Characterization of genomic clones using circulating tumor DNA in patients with hepatocarcinoma
Introduction
Cell-free tumor DNA (ctDNA), harboring tumor-specific mutations, is released by apoptotic and necrotic tumor cells and found in the cell-free fraction of blood (1). Plasma derived ctDNA has been recognized as a potential non-invasive surrogate for tumor tissue biopsies, also known as “liquid biopsy” (2). Massively parallel sequencing (MPS) studies of ctDNA have revealed that ctDNA is a potential marker related to various forms of human cancers (3-5). It can be used as a potential marker to predict disease risk, patient outcomes or response to treatment.
As is known, accurate and timely detection of treatment responses is pivotal because patients’ survival rates rapidly decline with late detection and delayed salvage surgery (6,7). The monitoring of treatment response is essential to avoid continuing ineffective therapies, to prevent unnecessary side effects, and to determine the benefit of new therapeutics. Treatment response is generally assessed with the use of serial imaging, but radiographic measurements often fail to detect changes in tumor burden. For example, patients with stage II or III NSCLC (non-small-cell lung cancer) undergoing definitive radiotherapy often have surveillance CT or PET-CT scans that are difficult to interpret owing to radiation-induced inflammatory and fibrotic changes in the lung and surrounding tissues. Plasma protein biomarkers (AFP, CEA, PSA, and CA15-3) are also commonly used in clinical management to reflect treatment responses of patients (8,9). However, the application of plasma protein biomarkers is limited because of low sensitivity and poor clinical correlations. The positive proportion of patients for these biomarkers is usually between 50–70% in cancer patients. Furthermore, it has also been reported that these biomarkers can also be found in lower concentrations in the serum of individuals without cancer (10,11). MPS studies of multiregional and repeated metastatic tumour biopsies analysis have been applied to guide choice and sequence of therapy (12,13). However, this is challenging due to tumor heterogeneity, which limited efficacy and duration of response to treatment. In this case the analysis of ctDNA could provide a whole picture of somatic mutations in a patient, avoiding the need to perform repeated invasive biopsy procedures. Using ctDNA to evaluate tumor heterogeneity as a prognostic factor and monitor therapeutic response in patients could guide choice and therapy. Liver cancer is one of the most common cancers and cause of cancer related death in China (14). However, studies comparing before and after treatment plasma samples of HCC patients to establish the extent of clonal heterogeneity captured in ctDNA is extremely limited.
In this study, we performed a Bayesian clustering analysis of preoperative and postoperative plasma samples collected from four HCC patients. We detected changes of genomic clones between preoperative and postoperative plasma samples. We identified reductions in cellular prevalences of private mutation clusters between preoperative and postoperative plasma samples, which correlated with disease status after surgery. In addition, we identified expansion and sharing sub-clonality of initially minor clones in plasma samples, and located mutation clusters which might be used to guide therapy. These results, from four patients with HCC, suggested that ctDNA could be used to reflect clonal population structures and captures sub-clonal dynamics in real time.
Methods
Data extraction
The study consisted of 4 patients with HCC from the Department of Surgery of the Prince of Wales Hospital, Hong Kong. These patients were initially recruited for the study in reference 1 (15). The maximal dimension of the tumor is 13.0 mm for HCC1, 6.2 mm for HCC2, 4.2 mm for HCC3 and 6.2 mm for HCC4. In this study, we focused on the data from the plasma ctDNA samples of the four patients, which were kindly provide by Y.M.D. Lo. The study and the protocols used were approved by the Institutional Ethics Committee of BGI (NO. BGI-IRB 15136). Written informed consent was obtained from all of the participants when enrolled in the study.
Data processing
Detailed information of the materials and methods was described in reference 1 (15). In short, after genomic DNA extraction of the 4 HCC patients, the DNA library was diluted and hybridized to the paired-end sequencing flow cells. DNA clusters were generated on a cBot cluster generation system (Illumina) with the TruSeq PE Cluster Generation Kit v2 (Illumina), followed by 51×2 or 76×2 cycles of sequencing on a HiSeq 2000 system (Illumina) with the TruSeq SBS Kit v2 (Illumina).
The analysis pipeline included data filteration, alignment, somatic variants detection, annotation and clustering analysis for both preoperative and postoperative plasma ctDNA samples (Figure 1). We sorted the bam file, and used bedtools (bedtools v2.25.0) to change the bam file to fq file (filtered some reads with the same read ID) for further analysis. The pipeline started from the clean reads generated in the above mentioned step: first, the clean reads with a length of 50 bps were mapped to the human reference genome (hg19) from the UCSC database using BWA (Burrows Wheeler Aligner). Second, after removing PCR derived duplications using Picard and realigning by GATK (http://www.broadinstitute.org/gatk/gatkdocs/org_broadinstitute_sting_gatk_walkers_indels_RealignerTargetCreator.html), the bam results were then used to do variants detection. We also included local realignment around indels. Somatic single nucleotide variants (SNVs) were identified by applying VarDict (16) with a P value threshold of 0.01. We only focused on the variants in the exome region in this study. The identified somatic variants were directly annotated by Catalogue of Somatic Mutations in Cancer (COSMIC) database (version 76), dbSNP (human_9606_b150_GRCh37p13), and dbNSFP (version 3.2 Academic, http://varianttools.sourceforge.net/Annotation/DbNSFP) using snpEff (snpEff_v4_3p_core) (17) and our own PERL scripts. After annotation, we used bam-readcount (version 0.8.0) to generate metrics at single nucleotide positions of the candidate SNVs, which were used to filter out false positive calls. Detailed filtering parameters followed the best practice guidelines. The ploidy, tumor content, and absolute copy number were estimated using Patchwork with default parameters (18).
Clustering using PyClone
After candidate non-synonymous SNVs calling and filtering, clonal population structures were identified based on variations from plasma ctDNA using Bayesian cluster with PyClone (version 0.13.0) (19). PyClone_binomial density was used to model read counts. In order to ensure convergence, MCMC sampling with iterations of 1,000,000 was used to approximate the posterior distribution of the model. We discarded clusters with fewer than two mutations assigned to them. This is mainly to exclude clusters with a single mutation, where PyClone gains no power to estimate cellular prevalence.
Statistical analysis
To assess statistical significance, we analyzed the number of tumor associated SNVs in plasma samples after treatment. In addition, the changes of cellular prevalences in preoperative and postoperative plasma samples were compared, and P values were calculated accordingly.
Results
Identification of somatic variants
We used the above mentioned pipeline to analyze the preoperative and postoperative plasma samples obtained from all 4 HCC patients. We only retained reads with an average mapping quality score of more than 30 (Phred). On average, the sequencing depth after removing of duplicate reads is 16.75-fold of the human genome in the plasma samples for the four HCC patients.
To reduce false positives, we used the following criteria in potential somatic SNV detection: (I) a mutation was kept only when the sequencing depth is more than 10-fold; (II) a mutation was kept when the variant frequency is more than 5%; (III) a mutation was kept only when it is present at less than 1% mutant allelic frequency in the paired normal sample (PBLs); (IV) only bases with Phred quality scores of more than 30 (less than 0.1% probability of a sequencing error) were kept; (V) the mutations were non-synonymous variants; (VI) we carried out analysis only focusing on the mutations which have been previously reported two or more times in COSMIC territory (http://www.sanger.ac.uk/genetics/CGP/cosmic/). This threshold was applied in order to lower the false-positive detection rate, and to only focus on tumor associated mutations. Finally, a total of 2,165 candidate non-synonymous SNVs for the 4 patients (Table 1) were selected for analysis of the number of tumor tissue associated SNVs.
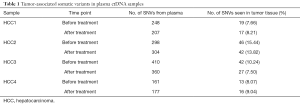
Full table
The number of tumor-associated SNVs ranged from 161 to 410 in the plasma samples of the 4 HCC patients (Table 1). In plasma samples, 7.66–15.44% of the tumor associated SNVs were detected in tumor tissue before treatment. After treatment, 7.50–13.82% of the tumor associated SNVs in the plasma samples were detected in tumor tissue. We did not detect significant reducing of the number of tumor associated SNVs in plasma samples after treatment (Paired samples t-tests, P value =0.3736). This suggested that total number of mutations cannot reflect the treatment response and monitor tumor dynamics in the 4 HCC patients. There might be new mutations detected in patients after treatment.
Mutation clusters in preoperative and postoperative plasma samples
We used PyClone (19), a Bayesian clustering method to group sets of somatic mutations into putative clonal clusters. We carried out PyClone clustering analysis focusing on all the mutations (both reported/not reported in COSMIC). All the mutations were listed in the Table (online: http://tcr.amegroups.com/public/system/tcr/supp-tcr.2018.03.17.pdf). An average of five major clusters were identified in the plasma samples of the 4 HCC patients (Figure 2). In the preoperative and postoperative plasma samples of each patient, we identified mutation clusters detected in all plasma samples, and private mutation clusters detected only in one of the plasma samples. Each patient has a large mutation cluster detected in all plasma samples (cluster 3 in HCC1, cluster 4 in HCC2, cluster 6 in HCC3, and cluster 6 in HCC4), which contain most of the mutations. We also detected 10 private mutations clusters: cluster 1, 2 in HCC1, cluster 1, 3, 4 in HCC4 and so on (Figure 2). Private mutation clusters suggested the changes in plasma samples between preoperative and postoperative plasma samples. For example, mutations with high confidence (mutations with sequencing depth of more than 30 and allele fraction >5%) in cluster 3 of HCC3 were detected mainly in the plasma sample before treatment, while most of these mutations were not detected in the plasma sample after treatment.
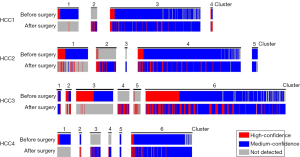
Cellular prevalence of mutation clusters
Previous studies used PyClone to infer cellular prevalence in tumour biopsies and to follow clonal dynamics in serially transplanted tumour xenografts (12,20). In this study, we used PyClone to estimate cellular prevalences in preoperative and postoperative plasma samples of the four HCC patients (Figure 3). Cellular prevalence is defined as the proportion of cancer cells with the mutation. We used PyClone to investigate the changes of estimated cellular prevalence in the preoperative and postoperative plasma samples.
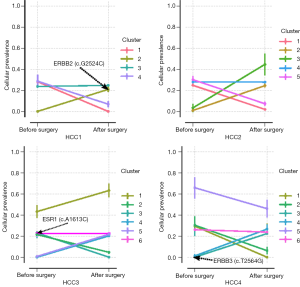
The cellular prevalence of the plasma samples were grouped into putative mutation clusters. For each of the 4 cases, we observed reductions in cellular prevalence of mutation clusters between preoperative and postoperative plasma samples (P value <0.001), which reflected the treatment responses after surgery (for example, cluster 1, 4 in HCC1, cluster 1, 5 in HCC2, cluster 2, 3 in HCC3 and cluster 1, 2, 5 in HCC4 respectively).
Notably, several cases contained mutation clusters with low (mean of 0.0005–43.27%) prevalences in the preoperative plasma samples but high (mean of 20.25–63.23%) prevalences in the postoperative plasma samples (P value <0.001) (Figure 3), implying expansion of initially minor clones. Those clones could be recognized as the emergence of treatment-resistant clones, which can be used as potential targets of therapy. We detected a mutation ERBB2-c.G2524C, which only existed in mutation cluster 2 of the postoperative plasma sample in HCC1. ERBB2, commonly referred to as HER2, is amplified and/or overexpressed in 20–30% of invasive breast carcinomas. ERBB2 (c.G2524A) was one of the first ERBB2 variants to be functionally classified (21). This mutation was shown to be an activating mutation in an in vitro assay: along with other ERBB2 activating mutations, mutation ERBB2 (c.G2524A) in MCF10A breast cancer cell lines have been shown to be sensitive to the kinase inhibitor neratinib. More recent evidence showed that HER2 activating mutations confer sensitivity to a host of tyrosine kinase inhibitors like Neratinib, Lapatinib, Trastuzumab, which means the ERBB2 (c.G2524C) mutation found in cluster 2 of the post plasma sample could be used as a target for therapy. In patient HCC4, we identified a mutation (ERBB3, c.T2564G) in cluster 3 of the post treatment plasma sample (Figure 3). A recent study reported a novel c.T2564C mutation located in exon 21 of the HER3 tyrosine kinase domain in an adolescent patient with a chemotherapy-resistant advanced NSCLC (22). Protein alignments indicated the c.T2564C mutation was analogous to EGFR-L858R and BRAF-L597V. Co-expression of wild type HER2 and HER3-c.T2564C in Ba/F3 cells led to sensitivity to afatinib and pertuzumab. This observation suggests that mutation cluster 3 in HCC4 might be used as a potential actionable target. However, the mechanism of mutation ERBB3-c.T2564G being an actionable mutation still needs further investigation.
Interestingly, we also identified large major clusters (containing an average of 333 mutations in each sample) sharing sub-clonality between the preoperative and postoperative plasma samples, which suggests the detection of direct evidence of residual disease in the form of ctDNA. This implicated that after treatment cells containing these mutations still exist. The cellular prevalences of these mutation clusters ranges between 22.55% and 27.90% (Figure 3). We identified a mutation in gene ESR1 (c.A1613C) in cluster 6 of preoperative plasma sample of HCC3. ESR1 mutations have been identified in breast cancer, endometrial, ovarian and other cancer types. Mutations in the ESR1 ligand binding domain have been shown to confer resistance to hormone therapy (23,24). This evidence has led to an increased use of targeted sequencing of the estrogen receptor in breast and ovarian cancer. Mutation c.A1613G is one of these ligand binding domain mutations, and is commonly implicated in this hormone resistance. In this study, c.A1613C mutation was detected in cluster 6 of preoperative plasma sample of HCC3. The sharing sub-clonality between the preoperative and postoperative plasma samples also suggests resistant in patient HCC3 when applying hormone therapy.
Discussion
In this study, we performed PyClone to investigate putative clonal clusters and infer cellular prevalence in plasma sample of 4 HCC patients. In total, we identified 21 clusters in the plasma ctDNA samples. We also detected serial changes in cellular prevalences of mutation clusters in preoperative and postoperative plasma samples. We found actionable targets in mutation clusters which could be used to guide therapy. The results suggest that ctDNA analysis of plasma samples can be used to investigate clonal population structures and captures sub-clonal dynamics in real time.
We used VarDict (16) to call somatic variants in this study. As is known, there are several widely used variants callers which were developed to find somatic mutations in whole genome sequencing. For example, MuTect (25) applies a probabilistic framework (Bayesian statistics) to detect somatic mutations and assess confidence in them. FreeBayes (26) is haplotype-based software, it calls variants based on the literal sequences of reads aligned to a particular target, not their precise alignment. VarScan2 (27) employs a robust heuristic/statistic approach to call somatic mutations that meet desired thresholds for read depth, base quality, variant allele frequency, and statistical significance (some statistical test like Fisher’s Exact). However, we focused on preoperative and postoperative plasma samples in this study. We compared the results of several callers, Mutect generated too much potential variants in the plasma samples compared to variants obtained from tumor tissue. The results performed by VarScan2 filtered some true variants in the plasma samples when we checking reads covering the position (data not shown). It has been reported that variant allele fraction in ctDNA can be as low as 0.01% (28). The extremely low ctDNA levels may make the above mentioned somatic mutation callers less sensitive when analyzing plasma samples. In addition, these above mentioned tools are not designed to work with sequencing data at multiple time points of the same patient. In this study, we performed VarDict to detect somatic mutations in preoperative and postoperative plasma samples, which could identify low allele frequency mutations with high sensitivity.
The average depth of the plasma samples of the four HCC patients is considered low coverage. For the purpose of PyClone analysis, less than 1,000 depth is considered low coverage. At low depth PyClone is likely to over cluster mutations as there is insufficient precision to separate clones. Single tumor sample analysis will probably not work well though. This is not PyClone specific, but an intrinsic issue of clone analysis software. So, in this study we focused on multiple samples per patient, and used binomial method, which works better and faster for low coverage samples. We discarded mutation clusters with fewer than two mutations assigned to them. Clusters with fewer than two mutations have more uncertainty about the cellular prevalence estimates than those with multiple mutations. This happens because without clustering PyClone has no idea which possible genotype the mutation has. However, in clusters with several mutations, the model can share information and infer what the common cellular prevalence is and by extension associated genotypes for mutations in the clusters are. In this study, we did not identify mutation cluster appearing as clonally dominant (prevalence ~100%). This could be because the founding point mutation was not identified due to low coverage or if the founding mutation is a larger genomic aberration. Further investigation still need to be performed here.
A recent research reported that metastatic breast cancer (mBC) patients with high heterogeneity (clonal population ≥3) in plasma ctDNA had a significantly worse PFS (HR, 2.79; 95% CI, 1.23 to 6.34; P=0.014) (29). In this study, we used PyClone to infer putative clonal clusters and cellular prevalence in plasma sample of 4 HCC patients. The more the clusters, the higher heterogeneity in plasma ctDNA. We identified 4 clusters in HCC1, 5 clusters in HCC2, and 6 clusters in HCC3 and HCC4. An average of five major clusters were identified in the plasma samples of the four HCC patients, which is more than metastatic breast cancer reported in another research (29). The size of the tumor does not appear to be correlated with the number of clusters in plasma before surgical resection. We identified only four clusters in HCC1 who had the largest tumor (13 cm) of the four HCC cases. The results could also be used as a prognostic factor to assess tumor heterogeneity in plasma ctDNA in the future, which could provide a more sensitive way to predict and monitor therapeutic response in patients with HCC. Unfortunately, we did not get the prognosis of the 4 HCC patients. As for the treatment responses of surgery, we found that reductions in cellular prevalence of mutation clusters between preoperative and postoperative plasma samples (P value <0.001), which reflected the treatment responses after surgery (for example, cluster 1, 4 in HCC1, cluster 1, 5 in HCC2, cluster 2, 3 in HCC3 and cluster 1, 2, 5 in HCC4 respectively).
Along with the development of next-generation sequencing, the research of ctDNA has become a hot spot in this field. Our analysis highlights the potential utility of clone analysis for tracking therapy effectiveness in real time. The clone analysis of circulating tumor DNA (ctDNA) could help to establish a more precise target, which could make ctDNA an informative, inherently specific, and highly sensitive biomarker for HCC.
Conclusions
Our findings provided a more complete picture of the liver cancer pathogenesis. The comparison of preoperative and postoperative plasma samples showed that ctDNA can be used to real-time sampling of clonal evolution in patients with hepatocarcinoma. In addition to dynamic monitoring of disease progression and response to therapy, characterizing of dynamics of genomic clones can be used to determine the benefit of new therapeutics and guide therapy.
Acknowledgments
We gratefully thank Y.M.D. Lo from The Chinese University of Hong Kong for providing us access to the sequencing data of the 4 HCC patients.
Funding: This work was supported by National Natural Science Foundation of China [81502593 to Y Sun].
Footnote
Conflicts of Interest: All authors have completed the ICMJE uniform disclosure form (available at http://dx.doi.org/10.21037/tcr.2018.03.17). The authors have no conflicts of interest to declare.
Ethical Statement: The authors are accountable for all aspects of the work in ensuring that questions related to the accuracy or integrity of any part of the work are appropriately investigated and resolved. The study was conducted in accordance with the Declaration of Helsinki (as revised in 2013). The study and the protocols used were approved by the Institutional Ethics Committee of BGI (NO. BGI-IRB 15136). Written informed consent was obtained from all of the participants when enrolled in the study.
Open Access Statement: This is an Open Access article distributed in accordance with the Creative Commons Attribution-NonCommercial-NoDerivs 4.0 International License (CC BY-NC-ND 4.0), which permits the non-commercial replication and distribution of the article with the strict proviso that no changes or edits are made and the original work is properly cited (including links to both the formal publication through the relevant DOI and the license). See: https://creativecommons.org/licenses/by-nc-nd/4.0/.
References
- Jahr S, Hentze H, Englisch S, et al. DNA fragments in the blood plasma of cancer patients: quantitations and evidence for their origin from apoptotic and necrotic cells. Cancer Res 2001;61:1659-65. [PubMed]
- Diaz LA Jr, Bardelli A. Liquid biopsies: genotyping circulating tumor DNA. J Clin Oncol 2014;32:579-86. [Crossref] [PubMed]
- Leary RJ, Kinde I, Diehl F, et al. Development of personalized tumor biomarkers using massively parallel sequencing. Sci Transl Med 2010;2:20ra14 [Crossref] [PubMed]
- van der Vaart M, Semenov DV, Kuligina EV, et al. Characterisation of circulating DNA by parallel tagged sequencing on the 454 platform. Clin Chim Acta 2009;409:21-7. [Crossref] [PubMed]
- Newman AM, Bratman SV, To J, et al. An ultrasensitive method for quantitating circulating tumor DNA with broad patient coverage. Nat Med 2014;20:548-54. [Crossref] [PubMed]
- Gleber-Netto FO, Braakhuis BJ, Triantafyllou A, et al. Molecular events in relapsed oral squamous cell carcinoma: Recurrence vs. secondary primary tumor. Oral Oncol 2015;51:738-44. [Crossref] [PubMed]
- Yom SS, Machtay M, Biel MA, et al. Survival impact of planned restaging and early surgical salvage following definitive chemoradiation for locally advanced squamous cell carcinomas of the oropharynx and hypopharynx. Am J Clin Oncol 2005;28:385-92. [Crossref] [PubMed]
- Tachibana M, Takemoto Y, Nakashima Y, et al. Serum carcinoembryonic antigen as a prognostic factor in resectable gastric cancer. J Am Coll Surg 1998;187:64-8. [Crossref] [PubMed]
- He CZ, Zhang KH, Li Q, et al. Combined use of AFP, CEA, CA125 and CAl9-9 improves the sensitivity for the diagnosis of gastric cancer. BMC Gastroenterol 2013;13:87. [Crossref] [PubMed]
- Ruibal Morell A. CEA serum levels in non-neoplastic disease. Int J Biol Markers 1992;7:160-6. [Crossref] [PubMed]
- Gomella LG, Liu XS, Trabulsi EJ, et al. Screening for prostate cancer: the current evidence and guidelines controversy. Can J Urol 2011;18:5875-83. [PubMed]
- Eirew P, Steif A, Khattra J, et al. Dynamics of genomic clones in breast cancer patient xenografts at single-cell resolution. Nature 2015;518:422-6. [Crossref] [PubMed]
- McPherson A, Roth A, Laks E, et al. Divergent modes of clonal spread and intraperitoneal mixing in high-grade serous ovarian cancer. Nat Genet 2016;48:758-67. [Crossref] [PubMed]
- Chen JG, Zhang SW. Liver cancer epidemic in China: past, present and future. Semin Cancer Biol 2011;21:59-69. [Crossref] [PubMed]
- Chan KC, Jiang P, Zheng YW, et al. Cancer genome scanning in plasma: detection of tumor-associated copy number aberrations, single-nucleotide variants, and tumoral heterogeneity by massively parallel sequencing. Clin Chem 2013;59:211-24. [Crossref] [PubMed]
- Lai Z, Markovets A, Ahdesmaki M, et al. VarDict: a novel and versatile variant caller for next-generation sequencing in cancer research. Nucleic Acids Res 2016;44:e108 [Crossref] [PubMed]
- Cingolani P, Platts A. A program for annotating and predicting the effects of single nucleotide polymorphisms, SnpEff: SNPs in the genome of Drosophila melanogaster strain w1118; iso-2; iso-3. Fly (Austin) 2012;6:80-92. [Crossref] [PubMed]
- Mayrhofer M, DiLorenzo S, Isaksson A. Patchwork: allele-specific copy number analysis of whole-genome sequenced tumor tissue. Genome Biol 2013;14:R24. [Crossref] [PubMed]
- Roth A, Khattra J, Yap D, et al. PyClone: statistical inference of clonal population structure in cancer. Nat Methods 2014;11:396-8. [Crossref] [PubMed]
- Shah SP, Roth A, Goya R, et al. The clonal and mutational evolution spectrum of primary triple-negative breast cancers. Nature 2012;486:395-9. [Crossref] [PubMed]
- Bose R, Kavuri SM, Searleman AC, et al. Activating HER2 mutations in HER2 gene amplification negative breast cancer. Cancer Discov 2013;3:224-37. [Crossref] [PubMed]
- Umelo I, Noeparast A, Chen G, et al. Identification of a novel HER3 activating mutation homologous to EGFR-L858R in lung cancer. Oncotarget 2016;7:3068-83. [Crossref] [PubMed]
- Robinson DR, Wu YM, Vats P, et al. Activating ESR1 mutations in hormone-resistant metastatic breast cancer. Nat Genet 2013;45:1446-51. [Crossref] [PubMed]
- Mohibi S, Mirza S, Band H, et al. Mouse models of estrogen receptor-positive breast cancer. J Carcinog 2011;10:35. [Crossref] [PubMed]
- Cibulskis K, Lawrence MS, Carter SL, et al. Sensitive detection of somatic point mutations in impure and heterogeneous cancer samples. Nat Biotechnol 2013;31:213-9. [Crossref] [PubMed]
- Garrison E, Marth G. Haplotype-based variant detection from short-read sequencing. arXiv 2012:1207.3907.
- Koboldt DC, Zhang Q, Larson DE, et al. VarScan 2: somatic mutation and copy number alteration discovery in cancer by exome sequencing. Genome Res 2012;22:568-76. [Crossref] [PubMed]
- Lipson EJ, Velculescu VE, Pritchard TS, et al. Circulating tumor DNA analysis as a real-time method for monitoring tumor burden in melanoma patients undergoing treatment with immune checkpoint blockade. J Immunother Cancer 2014;2:42. [Crossref] [PubMed]
- Ma F, Guan Y, Yi Z, et al. Assessing tumor heterogeneity using circulating tumor DNA to predict and monitor therapeutic response 9in metastatic breast cancer. J Clin Oncol 2017;35:11543.