Diagnostic application of lipidomics fingerprints to bladder carcinoma
Introduction
Urothelial carcinoma of the bladder is one of the most diffuse cancers in man and also is present in small percentage of women (Global Cancer Statistic) (1). Two clinical forms are present: non-muscle invasive bladder cancer (NMIBC) and muscle-invasive bladder cancer (MIBC) each with a different perspective for therapy and survival (2-4). The diagnosis of MIBC may be more easily on the basis of symptoms and especially of cytological analysis, instead the NMIBC are frequently asymptomatic and few cells are present in the urine as consequence only the cystoscopy may be utilized for a correct diagnosis. At initial diagnosis around 70% of the patients with urothelial cell carcinoma (UCC) of the bladder have cancers confined to the epithelium or subepithelial connective tissue (pTa, pT1 or pTis) (5). However, the low-grade bladder cancer has a high recurrence rate (50% to 70%), fortunately with low progression rate (10–15%) to muscle invasion over a 5-year period (4,6).
Therefore, in many cases the lifelong surveillance is mandatory (7). The standard methods (cystoscopy and urine cytology) for surveillance are costly, invasive and uncomfortable (7). Non-invasive urine markers might therefore significantly improve the management of NMIBC.
Therefore, many attempts have been made to find an early marker in either blood or urine. The cytological analysis of urinary cells shows the presence of atypical cells, but the success of this analysis depends on the tumor grade, on the subjective experience of the observer and may be complicated by the presence of inflammatory cells or hematuria. Some biomarkers e.g., BTA or NMP22 are used, but they do not present sufficient sensitivity or specificity (8).
Researches have been made also concerning nucleic acids, proteins, and metabolites as reported in detail by D’Costa et al., Goodison et al., Cataldi et al. (8-10).
There have been very few published analyses of lipids as potential biomarkers, although an increase in the serum lipids and proteins associated with sialic acid was demonstrated in patients, showing a sensitivity of about 80% and a specificity of 70% (11). Most of the results reported concern the cancer tissue lipid composition (12,13). In summary, there have been many proposals to reliably identify early stage bladder cancer, but to date no biomarker has been identified that may be adopted or can substitute or reduce the invasive cystoscopy examination. Therefore, aim of this preliminary study is to analyze the effectiveness of lipids as bladder cancer biomarkers in patient with papillary bladder carcinoma (Ta). Such a biomarker would need to exhibit 100% specificity and sensitivity, especially for low-grade tumors (such as Ta), when it is more difficult to analyze the cells in the urine.
In the present study, the multivariate statistical analysis on non-targeted lipidomic data was utilized to investigate urine samples. The results show that using fresh urine, it is possible to make a diagnosis of early tumor. This marker therefore, can be used for screening and prognostic purposes.
Methods
This research has been authorized by the Ethics Committee on 7th February 2013 (protocol n. 2012-047R) and the CEAS Committee of Umbria Region on 21 February 2013 (CEAS Register n 2085/13).
Patients and controls
Twenty five patients were selected from the urological clinic of the University of Perugia after they had given consent. The patients were both men (80%) and women (20%), with an average age of 70 years (41–94 years). Most of the patients were smokers, some of them for many years. No other particular risk factors were identified. Patients affected by inflammatory process or infections were excluded from the study. The tumors were classified as papillary urothelial neoplasms of low malignancy. The cytological test was positive in only 30%. Twenty-six controls were taken from healthy volunteers. They were divided in three groups on the basis of sample time conservation: controls were saved for less than 1 year, five experimental samples saved for less than 1 year and twenty experimental samples saved for 3 years, nobody followed specific diet.
Sample collection and lipid extraction and analysis
Reagents used
Phosphate buffered saline (PBS), albumin, highly polymerized calf thymus DNA-preparation, ribonucleic acid type IV from calf liver, LC-MS grade water, Acetonitrile, Methanol, Ammonium Formate, Formic Acid, Phosphoric Acid, BHT, HPLC grade chloroform and isopropanol were purchased from Sigma-Aldrich.
Urine was collected in the morning, using a 50 mL plastic vessel before any pharmacological treatment. Within 2 hours of collection, the samples were centrifuged at 600 g; the cells present in the sediment as well as the supernatant were frozen at −80 °C until they were used.
The virosomes which are particles isolated from the body fluid and containing circulating nucleic acids complex, were isolated from the voided urine by ultracentrifugation at 120,000 g for 1 hour, to remove organelles like microsomes and other cellular debris (14). Such previous researcher found the chemical composition of virosomes and it was demonstrated the presence of a lipidic fraction. The supernatant containing virosomes was used for further analysis (15). Cytosolic virosomes were isolated by gently, homogenizing the sedimented cells in PBS 1X (10 strokes with plastic pestle). The suspension was centrifuged as above for obtaining the virosomes. The supernatant was lyophilized and dialysed as described (14). The protein was determined with the colorimetric method of Lowry, using Folin reagent and albumin as standard (16), the DNA by the method of diphenylamine using DNA at scalar quantity (17). The RNA was measured with orcinol method using ribonucleic acid type IV from calf liver at scalar quantities (18).
Total lipids were extracted by the method of Folch (19) and their concentration determined by measuring inorganic phosphorus using Fiske and Subbarow method (20). The lipids were chromatographed on thin layer chromatography plates and each phospholipid (PL) was identified and measured by determining the inorganic phosphorus (21).
Extraction and LCMS analysis of urinary lipids
Urinary lipids were extracted from 6 mL urine sample aliquots of all individual patients according to the method of Matyash et al. (22) which represents an improvement of the Folch method permitting a faster and cleaner lipid recovery. The differences between lipid extraction protocols are described in Pellegrino et al. (23). Minor modification that takes into account the low lipid concentration and the salinity of the samples were introduced. The lipid extracts were reconstituted with 30 µL of methanol/MTBE/chloroform 1:1:1 (MMC) containing BHT (100 mg/L) and dicetyl phosphate (50 µM) as analytical standard to verify the reproducibility of runs. The 2 µL of each sample was injected in the Agilent LC-MS QTOF system equipped with an Agilent Dual Jet Stream source (ESI family) that operated in positive and negative ionization mode. Lipid extracts were separated on an Ascentis Express C8 2.1×100 mm 2.0 µm columns (Sigma-Aldrich, St. Louis, MO, UK) according to the method of Bird et al. (24). Specific lipid standards for each family were not used as the untargeted approach followed by multivariate statistical analysis has the purpose of making comparisons of lipids belonging to the same family.
Data analysis
Preliminary data processing was performed using the Agilent Qualitative Analysis MassHunter software (B.06.00). With this software, using the ‘find by formula’ algorithm and Lipid Maps derived database, the presence of the principal lipids class was controlled as performed by Bang et al. (25). Statistical analysis was performed using a homemade software named LipoStar that enables feature extractions from data files (each compound came with its m/z, RT and peak area), and subsequently patter recognition analyses with these data (26).
Lipid analysis
To investigate the differences of lipid content species between cancer and control samples, a consensus principal component analysis (CPCA) model was established to analyses the data. CPCA is an unsupervised clustering method employed here to gain insight into the nature of the multivariate data matrix. CPCA identifies and ranks major sources of variance within data set and allows similarity clustering of biological samples into groups based on experimental measured parameters.
CPCA was introduced as a method for comparing specified lipid classes and searching for trends that explain as much as of the variation as possible (27). The CPCA multiblock method allows comparison of the different block of variables to show their relative importance; thus each block starts with the same variance irrespective of its size (28).
Normalization of lipidomics data
According to a recent review, in lipidomics studies internal standards can be differently used based on the aim of the investigation (29). They can be added prior to the extraction (to control possible lower recoveries of specific lipids), or they can be part of the extraction solvents (to monitor the resolubilization process). Alternatively, internal standards can be added just prior to LC-MS analysis to monitor instrumental reproducibility. In the present study, the dicetyl phosphate standard was used to monitor the re-dissolution of the samples after the extraction process and the instrumental reproducibility (22). This is indeed a prerequisite of statistical multivariate analysis where the same lipid is compared among all samples, and potential errors due to signal saturation are removed.
Results
Evaluation of the isolated virosomes
The analysis of the particles presents in the voided urine and from the cell cytoplasm showed low protein and nucleic acid content and a large amount (98%) of phospholipid in both the control group and the patients (10). The nucleic acid content was lower in the patient group, but the difference was not significant and was not confirmed by the cytoplasmic results. The similarity of the results obtained by voided urine with that obtained from cells cytoplasm demonstrates that the lipid presence derives from the cells and not by kidney filtration or other sources.
The great presence of lipids in virosomes particles is a particular aspect; results obtained from the analysis of virosomes isolated from lymphocytes showed lipid fraction of only 19.9% and an increase of nucleic acids (10,14).
The lipids were practically absent in the small sediment obtained after ultracentrifugation of voided urine.
The chromatographic analysis of the phospholipids presents in the voided urine did not show any significant difference between the control and patient groups unless a small increase of the amount of sphingomyelin in the patient group. The most significative result was the presence of a large amount of lipid. Preliminary analysis highlighted some differences in the fatty acids that so indicating the need for a more detailed analysis of the lipid fraction. For this analysis, since it was demonstrated that the ultracentrifugation sediment is unaffluent, we decided to avoid ultracentrifugation which may cause a biodegradation of lipids.
LCMS analysis of urinary lipids
Samples of urine from controls and patients were analyzed both in LC/MS positive and negative ionization modes for the identification of lipid molecules. The EIC plot of all detected compounds from one control sample and one patient sample are shown in Figure 1. From the analysis of all the samples, after elimination of signal artefacts, LipoStar detected 460 peaks compatible (from exact mass and when available from MS/MS spectra) with lipid molecules. Therefore, the six control and five bladder cancer samples, saved for less than 1 year, were joined in a matrix containing 11 samples (called objects) and 460 unknown lipids molecules (named causal variables).
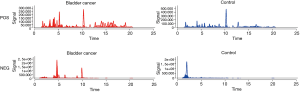
An unsupervised principal component analysis was performed on this matrix. The score plot (Figure 2A) clearly illustrates disease-dependent changes in the lipidoses of samples. It is important to point out that since CPCA is an unsupervised method, it does a pattern recognition without being influenced by the nature or information related to samples. Thus, the CPCA model in Figure 2A indicates that from the lipid analysis of urine samples it is possible to discriminate between control and bladder cancer patients on the basis of certain lipids that vary from control samples.
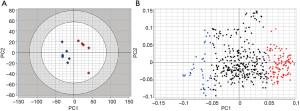
Lipids highlighted with red and blue in Figure 2A are those responsible for the differentiation between the two groups of samples in Figure 2A. However, while the red colored lipids are up regulated in bladder cancer samples, blue colored lipids are down regulated in bladder cancer patients. It is obvious to note that the up-regulated lipids in cancer can be very interesting and may represent potential biomarkers for bladder cancer. However, in the performed statistical analysis, lipids are only defined by a m/z value at a given retention time and in general, these values do not define unequivocally a single lipid or lipid class. Therefore, a structural identification of lipid species from urinary samples was required. The structural determination of each relevant molecular species (i.e., blue lipids in Figure 2B) was obtained by considering the characteristic fragments of MS/MS spectra when available (Figure 3). The procedure is exemplified in Figure 3 and Table 1.
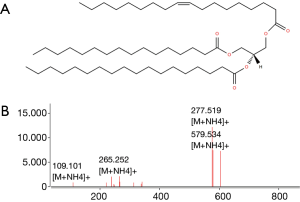
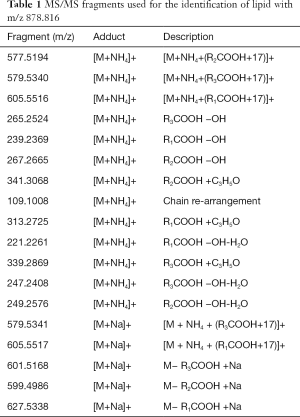
Full table
Such a procedure was automatically performed by LipoStar software for all the relevant lipids of Figure 2B and among the 150 lipids selected from the loadings plot, was able to automatically identify about 50% of them relying on the exact mass, isotopic pattern, adduct analysis and MS/MS data (Table 2). This subset of lipid variables was mainly composed of triacylglycerols, alkyl diacylglycerols, cholesteryl esters and one sphingomyelin. Their presence cannot be explained by the diet, since there is no difference respect to the control and no specific diet are used by patient.
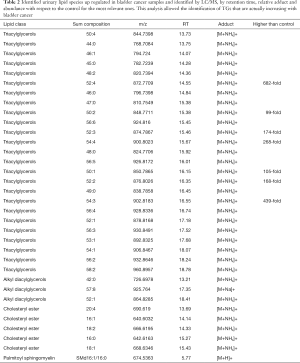
Full table
Very high doses of TGs 50:1, 50:2, 52:2, 52:3, 52:4, 54:3, 54:4 were noted in cancer patients respect to controls (values reported in Table 2; Figure 4A).
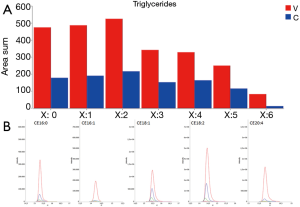
In addition, the identification analysis showed that the five identified cholesterol esters were in average three times more abundant in bladder cancer samples respect to controls (Figure 4B) and that palmitoyl-sphingomyelin was about four times more abundant in bladder cancer samples respect to control samples. The identity of a compound is obtaining checking the RT value and the MS/MS fragmentation signals, as reported Goracci et al. (30). As reported in Table 2, this untargeted approach did not pretend to identify each single lipid, but to monitor possible relative increase or decrease of lipid molecules relatively to the samples. Thus, lipid identification was obtained by MS, RT and MS/MS data. For example, in Table 2 TG are listed as sum-compositions, meaning that they were identified based on the chain fragments, but positional isomers could not be distinguished. For steryl esters, structures were identified, although the eventual position of insaturation in the chain was not distinguished. We could not distinguish between alkyl diacylglycerols and 1-alkenylacylglycerols in Table 2.
Model validation
In order to validate the procedure and the pattern recognition method developed, urinary lipid extracts from five healthy persons and five bladder cancer patients collected a few months earlier, the training samples, were characterized using exactly the same technique and parameters used in the training condition. At the same time, urinary lipid extracts from several patients collected some years before the training were also characterized. The new lipid extract groups were then merged in a new test-set matrix, peak area normalized by the same internal standard used in the training session. As before, three measurements were performed both for positive and negative ion modes of MS and joined together in a unique sample. Prediction of sample property (control or bladder cancer) was made by projection of the new matrix into the CPCA model discussed before. LipoStar software was utilized for property prediction (26).
The test set samples distance to the CPCA model of the training set reported before was displayed using a Coomans plot (31). Figure 5 demonstrates the Coomans plot for the classification of the new matrix of urine samples. The Mahalanobis distance is a useful way to determine the similarity or dissimilarity of a set of values from unknown samples to a set of values measured from a collection of known samples.
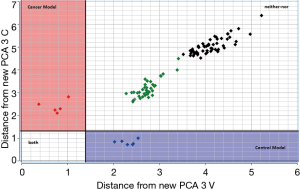
The Coomans plot clearly shows that the new test-set matrix is separated into three groups of samples. One group of samples (violet points) is positioned in the control region. Therefore, the predicted property for those patient-samples is extremely similar to those of the control in the training set. Vice versa one group of samples (magenta points) is positioned in the bladder cancer region. Therefore, the predicted property for these patient-samples is extremely similar to those of the bladder cancer patients. These points are correctly predicted and represent samples collected a few months before the training experiment model. However, it is noted that the majority of samples (reported in green and black colors) are more inclined toward the corner on the right side of the plot, indicating longer distances from both the control and bladder cancer patients. For these patients highlighted in green and black, the urine shows a different profile from the two-modelled class and therefore, for these last samples, a correct classification is not possible. This could happen when the biomatrices of training and tests are different, i.e., are obtained in different conditions, or are adulterated by external factors, or are polluted by biomolecules or by blood. Since the green and black points refer to samples collected more than 12- and 24-month before the training experiments, it could probably be that the urine samples, although correctly conserved at −80 °C, were altered by several bio transformations with a subsequent lipid profile modification. Overall, in this study, the CPCA model correctly classified 100% of all new control samples, but failed to classify ‘old’ samples, meaning that, at least for bladder cancer, classification from urine samples is possible only for samples in training and test collected in a close proximity of time.
Discussion
Previous studies on genomics, transcriptomics and metabolomics have failed to identify particular signatures related to bladder cancer (8,10).
Conversely, the lipidomic approach in urine biofluid has demonstrated the potential to provide a comprehensive understanding of lipid fingerprints in bladder cancer determination. Here, more than 150 lipid molecules were profiled to investigate global differential changes in lipid composition following bladder disease. Application of sound data pre-treatment (LipoStar), innovative multivariate statistical analysis, such as CPCA, followed by an automatic lipid identification approach helps the identification of correlations in lipids that are associated with a physiological phenotype. Moreover, a lipid-based biomarker for bladder cancer was obtained, relating differential changes in the abundances of particular lipids species with cancer samples. This study clearly shows that bladder cancer patients have a large accumulation of certain lipids in their urine.
As regard the possible role of these lipids modifications in tumor cells we can make at moment only speculative hypothesis.
Lipids have numerous functions, like chemical energy storage, but also cellular signalling, cell communications. All these processes are strongly related to carcinogenesis pathways, particularly to transformation, progression, and metastasis (12). Phospholipids regulates many functions related signal transduction, post-translational modifications, homeostasis, adhesion, migration, apoptosis (32-35). They are altered in many neoplastic diseases (36).
They may be also regulated by micro-RNAs which have the capability to control the expression of genes. Recently it was demonstrated, principally in the liver, that micro RNA is a post-transcriptional regulators of gene involved in cholesterol homeostasis and fatty acid oxidation (37).
It is also known how lipids may contribute to controlling cellular function through chromatin (38). The presence of lipids in chromatin has been demonstrated, also their role (39,40).
The fact that the increase in fatty acids is demonstrated in virosomes particles, which are secreted from the cells in body fluids suggests that the modifications may be in the chromatin lipids which may alter the gene expression and the cells proliferation. In fact the composition of virosomes lipids is similar to that of chromatin (14). The possibility of using these results for a simple diagnostic test will permit frequent and not invasive and expensive analysis. Cystoscopy is invasive and cannot repeat frequently. Urine cytology is useful in invasive tumor, but in low grade carcinoma, as that used in this research the cells present in the urine are few and may be confused with inflammatory cells (10). In our case the urine cytology was positive only in 30% of patients whereas the test indicates the presence of tumor in 100% of patients examined. Therefore this test may be useful in initial tumor diagnosis and in the recurrence since it can be repeated frequently.
Conclusions
Our results demonstrate that LC/MS along with multivariate statistical analysis can be utilized to distinguish lipid species with altered concentrations from normal urine samples compared to bladder cancer urine samples. Although the present work was made with a limited number of samples in training (11 samples) and in a validation test (10 samples) it provides a guideline to screen potential markers that, in the future, could be used as target molecules for high-speed scanning of a large number of patients. From this study, more than thirty lipid species are proposed as possible target biomolecules for bladder cancer. It was also evident that only samples conserved for less than 1 year must be used for a correct classification.
Acknowledgments
The authors are grateful to Peter B. Gahan for revising the manuscript and to “Cassa di Risparmio di Perugia” Foundation. We especially thank Prof. Mariapia Viola-Magni for the contributions of this article.
Funding: None.
Footnote
Conflicts of Interest: All authors have completed the ICMJE uniform disclosure form (available at http://dx.doi.org/10.21037/tcr.2017.11.11). The authors have no conflicts of interest to declare.
Ethical Statement: The authors are accountable for all aspects of the work in ensuring that questions related to the accuracy or integrity of any part of the work are appropriately investigated and resolved. The study was conducted in accordance with the Declaration of Helsinki (as revised in 2013). This research has been authorized by the Ethics Committee on 7th February 2013 (protocol n. 2012-047R) and the CEAS Committee of Umbria Region on 21 February 2013 (CEAS Register n 2085/13). Written informed consent was obtained from the patient for publication of this manuscript and any accompanying images.
Open Access Statement: This is an Open Access article distributed in accordance with the Creative Commons Attribution-NonCommercial-NoDerivs 4.0 International License (CC BY-NC-ND 4.0), which permits the non-commercial replication and distribution of the article with the strict proviso that no changes or edits are made and the original work is properly cited (including links to both the formal publication through the relevant DOI and the license). See: https://creativecommons.org/licenses/by-nc-nd/4.0/.
References
. Available online: https://www.cdc.gov/cancer/bladder/index.htmGlobal Cancer Statistic - Prout GR Jr, Barton BA, Griffin PP, et al. Treated history of noninvasive grade 1 transitional cell carcinoma. The National Bladder Cancer Group. J Urol 1992;148:1413-9. [Crossref] [PubMed]
- Herr HW. Tumor progression and survival of patients with high grade, noninvasive papillary (TaG3) bladder tumors: 15-year outcome. J Urol 2000;163:60-1. [Crossref] [PubMed]
- Sylvester RJ, van der Meijden AP, Oosterlinck W, et al. Predicting recurrence and progression in individual patients with stage Ta T1 bladder cancer using EORTC risk tables: a combined analysis of 2596 patients from seven EORTC trials. Eur Urol 2006;49:466-5;discussion 475-7.
- Babjuk M, Oosterlinck W, Sylvester R, et al. EAU guidelines on non–muscle-invasive urothelial carcinoma of the bladder, the 2011 update. Eur Urol 2011;59:997-1008. [Crossref] [PubMed]
- Witjes JA, Hendricksen K. Intravesical pharmacotherapy for non–muscle-invasive bladder cancer: a critical analysis of currently available drugs, treatment schedules, and long-term results. Eur Urol 2008;53:45-52. [Crossref] [PubMed]
- Van der Poel HG, Van Balken MR, Schamhart DH, et al. Bladder wash cytology, quantitative cytology, and the qualitative BTA test in patients with superficial bladder cancer. Urology 1998;51:44-50. [Crossref] [PubMed]
- D’Costa JJ, Goldsmith JC, Wilson JS, et al. A systematic review of the diagnostic and prognostic value of urinary protein biomarkers in urothelial bladder cancer. Bladder Cancer 2016;2:301-17. [Crossref] [PubMed]
- Goodison S, Rosser CJ, Urquidi V. Bladder cancer detection and monitoring: assessment of urine- and blood-based marker tests. Mol Diagn Ther 2013;17:71-84. [Crossref] [PubMed]
- Cataldi S, Marocco D, Viola-Magni M. Bladder cancer markers and recent innovations. In: M Hammad Ather, editors; Bladder Cancer - Management of NMI and Muscle-Invasive Cancer. Karaky: InTechOpen 2017;63-94.
- Llopis B, Gallego J, Cruz M, et al. Urinary lipids in vesical carcinoma: a new biological marker. Preliminary study. Eur Urol 1985;11:121-6. [Crossref] [PubMed]
- Perrotti F, Rosa C, Cicalini I, et al. Advances lipidomics for cancer biomarkers discovery. Int J Mol Sci 2016;17:1992. [Crossref] [PubMed]
- Miryaghoubzadeh J, Darabi M, Madaen K, et al. Tissue fatty acid composition in human urothelial carcinoma. Br J Biomed Sci 2013;70:1-5. [Crossref] [PubMed]
- Cataldi S, Viola-Magni M. Components of the cytosolic and released virtosomes from stimulated and non-stimulated human lymphocytes. Biochem Biophys Rep 2016;6:236-41. [Crossref] [PubMed]
- Gahan PB. Circulating nucleic acids in early diagnosis, prognosis and treatment monitoring. In: Peter B. Gahan, editor. The biology of CNAPS. London: Springer Netherlands, 2015;5:15-41.
- Lowry OH, Rosebrough NJ, Farr AL, et al. Protein measurement with the folin phenol reagent. J Biol Chem 1951;193:265-75. [PubMed]
- Burton K. A study of the conditions and mechanism of the diphenylamine reaction for the estimation of deoxyribonucleic acid. Biochem J 1956;62:315-23. [Crossref] [PubMed]
- Patterson J, Mura C. Rapid colorimetric assays to qualitatively distinguish RNA and DNA in biomolecular samples. J Vis Exp 2013;e50225 [PubMed]
- Folch J, Lees M, Sloene-Stanley GH. A simple method for the isolation and purification of total lipids from animal tissues. J Biol Chem 1957;226:497-509. [PubMed]
- Fiske CH, Subbarow Y. The colorimetric determination of phosphorus. J Biol Chem 1925;66:375-400.
- Christie WW, Hann X. Lipid Analysis: isolation, separation, identification and structural analysis of lipids. 4th editon. Buckinghamshire: Oily Press, 2010.
- Matyash V, Liebisch G, Kurzchalia TV, et al. Lipid extraction by methyl-tert-butyl ether for high-throughput lipidomics. J Lipid Res 2008;49:1137-46. [Crossref] [PubMed]
- Pellegrino RM, Di Veroli A, Valeri A, et al. LC/MS lipid profiling from human serum: a new method for global lipid extraction. Anal Bioanal Chem 2014;406:7937-48. [Crossref] [PubMed]
- Bird SS, Marur VR, Sniatynski MJ, et al. Serum lipidomics profiling using LC-MS and high-energy collisional dissociation fragmentation: focus on triglyceride detection and characterization. Anal Chem 2011;83:6648-57. [Crossref] [PubMed]
- Bang DY, Lim S, Moon MH. Effect of ionization modifiers on the simultaneous analysis of all classes of phospholipids by nanoflow liquid chromatography/tandem mass spectrometry in negative ion mode. J Chromatogr A 2012;1240:69-76. [Crossref] [PubMed]
- Lipostar software user manual. Available online: www.moldiscovery.com
- Baroni M, Costantino G, Cruciani G, et al. Generating optimal linear PLS estimations (GOLPE): an advanced chemometric tool for handling 3D-QSAR problems. Quant Struct Act Relat 1993;12:9-20. [Crossref]
- Westerhuis JA, Kourti T, MacGregor JF. Analysis of multiblock and hierarchical PCA and PLS models. J Chem 1998;12:301-21. [Crossref]
- Cajka T, Fiehn O. Comprehensive analysis of lipids in biological systems by liquid chromatography-mass spectrometry. Trends Analyt Chem 2014;61:192-206. [Crossref] [PubMed]
- Goracci L, Tortorella S, Tiberi P, et al. Lipostar, a Comprehensive Platform-Neutral Cheminformatics Tool for Lipidomics. Anal Chem. 2017;89:6257-64. [Crossref] [PubMed]
- Coomans D, Broeckaert I, Derde MP, et al. Use of a microcomputer for the definition of multivariate confidence regions in medical diagnosis based on clinical laboratory profiles. Comput Biomed Res 1984;17:1-14. [Crossref] [PubMed]
- Ivanova PT, Cerda BA, Horn DM, et al. Electrospray ionization mass spectrometry analysis of changes in phospholipids in RBL-2H3 mastocytoma cells during degranulation. Proc Natl Acad Sci U S A 2001;98:7152-7. [Crossref] [PubMed]
- Astigarraga E, Barreda-Gomez G, Lombardero L, et al. Profiling and imaging of lipids on brain and liver tissue by matrix-assisted laser desorption/ionization mass spectrometry using 2-mercaptobenzothiazole as a matrix. Anal Chem 2008;80:9105-14. [Crossref] [PubMed]
- Shevchenko A, Simons K. Lipidomics: Coming to grips with lipid diversity. Nat Rev Mol Cell Biol 2010;11:593-8. [Crossref] [PubMed]
- Wenk MR. The emerging field of lipidomics. Nat Rev Drug Discov 2005;4:594-610. [Crossref] [PubMed]
- Adibhatla RM, Hatcher JF, Dempsey RJ. Lipids and lipidomics in brain injury and diseases. AAPS J 2006;8:e314-21. [Crossref] [PubMed]
- Fernández-Hernando C, Suárez Y, Rayner KJ, et al. MicroRNAs in lipid metabolism. Curr Opin Lipidol 2011;22:86-92. [Crossref] [PubMed]
- Zhdanov R, Schirmer EC, Venkatasubramani AV, et al. Lipids contribute to epigenetic control via chromatin structure and functions. Science Open Research 2015;10:1-12.
- Albi E, Cataldi S, Rossi G, et al. A possible role of cholesterol-sphingomielin/phosphatidylcholine in nuclear matrix during rat liver regeneration. J Hepatol 2003;38:623-8. [Crossref] [PubMed]
- Albi E, Pieroni S, Viola Magni MP, et al. Chromatin sphingomyelin changes in cell proliferation and/or apoptosis induced by ciprofibrate. J Cell Physiol 2003;196:354-61. [Crossref] [PubMed]