Ambient mass spectrometry-based detection system for tumor cells in human blood
Introduction
Circulating tumor cells (CTCs), a cause of metastatic spread, are frequently observed in patients with different types of cancer (1-4). The detection of CTCs contributes to the improvement of cancer management in multiple ways and can be clinically useful in personalized medicine; for example, for monitoring therapeutic efficacy, screening for metastatic relapse, and for identification of therapeutic targets (5-8). However, CTCs are a rare population of cells, and whole blood contains a very large number of cell components, including erythrocytes, leukocytes and platelets (9). This background makes the detection of CTCs in cancer patients more challenging.
Enrichment of CTCs is required to improve the sensitivity of detection methods. Existing technologies that are commonly used for CTC enrichment in various cancers are based on immunocytochemical techniques. These use antibodies to detect cell surface proteins that are associated with the epithelial phenotype and are specifically expressed in epithelial tumor cells, and are not expressed in normal blood cells. These proteins include epithelial cell adhesion molecule (EpCAM) and cytokeratins (CK8, CK18, and CK19) (10). The “CellSearch” enrichment system, which uses antibodies coated with magnetic beads, has been in widespread use and has been approved by the U.S. Food and Drug Administration (FDA) for CTC detection in breast, colon, and prostate cancer (11).
However, there are still practical disadvantages that must be overcome in current methods. Contaminating non-tumor cells, such as granulocytes and circulating epithelial cells, have similar physical properties and cell markers to those of CTCs, which can reduce the sensitivity and accuracy of detection methods (12,13). Because of tumor heterogeneity, the utility of diagnostic tumor-markers depends on the type, phenotypic features, and progression of the tumor being targeted. Importantly, several epithelial markers on CTCs are repressed during the invasion and circulation process and during endothelial-mesenchymal transition (EMT), leading to false negative findings and a lack of reproducibility (4).
Recently, a mass spectrometry (MS)-based cancer diagnosis system has been developed and applied to clinical settings (14). In particular, ambient MS, which permits mass spectra with minimal or even no sample preparation, is a useful tool for rapid diagnosis. Probe electrospray ionization (PESI), a simple atmospheric pressure ionization method, can be applied to ambient MS (15,16). PESI-MS can be used to obtain mass spectra from biological samples, yielding information that allows discrimination of various biological conditions. We have previously used this method to demonstrate that cancerous tissues can be distinguished from non-cancerous tissues based on alterations in their spectral pattern (17-19).
Herein, we present a method of tumor cell detection using PESI-MS to detect tumor-associated metabolites in human blood. We spiked different numbers of tumor cells into whole human blood and analyzed the patterns of mass spectra from peripheral blood mononuclear cells (PBMCs) and tumor cells using PESI-MS. A total of 1,136 ion peaks were identified across a wide molecular range (m/z: 30–1,200). Spectral differences were found when comparing the mass spectra of PBMC samples with and without tumor cells added. Moreover, 16 spectral peaks were identified that were positively and negatively correlated with the number of spiked tumor cells, and were classified as “tumor associated metabolites”. These data show that PESI-MS can be used to detect tumor cells in human blood, and suggest that a PESI-MS-based detection strategy could allow rapid CTC detection without prior enrichment of tumor cells.
Methods
Cell lines
SAS cells, established from human oral squamous cell carcinoma, were purchased from the Japanese Collection of Research BioResources (Osaka, Japan). These cells were cultured as described previously (20). Trypsinized cells were counted and spiked into peripheral blood samples.
Preparation of PBMCs
This study was conducted in accordance with the ethical standards of the Declaration of Helsinki. A protocol was approved by the ethical committee of University of Yamanashi (1716), and written informed consent was obtained from all subjects. To simulate the presence of CTCs in human blood, different numbers of SAS cells (1×103–1×105) were spiked into 2 mL of human whole blood from a healthy donor. After spiking with SAS cells, the PBMCs and SAS cells were isolated by gradient centrifugation (Ficoll-Paque Premium, GE Healthcare) according to the manufacturer’s instructions. Approximately 3×106 PBMCs were isolated from each 2-mL aliquot of blood.
Immunofluorescence
Isolated PBMCs were run through a CellSieve Microfiltration Assay (Creatv MicroTech, Rockville, MD, USA) using a low-pressure filtration system (Analytical West, Corona, CA, USA) to collect floating SAS tumor cells, as described previously (21). The captured SAS tumor cells were stained with an antibody cocktail of isothiocyanate-conjugated anti-CK 8, 18, and 19, and cyanine 5-conjugated anti CD45 (Creatv MicroTech); and their fluorescence was examined using a Zeiss Axioskop2 Plus instrument (Carl Zeiss Microscopy GmbH, Jena, Germany).
MS
Isolated PBMCs with or without SAS tumor cells were washed with phosphate buffered saline and centrifuged at 800 ×g for 5 min. Two hundred microliters of 50% ethanol were added to each pellet of PBMCs in a 1.5-mL tube, and mixed by vortex for 2 min at 5 °C (Figure 1A). Eighty-five microliters of the resultant solution were directly analyzed by PESI-MS (DPiMS-2020; Shimadzu, Kyoto, Japan). Essentially, this is an ambient ionization method combined with single quadrupole MS. Details of this method and the data analysis are described previously (19,22). Positive-ion mode was used for the analyses and representative mass spectra were generated from the MS data using LabSolutions software (version 5.82 SP1; Shimadzu).
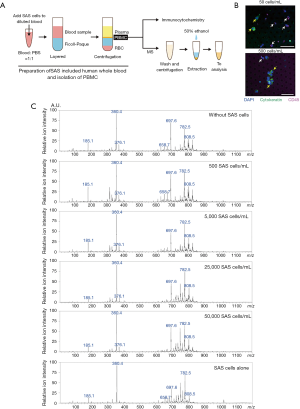
MS spectra data processing and statistical analyses
Raw mass spectra were exported from their .lcd file format to a .txt format, and imported into MATLAB (version R2013b; The MathWorks Inc., Natick, MA, USA), and the Bioinformatics Toolbox applied. To create a “peak matrix”—that is, unified peaks of multiple spectra in one matrix—the data were processed as follows: (I) the baseline was subtracted from each spectrum using the spline (msbackadj) function; (II) peaks were detected using the undecimated wavelet transform (mspeaks) function, to obtain peak lists for each spectrum; (III) the same m/z peaks from the obtained peak lists were aligned using the mspalign function, in order to create a peak matrix of 1,136 peaks; and (IV) each peak list was normalized to the ion intensity of the highest peak (m/z: 360.4).
The peak matrix was imported into the Python programming language (version 3.6; Python Software Foundation, Wilmington, DE, USA) and statistical analysis was performed using the SciPy software library. The following analyses were performed to evaluate the peak matrix: (I) the Pearson’s correlation coefficient, r, between peak lists was calculated using the Pearson function; with r taking a value from −1 to 1. If the similarity between the two peak lists used for the calculation is high, r is close to 1; (II) the Spearman’s rank correlation coefficient, 𝜌, was calculated using the spearman function, with the number of spiked SAS tumor cells (in ascending order) used for the ranking and 𝜌 taking a value from −1 to 1. If the peak intensities at the same m/z tended to decrease monotonically with the increase in spiked SAS tumor cells, 𝜌 takes a value close to −1; and if they tend to increase monotonically with increasing SAS cells, 𝜌 takes a value close to 1.
Results
Before the MS analysis, we examined whether floating SAS tumor cells can be distinguished from isolated PBMCs using conventional immunocytochemical methods (Figure 1A). Initially, different numbers of SAS tumor cells (100 and 1,000 cells) were spiked into 2 mL of whole blood from a healthy donor. After isolation of approximately 3×106 PBMCs from whole blood samples containing 50 and 500 cells/mL of SAS tumor cells, the leukocytes among the PBMCs were excluded using a CellSieve Microfiltration Assay and a low-pressure filtration system. CK8/18/19 and CD45 expression were then examined in the cells captured by the filter. As shown in Figure 1B, a small number of SAS tumor cells were detected as CD45–CK8/18/19+ cells in the PBMC samples. Therefore, we confirmed that the isolated PBMCs included the SAS tumor cells that were spiked into the whole blood samples.
For MS analysis, human whole blood samples containing different number of SAS tumor cells (0, 500, 5,000, 25,000, and 50,000 cells/mL) were prepared, and the PBMC samples were isolated by gradient centrifugation. Isolated PBMCs were analyzed by PESI-MS and representative mass spectra were obtained (Figure 1C). These mass spectra included 1,136 peaks, and the ion intensity of each peak was converted to a relative value using an ion intensity of m/z 360.4.
To further investigate whether the spiked SAS tumor cells affected the spectral pattern of PBMCs, we assessed the similarity of spectral patterns between PBMCs spiked with different numbers of SAS tumor cells, and PBMCs alone (Figure 2A). Using Pearson’s correlation analyses, the spectral patterns were compared between PBMC samples with different numbers of SAS tumor cells and PBMCs alone (Figure 2B). The Pearson’s correlation coefficient, r, was significantly reduced with an increase of SAS tumor cells (Spearman’s rank correlation: 𝜌=−0.8857, P value =0.0188; Figure 2B). This indicates that the similarity between the spectra decreases with an increasing concentration of SAS tumor cells when the spectral pattern of the PBMC sample without tumor cells is used as a reference. These data suggest that there are metabolic changes in PBMCs that are associated with the presence of tumor cells, and these changes can by recognized by PESI-MS.
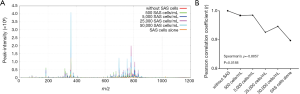
Finally, we searched for marker peaks that were specifically altered in PBMCs spiked with SAS tumor cells, and the intensity of each peak in the mass spectra is presented in Figure 3A. To examine whether there are statistical correlations between the peak intensity and the number of spiked SAS cells, Spearman’s rank-order correlation coefficients were calculated for each peak. A total of 10 (m/z: 146.1, 623.3, 651.5, 708.1, 748.4, 749.3, 762.4, 1,066.1, 1,072.0 and 1,115.9) and 6 (m/z: 376.1, 830.43, 832.4, 833.4, 835.4, and 883.6) peaks had strong positive and negative correlations between the peak intensity and the number of spiked SAS tumor cells, respectively (Figure 3B). These data suggest that the presence of SAS tumor cells among very large numbers of PBMCs might be causative for the metabolic changes indicated by the spectral peaks.
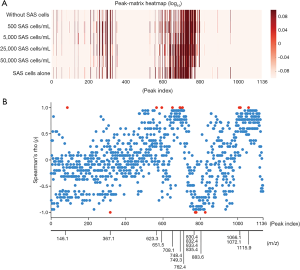
Discussion
The release of CTCs into the bloodstream is a random process that occurs from primary and metastatic sites, with the CTC half-life being 1.0–2.4 hours in the bloodstream (7,23). It has been technically challenging to detect CTCs in the bloodstream with high accuracy and high reproducibility, given that there are only 1–1,000 cells/mL of peripheral blood. Existing CTC detection technologies that focus on CTC markers are limited, due to false positive and negative results. These limitations arise because there are no universal CTC markers that are not also present on other blood cells or at times down-regulated during the EMT process (4,8).
Based on mass spectra in ambient MS analysis, we successfully identified differences in the spectral patterns between PBMCs spiked with tumor cells and PBMCs without tumor cells through comprehensive comparisons of all identified peaks, and detected the influence of a few hundred tumor cells present among very large numbers of PBMCs. No enrichment of tumor cells using antibodies for CTC-specific markers was required. These data suggest that differences in the spectral pattern obtained from PBMCs in the presence of a few hundred tumor cells can be applied to “pattern-recognition by supervised machine-learning”. After training on examples, this would be an effective computational approach to objectively analyze the spectral peaks and to identify relevant patterns that distinguish different groups. We previously reported a diagnosis algorithm for head and neck squamous cell carcinoma (HNSCC) that enables accurate discrimination of cancerous regions (17). This algorithm was based on machine-learning using a database that was constructed using mass spectra from normal mucosa and cancerous tissues. To suitably establish a highly accurate diagnosis algorithm for CTCs, a database containing a number of mass spectra from PBMCs with and without tumor cells needs to be compiled that will permit pattern recognition.
We also identified several peaks that had positive and negative correlations between the peak intensities and the number of spiked tumor cells. It is possible that these peaks represent “tumor-associated metabolites” including glycerophospholipids, sphingophospholipids, fatty acids and other metabolites, and could be utilized to detect CTCs in human blood. Alternatively, these peaks might represent metabolites produced by PBMCs that are altered by interactions with tumor cells. For example, leukocytes present among PBMCs immediately recognize tumor cells as allogeneic and target them for elimination. This process arises due to the presence of human leukocyte antigen proteins on the membranes of tumor cells. Our next challenge will be to identify the molecular species represented by the individual peaks, and thereafter confirm the presence of tumor-associated metabolites and their suitability as markers of CTCs.
Several of the conventional methods for CTC detection are problematic. Limitations include cumbersome sample preparation, long processing times, requirements for relatively large volumes of blood, and high costs of consumables and equipment. Therefore, there is a need to improve and optimize CTC-detection methods to allow for highly repeatable and reliable clinical results. Ambient MS, in particular, PESI-MS, is a valuable tool for rapid diagnosis because it requires little to no sample preparation and can acquire suitable mass spectra within 10 min. Moreover, ambient MS also allows examination and diagnosis from unknown samples.
In conclusion, our proof-of-concept experiment and results confirm that PESI-MS can be used for tumor cell detection in human blood.
Acknowledgments
We thank A. Iizuka and S. Fukuta for their assistance with this project.
Funding: This work was supported in part by KAKENHI grants from the Japan Society Promotion of Science (grant number 15K20196 to S. Takeda, 17K11374 to K. Chikamatsu, and 16K08964 to K. Yoshimura).
Footnote
Conflicts of Interest: All authors have completed the ICMJE uniform disclosure form (available at http://dx.doi.org/10.21037/tcr.2018.04.20). The authors have no conflicts of interest to declare.
Ethical Statement: The authors are accountable for all aspects of the work in ensuring that questions related to the accuracy or integrity of any part of the work are appropriately investigated and resolved. The study was conducted in accordance with the Declaration of Helsinki (as revised in 2013). This study was approved by the ethical committee of University of Yamanashi (1716), and written informed consent was obtained from all subjects.
Open Access Statement: This is an Open Access article distributed in accordance with the Creative Commons Attribution-NonCommercial-NoDerivs 4.0 International License (CC BY-NC-ND 4.0), which permits the non-commercial replication and distribution of the article with the strict proviso that no changes or edits are made and the original work is properly cited (including links to both the formal publication through the relevant DOI and the license). See: https://creativecommons.org/licenses/by-nc-nd/4.0/.
References
- Kim MY, Oskarsson T, Acharyya S, et al. Tumor self-seeding by circulating cancer cells. Cell 2009;139:1315-26. [Crossref] [PubMed]
- Cristofanilli M, Budd GT, Ellis MJ, et al. Circulating tumor cells, disease progression, and survival in metastatic breast cancer. N Engl J Med 2004;351:781-91. [Crossref] [PubMed]
- Scher HI, Jia X, de Bono JS, et al. Circulating tumour cells as prognostic markers in progressive, castration-resistant prostate cancer: a reanalysis of IMMC38 trial data. Lancet Oncol 2009;10:233-9. [Crossref] [PubMed]
- Alix-Panabières C, Pantel K. Challenges in circulating tumour cell research. Nat Rev Cancer 2014;14:623-31. [Crossref] [PubMed]
- Lohr JG, Adalsteinsson VA, Cibulskis K, et al. Whole-exome sequencing of circulating tumor cells provides a window into metastatic prostate cancer. Nat Biotechnol 2014;32:479-84. [Crossref] [PubMed]
- Polzer B, Medoro G, Pasch S, et al. Molecular profiling of single circulating tumor cells with diagnostic intention. EMBO Mol Med 2014;6:1371-86. [Crossref] [PubMed]
- Alix-Panabières C, Pantel K. Clinical Applications of Circulating Tumor Cells and Circulating Tumor DNA as Liquid Biopsy. Cancer Discov 2016;6:479-91. [Crossref] [PubMed]
- Siravegna G, Marsoni S, Siena S, et al. Integrating liquid biopsies into the management of cancer. Nat Rev Clin Oncol 2017;14:531-48. [Crossref] [PubMed]
- Zieglschmid V, Hollmann C, Böcher O. Detection of disseminated tumor cells in peripheral blood. Crit Rev Clin Lab Sci 2005;42:155-96. [Crossref] [PubMed]
- Allard WJ, Matera J, Miller MC, et al. Tumor cells circulate in the peripheral blood of all major carcinomas but not in healthy subjects or patients with nonmalignant diseases. Clin Cancer Res 2004;10:6897-904. [Crossref] [PubMed]
- Gabriel MT, Calleja LR, Chalopin A, et al. Circulating Tumor cells: A Review of Non-EpCAM-Based Approaches for Cell Enrichment and Isolation. Clin Chem 2016;62:571-81. [Crossref] [PubMed]
- Pantel K, Denève E, Nocca D, et al. Circulating epithelial cells in patients with benign colon diseases. Clin Chem 2012;58:936-40. [Crossref] [PubMed]
- Adams DL, Stefansson S, Haudenschild C, et al. Cytometric characterization of circulating tumor cells captured by microfiltration and their correlation to the CellSearch® CTC test. Cytometry A 2015;87:137-44. [Crossref] [PubMed]
- van der Merwe DE, Pikonomopoulou K, Marshall J, et al. Mass spectrometry: uncovering the cancer proteome for diagnostics. Adv Cancer Res 2007;96:23-50. [Crossref] [PubMed]
- Hiraoka K, Nishidate K, Mori K, et al. Development of probe electrospray using a solid needle. Rapid Commun Mass Spectrom 2007;21:3139-44. [Crossref] [PubMed]
- Yoshimura K, Chen LC, Asakawa D, et al. Physical properties of the probe electrospray ionization (PESI) needle applied to the biological samples. J Mass Spectrom 2009;44:978-85. [Crossref] [PubMed]
- Yoshimura K, Chen LC, Mandal MK, et al. Analysis of Renal Cell Carcinoma as a First Step for Developing Mass Spectrometry-Based Diagnostics. J Am Soc Mass Spectrom 2012;23:1741-9. [Crossref] [PubMed]
- Yoshimura K, Mandal MK, Hara M, et al. Real-time diagnosis of chemically induced hepatocellular carcinoma using a novel mass spectrometry-based technique. Anal Biochem 2013;441:32-7. [Crossref] [PubMed]
- Ashizawa K, Yoshimura K, Johno H, et al. Construction of mass spectra database and diagnosis algorithm for head and neck squamous cell carcinoma. Oral Oncol 2017;75:111-9. [Crossref] [PubMed]
- Ishii H, Saitoh M, Sakamoto K, et al. Epithelial splicing regulatory proteins 1 (ESRP1) and 2 (ESRP2) suppress cancer cell motility via different mechanisms. J Biol Chem 2014;289:27386-99. [Crossref] [PubMed]
- Kawada T, Takahashi H, Sakakura K, et al. Circulating tumor cells in patients with head and neck squamous cell carcinoma: Feasibility of detection and quantitation. Head Neck 2017;39:2180-6. [Crossref] [PubMed]
- Johno H, Yoshimura K, Mori Y, et al. Detection of potential new biomarkers of atherosclerosis by probe electrospray ionization mass spectrometry. Metabolomics 2018;14:38. [Crossref]
- Meng S, Tripathy D, Frenkel EP, et al. Circulating tumor cells in patients with breast cancer dormancy. Clin Cancer Res 2004;10:8152-62. [Crossref] [PubMed]