Risk stratification for prostate cancer via the integration of omics data of The Cancer Genome Atlas
Introduction
Prostate cancer (PCa) is one of the most common cancers in men and is the second most common cause of cancer related death in men in the Western World (1). Although the technology of diagnosing PCa has been improved in the past decades and prostate-specific antigen (PSA) level is closely related to the high incidence of prostate tumors, the management and treatment of this disease is not completely successful. In the practice, some patients with higher PSA have indolent PCa whereas death and metastasis occur in some patients with lower PSA. Recently, a newly-published study suggested alkaline phosphatase (ALP) and Ra-223, rather than PSA, may be good markers for assessing treatment response and are taken into consideration as part of a multimodal approach for carefully selected patients with advanced PCa (2).
To our knowledge, PCa shows a substantial clinical heterogeneity. However, the existing risk classification for PCa prognosis based on clinical factors is not sufficient (3). Currently, the increasing evidences suggest that classifying PCa patients into distinct molecular subtypes is critical in exploring the potential molecular variation underlying the biological heterogeneity. For example, Johnson et al. found that SPINK1 over expression is associated with PCa specific mortality in at risk men with biochemical and clinical recurrence after prostatectomy (4). You et al. developed a novel classification system consisting of three distinct subtypes (named PCS1-3) using pathway activation signatures of known relevance to PCa (5). Erho et al. reported that the early metastasis prediction model based on genomic expression in the primary tumor may be useful for the identification of aggressive PCa (6). Joniau et al. presented an intuitive stratification of high-risk PCa into three prognostic subgroups, and this stratification can help make decisions in the treatment for PCa patients (7). Li et al. provided the review to describe the typical clinicopathological features of the rare variants of PCa from pathology (8). These evidences support the risk stratification of PCa, which can help improve the understanding of disease and guide the treatment and prognosis of PCa patients more accurately.
In recent years, the rapid development of next-generation sequencing technology generated the multiple types of high-throughput data, which include gene expression data, copy number variation (CNV) data, somatic mutation data, DNA methylation data, microRNA expression data and so on. Integration and analysis of these data can help to discover biological heterogeneities affecting clinical outcomes in PCa (9). For example, Ross-Adams et al. provided risk stratification in PCa patients by integrating copy number and transcriptomics data, and they demonstrated the importance in identifying molecular alterations leading to the generation of robust gene sets that are predictive of clinical outcome in PCa patients (10). Yang et al. performed molecular classification of PCa by integrating somatic mutation profiles and molecular network (9).
More recently, as a systematic cancer genomic project, The Cancer Genome Atlas (TCGA) (11) generated a large number of sample-matched multi-omics data, and integration of different levels of data with the biology network context can help identify disease-related markers more effectively. Here, we performed the risk stratification of PCa and compared different subtypes from multiple molecular levels such as gene expression, DNA methylation and CNV via integration of omics data and biology network. Firstly, the omics data of PCa were downloaded from TCGA database. After applying Cox regression model, 620 risk genes were extracted and the corresponding RNA-seq expression profile of these genes were selected as the input matrix of consensus non-negative matrix factorization (CNMF) method (12) for classifying patients into two distinct molecular subtypes. Secondly, by combining with survival analysis and differential expression analysis based on two distinct molecular subtypes into protein-protein interaction (PPI) network, a PCa subtype-related network module was identified. This network module can serve as biomarkers such as SRC to predict PCa risk. Finally, the DNA methylation profiles and CNV data of genes involved in the module were analyzed. The results showed that PCa patients can be performed risk stratification, and this risk stratification can be used to promote more accurate diagnosis and more effective prognostic for PCa patients.
To our knowledge, this analysis integrates most types of molecular data which include RNA-seq expression data, survival data, DNA methylation data and CNV data in the PCa-related study. In addition, combining with survival analysis, differential expression analysis and network analysis is also the novel frame to provide an effective strategy for the integration of TCGA and can help highlight the prevention and treatment stratification for PCa patients. Some identified PCa subtype-related risk genes, such as SRC, ARR3 and RCOR2, can be used for subsequent validation based on molecular biological experiments.
Methods
Dataset
The PCa RNA-seq data was downloaded from TCGA data portal (https://tcga-data.nci.nih.gov/tcga/). For gene expression data, reads were aligned to hg19 with Tophat2, and FPKM (fragments per kilobase of transcript per million fragments mapped) values were generated and normalized (13). A total of 568 samples and 20,530 genes were included in the dataset. The CNV data and the clinical variables including over survival (OS) time, OS status, age, PSA and Gleason score were also obtained from the TCGA data portal and manually curated. After removed those samples with missing OS time or OS status, 519 samples were selected for further analysis.
Risk genes extraction based on Cox regression
To extract risk genes associated with PCa survival, the Cox proportional hazards regression was used to assess genes association with overall survival using R software (http://www.r-project.org). P values obtained from univariate Cox regression models were used to identify risk genes. Here, those genes with P<0.05 were considered as statistically significant.
Cluster analysis to obtain PCa subtypes
To acquire the potential risk stratification for PCa, we performed cluster analysis to RNA-seq expression profile of those identified risk genes obtained from Cox regression models. All of PCa patients were clustered into two subtypes based on CNMF method. Non-negative matrix factorization (NMF) is an unsupervised, parts-based learning algorithm that has been applied on the analysis of data matrices whose elements are non-negative. In the practice, NMF is an efficient method for identification of distinct molecular patterns and provides a powerful method for classification discovery (14). Here, we used CancerSubtypes (15) package of R software (http://www.bioconductor.org) to implement this analysis.
Identification of PCa subtype-related network module
The aggregated P values
For two identified PCa subtypes, we applied limma package of R software to conduct the differential expression analysis, and the corresponding P values of those risk genes were retained. Consider the input data type is RNA-seq expression profile, thus the option is RNAseq when implement the differential expression analysis. Then, for each risk gene, two P values for evaluating differently expressed and survival relevance respectively were aggregated into one P value using order statistics. Next, the aggregated P values were used to fit the beta-uniform mixture (BUM) model (16) to the distribution. In this process, an optimal mixture parameter and a shape parameter of the BUM model were obtained.
Identification of PCa subtypE-related network module
For the network data, we used a data set of literaturE-curated human PPI obtained from Human Protein Reference Database (HPRD) (17). Altogether the entire network used here comprises 9,386 nodes and 36,504 edges. We mapped the risk genes into the entire network, and the corresponding sub-network was extracted. In the extracted sub-network, self-loops were removed from the network. Then, the risk genes involved in the sub-network are scored using the fitted BUM model and a false discovery rate (FDR) cutoff of 0.01 is selected. Finally, the Heinz algorithm (18) was used to calculate the maximum-scoring network module. This process was implemented using BioNet package of R software (19).
Compare two PCa subtypes from genes involved in the identified network module based on multiple levels of molecular expressions
Sample matching of two PCa subtypes
In order to alleviate confounding effects during the process of comparing two PCa subtypes from multiple molecular levels of genes involved in the identified network module, we need to select the matched samples that are most similar in clinical variables to ensure the comparisons effectively. We thus performed the matching based on the propensity score (PS) method (20) according to the ratio of 1:1 sub-samples from two PCa subtypes. The matched clinical variables include age, Gleason score and PSA. Finally, the matched samples of two PCa subtypes were selected for further analysis.
The cutoffs contributing to the survival of genes involved in the identified network module
For those genes involved in the identified network module, we used Maximally Selected Log-Rank Statistic (21) to find their cutoffs contributing to the survival. We applied the maxstat package of R software to implement this analysis.
Compare two PCa subtypes from genes involved in the identified network module based on DNA methylation and CNV data
For those genes involved in the identified network module, we used the MethHC database (http://MethHC.mbc.nctu.edu.tw) (22) to obtain their DNA methylation levels of PCa patients in promoter regions. MethHC currently consists of 6,548 DNA methylation data generated using the Illumina HumanMethylation450K BeadChip, which includes more than 480,000 CpG sites and 12,567 mRNA/microRNA expression data calculated by RNAseq/microRNA-seq. For those genes involved in the network module, we also extracted the CNV matrix downloaded from TCGA. We analyzed DNA methylation status (high methylation vs. low methylation) and copy number alterations (CNAs) (gain vs. loss) of these genes in two different subtypes. Specially, we used caOmicsV package of R software (23) to visualize the identified network module based on multiple molecular expression levels.
The flowchart of our work was shown in Figure 1.
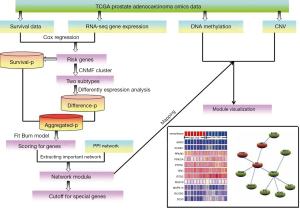
Results
Risk genes extraction based on Cox regression model
In order to extract risk genes associated with PCa survival, we performed the Univariate Cox proportional hazards regression to assess genes association with overall survival. The result showed that 620 genes were statistically significantly correlated with overall survival at the P values of less than 0.05 (online: http://tcr.amegroups.com/public/system/tcr/supp-tcr.2018.06.01-1.pdf). Among the top five genes displaying most significant association with survival, SNORA55 is reported as a novel biomarker and therapeutic target which is significantly associated with growth factor signaling and pro-inflammatory cytokine expression in PCa cell proliferation and metastatic potential (24). In addition, we found that H2AFZ is reported up-regulated at transcript level in primary PCa and high-grade prostatic intraepithelial neoplasia compared to normal prostatic tissues (25). These survival related risk genes were retained for further analysis.
Cluster analysis to identify potential PCa subtypes
To acquire the potential risk stratification for PCa patients, we performed cluster analysis to RNA-seq expression profile of 620 risk genes based on CNMF method which can distinct molecular patterns effectively. As a powerful classification, PCa patients were divided into two subtypes (clusters). Because 7 death patients were all included in the second subtype, therefore the second subtype is defined as high risk subtype which includes 302 samples. Naturally, the first subtype is defined as low risk subtype which includes 217 samples (Figure 2A). A higher silhouette score indicates the greater similarity within the samples involved in the same cluster (26), therefore the silhouette scores of 0.99 for low risk subtype and 0.98 for high risk subtype indicate the consistency within clusters of data (See Figure 2B). Figure 2C exhibited the paired comparison between two subtypes, and the results showed that there was significant difference detected between two subtypes (P<0.001). These results support the effectiveness of our risk stratification for PCa patients.
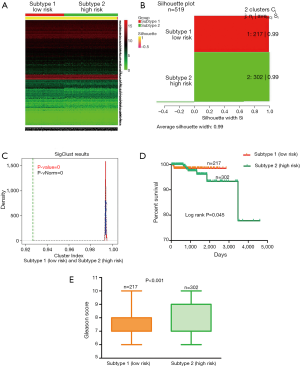
We also compared the survival curves between two subtypes using the two-sided Log-rank test. The result showed that there was significant difference in the overall survival rate between two subtypes of patients, and the low risk subtype was associated with higher survival (P=0.045, See Figure 2D). In addition, we compared the Gleason score between two subtypes based on Manny-Whitney test. The Gleason score is directly related to clinical stage, survival, progression to metastatic disease, tumor size, margin status, and pathologic stage. A high Gleason score indicates that the tumor is more likely to show aggressive behavior and therefore more likely to have spread outside of the gland to lymph nodes metastases (27). The comparison of Gleason score between two subtypes showed that the patients involved in high risk subtype have higher Gleason score than those patients involved in low risk subtype (See Figure 2E).
Identification of PCa subtype-related network module
The aggregated P values and the fitted BUM model
For two PCa subtypes, we applied limma package to conduct the differential expression analysis and obtained the corresponding P values of risk genes. Then, for each risk gene, P value for differently expressed and P value for survival relevance were aggregated into one P value using order statistics. The aggregated P values were used to fit the BUM model to the distribution. The histogram of aggregated P values was shown in Figure 3A. The quantile-quantile plot indicates that the BUM model fitted well to the aggregated P values distribution (see Figure 3B). An optimal mixture parameter of 0.1 and a shape parameter of 0.2114 in the fitted BUM model were obtained (see Figure 3C).
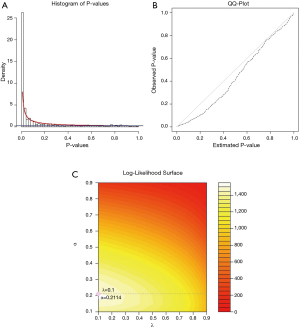
Identification of PCa-subtype related network module
We mapped the risk genes into HPRD network, and the corresponding sub-network was extracted. After self-loops were removed, 125 genes and 621 edges were kept in the network. Risk genes of the sub-network are scored using the fitted BUM model according to a FDR cutoff of 0.01. The Heinz algorithm (18) was used to calculate the maximum-scoring network module. Finally, a PCa subtype-related network module with 11 genes was identified (see Figure 4). In Figure 4, the green ellipses indicate that genes are up-regulated in high risk subtype whereas the orange ellipses indicate that genes are down-regulated in high risk subtype. From Figure 4, we can see that SRC and PRKCA are important nodes in the network module. A newly study reported that SRC can promote castration-recurrent PCa through androgen receptor-dependent canonical and non-canonical transcriptional signatures (28). In addition, previous study suggested that annatto tocotrienol effectively induces cytotoxicity in androgen-independent PCa cells via the suppression of SRC and STAT3 (29). PRKCA is a member of PKC family which serves as major receptors for some of tumor promoters and plays important roles in many different cellular processes. Meanwhile, PRKC-ζ-PrC has been found a novel biomarker of human PCa (30). Interestingly, we found that some genes linked to PRKCA are also associated with PCa risk. For example, Chiang et al. indicated that cisplatin attenuates PCa cell proliferation partly mediated by up-regulation of BTG2 through the p53-dependent pathway or p53-independent NFκB pathway (31).
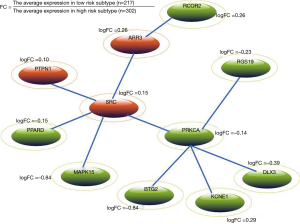
Compare multiple molecular expressions of genes involved in the network module between two PCa subtypes
The cutoffs contributing to the survival of genes involved in the network module
It is known that the differentiation between lethal and non-lethal PCa subtypes has become a very important issue in avoiding excessive treatment, thus the exploration of potential biomarkers distinguishing the high risk death patients from low risk patients can help improve the outcomes of surveillance PCa patients. Because 7 death patients were all included in the high risk subtype, we thus selected these samples to represent the especial high risk group. Then we performed the sample matching based on the PS method according to the ratio of 1:1 sub-samples from low risk subtype with the matching variables: age, Gleason score and PSA. Finally, 7 matched samples from low risk subtype were selected and compared with high risk group. For 11 genes involved in the network module, we used Maximally Selected Log-Rank Statistic (32) to find their cutoffs contributing to the survival. As a result, the survival curve comparisons showed a significant difference between the two groups according to the cutoffs of SRC and RCOR2 respectively. The cutoffs of SRC and RCOR2 are 11.671 and 2.674 respectively (Figure 5A,B), and the log rank tests comparing two groups based on > cutoff or < cutoff showed the significant difference in survival rate for SRC (P=0.043, Figure 5C) and for RCOR2 (P=0.01, Figure 5D). To our knowledge, patients with Gleason score ≤6 tumors typically have a favorable prognosis whereas patients with Gleason score 8–10 tumors often have a poor prognosis. However, patients with intermediate Gleason score 7 tumors have a more variable prognosis (33). Interestingly, 12 of 14 matched patients in this analysis all have Gleason score 7 tumors, and SRC and RCOR2 effectively stratify the clinically heterogeneous subset of these patients with intermediate Gleason score 7 tumors.
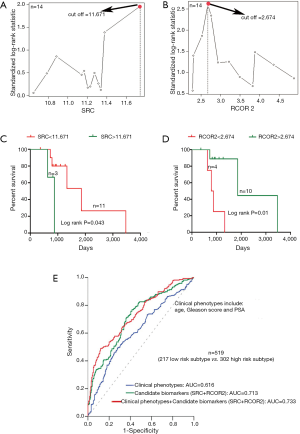
Specially, for all of PCa patients, we calculated the area under the curve (AUC) to assess the risk stratification ability of the clinical phenotypes (age, Gleason score and PSA) and the identified candidate biomarkers (SRC and RCOR2) respectively. As the predictors of logistic regression model, the clinical phenotypes alone and the candidate biomarkers alone had AUCs of 0.616 and 0.713 for performing risk stratification of PCa patients. When adding the candidate biomarkers to the clinical phenotypes in a logistic regression model, the goodness of fit of the model for performing risk stratification was improved significantly (AUC =0.733, Figure 5E).
Compare two PCa subtypes from genes involved in the identified network module based on DNA methylation and CNV data
For 11 genes involved in the network module, we used the MethHC database (22) to obtain DNA methylation levels of PCa patients in promoter regions. We compared the DNA methylation levels between two PCa subtypes, and it was observed that ARR3 displayed lower DNA methylation level in high risk subtype (Figure 6A) whereas SRC showed higher DNA methylation level in high risk subtype (Figure 6B). In previous study, Mello et al. found that alteration in the SRC DNA methylation pattern was associated with the gastric cancer onset, advanced gastric cancer, deeper tumor invasion and the presence of metastasis. Their study suggested SRC expression or DNA methylation could be useful marker for predicting tumor progression and targeting in anti-cancer strategies (34). Majid et al. elucidated that miR-23b represses proto-oncogene SRC kinase and functions as methylation-silenced tumor suppressor in PCa (35). Although it has not been proven that the increased SRC methylation is associated with high risk of PCa, our study can help highlight the importance of SRC in the risk stratification of PCa.
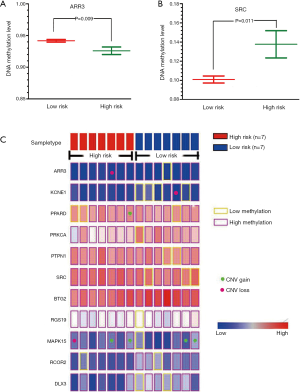
We applied caOmicsV package of R software to visualize the identified network module by integrating the omics data which include sample type, RNA-seq expression, DNA methylation status and CNAs of 11 genes (Figure 6C). Specially, we inspected the CNV data of 11 genes involved in the identified network module. Although we can not find direct evidences for these CNAs of genes are associated with PCa, some previous studies observed the potential links between these CNAs and cancers. For example, the findings of Demichelis et al. established non-coding and coding germ line CNVs as significant risk factors for PCa susceptibility and implicate their role in disease development and progression (36). From Figure 6C, we can see that MAPK15 displayed more associations with copy number gain. Recent studies observed that patients with copy number gain of MAPK15 in normal or premalignant tissues of stomach may have a chance to progress to invasive cancer (37). In addition, previous study results have suggested that constitutional CNVs may modulate subtle pathway changes through specific pathway enzymes, such as MAPK signaling pathway (38).
Another dataset to validate PCa-related risk genes
In order to validate the potential PCa-related risk genes involved in the identified network module, we selected another miRNA-mRNA dual expression profiling dataset which includes 60 PCa tissue samples and 15 normal prostate tissue samples to perform a silico analysis. The data were downloaded from GSE8126 and GSE6956 (https://www.ncbi.nlm.nih.gov/geo/). We applied general canonical correlation analysis to extract PCa-related risk genes. After complete data preprocessing (centralization, logarithmic transformation and normalization), 326 miRNAs and 13,787 mRNAs were reserved. According to P<0.05 and FDR <0.01, 117 differentially expressed miRNAs and 5,722 differentially expressed mRNAs were used to implement general canonical correlation analysis. According to the first extracted component, the PCa associated characteristic mRNAs are extracted based on the weight coefficient of mRNA in the first component. Here we extracted the top 20 mRNAs (genes) as the characteristic biomarkers of PCa. Generalized canonical correlation analysis is performed using mixOmics package of R software. We found that ARR3 (P=2.41E−5), SRC (P=9.63E−7), KCNE1 (P=6.89E−5) and BTG2 (P=0.0016) were validated as PCa-related genes which are overlapped with those genes involved in the identified network module. The results were shown in Figure S1.
Discussion
PCa is frequently occurring among men, and its mortality is continuing to rise. The latest statistics from the American Cancer Society have predicted that PCa accounts for 21 per cent of all new cancer cases in men, and for 8 per cent of all male cancer deaths in the United States (39). PCa is a clinically heterogeneous disease but its risk stratification is insufficient. The inaccurate classification causes physicians can not select a suitable treatment for patients. In the practice, it is difficult to determine the patients who may or may not benefit from immediate treatment interventions at the time of the initial diagnosis (40). In an effort to understand PCa risk stratification, we present a novel frame for integrating of PCa omics data of TCGA. In this study, PCa patients were stratified into two distinct molecular subtypes that were clinically informative. Specially, by combining with survival analysis and differential expression analysis for two distinct molecular subtypes, a PCa subtype-related network module was identified. Notably, this module can serve as biomarkers such as SRC to predict PCa risk. In this study, we applied bioinformatics tools to reduce the dimensionality and extract the essential information from genome-wide data, the work presented here thus may provide some biologically and clinically meaningful knowledge for exploring PCa subtypes.
Interestingly, we found that the Gleason scores of patients in high-risk subtype are higher than that of patients in low risk subtype, which indicates that patients in high risk subtype are more likely to show aggressive behavior. We also compared PSA between two subtypes, and no significance was found (P>0.05). Indeed, there remain disagreements on issues as whether PSA rises can determine PCa risk and whether a low PSA level can rule out PCa. Vickers et al. showed that the relationship between PSA and the risk of biopsy-detectable PCa systematically depending on the type of cohort studied by analyzing data from multiple cohorts (41). Their study poses challenges to the use of PSA to determine the risk of biopsy-detectable PCa. In the present study, we compared the DNA methylation profiles and CNV data of risk genes involved in the identified network module between the death high risk patients and the matched low risk patients. We found that some PCa subtype-related genes, such as SRC and ARR3, showing the different DNA methylation levels in high-risk group and low-risk group. Therefore, the candidate biomarkers identified in our study may contribute to the personalization of PCa treatment.
In recent years, RNA-sequencing technology is rapidly developed as a major quantitative transcriptome profiling platform. It is investigated that microarray experiments data have biases and limitations, and the continually improving RNA-seq platforms provide the comprehensive expression that will help predict therapeutic response in the substantial proportion of tumors that lack a classical targetable alteration (13,42). In the practice, researchers have proposed many methods based on the sparse theory to identify the differentially expressed genes from RNA-seq data (43). Here, the CNMF algorithm was applied to stratify the RNA-seq expression data of PCa patients into different molecular subtypes without applying any biological or clinical information. We found that 7 death patients were all included in the high risk subtype. This result indicated that the appropriate risk stratification can be used for PCa, and this stratification can help improve the treatment and prognosis of PCa patients.
Moreover, for all of genes associated with PCa survival, we applied Generally Applicable Gene-set Enrichment for Pathway Analysis (GAGE) provided by Bioconductor (44) to identify differentially expressed pathways between low risk subtype and high risk subtype. We identified Spliceosome pathway as a significant up-regulated pathway (corrected P=0.012). Indeed, a recent study found the important role of SUMOylation of spliceosome factors in PCa cells (45). Specially, the pathway enrichment analysis for genes involved in the identified network module showed that VEGF signaling pathway (P=0.02), Wnt signaling pathway (P=0.03) and apoptosis (P=0.04) are all significant enriched pathways. Some previous studies found the evidences that these pathways are important in prostate tumor progression (46,47).
Although our research on risk stratification of PCA may have some potential advantages, the limitations of our study should be pointed out. On one hand, there has been no gold standard for evaluating the performance of the molecular subtypes of PCa, therefore it is difficult to assess the accurate of this stratification (9). On the other hand, the obtained network module and the identified risk genes, such as SRC and ARR3, were only extracted by the integration analysis of omics data but not approved by molecular biology experiment. Our method depends on various tuning parameters and cutoffs of the models which can affect the risk stratification of PCa into truly molecular subtypes. Therefore, the identified candidate genes need to be validated for further lab confirmation with the help of other molecular biology laboratories. In addition, RNA-seq data have some sensitivity to bioinformatics parameters, which may affect the performance of the identification of biomarkers. Therefore, the findings of this paper must be validated prospectively, and the limitations will be addressed in our future studies.
Conclusions
In summary, we provided a novel frame to perform risk stratification for PCa patients via the integration of omics data of TCGA. A PCa subtype-related network module was identified, and this module can serve as biomarkers such as SRC to predict PCa risk. The proposed frame provides an effective strategy for the integrative analysis of TCGA and can help highlight the prevention and treatment for PCA patients.
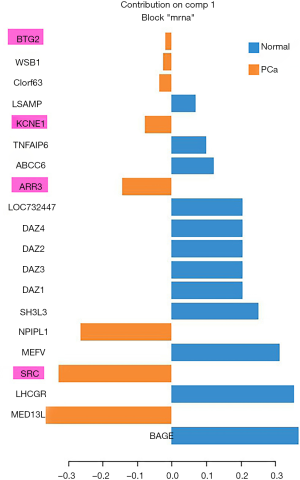
Acknowledgments
Funding: This work is supported by Beijing Natural Science Foundation (Grant No. 7142015). This study is also funded by the foundation-clinical cooperation project of capital medical university (16JL58 and 17JL54).
Footnote
Conflicts of Interest: All authors have completed the ICMJE uniform disclosure form (available at http://dx.doi.org/10.21037/tcr.2018.06.01). The authors have no conflicts of interest to declare.
Ethical Statement: The authors are accountable for all aspects of the work in ensuring that questions related to the accuracy or integrity of any part of the work are appropriately investigated and resolved. The study was conducted in accordance with the Declaration of Helsinki (as revised in 2013). Institutional ethical approval and informed consent were waived.
Open Access Statement: This is an Open Access article distributed in accordance with the Creative Commons Attribution-NonCommercial-NoDerivs 4.0 International License (CC BY-NC-ND 4.0), which permits the non-commercial replication and distribution of the article with the strict proviso that no changes or edits are made and the original work is properly cited (including links to both the formal publication through the relevant DOI and the license). See: https://creativecommons.org/licenses/by-nc-nd/4.0/.
References
- Zedan AH, Blavnsfeldt SG, Hansen TF, et al. Heterogeneity of miRNA expression in localized prostate cancer with clinicopathological correlations. PLoS One 2017;12:e0179113 [Crossref] [PubMed]
- Wenter V, Herlemann A, Fendler WP, et al. Radium-223 for primary bone metastases in patients with hormonE−sensitive prostate cancer after radical prostatectomy. Oncotarget 2017;8:44131-40. [Crossref] [PubMed]
- Lin HY, Cheng CH, Chen DT, et al. Coexpression and expression quantitative trait loci analyses of the angiogenesis genE−gene interaction network in prostate cancer. Transl Cancer Res 2016;5:S951-63. [Crossref] [PubMed]
- Johnson MH, Ross AE, Alshalalfa M, et al. SPINK1 Defines a Molecular Subtype of Prostate Cancer in Men with More Rapid Progression in an at Risk, Natural History Radical Prostatectomy Cohor. The J Urol 2016;196:1436-44. [Crossref] [PubMed]
- You S, Knudsen BS, Erho N, et al. Integrated Classification of Prostate Cancer Reveals a Novel Luminal Subtype with Poor Outcome. Cancer Res 2016;76:4948-58. [Crossref] [PubMed]
- Erho N, Crisan A, Vergara IA, et al. Discovery and Validation of a Prostate Cancer Genomic Classifier that Predicts Early Metastasis Following Radical Prostatectomy. PLoS One 2013;8:e66855 [Crossref] [PubMed]
- Joniau S, Briganti A, Gontero P, et al. Stratification of High-risk Prostate Cancer into Prognostic Categories: A European Multi-institutional Study. Eur Urol 2015;67:157-64. [Crossref] [PubMed]
- Li J, Wang Z. The pathology of unusual subtypes of prostate cancer. Chin J Cancer Res 2016;28:130-43. [PubMed]
- Yang L, Wang S, Zhou M, et al. Molecular classification of prostate adenocarcinoma by the integrated somatic mutation profiles and molecular network. Sci Rep 2017;7:738. [Crossref] [PubMed]
- Ross-Adams H, Lamb AD, Dunning MJ, et al. Integration of copy number and transcriptomics provides risk stratification in prostate cancer: A discovery and validation cohort study. EBioMedicine 2015;2:1133-44. [Crossref] [PubMed]
- Tomczak K, Czerwińska P, Wiznerowicz M. The Cancer Genome Atlas (TCGA): an immeasurable source of knowledge. Contemp Oncol (Pozn) 2015;19:A68-77. [Crossref] [PubMed]
- Brdar S, Crnojević V, Zupan B. Integrative Clustering by Nonnegative Matrix Factorization Can Reveal Coherent Functional Groups From Gene Profile Data. IEEE J Biomed Health Inform 2015;19:698-708. [Crossref] [PubMed]
- Shukla S, Evans JR, Malik R, et al. Development of a RNA-Seq Based Prognostic Signature in Lung Adenocarcinoma. JNCI J Natl Cancer Inst 2017;109(1). pii: djw200.
- Gaujoux R, Seoighe C. A flexible R package for nonnegative matrix factorization. BMC Bioinformatics 2010;11:367. [Crossref] [PubMed]
- Xu T, Le TD, Liu L, et al. CancerSubtypes: an R/Bioconductor package for molecular cancer subtype identification, validation, and visualization. Bioinformatics 2017;33:3131-3. [Crossref] [PubMed]
- Markitsis A, Lai Y. A censored beta mixture model for the estimation of the proportion of non-differentially expressed genes. Bioinformatics 2010;26:640-6. [Crossref] [PubMed]
- Prasad TSK, Kandasamy K, Pandey A. Human protein reference database and human proteinpedia as discovery tools for systems biology. Methods Mol Biol 2009;577:67-79. [Crossref] [PubMed]
- Dittrich MT, Klau GW, Rosenwald A, et al. Identifying functional modules in protein–protein interaction networks: an integrated exact approach. Bioinformatics 2008;24:i223-31. [Crossref] [PubMed]
- Beisser D, Klau GW, Dandekar T, et al. BioNet: an R-Package for the functional analysis of biological networks. Bioinformatics 2010;26:1129-30. [Crossref] [PubMed]
- Lunt M, Solomon D, Rothman K, et al. Different Methods of Balancing Covariates Leading to Different Effect Estimates in the Presence of Effect Modification. Am J Epidemiol 2009;169:909-17. [Crossref] [PubMed]
- Lausen B, Lerche R, Schumacher M. Maximally Selected Rank Statisticsfor DosE−Response Problems. Biometrical J 2002;44:131-47. [Crossref]
- Huang WY, Hsu SD, Huang HY, et al. MethHC: a database of DNA methylation and gene expression in human cancer. Nucleic Acids Res 2015;43:D856-61. [Crossref] [PubMed]
- Zhang H, Meltzer PS, Davis SR. caOmicsV: an R package for visualizing multidimensional cancer genomic data. BMC Bioinformatics 2016;17:141. [Crossref] [PubMed]
- Francesco C, Luca Q, Agnieszka M, et al. Integrated analysis of the prostate cancer small-nucleolar transcriptome reveals SNORA55 as a driver of prostate cancer progression. Mol Oncol 2016;10:693-703. [PubMed]
- Baptista T, Graça I, Sousa EJ, et al. Regulation of histone H2A.Z expression is mediated by sirtuin 1 in prostate cancer. Oncotarget 2013;4:1673-85. [Crossref] [PubMed]
- Lovmar L, Ahlford A, Jonsson M, et al. Silhouette scores for assessment of SNP genotype clusters. BMC Genomics 2005;6:35. [Crossref] [PubMed]
- Jefferies MT, Pope CS, Kynaston HG, et al. Analysis of Fascin-1 in Relation to Gleason Risk Classification and Nuclear ETS-Related Gene Status of Human Prostate Carcinomas: An Immunohistochemical Study of Clinically Annotated Tumours From the Wales Cancer Bank. Biomark Cancer 2017;9:1179299X17710944.
- Chattopadhyay I, Wang J, Qin M, et al. Src promotes castration-recurrent prostate cancer through androgen receptor-dependent canonical and non-canonical transcriptional signatures. Oncotarget 2017;8:10324-47. [Crossref] [PubMed]
- Sugahara R, Sato A, Uchida A, et al. Annatto Tocotrienol Induces a Cytotoxic Effect on Human Prostate Cancer PC3 Cells via the Simultaneous Inhibition of Src and Stat3. J Nutr Sci Vitaminol (Tokyo) 2015;61:497-501. [Crossref] [PubMed]
- Yao S, Ireland SJ, Bee A, et al. Splice variant PRKC-ζ-PrC is a novel biomarker of human prostate cancer. Br J Cancer 2012;107:388-99. [Crossref] [PubMed]
- Chiang KC, Tsui KH, Chung LC, et al. Cisplatin modulates B-cell translocation gene 2 to attenuate cell proliferation of prostate carcinoma cells in both p53-dependent and p53-independent pathways. Sci Rep 2014;4:5511. [Crossref] [PubMed]
- Wright MN, Dankowski T, Ziegler A. Unbiased split variable selection for random survival forests using maximally selected rank statistics. Stat Med 2017;36:1272-84. [Crossref] [PubMed]
- Jhun MA, Geybels MS, Wright JL, et al. Gene expression signature of Gleason score is associated with prostate cancer outcomes in a radical prostatectomy cohort. Oncotarget 2017;8:43035-47. [Crossref] [PubMed]
- Mello AA, Leal MF, Rey JA, et al. Deregulated Expression of SRC, LYN and CKB Kinases by DNA Methylation and Its Potential Role in Gastric Cancer Invasiveness and Metastasis. PLoS One 2015;10:e0140492 [Crossref] [PubMed]
- Majid S, Dar AA, Saini S, et al. MicroRNA-23b represses proto-oncogene Src kinase and functions as methylation-silenced tumor suppressor with diagnostic and prognostic significance in prostate cancer. Cancer Res 2012;72:6435-46. [Crossref] [PubMed]
- Demichelis F, Setlur SR, Banerjee S, et al. Identification of functionally active, low frequency copy number variants at 15q21.3 and 12q21.31 associated with prostate cancer risk. PNAS 2012;109:6686-91. [Crossref] [PubMed]
- Jin DH, Lee J, Kim KM, et al. Overexpression of MAPK15 in gastric cancer is associated with copy number gain and contributes to the stability of c-Jun. Oncotarget 2015;6:20190-203. [Crossref] [PubMed]
- Poptsova M, Banerjee S, Gokcumen O, et al. Impact of constitutional copy number variants on biological pathway evolution. BMC Evol Biol 2013;13:19. [Crossref] [PubMed]
- Men T, Yu C, Wang D, et al. The impact of interleukin-10 (IL-10) gene 4 polymorphisms on peripheral blood IL-10 variation and prostate cancer risk based on published studies. Oncotarget 2017;8:45994-6005. [Crossref] [PubMed]
- Kakehi Y. Active surveillance as practical lethal strategy to differentiate lethal and non-lethal prostate cancer subtypes. Asian J Androl 2012;14:361-4. [Crossref] [PubMed]
- Vickers AJ, Cronin AM, Roobol MJ, et al. The relationship between prostatE−specific antigen and prostate cancer risk: the Prostate Biopsy Collaborative Group. Clin Cancer Res 2010;16:4374-81. [Crossref] [PubMed]
- Network CGAR. Comprehensive molecular profiling of lung adenocarcinoma. Nature 2014;511:543-50. [Crossref] [PubMed]
- Liu JX, Xu Y, Gao YL, et al. A Class-Information-Based Sparse Component Analysis Method to Identify Differentially Expressed Genes on RNA-Seq Data. IEEE/ACM Trans Comput Biol Bioinform 2016;13:392-8.
- Zhang R, Strong MJ, Baddoo M, et al. Interaction of Epstein-Barr virus genes with human gastric carcinoma transcriptome. Oncotarget 2017;8:38399-412. [PubMed]
- Wen D, Xu Z, Xia L, et al. Important Role of SUMOylation of Spliceosome Factors in Prostate Cancer Cells. J Proteome Res 2014;13:3571-82. [Crossref] [PubMed]
- Pang X, Wu Y, Wu Y, et al. (−)-Gossypol, a Natural BH3 Mimetic, Suppresses the Growth of Human Prostate Cancer Xenografts via Modulating VEGF Signaling-Mediated Angiogenesis. Mol Cancer Ther 2011;10:795-805. [Crossref] [PubMed]
- Wang Y, Yang QW, Yang Q, et al. Cuprous oxide nanoparticles inhibit prostate cancer by attenuating the stemness of cancer cells via inhibition of the Wnt signaling pathway. Int J Nanomedicine 2017;12:2569-79. [Crossref] [PubMed]