Survival-based bioinformatics analysis to identify hub genes and key pathways in non-small cell lung cancer
Introduction
Lung cancer is the most prevalent cancer and the leading cause of cancer-associated death (1). Recent global cancer statistics report that the number of new lung cancer cases was 2,093,876 (11.6% of the total cases), and the number of deaths was 1,761,007 (18.4% of the total cancer deaths) in 2018 (2).
Lung cancer can be classified into small cell lung cancer (SCLC, 15% of cases) and NSCLC (85% of cases) based on histopathologic diagnosis (3-5). For non-small cell lung cancer (NSCLC), the 5-year survival rates are about 4–17% mainly depending on stage (3-5). Mechanisms underlying NSCLC have been intensively investigated in the past decades. Abnormal expression or mutation of genes such as epidermal growth factor receptor (EGFR) and tumor suppressor protein 53 (TP53) play essential roles in carcinogenesis, progression, and metastasis of NSCLC (3-6). However, the molecular interaction mechanisms and dysregulated pathways remain unclear.
High-throughput technologies such as microarray or RNA-seq allow researchers to detect the gene expression profiles at a global level (7-10). Based on this technology, new hub genes that play essential roles in the pathology of NSCLC have been identified (11,12). However, all of these pieces of research were based on a single dataset analysis, which might have led to biased results. Here, we have screened the hub genes based on multiple-microarrays and overall survival, and, in doing so, have made new discoveries.
Methods
Gene expression dataset
Four NSCLC mRNA microarray datasets (GSE18842, GSE19188, GSE27262, and GSE33532) were downloaded from the National Center of Biotechnology Information Gene Expression Omnibus database (http://www.ncbi.nlm.nih.gov/geo/), which is the most significant resource for high throughput data submitted by scientists under strict standards. These datasets were based on the GPL570 platform (Affymetrix Human Genome U133 Plus 2.0 Array). Each dataset contained tumor samples and adjacent healthy tissue samples and were each validated by their submitter. The datasets and their accompanying sample counts are as follows: 46 tumor samples and 45 normal samples for GSE18842, 91 tumor samples and 65 normal samples for GSE19188, 25 tumor samples and 25 normal samples for GSE27262, and 80 tumor samples and 20 normal samples for GSE33532.
Screening differentially expressed genes (DEGs)
The R software (version 3.4.0) and Bioconductor packages were used to analyze the microarray datasets (13). The process for analysis was as follows: we imported CEL files using the affy package, assessed the microarray data quality using the simpleaffy package, preprocessed the raw data into expression metrics using the robust multi-array average (RMA) algorithm in the gcrma package, and used the genefilter package to filter the probe with low sensitivity before comparison (14-16); the t-test then analyzed the DEGs between the tumor and normal group samples based on the limma package (17); multiple testing was corrected by the Benjamini-Hochberg method to obtain the adjusted P-value; finally, the genes with adjusted P<0.001 and |log2fold-change (FC)|>1 were selected as the candidate DEGs. To further enhance the reliability of the DEG analysis, the overlapping DEGs co-existing in all four datasets were identified using FunRich software (version 3.1.3) (18).
Screening overlapping DEGs correlated with overall survival in the Kaplan-Meier plotter database
An online global survival database Kaplan-Meier plotter (http://kmplot.com/analysis/), which contains both the survival data and gene expression data of breast, gastric, ovarian and lung cancers, was used to evaluate the correlation between the prognostic significance and each DEGs of NSCLC (19). The patient samples were classified into high and low expression groups by the median expression value of a gene. In our study, we used the default parameters, which in brief, presented no subtypes restrictions in the “univariate” for Cox regression and the “exclude biased arrays” for array quality control. For each gene, the log-rank P value and median survival were calculated. The gene with log-rank P value <0.001 were considered to be statistically significant.
Function enrichment analysis of DEGs correlated with overall survival
Both Gene Ontology (GO) enrichment analysis and Kyoto Encyclopedia of Genes and Genomes (KEGG) pathway enrichment analysis are widely used to identify functions and pathways for a large number of genes. Here, we used a professional, comprehensive R package clusterProfiler to investigate enriched GO biological processes and KEGG pathways of the overlapping DEGs correlated with overall survival. The cut-off criterion was set at Benjamini-Hochberg adjusted P<0.05 and q<0.05 (20).
PPI network construction and hub gene analysis
The protein-protein interactions played an essential role in regulating biological processes. These kinds of relationship can be presented by a PPI network in which vertices represent proteins, and edges represent the interaction between proteins. The densely connected regions may serve the enriched function clusters. The proteins are shown to have a high connection (interaction) with several other proteins suggesting a central regulatory role, likely to be regulatory “hubs” (21).
To gain the protein-protein interaction information, the DEGs correlated with overall survival were imported into the STRING database to construct a PPI network. The confidence score cutoff was set as 0.9, and only the interaction information validated by experiment was adopted to increase the confidence. Then, the interaction information was imported into the Cytoscape software (version 3.6.1) (22). MCODE was used to explore densely connected regions in large protein-protein interaction networks and the scores >4 and nodes >5 were set as the cutoff criteria (23). ClusterProfiler package was used to analyze the functions of the clusters. CytoHubba was used to reveal the hub nodes in the regulation network (24). The overlapping genes in the top 10 calculated by degree and maximal clique centrality (MCC) were selected as hub genes. Then, we identified their expression level in their original datasets.
Validation of hub genes in the Cancer Genome Atlas (TCGA) and Human Protein Atlas (HPA) database
The mRNA level and overall survival of hub genes were validated by TCGA data. The RNA-seq data was downloaded and analyzed by TCGAbiolinks package in R (25,26). The overall survival analysis of TCGA data was performed using the GEPIA online tool (27). The protein level in healthy tissue and lung cancer tissue was validated using the immunohistochemical data of the HPA database (28).
Results
Screening DEGs correlated with overall survival in a K-M plotter database
To find DEGs of NSCLC, we selected four mRNA microarrays from the GEO database for further analysis. |logFC| >1 and adjust P value <0.001 were set as a strict cutoff to avoid false positive results. After analysis, we obtained 4,216 DEGs (1,873 up-regulated and 2,253 down-regulated) in GSE18842, 3,114 DEGs (1,134 up-regulated and 1,980 down-regulated) in GSE19188, 3,035 DEGs (1,334 up-regulated and 1,701 down-regulated) in GSE27262, and 3,689 DEGs (1,565 up-regulated and 2,124 down-regulated) in GSE33532. As shown in the gene expression heatmaps (Figure 1A,B,C,D), in each dataset these DEGs showed distinct expression patterns between normal samples and tumor samples. To obtain robust DEGs in NSCLC, we performed Venn diagrams (Figure 1E,F) of up-regulated DEGs and down-regulated DEGs between each of the four datasets, and found 522 up-regulated DEGs and 989 down-regulated DEGs. These genes were considered as the DEGs of NSCLC for further analysis.
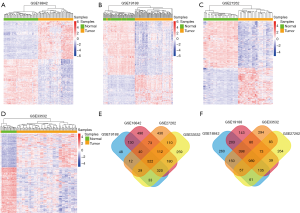
To find out how these DEGs were correlated with overall survival, we put these DEGs into the K-M plotter database to analyze the median survival and log-rank p-value between the low expression cohort and the high expression cohort. DEGs with (log rank P) <0.001 were considered as DEGs correlated with overall survival (DEGOSs). After screening, we obtained 895 DEGOSs, among which 265 genes were up-regulated and 630 genes were down-regulated (Table 1 and online: http://fp.amegroups.cn/cms/tcr.2019.06.35-1.pdf). Importantly, high expression of up-regulated genes was significantly correlated with poor overall survival while the low expression of down-regulated genes was correlated with poor overall survival of patients (Table 1 and online: http://fp.amegroups.cn/cms/tcr.2019.06.35-1.pdf).
Table 1
Gene symbol | Median survival (month) | Log rank P | Hazard ratio | |
---|---|---|---|---|
Low expression cohort | High expression cohort | |||
Upregulated genes | ||||
ASPM | 96 | 43.83 | <1E-16 | 1.76 (1.55–2.01) |
CDC20 | 96 | 42.8 | <1E-16 | 1.82 (1.6–2.07) |
TPX2 | 96.2 | 42 | <1E-16 | 1.87 (1.64–2.12) |
KIF2C | 96.1 | 44 | <1E-16 | 1.78 (1.57–2.03) |
DLGAP5 | 94.5 | 43.83 | <1E-16 | 1.73 (1.52–1.97) |
Downregulated genes | ||||
CPED1 | 45.47 | 125 | <1E-16 | 0.66 (0.56–0.77) |
SYNE1 | 44 | 95.5 | <1E-16 | 0.68 (0.6–0.75) |
CBX7 | 42.23 | 91 | <1E-16 | 0.53 (0.47–0.59) |
PTEN | 48 | 124 | <1E-16 | 0.96 (0.82–1.12) |
PPM1K | 46 | 134 | <1E-16 | 0.65 (0.56–0.77) |
Function analysis of DEGOSs
To get insight into the function of DEGOSs, we performed GO enrichment analysis; the top 10 biological processes with statistical significance were shown in Figure 2A,B. The dysregulated genes of each pathway were listed in the Table S1. The upregulated genes enriched in the biological processes that associated with cell division, organelle division and chromosome division. The downregulated genes were enriched in the biological processes associated with angiogenesis and negative regulation of cell migration. The angiogenesis switch was governed by the countervailing factors that either induce or oppose angiogenesis (29,30). In this study, we found most angiogenesis inhibitors (MMRN2, RECK, ANGPT1, PECAM1, ROBO4 and S1PR1) and HIF 1α inhibitor (KLF2) were downregulated and enriched in the angiogenesis biological process (31-36). This indicated the angiogenesis inhibited switch was downregulated in the NSCLC.
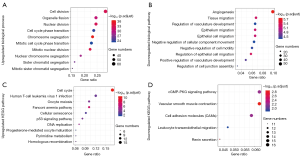
For a further exploration of the dysregulated pathways influenced by the DEGOSs, we performed the KEGG pathway enrichment (Figure 2C,D and Table S2). The upregulated genes were enriched in the KEGG pathways associated with DNA replication and cell cycle, while the downregulated genes were enriched in the KEGG pathways associated with cell adhesion and cell migration. This was consistent with the GO enrichment analysis.
PPI network construction and cluster analysis
The PPI network provided a convenient method to visualize the protein-protein interactions and find the potential molecular mechanism. The PPI network was composed of DEGOSs in which we found 7 function clusters ranked by scores. We performed a GO analysis of each cluster to define its function (Figure 3 and Table S3). Cluster 1 and cluster 4 took part in the cell division mainly by chromosome segregation and organelle fusion. Cluster 2 seemed to have a function in blood coagulation. Cluster 3 and cluster 7 were functionally enriched in the G-protein coupled receptor signaling pathway. Cluster 4 played a role in the G2/M transition in the cell cycle. Cluster 5 had the only protein poly-ubiquitination with statistical significance. These clusters provided an overview of the potential molecular mechanisms in NSCLC by bioinformatics and may help us to understand the pathogenesis of NSCLC.
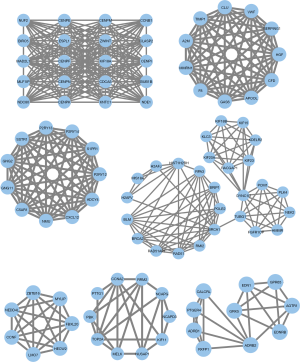
Hub gene identification
Hub genes are highly interconnected genes and play central roles in the PPI network. They have the potential to be biomarkers and therapeutic targets. To obtain a robust result, we calculated the degree and MCC scores for each node. The top 10 in both algorithms were selected as hub genes and used to construct PPI networks to visualize the interaction. We identified six hub genes which were BUB1B, CCNB1, CENPE, KIF18A, MAD2L1, and NDC80 (Figure 4). All these genes had a function associated with chromosome and spindle behavior of mitotic cell division and showed an up-regulated expression level in NSCLC tissue (Table S4) (37-39).
Validation of hub genes in TCGA and HPA
To validate whether the hub genes identified above were of universal significance, we analyzed their mRNA expression level and overall survival in TCGA lung cancer RNA-seq data and protein level in HPA immunohistochemical data. We found that all these hub genes were abnormally up-regulated expressed (Figure 5A,B,C,D,E,F), and the protein level was up-regulated consistently with mRNA level, as shown in Figure 5G. They were also correlated with overall survival (Figure 6). This evidence gives support to the universal significance of our results.
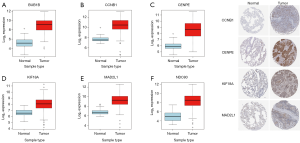
Discussion
In our study, we explored the hub genes and potential mechanisms based on the DEGs correlated with overall survival in NSCLC for the first time. We found that cell division, cell cycle, cell migration, DNA replication, and angiogenesis might be the major dysregulated functions in NSCLC. P53 signaling pathway and cGMP-PKG signaling pathway might be the major dysregulated signaling pathways. Furthermore, there were six genes associated with cell division that were identified as hub genes. Finally, we performed an independent validation of hub genes in TCGA and HPA and found that these hub genes did show abnormal expression patterns in NSCLC and correlated with overall survival of NSCLC patients.
In our function analysis, we found the upregulated genes enriched in the cell division, cell cycle and chromosome segregation process. Spindle assembly checkpoint played important roles in these processes. And the hub genes were the key components of the spindle assembly checkpoint. The downregulated genes enriched in the negative regulation of cell migration and angiogenesis. In the cell migration process, cell adhesion molecules like JAM2 and PECAM1 were downregulated to break the cell junction and increased cell motility (33,40). In the angiogenesis process, key inhibitors of several angiogenic switch were downregulated. ANGPT1/TEK and ROBO4/SLIT2 were both the receptor/ligand system to promote the vascular stability in the physiological condition (32,35,36,41-43). Many clinical studies showed that ANGPT1 and TEK expression level was downregulated in the NSCLC tissue and loss of ANGPT1/TEK system increased metastasis and angiogenesis in the mice model (32,44,45). But the mechanism was still to be clarified. ROBO4/SLIT2 system suppressed the tumor growth and metastasis through the tumor angiogenesis in breast cancer (41). SLIT2 could suppress cell migration by the β-catenin and the AKT-GSK3β signaling pathway in colorectal cancer (42). The downregulation of SLIT2 expression was also observed in the clinical lung cancer patient cases (44,45). In vitro experiment showed that SLIT2 could promote the NSCLC cells metastasis to the brain by downregulating the CDH2. The KEGG pathway enrichment results were consistent with GO analysis. The NSCLC tissue showed upregulated pathways associated with cell division and DNA replication and downregulated pathways involved of cell adhesion and negative regulation of cell migration. The KEGG analysis also provided a new clue that renin secretion pathway might have some special roles in the NSCLC progress. Many reports showed ACE protein and mRNA level was decreased and associated with the angiogenesis and tumor growth in the NSCLC patients (46,47). But the molecular mechanism had not been reported.
In the six hub genes, CENPE and KIF18A were identified for the first time. Both of these are kinesins superfamily members that take part in the mitotic spindle (48,49). The mitotic spindle is a popular drug target validated in cancer chemotherapy. Taxanes and vinca alkaloids, which target the tubulin in mitotic spindle, have been used in successful clinical application (49). Centromeric protein E (CENPE) is a kinesin-like motor protein that establishes and maintains the connection between spindle microtubules and chromosomes and shifts the chromosomes to the metaphase plate powered by ATPase activity. It is required for the transformation from the metaphase to the anaphase. Aurora B and protein phosphate 1 control the phosphorylation and dephosphorylation of CENPE to modulate its operation, which is essential for chromosome congression. BUB1B also controls its action at the kinetics as a mitotic checkpoint event (49,50).
The protein level of CENPE should kept in balance to maintain cell division. Up-regulation of CENPE has been reported with a worse overall survival in breast cancer (51), but down-regulation of CENPE has been found in liver cancer (50). However, reports about CENPE in NSCLC are rare. Due to the importance of CENPE, it is considered a drug target in cancer therapy. There are three inhibitors of CENPE that showed good effects (50). GSK923295 is an allosteric inhibitor of CENPE kinesin motor ATPase activity that induced tumor cell apoptosis and tumor regression. In the phase I clinical study which involved 39 refractory cancer patients (including 4 NSCLC patients), GSK923295 exhibited dose-proportional pharmacokinetics with a low incidence of myelosuppression and neuropathy. PF-2271 is a highly specific CENPE motor activity which shows no inhibition of protein kinase and ATPase activity of highly related kinesins. It inhibits basal-like breast cancer cell survival without inhibition to normal breast epithelial cells. Despite this, there is no report about the effect of PF-2271 in NSCLC. Compound A is a CENPE ATPase inhibitor showed a time-dependent anti-proliferative activity. It has been proved to inhibit the proliferation of multiple lung cancer cell lines. KIF18A takes part in the chromosome congression by reducing the amplitude of preanaphase oscillations and slowing poleward movement during anaphase. It has been reported that KIF18A is overexpressed in colorectal cancer and its overexpression is also correlated with the grade, metastasis and worse survival in breast cancer (52-54). There still is no report about KIF18A. BTB-1 has been the only inhibitor of KIF18A known until now. However, it has shown a high specificity and inhibition effect to KIF18A (50). Based on the clues above and the high correlation with NSCLC survival, we think CENPE and KIF18A are worthy of further study and may serve as therapeutic targets in NSCLC.
In summary, we identified some new pathways and two new hub genes in NSCLC based on the DEGs correlated with overall survival. The two hub genes, CENPE and KIF18A, are associated with solid tumors, and the inhibitor of CENPE in the phase I clinical study. Hub gene screening based on survival might be more clinically significant, and our method might be more powerful. However, hub gene function in NSCLC still needs further investigation.
Table S1
ID | Description | P adjust | Gene ID |
---|---|---|---|
Upregulated | |||
GO: 0007059 | Chromosome segregation | 7.38E-37 | NEK2/NUSAP1/BUB1B/CENPE/SPAG5/CCNE1/PTTG1/KIF18B/TOP2A/BIRC5/MKI67/CENPN/BRIP1/KIF14/NCAPG/KIF18A/RACGAP1/CENPF/ESPL1/MAD2L1/MIS18A/ZWINT/BLM/NDC80/RCC1/CCNB1/NDE1/TUBG1/NCAPD3/KIF23/BRCA1/ECT2/FEN1/CCNE2/CDCA8/CDC20/KIF2C/DLGAP5/BUB1/KIF4A/HJURP/TTK/PRC1/AURKB/TRIP13/CDT1/NCAPH/CDC6/SPC25/OIP5 |
GO: 0140014 | Mitotic nuclear division | 6.04E-34 | NEK2/NUSAP1/BUB1B/CENPE/SPAG5/CCNE1/PTTG1/KIF18B/MKI67/KIF14/NCAPG/KIF18A/CCNA2/RACGAP1/CENPF/ESPL1/MAD2L1/KIF11/AURKA/ZWINT/NDC80/RCC1/CCNB1/NDE1/CCNF/TUBG1/NCAPD3/KNTC1/KIF23/CCNE2/CDCA8/CDC20/TPX2/KIF2C/DLGAP5/BUB1/KIF4A/UBE2C/TTK/CCNB2/CHEK1/PRC1/AURKB/MYBL2/TRIP13/CDT1/NCAPH/CDC6 |
GO: 0051301 | Cell division | 2.92E-33 | ASPM/NEK2/NUSAP1/BUB1B/CENPE/SPAG5/KIF20A/CCNE1/PTTG1/KIF18B/TOP2A/BIRC5/NCAPG2/BRIP1/KIF14/NCAPG/CCNA2/RACGAP1/CENPF/ESPL1/MAD2L1/MIS18A/HELLS/KIF11/AURKA/ZWINT/BRCA2/BLM/NDC80/MDK/RCC1/CCNB1/NDE1/CKS2/CCNF/NCAPD3/KNTC1/E2F8/KIF23/PIMREG/ECT2/CKAP2/TIMELESS/CCNE2/CDCA8/CDC20/TPX2/KIF2C/BUB1/CEP55/KIF4A/UBE2C/CCNB2/PRC1/AURKB/CDCA3/CDT1/NCAPH/CDC6/SPC25/OIP5 |
GO: 0000280 | Nuclear division | 3.08E-33 | ASPM/NEK2/NUSAP1/BUB1B/CENPE/SPAG5/CCNE1/PTTG1/KIF18B/TOP2A/MKI67/BRIP1/KIF14/NCAPG/KIF18A/CCNA2/RACGAP1/CENPF/ESPL1/MAD2L1/KIF11/AURKA/ZWINT/BLM/NDC80/RCC1/CCNB1/NDE1/CKS2/CCNF/TUBG1/NCAPD3/KNTC1/KIF23/CCNE2/CDCA8/CDC20/TPX2/KIF2C/DLGAP5/BUB1/KIF4A/UBE2C/TTK/CCNB2/CHEK1/PRC1/AURKB/MYBL2/TRIP13/CDT1/NCAPH/CDC6 |
GO: 0048285 | Organelle fission | 3.48E-31 | ASPM/NEK2/NUSAP1/BUB1B/CENPE/SPAG5/CCNE1/PTTG1/KIF18B/TOP2A/MKI67/BRIP1/KIF14/NCAPG/KIF18A/CCNA2/RACGAP1/CENPF/ESPL1/MAD2L1/KIF11/AURKA/ZWINT/BLM/NDC80/RCC1/CCNB1/NDE1/CKS2/CCNF/TUBG1/NCAPD3/KNTC1/KIF23/CCNE2/CDCA8/CDC20/TPX2/KIF2C/DLGAP5/BUB1/KIF4A/UBE2C/TTK/CCNB2/CHEK1/PRC1/AURKB/MYBL2/TRIP13/CDT1/NCAPH/CDC6 |
GO: 0098813 | Nuclear chromosome segregation | 3.33E-29 | NEK2/NUSAP1/BUB1B/CENPE/SPAG5/CCNE1/PTTG1/KIF18B/TOP2A/BRIP1/KIF14/NCAPG/KIF18A/RACGAP1/CENPF/ESPL1/MAD2L1/ZWINT/BLM/NDC80/CCNB1/TUBG1/NCAPD3/KIF23/ECT2/FEN1/CCNE2/CDCA8/CDC20/KIF2C/DLGAP5/BUB1/KIF4A/TTK/PRC1/AURKB/TRIP13/CDT1/NCAPH/CDC6 |
GO: 0000819 | Sister chromatid segregation | 3.73E-27 | NEK2/NUSAP1/BUB1B/CENPE/SPAG5/PTTG1/KIF18B/TOP2A/KIF14/NCAPG/KIF18A/RACGAP1/CENPF/ESPL1/MAD2L1/ZWINT/NDC80/CCNB1/TUBG1/NCAPD3/KIF23/FEN1/CDCA8/CDC20/KIF2C/DLGAP5/BUB1/KIF4A/TTK/PRC1/AURKB/TRIP13/CDT1/NCAPH/CDC6 |
GO: 0000070 | Mitotic sister chromatid segregation | 7.59E-27 | NEK2/NUSAP1/BUB1B/CENPE/SPAG5/PTTG1/KIF18B/KIF14/NCAPG/KIF18A/RACGAP1/CENPF/ESPL1/MAD2L1/ZWINT/NDC80/CCNB1/TUBG1/NCAPD3/KIF23/CDCA8/CDC20/KIF2C/DLGAP5/BUB1/KIF4A/TTK/PRC1/AURKB/TRIP13/CDT1/NCAPH/CDC6 |
GO: 0044770 | Cell cycle phase transition | 1.95E-24 | NEK2/BUB1B/CENPE/GTSE1/CCNE1/TYMS/POLE2/MELK/KIF14/CCNA2/CENPF/MCM2/ESPL1/MAD2L1/AURKA/FGFR1OP/BLM/RPA3/NDC80/RCC1/RECQL4/CCNB1/RRM2/DONSON/NDE1/HMMR/CKS2/TUBG1/KNTC1/E2F8/PLK4/BRCA1/EZH2/CDKN2A/TIMELESS/CCNE2/CDC20/TPX2/DLGAP5/BUB1/UBE2C/TTK/CCNB2/CHEK1/AURKB/FOXM1/CDKN3/MCM4/TRIP13/CDT1/CDC6 |
GO: 0044772 | Mitotic cell cycle phase transition | 3.96E-24 | NEK2/BUB1B/CENPE/GTSE1/CCNE1/TYMS/POLE2/MELK/KIF14/CCNA2/CENPF/MCM2/ESPL1/MAD2L1/AURKA/FGFR1OP/BLM/RPA3/NDC80/RCC1/RECQL4/CCNB1/RRM2/DONSON/NDE1/HMMR/CKS2/TUBG1/KNTC1/E2F8/PLK4/BRCA1/EZH2/CDKN2A/CCNE2/CDC20/TPX2/DLGAP5/BUB1/UBE2C/TTK/CCNB2/AURKB/FOXM1/CDKN3/MCM4/TRIP13/CDT1/CDC6 |
Downregulated | |||
GO: 0001525 | Angiogenesis | 1.87E-06 | TEK/MAP3K3/MMRN2/TMEM100/ETS1/PTPRM/SOX17/RECK/PPP1R16B/DCN/CX3CR1/SASH1/NPR1/TGFBR2/AGTR1/SLIT2/GATA2/GATA6/MEOX2/PDE3B/TCF21/JAM3/EMP2/KLF2/ANGPT1/STARD13/KDR/CDH5/FGF2/PTPRB/PECAM1/HGF/S1PR1/FGF18/ROBO4/PRKCB/GJA5/EDNRA/CAV1/ARHGAP24/CYR61/TAL1/CALCRL/ACVRL1/ENG/ANXA3/NR4A1/SEMA5A/HYAL1/EDN1/CX3CL1 |
GO: 0010632 | Regulation of epithelial cell migration | 4.99E-06 | TEK/EPB41L5/MAP3K3/MMRN2/ETS1/PTPRM/DCN/PRKCE/SASH1/MACF1/TGFBR2/SLIT2/GATA2/MEOX2/EMP2/RAB11A/ANGPT1/STARD13/KDR/FGF2/FGF18/CLASP2/PPM1F/BMPR2/PTPRG/ACVRL1/ANXA3/SEMA5A/HYAL1/EDN1 |
GO: 0090130 | Tissue migration | 5.52E-06 | TEK/EPB41L5/KANK2/MAP3K3/MMRN2/ETS1/PTPRM/DCN/FOXF1/PRKCE/SASH1/MACF1/TGFBR2/SLIT2/GATA2/MEOX2/EMP2/RAB11A/ANGPT1/STARD13/KDR/FGF2/PECAM1/ZEB2/FGF18/CLASP2/PPM1F/BMPR2/PTPRG/ACVRL1/ANXA3/NR4A1/SEMA5A/HYAL1/EDN1 |
GO: 0010631 | Epithelial cell migration | 6.36E-06 | TEK/EPB41L5/KANK2/MAP3K3/MMRN2/ETS1/PTPRM/DCN/PRKCE/SASH1/MACF1/TGFBR2/SLIT2/GATA2/MEOX2/EMP2/RAB11A/ANGPT1/STARD13/KDR/FGF2/PECAM1/ZEB2/FGF18/CLASP2/PPM1F/BMPR2/PTPRG/ACVRL1/ANXA3/NR4A1/SEMA5A/HYAL1/EDN1 |
GO: 0090132 | Epithelium migration | 6.36E-06 | TEK/EPB41L5/KANK2/MAP3K3/MMRN2/ETS1/PTPRM/DCN/PRKCE/SASH1/MACF1/TGFBR2/SLIT2/GATA2/MEOX2/EMP2/RAB11A/ANGPT1/STARD13/KDR/FGF2/PECAM1/ZEB2/FGF18/CLASP2/PPM1F/BMPR2/PTPRG/ACVRL1/ANXA3/NR4A1/SEMA5A/HYAL1/EDN1 |
GO: 1904018 | Positive regulation of vasculature development | 2.78E-05 | TEK/MAP3K3/TMEM100/ETS1/RAP1A/CFLAR/PPP1R16B/CX3CR1/SASH1/TGFBR2/AGTR1/GATA2/GATA6/KDR/CDH5/FGF2/HGF/FGF18/PRKCB/ACVRL1/ENG/ANXA3/SEMA5A/HYAL1/CX3CL1 |
GO: 1901888 | Regulation of cell junction assembly | 3.69E-05 | ARHGAP6/TEK/EPB41L5/DLC1/LIMCH1/RAP1A/PEAK1/MACF1/KDR/GPM6B/CLASP2/PPM1F/CAV1/ACVRL1/PRKCH/ACE/CLDN5 |
GO: 2000146 | Negative regulation of cell motility | 3.69E-05 | RGN/DLC1/MMRN2/PTPRM/ADARB1/LIMCH1/TBX5/SMAD7/RECK/DCN/CX3CR1/LRCH1/PRKG1/SLIT2/MEOX2/CXCL12/STARD13/SCAI/IL33/FGF2/PTGER4/CLASP2/PTPRG/MMP28/FBLN1/DUSP1/ACVRL1/ENG/PPARGC1A/NAV3/CX3CL1 |
GO: 0051271 | Negative regulation of cellular component movement | 3.94E-05 | RGN/DLC1/MMRN2/PTPRM/ADARB1/LIMCH1/TBX5/SMAD7/RECK/DCN/CX3CR1/LRCH1/PRKG1/SLIT2/MEOX2/CXCL12/STARD13/SCAI/IL33/FGF2/PTGER4/TGFBR3/CLASP2/PTPRG/MMP28/FBLN1/DUSP1/ACVRL1/ENG/PPARGC1A/NAV3/SEMA5A/CX3CL1 |
GO: 1901342 | Regulation of vasculature development | 3.94E-05 | TEK/MAP3K3/MMRN2/TMEM100/ETS1/PTPRM/RAP1A/CFLAR/PPP1R16B/DCN/CX3CR1/SASH1/NPR1/TGFBR2/AGTR1/GATA2/GATA6/MEOX2/PDE3B/EMP2/KLF2/STARD13/KDR/CDH5/FGF2/HGF/FGF18/PRKCB/ACVRL1/ENG/ANXA3/SEMA5A/HYAL1/CX3CL1 |
Table S2
ID | Description | P adjust | Gene ID |
---|---|---|---|
Up-regulated | |||
hsa03440 | Homologous recombination | 2.08E-04 | BRIP1/BRCA2/BLM/EME1/RPA3/BRCA1 |
hsa00240 | Pyrimidine metabolism | 1.07E-03 | NME1/TYMS/DTYMK/TK1/RRM2/DCTPP1 |
hsa04914 | Progesterone-mediated oocyte maturation | 1.72E-02 | CCNA2/MAD2L1/AURKA/CCNB1/BUB1/CCNB2 |
hsa03030 | DNA replication | 9.69E-06 | RNASEH2A/POLE2/MCM2/RPA3/FEN1/MCM4/RFC4 |
hsa04115 | p53 signaling pathway | 7.90E-05 | GTSE1/CCNE1/CCNB1/RRM2/CDKN2A/CCNE2/CCNB2/CHEK1 |
hsa04218 | Cellular senescence | 2.23E-03 | CCNE1/CCNA2/CCNB1/CDKN2A/CCNE2/CCNB2/CHEK1/FOXM1/MYBL2 |
hsa03460 | Fanconi anemia pathway | 5.58E-08 | BRIP1/FANCI/FANCD2/BRCA2/BLM/EME1/RPA3/RMI2/BRCA1/UBE2T |
hsa04114 | Oocyte meiosis | 7.90E-05 | CCNE1/PTTG1/ESPL1/MAD2L1/AURKA/CCNB1/CCNE2/CDC20/BUB1/CCNB2 |
hsa05166 | Human T-cell leukemia virus 1 infection | 2.94E-04 | BUB1B/CCNE1/PTTG1/CCNA2/ESPL1/MAD2L1/SLC2A1/CDKN2A/CCNE2/CDC20/CCNB2/FGFR1OP |
hsa04110 | Cell cycle | 1.68E-11 | BUB1B/CCNE1/PTTG1/CCNA2/MCM2/ESPL1/MAD2L1/CCNB1/CDKN2A/CCNE2/CDC20/BUB1/TTK/CCNB2/FGFR1OP/MCM4/BRIP1 |
Down-regulated | |||
hsa04270 | Vascular smooth muscle contraction | 1.41E-03 | MYH11/PRKCE/GUCY1A2/NPR1/AGTR1/PRKG1/CACNA1D/PLA2G1B/ADCY4/PRKCB/EDNRA/PPP1R12B/CALCRL/PPP1R14A/PRKCH/EDN1 |
hsa04924 | Renin secretion | 1.58E-03 | ADRB2/GUCY1A2/NPR1/AGTR1/CACNA1D/PDE3B/PTGER4/EDNRA/ADRB1/ACE/EDN1 |
hsa04514 | Cell adhesion molecules (CAMs) | 6.26E-03 | JAM2/PTPRM/ITGA8/CADM1/JAM3/CDH5/ITGAL/PECAM1/ESAM/CLDN18/ICAM2/HLA-E/SELPLG/NFASC/CLDN5 |
hsa04022 | cGMP-PKG signaling pathway | 6.26E-03 | ADRB2/ATP1A2/PRKCE/GUCY1A2/NPR1/AGTR1/PRKG1/CACNA1D/PDE3B/PDE5A/ADCY4/EDNRB/PIK3R5/TRPC6/EDNRA/ADRB1 |
hsa04670 | Leukocyte transendothelial migration | 1.41E-02 | JAM2/RAP1A/JAM3/CXCL12/CDH5/ITGAL/PECAM1/ITK/PRKCB/ESAM/CLDN18/CLDN5 |
Table S3
Cluster | ID | Description | P adjust |
---|---|---|---|
Cluster 1 | GO: 0007059 | Chromosome segregation | 1.01E-13 |
GO: 0140014 | Mitotic nuclear division | 1.01E-13 | |
GO: 0000280 | Nuclear division | 2.81E-12 | |
GO: 0048285 | Organelle fission | 6.48E-12 | |
GO: 0000070 | Mitotic sister chromatid segregation | 2.40E-11 | |
Cluster 2 | GO: 0007059 | Chromosome segregation | 1.01E-13 |
GO: 0140014 | Mitotic nuclear division | 1.01E-13 | |
GO: 0000280 | Nuclear division | 2.81E-12 | |
GO: 0048285 | Organelle fission | 6.48E-12 | |
GO: 0000070 | Mitotic sister chromatid segregation | 2.40E-11 | |
Cluster 3 | GO: 0035589 | G protein-coupled purinergic nucleotide receptor signaling pathway | 0.021666 |
GO: 0035590 | Purinergic nucleotide receptor signaling pathway | 0.021986 | |
GO: 0035588 | G protein-coupled purinergic receptor signaling pathway | 0.021986 | |
GO: 0050921 | Positive regulation of chemotaxis | 0.021986 | |
GO: 0050927 | Positive regulation of positive chemotaxis | 0.021986 | |
Cluster 4 | GO: 0010389 | Regulation of G2/M transition of the mitotic cell cycle | 5.27E-09 |
GO: 1902749 | Regulation of cell cycle G2/M phase transition | 7.15E-09 | |
GO: 0000086 | G2/M transition of the mitotic cell cycle | 1.91E-08 | |
GO: 0044839 | Cell cycle G2/M phase transition | 3.41E-08 | |
GO: 0000226 | Microtubule cytoskeleton organization | 7.26E-08 | |
Cluster 5 | GO: 0000209 | Protein polyubiquitination | 0.000605 |
GO: 0043687 | Post-translational protein modification | 0.066311 | |
GO: 0006511 | Ubiquitin-dependent protein catabolic process | 0.096811 | |
GO: 0019941 | Modification-dependent protein catabolic process | 0.096811 | |
Cluster 6 | GO: 0000280 | Nuclear division | 3.10E-07 |
GO: 0048285 | Organelle fission | 3.10E-07 | |
GO: 0140014 | Mitotic nuclear division | 9.30E-07 | |
GO: 0051301 | Cell division | 9.30E-07 | |
GO: 0030261 | Chromosome condensation | 9.69E-07 | |
Cluster 7 | GO: 0007188 | Adenylate cyclase-modulating G protein-coupled receptor signaling pathway | 1.83E-08 |
GO: 0019932 | Second-messenger-mediated signaling | 1.83E-08 | |
GO: 0007187 | G protein-coupled receptor signaling pathway coupled to cyclic nucleotide second messenger | 1.91E-08 | |
GO: 0019935 | Cyclic-nucleotide-mediated signaling | 4.95E-07 | |
GO: 0007189 | Adenylate cyclase-activating G protein-coupled receptor signaling pathway | 2.60E-06 |
Table S4
Datasets | GSE18842 | GSE19188 | GSE27262 | GSE33532 | |||||||
---|---|---|---|---|---|---|---|---|---|---|---|
LogFC | P adjust | LogFC | P adjust | LogFC | P adjust | LogFC | P adjust | ||||
BUB1B | 4.73 | 1.92E-36 | 3.61 | 8.79E-32 | 3.37 | 1.58E-12 | 3.87 | 7.69E-17 | |||
CCNB1 | 4.72 | 4.17E-41 | 3.81 | 7.10E-35 | 3.17 | 1.11E-12 | 4.33 | 2.31E-22 | |||
CENPE | 3.39 | 8.97E-37 | 2.61 | 1.04E-27 | 1.58 | 1.30E-05 | 2.77 | 1.55E-11 | |||
KIF18A | 2.27 | 1.02E-18 | 1.57 | 7.68E-14 | 1.47 | 1.95E-06 | 1.59 | 4.44E-08 | |||
MAD2L1 | 4.64 | 1.61E-37 | 2.98 | 2.10E-22 | 2.81 | 7.32E-12 | 3.79 | 1.88E-14 | |||
NDC80 | 3.64 | 3.92E-32 | 3.17 | 1.12E-27 | 2.54 | 2.79E-09 | 3.78 | 2.17E-16 |
Acknowledgments
Funding: This work was supported by
Footnote
Conflicts of Interest: All authors have completed the ICMJE uniform disclosure form (available at http://dx.doi.org/10.21037/tcr.2019.06.35). The authors have no conflicts of interest to declare.
Ethical Statement: The authors are accountable for all aspects of the work in ensuring that questions related to the accuracy or integrity of any part of the work are appropriately investigated and resolved. This study was conducted in accordance with the Declaration of Helsinki (as revised in 2013). The institutional ethical approval and informed consent were waived.
Open Access Statement: This is an Open Access article distributed in accordance with the Creative Commons Attribution-NonCommercial-NoDerivs 4.0 International License (CC BY-NC-ND 4.0), which permits the non-commercial replication and distribution of the article with the strict proviso that no changes or edits are made and the original work is properly cited (including links to both the formal publication through the relevant DOI and the license). See: https://creativecommons.org/licenses/by-nc-nd/4.0/.
References
- Gridelli C, Rossi A, Carbone DP, et al. Non-small-cell lung cancer. Nat Rev Dis Prim 2015;1:15009. [Crossref] [PubMed]
- Bray F, Ferlay J, Soerjomataram I, et al. Global Cancer Statistics 2018: GLOBOCAN Estimates of Incidence and Mortality Worldwide for 36 Cancers in 185 Countries. CA Cancer J Clin 2018;68:394-424. [Crossref] [PubMed]
- Herbst RS, Heymach JV, Lippman SM. Lung cancer. N Engl J Med 2008;359:1367-80. [Crossref] [PubMed]
- Hirsch FR, Scagliotti GV, Mulshine JL, et al. Lung cancer: current therapies and new targeted treatments. Lancet 2017;389:299-311. [Crossref] [PubMed]
- Chen Z, Fillmore CM, Hammerman PS, et al. Non-small-cell lung cancers: A heterogeneous set of diseases. Nat Rev Cancer 2014;14:535-46. [Crossref] [PubMed]
- Devarakonda S, Morgensztern D, Govindan R. Genomic alterations in lung adenocarcinoma. Lancet Oncol 2015;16:e342-51. [Crossref] [PubMed]
- Russo G, Zegar C, Giordano A. Advantages and limitations of microarray technology in human cancer. Oncogene 2003;22:6497-507. [Crossref] [PubMed]
- Broadhead ML, Clark JCM, Dass CR, et al. Microarray: An instrument for cancer surgeons of the future?: Surgical science. ANZ J Surg 2010;80:531-6. [Crossref] [PubMed]
- Jin B, Wang W, Du G, et al. Identifying hub genes and dysregulated pathways in hepatocellular carcinoma. Eur Rev Med Pharmacol Sci 2015;19:592-601. [PubMed]
- Fong KM, Sekido Y, Minna JD. Molecular pathogenesis of lung cancer. J Thorac Cardiovasc Surg 1999;118:1136-52. [Crossref] [PubMed]
- Tang Q, Zhang H, Kong M, et al. Hub genes and key pathways of non-small lung cancer identified using bioinformatics. Oncol Lett 2018;16:2344-54. [PubMed]
- Tian ZQ, Li ZH, Wen SW, et al. Identification of Commonly Dysregulated Genes in Non-small-cell Lung Cancer by Integrated Analysis of Microarray Data and qRT-PCR Validation. Lung 2015;193:583-92. [Crossref] [PubMed]
- Gentleman RC, Carey VJ, Bates DM, et al. Bioconductor: open software development for computational biology and bioinformatics. Genome Biol 2004;5:R80. [Crossref] [PubMed]
- Gautier L, Cope L, Bolstad BM, et al. affy--analysis of Affymetrix GeneChip data at the probe level. Bioinformatics 2004;20:307-15. [Crossref] [PubMed]
- Olson NE. The Microarray Data Analysis Process: From Raw Data to Biological Significance. NeuroRx 2006;3:373-83. [Crossref] [PubMed]
- Brettschneider J, Bolstad B, Collin F, et al. Quality assessment for short oligonucleotide microarray data. Technometrics 2008;50:241-64. [Crossref]
- Ritchie ME, Phipson B, Wu D, et al. Limma powers differential expression analyses for RNA-sequencing and microarray studies. Nucleic Acids Res 2015;43:e47. [Crossref] [PubMed]
- Pathan M, Keerthikumar S, Ang CS, et al. FunRich: An open access standalone functional enrichment and interaction network analysis tool. Proteomics 2015;15:2597-601. [Crossref] [PubMed]
- Győrffy B, Surowiak P, Budczies J, et al. Online Survival Analysis Software to Assess the Prognostic Value of Biomarkers Using Transcriptomic Data in Non-Small-Cell Lung Cancer. PLoS One 2013;8:e82241. [Crossref] [PubMed]
- Yu G, Wang LG, Han Y, et al. clusterProfiler: An R Package for Comparing Biological Themes Among Gene Clusters. OMICS 2012;16:284-7. [Crossref] [PubMed]
- Jin B, Wang W, Du G, et al. Identifying hub genes and dysregulated pathways in hepatocellular carcinoma. Eur Rev Med Pharmacol Sci 2015;19:592-601. [PubMed]
- Shannon P, Markiel A, Ozier O, et al. Cytoscape: A Software Environment for Integrated Models of Biomolecular Interaction Networks. Genome Res 2003;13:2498-504. [Crossref] [PubMed]
- Pruitt KD, Hogue CW, Groll M, et al. An automated method for finding molecular complexes in large protein interaction networks. Nucleic Acids Res 2001;29:137-40. [Crossref] [PubMed]
- Chin CH, Chen SH, Wu HH, et al. cytoHubba: Identifying hub objects and sub-networks from complex interactome. BMC Syst Biol 2014;8:S11-7. [Crossref] [PubMed]
- Tomczak K, Czerwińska P, Wiznerowicz M. The Cancer Genome Atlas (TCGA): An immeasurable source of knowledge. Contemp Oncol (Pozn) 2015;19:A68-77. [Crossref] [PubMed]
- Colaprico A, Silva TC, Olsen C, et al. TCGAbiolinks: An R/Bioconductor package for integrative analysis of TCGA data. Nucleic Acids Res 2016;44:e71. [Crossref] [PubMed]
- Tang Z, Li C, Kang B, et al. GEPIA: A web server for cancer and normal gene expression profiling and interactive analyses. Nucleic Acids Res 2017;45:W98-102. [Crossref] [PubMed]
- Uhlen M, Oksvold P, Fagerberg L, et al. Towards a knowledge-based Human Protein Atlas. Nat Biotechnol 2010;28:1248-50. [Crossref] [PubMed]
- Rao N, Lee YF, Ge R. Novel endogenous angiogenesis inhibitors and their therapeutic potential. Acta Pharmacologica Sinica 2015;36:1177-90. [Crossref] [PubMed]
- Hanahan D, Weinberg RA. Hallmarks of cancer: the next generation. Cell 2011;144:646-74. [Crossref] [PubMed]
- Alexius-Lindgren M, Andersson E, Lindstedt I, et al. The RECK gene and biological malignancy--its significance in angiogenesis and inhibition of matrix metalloproteinases. Anticancer Res 2014;34:3867-73. [PubMed]
- Michael IP, Orebrand M, Lima M, et al. Angiopoietin-1 deficiency increases tumor metastasis in mice. BMC Cancer 2017;17:539. [Crossref] [PubMed]
- Park S, Sorenson CM, Sheibani N, et al. PECAM-1 isoforms, eNOS and endoglin axis in regulation of angiogenesis. Clin Sci (Lond) 2015;129:217-34. [Crossref] [PubMed]
- Xie Z, Liu H, Geng M. Targeting sphingosine-1-phosphate signaling for cancer therapy. Sci China Life Sci 2017;60:585-600. [Crossref] [PubMed]
- Enomoto S, Mitsui K, Kawamura T, et al. Suppression of Slit2/Robo1 mediated HUVEC migration by Robo4. Biochem Biophys Res Commun 2016;469:797-802. [Crossref] [PubMed]
- Novodvorsky P, Chico TJ. The Role of the Transcription Factor KLF2 in Vascular Development and Disease. Prog Mol Biol Transl Sci 2014;124:155-88. [Crossref] [PubMed]
- Chen H, Lee J, Kljavin NM, et al. Requirement for BUB1B/BUBR1 in tumor progression of lung adenocarcinoma. Genes Cancer 2015;6:106. [PubMed]
- Zhang LL, Feng ZL, Su MX, et al. Downregulation of Cyclin B1 mediates nagilactone E-induced G2 phase cell cycle arrest in non-small cell lung cancer cells. Eur J Pharmacol 2018;830:17-25. [Crossref] [PubMed]
- Shi YX, Zhu T, Zou T, et al. Prognostic and predictive values of CDK1 and MAD2L1 in lung adenocarcinoma. Oncotarget 2016;7:85235-43. [Crossref] [PubMed]
- Wang Y, Yu L, Wang T. MicroRNA-374b inhibits the tumor growth and promotes apoptosis in non-small cell lung cancer tissue through the p38/ERK signaling pathway by targeting JAM-2. J Thorac Dis 2018;10:5489-98. [Crossref] [PubMed]
- Zhao H, Ahirwar DK, Oghumu S, et al. Endothelial Robo4 suppresses breast cancer growth and metastasis through regulation of tumor angiogenesis. Mol Oncol 2016;10:272-81. [Crossref] [PubMed]
- Chen WF, Gao WD, Li QL, et al. SLIT2 inhibits cell migration in colorectal cancer through the AKT-GSK3β signaling pathway. Int J Colorectal Dis 2013;28:933-40. [Crossref] [PubMed]
- Chang PH, Hwang-Verslues WW, Chang YC, et al. Activation of Robo1 signaling of breast cancer cells by Slit2 from stromal fibroblast restrains tumorigenesis via blocking PI3K/Akt/β-catenin pathway. Cancer Res 2012;72:4652-61. [Crossref] [PubMed]
- Piao J, Sun J, Yang Y, et al. Target gene screening and evaluation of prognostic values in non-small cell lung cancers by bioinformatics analysis. Gene 2018;647:306-11. [Crossref] [PubMed]
- Metodieva SN, Nikolova DN, Cherneva RV. Expression analysis of angiogenesis-related genes in Bulgarian patients with early-stage non-small cell lung cancer. Tumori 2011;97:86-94. [Crossref] [PubMed]
- Goldstein B, Trivedi M, Speth RC. Alterations in Gene Expression of Components of the Renin-Angiotensin System and Its Related Enzymes in Lung Cancer. Lung Cancer Int 2017;2017:6914976. [Crossref] [PubMed]
- Wegman-Ostrosky T, Soto-Reyes E, Vidal-Millán S, et al. The renin-angiotensin system meets the hallmarks of cancer. J Renin Angiotensin Aldosterone Syst 2015;16:227-33. [Crossref] [PubMed]
- Silk AD, Zasadil LM, Holland AJ, et al. Chromosome missegregation rate predicts whether aneuploidy will promote or suppress tumors. Proc Natl Acad Sci 2013;110:E4134-41. [Crossref] [PubMed]
- Rath O, Kozielski F. Kinesins and cancer Oliver. Nat Rev Cancer 2012;12:527-39. [Crossref] [PubMed]
- El-Arabey AA, Salama SA, Abd-Allah AR. CENP-E as a target for cancer therapy: Where are we now? Life Sci 2018;208:192-200. [Crossref] [PubMed]
- Kung PP, Martinez R, Zhu Z, et al. Chemogenetic Evaluation of the Mitotic Kinesin CENP-E Reveals a Critical Role in Triple-Negative Breast Cancer. Mol Cancer Ther 2014;13:2104-15. [Crossref] [PubMed]
- Zhu H, Xu W, Zhang H, et al. Targeted deletion of Kif18a protects from colitis-associated colorectal (CAC) tumors in mice through impairing Akt phosphorylation. Biochem Biophys Res Commun 2013;438:97-102. [Crossref] [PubMed]
- Alfarsi LH, Elansari R, Toss MS, et al. Kinesin family member-18A (KIF18A) is a predictive biomarker of poor benefit from endocrine therapy in early ER+ breast cancer. Breast Cancer Res Treat 2019;173:93-102. [Crossref] [PubMed]
- Zhang C, Zhu C, Chen H, et al. Kif18A is involved in human breast carcinogenesis. Carcinogenesis 2010;31:1676-84. [Crossref] [PubMed]