Predictive implications of genomic microarray for the diagnosis of prostate cancer metastasis following radical surgery
Introduction
Prostate cancer (PCa) is a common malignant tumor with an estimated 161,360 newly diagnosed patients in United States in 2017. It is also the third leading cause of cancer deaths among men, accounting for 8% of the total amount with an estimated 26,730 deaths (1). It is well known that a localized PCa can be treated with a radical prostatectomy (RP) or radical radiotherapy treatments, but it is estimated that approximately 20% of the PCa patients who undergo RP will experience disease recurrence, ending with an incurable metastasis which is responsible for more than 70% of the PCa deaths (2). Therefore, it is important to identify the population at a high-risk of developing a PCa recurrent metastasis after RP therapy.
Multiple genes participate in tumor metastasis; it has multiple steps and a complicated biological process (3,4). Although many genes have been widely confirmed to be associated with a metastasis of PCa, the predictive potential of using these genes is limited and uncertain (5-7). In addition, the tumor microenvironment varies significantly in every patient who receives treatment management when compared with the untreated cases. This difference may greatly affect the evaluation of the gene associations with a prognostic outcome (8-10). Thus, it is urgently necessary to use the appropriate genetic combination for predicting the metastasis risk for PCa patients after a radical surgery in order to manage the timely corresponding treatment. The Decipher (GC) test (11), which analyzed the expression of 22 genes, indicated that there was a significant association between the patient’s GC score and the chance of a clinical metastasis. However, the candidate gene selection was based on the comparison between 359 training and 186 validating cases in the microarray dataset, which may have had race or individual variations that could have caused bias.
In this study, we selected 10 candidate genes based on the microarray dataset of two single-patient derived tumor lines, which had simple characteristics except for the metastasis potential (12). PCa patients who underwent a radical surgery in the MSKCC (13) cohort were enrolled to evaluate the predictive performance of a single gene by way of the receiver operating characteristic (ROC) curve. Multivariate logistic regression analysis and the combined ROC curves were used to compare the metastatic prediction capability and efficiency between the candidate gene combinations and the single genes.
Methods
Patient-derived xenograft (PDX) model
The microarray dataset (12) of PDX models was used in our study. The LTL-313B and LTL-313H were derived from different biopsy sites of a single PCa patient, and the LTL-313H tumor lines were more likely to develop a lung metastasis, whereas the LTL-313B tumor line was not found (12). The microarray dataset of the two tumor lines was investigated and compared, and the up/down-regulated genes were screened to select the candidates. The gene expression microarray dataset is available on the GEO site: GSE41193.
The candidate gene expressions from the LTL-313B and LTL-313H datasheets underwent a log transformation to approximate normality using the Z-score, and the fold change (FC). 1/FC was used to compare differences between the gene expression with the cut-off point set at 1.5. There were 1998 up-regulated and 1738 down-regulated genes left after the comparison. An overlapping of the data for the difference in expression with the clinical PCa patient’s cohort was performed to narrow the choice down, and we discovered 33 and 21 candidates for future investigation. Based on a literature search and analysis, 10 unreported candidate genes were introduced into our study.
Patient population
The MSKCC Pca patient cohort was introduced to evaluate the association between gene expression and a metastasis prognosis (13). Localized PCa patients who underwent a radical surgery were enrolled in our study, and all cases had the necessary information for continued research. This included all the microarray data, prostate specific antigen (PSA) (diagnosis), PSA (prior to RP), Gleason score (biopsy), Gleason score (pathology), clinical T stage, pathologic T stage, type of RP, surgical margin status (SMS), extra-capsular extension (ECE), seminal vesicle invasion (SVI), lymph node involvement (LNI), biochemical recurrence (BCR), metastasis (Met), and death statistics.
Statistical analysis
The quantitative variables are presented as the mean and standard deviation (SD). An analysis of covariance (ANCOVA) was used to compare the expression between different groups with various features and prognoses in the MSKCC PCa cohort. ROC analyses were performed to evaluate the predictive potential of single genes and combinations of genes by analyzing the area under the curve (AUC) for each sample. Multivariate logistic regression was used to investigate the independent risk gene, and a Bonferroni correction was used for multiple comparisons among the 10 genes. Gene microarray data was normalized with Z-score transformation using a formula in Excel. Other statistics were analyzed using the SPSS (22.0 version) software package. Statistical significance was set at P<0.05.
Results
Based on the microarray data from the PDX model LTL-313B and LTL-313H datasets, 10 candidate genes, which included 3 up-regulated and 7 down-regulated candidates in the high-metastatic tumor line, were selected for future analysis. The expression of the candidate genes in the two tumor lines is shown in the Table 1.
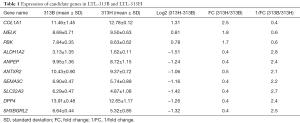
Full table
The MSKCC PCa patient cohort was used to evaluate the predictive potential of the candidates, and the clinical characteristic of all enrolled patients is shown in Table 2.
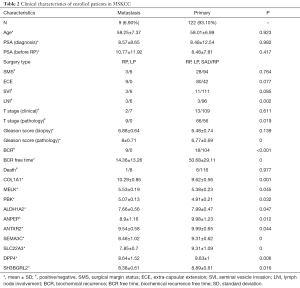
Full table
An analysis of variance (ANOVA) was performed to explore the association of clinical features and prognosis with the selected genes among the primary patient cohort who underwent radical surgery. It showed that the up/down-expressed candidate genes were significantly associated with the PCa metastasis, and some were also related to BCR, death (Table 3) as well as the PSA, Gleason score, SMS, ECE, SVI, and LNI (data not shown).
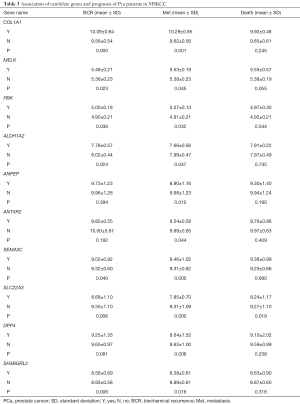
Full table
The predicative value of the single candidate gene was assessed using ROC curves. SLC22A3 had the highest AUC of 0.862, with sensitivity of 100.00% and specificity of 71.31% among the selected genes, whereas the AUC of the remaining candidates was distributed from 0.661 to 0.765 (Figure 1).
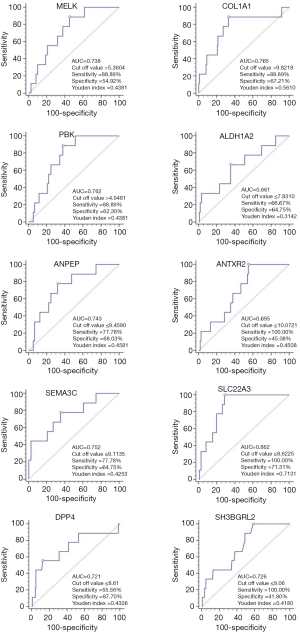
As shown in Table 4, multivariate logistic regression analysis was conducted to investigate the independent predictive genes. It was found that three candidates may associate with the metastasis of Pca (COL1A1, SEMA3C, SLC22A3,). However, a Bonferroni correction was performed as shown in Table 5, and the difference did not reach statistical significance among the three genes.
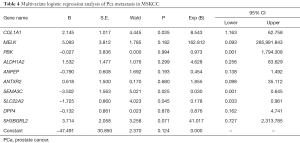
Full table
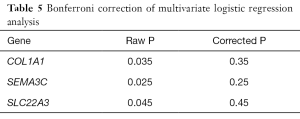
Full table
According to the combined predictive rate, the combination ROC curve was performed. The AUC of the combination group (AUC =0.972, sensitivity =100.00% and specificity =90.16%) was significantly higher than any candidate gene alone (P<0.05), which is illustrated in Figure 2 and Table 6.
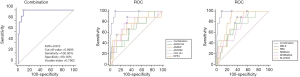
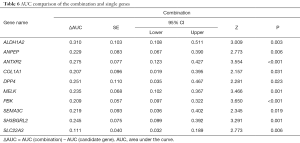
Full table
Discussion
The metastasis of PCa after RP therapy is associated with various clinical risk factors, such as tumor stage, Gleason score, SMS, LNI, as well as others (14). Due to the biological heterogeneity of PCa, it is complicated and too easily affected to use clinical factors alone as predictors for the postoperative PCa patient. It is known that all biological behavior of the tumor is accompanied by complicated genetic changes; thus, it is feasible to combine the biomarker genes to improve predictive efficiency and accuracy.
The prediction of a PCa metastasis for patients who undergo a radical cystectomy (RC) by way of a gene microarray dataset is part of precision medicine (15). The purpose for precision medicine is to identify molecular alterations in the individual cancer patients, such as the copy number alterations (CNAs), gene mutations or fusions and protein expression, to predict disease risk, and to provide optimal therapeutic options (16-18). Compared with the “one size fits all” approach, precision medicine would predict a personalized prognosis for malignant patients and tailor an individual adjuvant treatment according to their gene map landmark. Dai et al. (19) constructed an integrated diagnostic network and provided a gene panel of reasonable size to investigate the pivotal genes and the primarily hallmarks to offer novel diagnostic markers or therapeutic targets for breast cancer patients. The “Oncotype DX” system, which is based on a quantitative RT-PCR, has 21-gene signatures, and has been successfully developed to be commercially available (20). The system predicts the likelihood of a breast cancer recurrence, and helps physicians to identify the potential risk for the patient, who can obtain benefits from additional adjuvant chemotherapy before hormonal therapy. In addition, the “Oncotype DX” also has a 12-gene signature system which can be used in the application of determining the risk of a relapse for colorectal cancer patients (21). Moreover, molecular precision medicine is also used in other tumor types, such as pancreatic cancer (22), lung cancer (23,24), and hematological cancer (25).
For PCa, Hieronymus et al. (26) analyzed the genome-wide CNAs of PCa patients and found an average CNA burden of 5%. In addition, they also reported that the CNA burden was associated with BCR in adapted multivariate models. The Oncotype DX system in PCa, named the genomic prostate score (GPS) (27), assessed the RNA expression of 12 cancer-related genes (and five reference genes), which was significantly associated with disease risk. Cullen et al. (27) investigated 431 PCa patients and found the time to BCR to be closely related with the GPS in both the univariate and multivariate analyses. In addition, Cuzick et al. (28) constructed a cell-cycle progression score (also known as Prolaris) and predicted which were the high risk patients with BCR and DSS by analyzing a set of 46 genes. Obviously, it is useful and effective to apply a gene combination panel as a prognostic predictor for PCa. Several prognosis predictive systems for PCa have been applied in clinical practice, but no research regarding the relationship of CNAs and metastasis after PR has been looked into.
In our current study, 10 candidate genes were selected according to the genetically distinct LTL-313B and LTL-313H datasheets, which may reduce the bias from different individuals. The predicative values of all selected genes were distributed from 0.661 to 0.862. Based on multivariate logistic regression analysis, three genes (COL1A1, SEMA3C and SLC22A3) may associate with the metastasis of PCa, but there was no significant statistical difference based on the Bonferroni adjustment. However, The AUC of the gene combination was 0.972 with a sensitivity of 100.00% and specificity of 90.16%, which was significantly better than any candidate alone (P<0.05).
Overall, our research supports the application for the molecular prediction for PCa patients who underwent RP to aid in the selection of optimal treatment. The strength of this study was the candidate gene selection approach. The candidate genes were chosen from the paired PDX model tumor lines with different metastatic potentials, which were derived from a single PCa patient. It excluded the individual (different PCa patients) and tissue noises (primary and metastatic lesions) (29). However, there were several limitations in our study. First, retrospective research has inherent limitations. Second, the total and metastatic populations were relatively small. Finally, our observations need to be validated in a Chinese PCa patient cohort. Thus, future multi-center and large population research needs to be conducted to confirm our conclusions.
Conclusions
In summary, our findings suggest that the genomic microarray tests could assist in the determination of metastasis in high-risk PCa patients following a radical operation, and could help urologists to determine which optimal treatment approaches would benefit the prognosis of the PCa patient.
Acknowledgments
Funding: This study was supported by the National Natural Science Foundation of China (NSFC) (No. 81702534) and the Science and Technology Fund of Tianjin Provincial Commission of Health and Family Planning (No. 16KG156).
Footnote
Conflicts of Interest: All authors have completed the ICMJE uniform disclosure form (available at http://dx.doi.org/10.21037/tcr.2018.11.16). The authors have no conflicts of interest to declare.
Ethical Statement: The authors are accountable for all aspects of the work in ensuring that questions related to the accuracy or integrity of any part of the work are appropriately investigated and resolved. The study was conducted in accordance with the Declaration of Helsinki (as revised in 2013). The study was approved by the Institute Research Medical Ethics Committee of the Tianjin medical union center (No. 2018-B02). Informed consent was not required according to our research in the study.
Open Access Statement: This is an Open Access article distributed in accordance with the Creative Commons Attribution-NonCommercial-NoDerivs 4.0 International License (CC BY-NC-ND 4.0), which permits the non-commercial replication and distribution of the article with the strict proviso that no changes or edits are made and the original work is properly cited (including links to both the formal publication through the relevant DOI and the license). See: https://creativecommons.org/licenses/by-nc-nd/4.0/.
References
- Siegel RL, Miller KD, Jemal A. Cancer Statistics, 2017. CA Cancer J Clin 2017;67:7-30. [Crossref] [PubMed]
- Batty M, Pugh R, Rathinam I, et al. The Role of alpha1-Adrenoceptor Antagonists in the Treatment of Prostate and Other Cancers. Int J Mol Sci 2016;17: [Crossref] [PubMed]
- Maishi N, Hida K. Tumor endothelial cells accelerate tumor metastasis. Cancer Sci 2017;108:1921-6. [Crossref] [PubMed]
- Erdogan B, Webb DJ. Cancer-associated fibroblasts modulate growth factor signaling and extracellular matrix remodeling to regulate tumor metastasis. Biochem Soc Trans 2017;45:229-36. [Crossref] [PubMed]
- Fraser M, Sabelnykova VY, Yamaguchi TN, et al. Genomic hallmarks of localized, non-indolent prostate cancer. Nature 2017;541:359-64. [Crossref] [PubMed]
- Petrylak DP, Crawford ED. Biomarkers for the Management of Castration-Resistant Prostate Cancer: We Are Not There Yet. Target Oncol 2017;12:401-12. [Crossref] [PubMed]
- Lamy PJ, Allory Y, Gauchez AS, et al. Prognostic Biomarkers Used for Localised Prostate Cancer Management: A Systematic Review. Eur Urol Focus 2017; [Epub ahead of print]. [Crossref] [PubMed]
- Shiao SL, Chu GC, Chung LW. Regulation of prostate cancer progression by the tumor microenvironment. Cancer Lett 2016;380:340-8. [Crossref] [PubMed]
- Fong L, Carroll P, Weinberg V, et al. Activated lymphocyte recruitment into the tumor microenvironment following preoperative sipuleucel-T for localized prostate cancer. J Natl Cancer Inst 2014;106: [Crossref] [PubMed]
- Casey SC, Amedei A, Aquilano K, et al. Cancer prevention and therapy through the modulation of the tumor microenvironment. Semin Cancer Biol 2015;35:S199-223. [Crossref] [PubMed]
- Erho N, Crisan A, Vergara IA, et al. Discovery and validation of a prostate cancer genomic classifier that predicts early metastasis following radical prostatectomy. PLoS One 2013;8:e66855 [Crossref] [PubMed]
- Lin D, Wyatt AW, Xue H, et al. High fidelity patient-derived xenografts for accelerating prostate cancer discovery and drug development. Cancer Res 2014;74:1272-83. [Crossref] [PubMed]
- Taylor BS, Schultz N, Hieronymus H, et al. Integrative genomic profiling of human prostate cancer. Cancer Cell 2010;18:11-22. [Crossref] [PubMed]
- Yamada Y, Naruse K, Nakamura K, et al. Investigation of risk factors for prostate cancer patients with bone metastasis based on clinical data. Exp Ther Med 2010;1:635-9. [Crossref] [PubMed]
- Smits M, Mehra N, Sedelaar M, et al. Molecular biomarkers to guide precision medicine in localized prostate cancer. Expert Rev Mol Diagn 2017;17:791-804. [Crossref] [PubMed]
- Streeter OE Jr, Beron PJ, Iyer PN. Precision Medicine: Genomic Profiles to Individualize Therapy. Otolaryngol Clin North Am 2017;50:765-73. [Crossref] [PubMed]
- Ceci F, Fiorentino M, Castellucci P, et al. Molecular Imaging and Precision Medicine in Prostate Cancer. PET Clin 2017;12:83-92. [Crossref] [PubMed]
- Galazi M, Rodriguez-Vida A, Ng T, et al. Precision medicine for prostate cancer. Expert Rev Anticancer Ther 2014;14:1305-15. [Crossref] [PubMed]
- Dai X, Hua T, Hong T. Integrated diagnostic network construction reveals a 4-gene panel and 5 cancer hallmarks driving breast cancer heterogeneity. Sci Rep 2017;7:6827. [Crossref] [PubMed]
- Roberts MC, Miller DP, Shak S, et al. Breast cancer-specific survival in patients with lymph node-positive hormone receptor-positive invasive breast cancer and Oncotype DX Recurrence Score results in the SEER database. Breast Cancer Res Treat 2017;163:303-10. [Crossref] [PubMed]
- Reimers MS, Zeestraten EC, Kuppen PJ, et al. Biomarkers in precision therapy in colorectal cancer. Gastroenterol Rep (Oxf) 2013;1:166-83. [Crossref] [PubMed]
- Pishvaian MJ, Brody JR. Therapeutic Implications of Molecular Subtyping for Pancreatic Cancer. Oncology (Williston Park) 2017;31:159-66, 68.
- Garofalo A, Sholl L, Reardon B, et al. The impact of tumor profiling approaches and genomic data strategies for cancer precision medicine. Genome Med 2016;8:79. [Crossref] [PubMed]
- Vargas AJ, Harris CC. Biomarker development in the precision medicine era: lung cancer as a case study. Nat Rev Cancer 2016;16:525-37. [Crossref] [PubMed]
- Sun R, Medeiros LJ, Young KH. Diagnostic and predictive biomarkers for lymphoma diagnosis and treatment in the era of precision medicine. Mod Pathol 2016;29:1118-42. [Crossref] [PubMed]
- Hieronymus H, Schultz N, Gopalan A, et al. Copy number alteration burden predicts prostate cancer relapse. Proc Natl Acad Sci U S A 2014;111:11139-44. [Crossref] [PubMed]
- Cullen J, Rosner IL, Brand TC, et al. A Biopsy-based 17-gene Genomic Prostate Score Predicts Recurrence After Radical Prostatectomy and Adverse Surgical Pathology in a Racially Diverse Population of Men with Clinically Low- and Intermediate-risk Prostate Cancer. Eur Urol 2015;68:123-31. [Crossref] [PubMed]
- Cuzick J, Swanson GP, Fisher G, et al. Prognostic value of an RNA expression signature derived from cell cycle proliferation genes in patients with prostate cancer: a retrospective study. Lancet Oncol 2011;12:245-55. [Crossref] [PubMed]
- Wang Y, Wang JX, Xue H, et al. Subrenal capsule grafting technology in human cancer modeling and translational cancer research. Differentiation 2016;91:15-9. [Crossref] [PubMed]