrosis stages. Our finding can be similar to the previous research (35), which demonstrated that D value decreased significantly in patients with severe liver fibrosis (stage F3 or F4). However, D value had low correlation with fibrosis stages. We speculate the reason for decreased D value in liver fibrosis process is associated with the pathological mechanism of liver fibrosis. In the process from normal to cirrhotic liver, a markedly increased extra-cellular constituents and collagen deposit in the Disse space and the extra-cellular space become constricted, which will result in the limitation of Brownian motion of water within liver fibers. When Brownian motion of water becomes limited, which restricts the diffusion of water protons, thus a marked decrease of D value could be found in the progress of liver fibrosis. On the other hand, the total content of water protons within the liver fibers would decrease in this fibrotic process. Theoretically, less abundant of water and tightly bound of collagen fibers would cause the increase of liver’s stiffness, and then water diffusion restriction might contribute to the decrease of D value with the progress of liver fibrosis (36-39).
As shown in this study, the D* an f value trended to decrease with the progress of liver fibrosis, but they were not significantly associated with liver fibrosis severity, nor they could differentiate between normal and fibrotic liver. However, there is a widely recognized hypothesis that liver cirrhosis is related to the decreased liver perfusion, especially the less portal flow (24). In the advancing progression of fibrosis, the deposition of accumulated collagen leads to an increase of hepatic resistance to portal blood flow, then portal hypertension is formed, finally the portal flow decreases, whereas the increased arterial flow can not sufficiently compensate for the reduce of portal flow. And this hypothesis could have been proved in previous researches (24,34,40-42). Luciani et al. (24) showed that D* reduced significantly in liver fibrosis compared to that in healthy liver, while f was of no significant difference. In an animal experiment performed by Chow et al. (42), they also receive a similar finding with Luciani’s finding. However, Zhang et al. (34) reported that f value decreased significantly with the progression of fibrosis level in a rat model. Lu et al. (26) demonstrated that both D* and f values decreased in fibrotic liver compared with healthy liver. To date, it is hard to propose a reliable explanation for these discrepancies of the association of D* or f values with liver fibrosis. A recent research from Li et al. (31) showed the current IVIM technique is still not capable of providing reliable measurement to liver fibrosis. The factors influencing IVIM derived parameter measurement accuracy could be due to magnetic field strength, number of b values, free-breathing or respiratory triggering for data acquisition, or the image post-processing methods (31,43-45). We speculated the discrepancies from the published researches and our study could be owing to the different b values used in the IVIM-DWI data acquisition (46). As known to all, IVIM parameters were strongly dependent on the distribution of b value and threshold computed in post-process. The D* is closely related to the low b values. An optimal choice of b value should include more b values at the range from 0 to 50 s/mm2. According to ter Voert’s study (47), at least 11 b values (generally 16 b values) are required. However, we only used 8 b values in this study, and this study might not have achieved the ideal result. Previous studies have shown that among the three IVIM parameters, D has the best measurement reproducibility, followed by f, while D* tend to have poor measurement reproducibility (31).
Clinically, the therapy of liver fibrosis might focus on the reverse of early stage of fibrosis and the delaying of its progression to cirrhosis, so accurately detecting and staging the early liver fibrosis is of great importance. A study from Wáng et al. (27) suggested that a combination of the three IVIM derived parameters had the potential to detect early stage liver fibrosis and with an AUC of 1 for the differentiation between F0 and F2–4. In Lu’s study (26), although D, f and D* values decreased as the fibrosis severity progressed, however, a large overlap of the three IVIM parameters between different stages implied that IVIM could not be used to reliably differentiate fibrosis stage. In addition, the two researches could not discriminate F0 from F1 by using any IVIM parameter. In our study, we just found D value could differentiate stages F0 from F3 or F4. The three IVIM derived parameters could not differentiate F1 or F2 from any other stages, and there was a large overlap in D, f and D* between different stages. Therefore, more technical innovations are warranted for IVIM technique to be reliably applied in detecting early liver fibrosis.
Limitations of the current research include the following contents. First, the measurement on IVIM quantification could be easily influenced by the choice of b values, particularly low b value. In our study, the number of b values was only 8, which may affect the measurement accuracy. Second, the size of the enrolled samples was relatively small. It is necessary to enlarge the sample size for further studies to assess the association of IVIM parameters with the stages of liver fibrosis. Third, an animal model of liver fibrosis was involved in the current study. Compared to the process of human liver fibrosis, there might be some different pathological changes in this animal model. However, our findings could offer some useful information that the IVIM derived parameters especially D might differentiate the stage of fibrosis, which is similar to some clinical studies. The last, lack of quantitative analysis of hepatic collagen content and iron deposition were also the limitation of this study.
Conclusions
Our IVIM-DWI research shows a significant decrease of D with the progress of liver fibrosis, and D might be noninvasive and valuable for monitoring the progression of liver fibrosis in vivo sample. Due to the limited number of b values used in this study, the possibility of any IVIM derived parameter used to detect early stage of liver fibrosis is still uncertain. We will carry out an optimal IVIM-DWI study with sufficient number of b values to further make clear the uncertainty in clinical settings.
Acknowledgments
We thank Dr. Li Li and Dr. Shunhai Jian at the Department of Pathology of the Affiliated Hospital of North Sichuan Medical College for their support of this study.
Funding: This study was supported by the National Natural Science Foundation of China (Grant No. 81050033), and the Key Projects in the Sichuan Province Science & Technology Pillar Program (Grant No. 2011SZ0237).
Footnote
Provenance and Peer Review: This article was commissioned by the Guest Editors (Yì-Xiáng J. Wáng, Yong Wang) for the series “Translational Imaging in Cancer Patient Care” published in Translational Cancer Research. The article has undergone external peer review.
Conflicts of Interest: All authors have completed the ICMJE uniform disclosure form (available at http://dx.doi.org/10.21037/tcr.2017.10.34). The series “Translational Imaging in Cancer Patient Care” was commissioned by the editorial office without any funding or sponsorship. The authors have no other conflicts of interest to declare.
Ethical Statement: The authors are accountable for all aspects of the work in ensuring that questions related to the accuracy or integrity of any part of the work are appropriately investigated and resolved. The Institutional Committee for Animal Care at our institution approved all the experimental protocols in this study. The animals were housed and provided by our animal laboratory.
Open Access Statement: This is an Open Access article distributed in accordance with the Creative Commons Attribution-NonCommercial-NoDerivs 4.0 International License (CC BY-NC-ND 4.0), which permits the non-commercial replication and distribution of the article with the strict proviso that no changes or edits are made and the original work is properly cited (including links to both the formal publication through the relevant DOI and the license). See: https://creativecommons.org/licenses/by-nc-nd/4.0/.
References
- Bhat M, Ghali P, Deschenes M, et al. Prevention and management of chronic hepatitis B. Int J Prev Med 2014;5:S200-7. [PubMed]
- Povero D, Busletta C, Novo E, et al. Liver fibrosis: a dynamic and potentially reversible process. Histol Histopathol 2010;25:1075-91. [PubMed]
- Kisseleva T, Brenner DA. Anti-fibrogenic strategies and the regression of fibrosis. Best Pract Res Clin Gastroenterol 2011;25:305-17. [Crossref] [PubMed]
- The French METAVIR Cooperative Study Group. Intraobserver and interobserver variations in liver biopsy interpretation in patients with chronic hepatitis C. Hepatology 1994;20:15-20. [Crossref] [PubMed]
- Schiavon LL, Narciso-Schiavon JL, de Carvalho-Filho RJ. Noninvasive diagnosis of liver fibrosis in chronic hepatitis C. World J Gastroenterol 2014;20:2854-66. [Crossref] [PubMed]
- Szymczak A, Simon K, Inglot M, et al. Safety and effectiveness of blind percutaneous liver biopsy: analysis of 1412 procedures. Hepat Mon 2012;12:32-7. [Crossref] [PubMed]
- Patel K, Shackel NA. Current status of fibrosis markers. Curr Opin Gastroenterol 2014;30:253-9. [Crossref] [PubMed]
- Zhang L, Li QY, Duan YY, et al. Artificial neural network aided non-invasive grading evaluation of hepatic fibrosis by duplex ultrasonography. BMC Med Inform Decis Mak 2012;12:55. [Crossref] [PubMed]
- Castera L, Bedossa P. How to assess fiver fibrosis in chronic hepatitis C: serum markers or transient elastography vs. 1iver biopsy. Liver Int 2011;31:13-7. [Crossref] [PubMed]
- Ligabue G, Besutti G, Scaglioni R, et al. MR quantitative biomarkers of non- alcoholic fatty liver disease: technical evolutions and future trends. Quant Imaging Med Surg 2013;3:192-5. [PubMed]
- Wang YX, Yuan J, Chu ES, et al. T1rho MR imaging is sensitive to evaluate liver fibrosis: an experimental study in a rat biliary duct ligation model. Radiology 2011;259:712-9. [Crossref] [PubMed]
- Zhao F, Wang YX, Yuan J, et al. MR T1ρ as an imaging biomarker for monitoring liver injury progression and regression: an experimental study in rats with carbon tetrachloride intoxication. Eur Radiol 2012;22:1709-16. [Crossref] [PubMed]
- Allkemper T, Sagmeister F, Cicinnati V, et al. Evaluation of fibrotic liver disease with whole liver T1ρ MR imaging: a feasibility study at 1.5 T. Radiology 2014;271:408-15. [Crossref] [PubMed]
- Zhao F, Yuan J, Deng M, et al. Further exploration of MRI techniques for liver T1rho quantification. Quant Imaging Med Surg 2013;3:308-15. [PubMed]
- Wang YX, Yuan J. Evaluation of liver fibrosis with T1ρ MR imaging. Quant Imaging Med Surg 2014;4:152-5. [PubMed]
- Koon CM, Zhang X, Chen W, et al. Black blood T1rho MR imaging may diagnose early stage liver fibrosis: a proof-of-principle study with rat biliary duct ligation model. Quant Imaging Med Surg. 2016;6:353-63. [Crossref] [PubMed]
- Goshima S, Kanematsu M, Watanabe H, et al. Gd-EOB-DTPA-enhanced MR imaging: prediction of hepatic fibrosis stages using liver contrast enhancement index and liver-to-spleen volumetric ratio. J Magn Reson Imaging 2012;36:1148-53. [Crossref] [PubMed]
- Zhou L, Chen TW, Zhang XM, et al. Spleen dynamic contrast-enhanced magnetic resonance imaging as a new method for staging liver fibrosis in a piglet model. PLoS One 2013;8:e83697 [Crossref] [PubMed]
- Juluru K, Talal AH, Yantiss RK, et al. Diagnostic accuracy of intracellular uptake rates calculated using dynamic Gd-EOB-DTPA-enhanced MRI for hepatic fibrosis stage. J Magn Reson Imaging 2017;45:1177-85. [Crossref] [PubMed]
- Hennedige TP, Wang G, Leung FP, et al. Magnetic Resonance Elastography and Diffusion Weighted Imaging in the Evaluation of Hepatic Fibrosis in Chronic Hepatitis B. Gut Liver 2017;11:401-8. [Crossref] [PubMed]
- Wang J, Malik N, Yin M, et al. Magnetic resonance elastography is accurate in detecting advanced fibrosis in autoimmune hepatitis. World J Gastroenterol 2017;23:859-68. [Crossref] [PubMed]
- Hong Y, Shi Y, Liao W, et al. Relative ADC measurement for liver fibrosis diagnosis in chronic hepatitis B using spleen/renal cortex as the reference organs at 3 T. Clin Radiol 2014;69:581-8. [Crossref] [PubMed]
- Li H, Chen TW, Chen XL, et al. Magnetic resonance-based total liver volume and magnetic resonance-diffusion weighted imaging for staging liver fibrosis in mini-pigs. World J Gastroenterol 2012;18:7225-33. [Crossref] [PubMed]
- Luciani A, Vignaud A, Cavet M, et al. Liver cirrhosis: intravoxel incoherent motion MR imaging-pilot study. Radiology 2008;249:891-9. [Crossref] [PubMed]
- Le Bihan D, Breton E, Lallemand D, et al. MR imaging of intravoxel incoherent motions: application to diffusion and perfusion in neurologic disorders. Radiology 1986;161:401-7. [Crossref] [PubMed]
- Lu PX, Huang H, Yuan J, et al. Decreases in molecular diffusion, perfusion fraction and perfusion-related diffusion in fibrotic livers: a prospective clinical intravoxel incoherent motion MR imaging study. PLoS One 2014;9:e113846 [Crossref] [PubMed]
- Wáng YX, Deng M, Li YT, et al. A Combined Use of Intravoxel Incoherent Motion MRI Parameters Can Differentiate Early Stage Hepatitis-B Fibrotic Livers From Healthy Livers. SLAS Technol 2017; [Epub ahead of print]. [Crossref] [PubMed]
- Hu G, Chan Q, Quan X, et al. Intravoxel incoherent motion MRI evaluation for the staging of liver fibrosis in a rat model. J Magn Reson Imaging 2015;42:331-9. [Crossref]
- Chen C, Wang B, Shi D, et al. Initial study of biexponential model of intravoxel incoherent motion magnetic resonance imaging in evaluation of the liver fibrosis. Chin Med J (Engl) 2014;127:3082-7. [PubMed]
- Patel J, Sigmund EE, Rusinek H, et al. Diagnosis of cirrhosis with intravoxel incoherent motion diffusion MRI and dynamic contrast-enhanced MRI alone and in combination: preliminary experience. J Magn Reson Imaging 2010;31:589-600. [Crossref] [PubMed]
- Li YT, Cercueil JP, Yuan J, et al. Liver intravoxel incoherent motion (IVIM) magnetic resonance imaging: a comprehensive review of published data on normal values and applications for fibrosis and tumor evaluation. Quant Imaging Med Surg 2017;7:59-78. [Crossref] [PubMed]
- Zou L, Jiang J, Zhong W, et al. Magnetic resonance elastography in a rabbit model of liver fibrosis: a 3-T longitudinal validation for clinical translation. Am J Transl Res 2016;8:4922-31. [PubMed]
- Morita S, Suzuki K, Machida H, et al. Compression belt for navigator-triggered true FISP whole-heart coronary magnetic resonance angiography: study in healthy volunteers. Magn Reson Med 2008;7:9-83.
- Zhang Y, Jin N, Deng J, et al. Intra-voxel incoherent motion MRI in rodent model of diethylnitrosamine-induced liver fibrosis. Magn Reson Imaging 2013;31:1017-21. [Crossref] [PubMed]
- Yoon JH, Lee JM, Baek JH, et al. Evaluation of hepatic fibrosis using intravoxel incoherent motion in diffusion-weighted liver MRI. J Comput Assist Tomogr 2014;38:110-6. [Crossref] [PubMed]
- Hayashi T, Miyati T, Takahashi J, et al. Diffusion analysis with triexponential function in liver cirrhosis. J Magn Reson Imaging 2013;38:148-53. [Crossref] [PubMed]
- Lee JT, Liau J, Murphy P, et al. Cross-sectional investigation of correlation between hepatic steatosis and IVIM perfusion on MR imaging. Magn Reson Imaging 2012;30:572-8. [Crossref] [PubMed]
- Atzori L, Poli G, Perra A. Hepatic stellate cell: a star cell in the liver. Int J Biochem Cell Biol 2009;41:1639-42. [Crossref] [PubMed]
- Guiu B, Petit JM, Capitan V, et al. Intravoxel incoherent motion diffusion weighted imaging in nonalcoholic fatty liver disease: a 3.0-T MR study. Radiology 2012;265:96-103. [Crossref] [PubMed]
- Yamada I, Aung W, Himeno Y, et al. Diffusion coefficients in abdominal organs and hepatic lesions: evaluation with intravoxel incoherent motion echo-planar MR imaging. Radiology 1999;210:617-23. [Crossref] [PubMed]
- Hagiwara M, Rusinek H, Lee VS, et al. Advanced liver fibrosis: diagnosis with 3D whole liver perfusion MR imaging-initial experience. Radiology 2008;246:926-34. [Crossref] [PubMed]
- Chow AM, Gao DS, Fan SJ, et al. Liver fibrosis: an intravoxel incoherent motion (IVIM) study. J Magn Reson Imaging 2012;36:159-67. [Crossref] [PubMed]
- Yuan J, Wong OL, Lo GG, et al. Statistical assessment of bi-exponential diffusion weighted imaging signal characteristics induced by intravoxel incoherent motion in malignant breast tumors. Quant Imaging Med Surg 2016;6:418-29. [Crossref] [PubMed]
- Eghtedari M, Ma J, Fox P, et al. Effects of magnetic field strength and b value on the sensitivity and specificity of quantitative breast diffusion-weighted MRI. Quant Imaging Med Surg 2016;6:374-80. [Crossref] [PubMed]
- Zhang Q, Wang YX, Ma HT, et al. Cramér-Rao bound for Intravoxel Incoherent Motion Diffusion Weighted Imaging fitting. Conf Proc IEEE Eng Med Biol Soc 2013;2013:511-4. [PubMed]
- Lemke A, Stieltjes B, Schad LR, et al. Toward an optimal distribution of b values for intravoxel incoherent motion imaging. Magn Reson Imaging 2011;29:766-76. [Crossref] [PubMed]
- ter Voert EE, Delso G, Porto M, et al. Intravoxel Incoherent Motion Protocol Evaluation and Data Quality in Normal and Malignant Liver Tissue and Comparison to the Literature. Invest Radiol 2016;51:90-9. [Crossref] [PubMed]