Molecular imaging biomarkers for breast cancer risk and personalized screening
Background parenchymal uptake (BPU), a new imaging biomarker to predict breast cancer risk
Recently, Hruska and colleagues (1) published an interesting paper in Breast Cancer Research on a crucial topic to understanding breast cancer risk factors. In 2016, they assessed BPU with molecular breast imaging (MBI) as a risk factor through a case-control study nested in a cohort study (2); in their recent study they showed that a semi-automated system can be used for a quantitative measurement of BPU (1). The study, though conducted retrospectively, has all the strengths of purely prospective cohort studies, since the authors had the opportunity to construct a cohort of asymptomatic women screened for breast cancer with MBI and included in their case-control study all the cases occurring in the cohort. Furthermore, the cohort had a sufficiently long follow up (3.5 y average) to include both incident cases and prevalent cases missed by the screening tests. Assessment of Tc-99m sestamibi BPU was conducted retrospectively. In the study published in 2016, two radiologists, blinded to the outcome, interpreted the uptake with a subjective four-point qualitative scale, while in the present study two non-radiologist readers, blinded to the outcome, performed a quantitative semi-automated measurement: on MBI images analyzed with the corresponding digital mammograms, they defined two regions-of-interest (ROI), one of purely fat tissue and one of fibroglandular tissue. Quantitative BPU was defined as a unitless ratio of the average pixel intensity (counts/pixel) within the fibroglandular tissue versus the average pixel intensity in fat.
A semi-automated system to quantify BPU has almost the same performance as that of radiologists
Both the qualitative uptake categories and the quantitative BPU resulted associated with breast cancer (Table 1); the semi-automated measurement resulted definitely more reproducible.
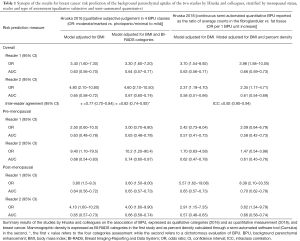
Full table
The strength of association between BPU and breast cancer risk obtained with the radiologists’ subjective interpretation and with the semi-automated system cannot be directly compared through the reported odds ratios because the two technique do not produce the same scale: radiologists classified the breasts in four categories, while the semi-automated system produces a continuous measure. Nevertheless, the area under the curve (AUC) of the model is somewhat independent from the adopted scale, thus allowing a comparison of the accuracy of the variable in predicting the outcome.
The authors did not produce any formal comparison between the two techniques, subjective vs. semi-automated, though they seem to perform quite similarly. In fact, the subjective classification by radiologists seems to be slightly better in terms of AUC, despite the fact that a continuous classification has an intrinsic advantage compared to a four-class scale.
This is not the first example of an automatic classification proving to be more reproducible than a subjective one, although it is less predictive. The example of mammographic breast density evaluation is clear: despite enormous efforts to produce software, the human eye’s ability to identify density patterns that are particularly at risk of developing cancers is still unmatched (3), and the best algorithms are probably those that empirically try to predict the visual classification instead of defining density parameters (4). Understanding why the human eye is able to capture some aspects conferring higher breast cancer risk that are not captured by the machine algorithms is beyond the scope of this contribution, but we must accept that this phenomenon often occurs.
Is reproducibility an important feature of tests predicting risk?
The rationale for seeking an automatic classification in imaging is usually based on three points: (I) increase reproducibility; (II) increase accuracy; (III) reduce human resource consumption. We implicitly consider the first two points as strictly related; we know that a non-reproducible test cannot be very accurate on average, but the opposite is not necessarily true, and we can have very reproducible tests that are systematically not accurate. In the case of a test applied on symptomatic cases for differential diagnosis, we expect to have a very high positive predictive value. Thus, the assumption that low reproducibility corresponds to low accuracy is always true; if a reader has 95% PPV and 95% sensitivity, it is unlikely for another reader to disagree with test results without increasing false positives or false negatives, i.e., reducing accuracy. On the other hand, when we apply a test to classify the general population in groups with different risks, particularly if the absolute risk is low, the ability to predict cancer, i.e., mathematically corresponding to the positive predictive value in a clinical test, will be very low. In the nested case-control study presented by Hruska and colleagues (2), we are fortunate to have an unbiased estimation of the risk in each group because we know the outcomes of the whole initial cohort of 3,000 women in which cases occurred and controls are a representative sample of this cohort for the distribution of the exposure. Let’s imagine screening the whole cohort of 3,000 women: according to the reader with the best performance, the prevalence of marked BPU in the whole cohort is about 7%, 208 women (i.e., 6.7% among the 2,938 non-cases and 17.7% among the 62 cases); thus, the probability of developing a cancer in the highest risk group (marked) is 11 out of 208. On the other hand, only 11 cases out of 62 are classified in the highest risk group. Now it is clear that it is likely for a different reader to disagree without impacting performance; the second reader can exchange any of the 219 false positives with some of the other 2,800 non-cases, the same for classification of cases with 51 false-negatives, obtaining the same specificity and sensitivity. There are many opportunities to disagree while maintaining the same accuracy of prediction (Figure 1).
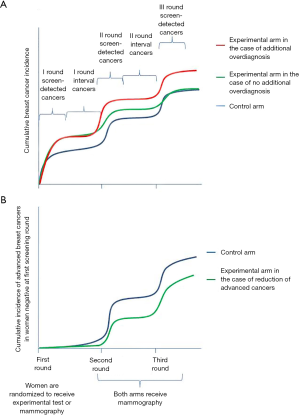
The lesson we can learn is that, dealing with tests for risk factors with the aim of stratifying the population according to the risk of disease, we usually have very low positive predictive value. Reproducibility, therefore, is not a necessary condition to reach acceptable accuracy in risk prediction. A similar phenomenon has also been observed for HPV DNA test in screening for cervical cancer precursors. This is a very sensitive test, with low positive predictive value. It is a molecular test with a clear target (the DNA of 12 HPV types), and as almost all the available commercial tests showed very good inter-laboratory reproducibility, it was thought to be highly reproducible even between different commercially available tests. Rebolj et al. (5) showed that this was not the case; different commercially available tests were highly discordant, even though the performance in terms of sensitivity and specificity was very similar. In fact, the tests were very consistent on the classification of the few true positives, but largely discordant on those classified as false positives, maintaining approximately the same total rate of positivity, i.e., similar specificity.
What could the potential use of BPU be in clinical practice and prevention?
What makes BPU an interesting risk factor is that it is a predictor of breast cancer risk regardless of breast density. This has been observed also for the background parenchymal enhancement (BPE) with magnetic resonance imaging (MRI) and contrast-enhanced spectral mammography (CESM) (6-9). Since an association between MRI BPE and MBI BPU has already been described (10), it would also be interesting to discover whether MBI BPU and MRI/CESM BPE are each predictors of cancer risk. For the moment, let’s consider them two ways of measuring the activity of the tissue. Hruska and Coll. did not find any association between MBI BPU and mammographic breast density, but their cohort included women with very homogenous breast density [the vast majority Breast Imaging Reporting and Data System (BI-RADS)]. They could, therefore, have missed the association. On the other hand, study results on the association between MRI/CESM BPE and mammographic density are controversial (11-15). Nevertheless, what is important is that BPU (like MRI and CESM BPE) can add some information to our prediction models that is not already included in what we know from density. While mammographic density, a well-established breast cancer risk factor, takes into account the amount of fibroglandular tissue, BPU and BPE introduce additional functional features of this tissue, including mitochondrial activity, cellular proliferation, blood flow, angiogenesis, and inflammation, which are in their turn linked to cancer development (16-18).
In the era of personalized screening, risk prediction models are fundamental. Research is now moving in the direction of using multiple sources of information to tailor the screening intervention to a woman’s breast cancer risk and breast characteristics: family history, metabolic and behavioral factors (body mass index, hormone replacement therapy, physical activity, diet), breast density, and genetic risk, both as high penetrance mutations (BRCA1 and 2) and SNIPs polymorphism, which have very weak association when considered individually, but with considerable predictive value when analyzed together (19,20). These factors can be used for tailoring screening for two reasons: (I) they modify the woman’s risk and therefore the balance between screening desirable and undesirable effects, without affecting mammographic screening efficacy; (II) they can alter the mammography sensitivity, thus affecting screening efficacy, thus suggesting the use of different/additional tests, i.e., ultrasound, tomosynthesis, or MRI. These new fibroglandular tissue activity biomarkers such as BPU or BPE are interesting because they can probably add something that is not yet included in the existing risk prediction models.
Unfortunately, the scientific community has not yet produced any convincing evidence regarding the efficacy of personalized screening (21,22). Properly designed studies are ongoing (23-25) but we will see results in future decades.
While the findings of Hruska and colleague contribute to understanding the mechanism underlying breast cancer risk and to future developments of risk-based screening, the practical application of BPU (or MRI and CESM BPE) are not clear.
At the moment, only mammographic screening has shown to be effective in reducing mortality. The balance between desirable and undesirable effects is definitely in favor of the desirable ones in the age range 50–69 (21,26,27), while it is less clear in the age range 40–50 (21,26). In the balance between benefits and harms, overdiagnosis plays an important role. Thus, when introducing a new screening test that adds relevant detection rate, we should demonstrate that it is not introducing additional overdiagnosis, and that, simultaneously, early diagnosis is actually reducing mortality. Many researchers and clinicians claim that it is theoretically impossible to assess overdiagnosis because it can be measured only by following the two groups for more than 30 years, and that proving the efficacy of a new screening technique would require studies that are too large with too long a follow up. If these claims are true for measuring absolute overdiagnosis, we do not need 30 year-long follow-up studies to estimate additional overdiagnosis: the additional overdiagnosis can be done with study design requiring much shorter follow up, as demonstrated by the HPV test studies (28); such study designs are now being adopted in most tomosynthesis trials (29,30). This design requires that we randomize the women to be screened with mammography or with the new experimental procedure; we maintain the two different procedures for one or two screening rounds and then we screen both groups with the standard screening test (i.e., mammography) for at least two rounds. If the cumulative overall incidence is similar at the end of the two rounds with standard test, we have demonstrated that there is no excess overdiagnosis. The scientific community has made an exception to this principle only for the introduction of more sensitive test (i.e., MRI) in the BRCA1-2 mutated women (or those with similar risk) because the lifetime probability of having a cancer is about 80%, overdiagnosis is no longer an issue. For the impact on mortality as well, we can use surrogate hard outcomes as the cumulative incidence of advanced cancers: if we are reducing the cumulative incidence of cancers in stage 2 or more severe, we can be reasonably sure that we are reducing the burden of breast cancers in terms of mortality and morbidity.
Therefore, in the situations in which the risk of having a cancer is far below 80%, before introducing a screening test that is effective in improving the detection rate, we should demonstrate, first, the reduction of cumulative incidence of advanced cancers, and second, that there is no (or acceptable) additional overdiagnosis compared to standard screening.
Furthermore, overdiagnosis is not the only negative effect of screening. Undesirable consequences also come from radiation exposure. Induced cancers are probably irrelevant with mammography screening, but MBI has a completely different source of radiation, even when minimized (31), and the balance between benefits and harms could be affected. Finally, even if MBI has been proposed as screening test for women with dense breast not justifying an MRI, the resource consumption of this approach is huge and would thus probably make such an intervention unsustainable for any public health system.
In this landscape, the application of MBI BPU (or MRI/CESM BPE) as a component of risk stratification models could be used only occasionally, because a baseline evaluation of this factor will be available only if we demonstrate the efficacy and suitability of MBI as a screening tool, at least for a group of women.
In conclusion, MBI BPU is an interesting biomarker which can add new information to the large panorama of breast cancer risk factors to be used for personalized screening. However, when the need for a tailored screening is advocated, we must remember that, when considering the balance between desirable and undesirable effects, tailoring should lead us to add a new test to a small group of women with high risk or for whom mammography is not effective or, on the other hand, to avoid additional tests for some women in whom the risk will, by definition, be below the average. To date, most of the personalized screening models are completely skewed to increase the intensity of screening in women in the high-risk group, without decreasing the intensity in those at low risk.
Acknowledgments
We thank Jacqueline Costa for the English editing.
Funding: None.
Footnote
Provenance and Peer Review: This article was commissioned by the editorial office, Translational Cancer Research. The article did not undergo external peer review.
Conflicts of Interest: All authors have completed the ICMJE uniform disclosure form (available at http://dx.doi.org/10.21037/tcr.2018.09.04). The authors have no conflicts of interest to declare.
Ethical Statement: The authors are accountable for all aspects of the work in ensuring that questions related to the accuracy or integrity of any part of the work are appropriately investigated and resolved.
Open Access Statement: This is an Open Access article distributed in accordance with the Creative Commons Attribution-NonCommercial-NoDerivs 4.0 International License (CC BY-NC-ND 4.0), which permits the non-commercial replication and distribution of the article with the strict proviso that no changes or edits are made and the original work is properly cited (including links to both the formal publication through the relevant DOI and the license). See: https://creativecommons.org/licenses/by-nc-nd/4.0/.
References
- Hruska CB, Geske JR, Swanson TN, et al. Quantitative background parenchymal uptake on molecular breast imaging and breast cancer risk: a case-control study. Breast Cancer Res 2018;20:46. [Crossref] [PubMed]
- Hruska CB, Scott CG, Conners AL, et al. Background parenchymal uptake on molecular breast imaging as a breast cancer risk factor: A case-control study. Breast Cancer Res 2016;18:42. [Crossref] [PubMed]
- Astley SM, Harkness EF, Sergeant JC, et al. A comparison of five methods of measuring mammographic density: A case-control study. Breast Cancer Res 2018;20:10. [Crossref] [PubMed]
- Wang C, Brentnall AR, Cuzick J, et al. A novel and fully automated mammographic texture analysis for risk prediction: Results from two case-control studies. Breast Cancer Res 2017;19:114. [Crossref] [PubMed]
- Rebolj M, Preisler S, Ejegod DM, et al. Disagreement between human papillomavirus assays: An unexpected challenge for the choice of an assay in primary cervical screening. PLoS One 2014;9:e86835 [Crossref] [PubMed]
- Dontchos BN, Rahbar H, Partridge SC, et al. Are Qualitative Assessments of Background Parenchymal Enhancement, Amount of Fibroglandular Tissue on MR Images, and Mammographic Density Associated with Breast Cancer Risk? Radiology 2015;276:371-80. [Crossref] [PubMed]
- Hu X, Jiang L, Li Q, et al. Quantitative assessment of background parenchymal enhancement in breast magnetic resonance images predicts the risk of breast cancer. Oncotarget 2017;8:10620-7. [PubMed]
- Telegrafo M, Rella L, Stabile Ianora AA, et al. Breast MRI background parenchymal enhancement (BPE) correlates with the risk of breast cancer. Magn Reson Imaging 2016;34:173-6. [Crossref] [PubMed]
- King V, Brooks JD, Bernstein JL, et al. Background Parenchymal Enhancement at Breast MR Imaging and Breast Cancer Risk. Radiology 2011;260:50-60. [Crossref] [PubMed]
- Yoon HJ, Kim Y, Lee JE, et al. Background 99mTc-methoxyisobutylisonitrile uptake of breast-specific gamma imaging in relation to background parenchymal enhancement in magnetic resonance imaging. Eur Radiol 2015;25:32-40. [Crossref] [PubMed]
- Hansen NL, Kuhl CK, Barabasch A, et al. Does MRI breast “density” (degree of background enhancement) correlate with mammographic breast density? J Magn Reson Imaging 2014;40:483-9. [Crossref] [PubMed]
- Savaridas SL, Taylor DB, Gunawardana D, et al. Could parenchymal enhancement on contrast-enhanced spectral mammography (CESM) represent a new breast cancer risk factor? Correlation with known radiology risk factors. Clin Radiol 2017;72:1085.e1-9. [Crossref] [PubMed]
- Sogani J, Morris EA, Kaplan JB, et al. Comparison of Background Parenchymal Enhancement at Contrast-enhanced Spectral Mammography and Breast MR Imaging. Radiology 2017;282:63-73. [Crossref] [PubMed]
- Ko ES, Lee BH, Choi HY, et al. Background enhancement in breast MR: Correlation with breast density in mammography and background echotexture in ultrasound. Eur J Radiol 2011;80:719-23. [Crossref] [PubMed]
- Cubuk R, Tasali N, Narin B, et al. Correlation between breast density in mammography and background enhancement in MR mammography. Radiol Med 2010;115:434-41. [Crossref] [PubMed]
- Del Vecchio S, Salvatore M. 99mTc-MIBI in the evaluation of breast cancer biology. Eur J Nucl Med Mol Imaging 2004;31:S88-96. [Crossref] [PubMed]
- Knopp MV, Weiss E, Sinn HP, et al. Pathophysiologic basis of contrast enhancement in breast tumors. J Magn Reson Imaging 1999;10:260-6. [Crossref] [PubMed]
- Bhatelia K, Singh K, Singh R. TLRs: Linking inflammation and breast cancer. Cell Signal 2014;26:2350-7. [Crossref] [PubMed]
- Michailidou K, Lindström S, Dennis J, et al. Association analysis identifies 65 new breast cancer risk loci. Nature 2017;551:92-4. [Crossref] [PubMed]
- van Veen EM, Brentnall AR, Byers H, et al. Use of single-nucleotide polymorphisms and mammographic density plus classic risk factors for breast cancer risk prediction. JAMA Oncol 2018;4:476-82. [Crossref] [PubMed]
- European Commission Initiative on Breast Cancer. Recommendations from European Breast Guidelines. Available online: http://ecibc.jrc.ec.europa.eu/recommendations/list/3
- Perry N, Broeders M, de Wolf C, et al. European guidelines for quality assurance in breast cancer screening and diagnosis. Annals of oncology 2008;19:614-22. [Crossref] [PubMed]
- Esserman LJ. The WISDOM Study: breaking the deadlock in the breast cancer screening debate. NPJ Breast Cancer 2017;13:3:34.
- Paci E, Mantellini P, Giorgi Rossi P, et al. Tailored Breast Screening Trial (TBST). Epidemiol Prev 2013;37:317-27. [PubMed]
- Gilbert FJ, Selamoglu A. Personalised screening: is this the way forward? Clin Radiol 2018;73:327-33. [Crossref] [PubMed]
- Oeffinger KC, Fontham ET, Etzioni R, et al. Breast Cancer Screening for Women at Average Risk. JAMA 2015;314:1599-614. [Crossref] [PubMed]
- Marmot MG, Altman DG, Cameron DA, et al. The benefits and harms of breast cancer screening: an independent review. Br J Cancer 2013;108:2205-40. [Crossref] [PubMed]
- Ronco G, Segnan N. HPV testing for primary cervical cancer screening. Lancet 2007;370:1740-2. [Crossref] [PubMed]
- Pattacini P, Nitrosi A, Rossi PG, et al. Digital Mammography versus Digital Mammography Plus Tomosynthesis for Breast Cancer Screening: The Reggio Emilia Tomosynthesis Randomized Trial. Radiology 2018;288:375-85. [Crossref] [PubMed]
- Houssami N, Lång K, Hofvind S, et al. Effectiveness of digital breast tomosynthesis (3D-mammography) in population breast cancer screening: A protocol for a collaborative individual participant data (IPD) meta-analysis. Transl Cancer Res 2017;6:869-77. [Crossref]
- Hruska CB, Weinmann AL, O’Connor MK. Proof of concept for low-dose molecular breast imaging with a dual-head CZT gamma camera. Part I. Evaluation in phantoms. Med Phys 2012;39:3466-75. [Crossref] [PubMed]