A 4-miRNA signature act as a novel prognostic biomarker in patients with Sarcoma
Introduction
Sarcomas are malignant tumors originating from mesenchymal tissue (1). It has more than 50 subtypes and can occur throughout the body. There are more than 130,000 new sarcoma cases that are diagnosed in the world every year, accounting for 1–3% of all tumors (2). Sarcomas metastasize through the blood; once metastasized the prognosis is poor. The identification of sarcoma-associated molecular markers that help to predict progression and prognosis is important in clinical application. It was found that p53 (3), RB (4), PI3K (5) and other genes are involved in the progression of sarcomas.
MiRNAs are small regulatory RNAs of approximately 22 nucleotides (nt) in length, which regulate the expression of genes by interacting with target mRNAs. MiRNAs are involved in the occurrence, development and metastasis of cancer, by regulating the expression of genes related to cytokines, growth factors, pro-apoptotsis and anti-apoptosis (6,7). Recent studies have found that tumor cells can secrete miRNAs into the bloodstream (8-10), and that circulating miRNAs are found in patients with RMS (rhabdomyosarcoma, rhabdomyosarcoma) and MPNST (neural sheath tumors) (11). MiRNAs in serum or plasma may become a new method for screening or detecting cancer.
A large number of studies have found that miRNAs are involved in the development of sarcomas and miRNAs may become a new indicator for predicting the prognosis of disease. The expression of miR-126, -223, -451, and -1274b were significantly higher in pleomorphic undifferentiated sarcoma (UPS) tissues compared with controls (12). On the other hand, miRNAs can also be used to predict prognosis of sarcoma patients. Sarcoma patients with a higher level of miR-138-5p show a better prognosis (13). Moreover, miR-1301 is involved in the proliferation, migration and invasion of osteosarcoma cells (14). In Kaposi’ sarcoma, miRNA-126 regulates the phosphatidylinositol-3 kinase (PI3K)/protein kinase B (AKT) pathway (15). MiR-34a, miR-93 and miR-200c can destroy the generation of fast-growing osteosarcoma vessels (16). Although these miRNAs could predict the prognosis of sarcoma patients, the specificity and sensitivity were not well for clinical application. Predictive models based on expression characteristics of multiple miRNAs can improve specificity and sensitivity to prognosis prediction. There were many studied build prognosis predict model by combined the miRNA expression signature in different cancers, such as breast cancer (17), clear cell renal cell carcinoma (18) and cervical cancer (19). However, there was rarely study used miRNA expression signature to predict the prognosis of Sarcoma.
In this study, we attempted to identify miRNAs involved in the progression of sarcomas by mining the miRNA data of sarcoma tissues downloaded from the TCGA database. The Cox proportional hazard regression model was used to identify the expression signatures of miRNA, and to establish a risk prediction model for the prognosis of the Sarcoma patients.
Methods
Data sources
MiRNA data and of clinical data of sarcoma patients were downloaded from the TCGA database in October 2018. A total of 259 samples were included in the miRNA-Seq data. Clinical data predominantly included the overall survival (OS) information. The OS was defined as the date from the patient's diagnosis to the date of death or the end of follow-up. The specific information is detailed in http://fp.amegroups.cn/cms/tcr.2019.07.46-1.pdf. The downloaded data is sorted by the R software. Patients were randomly assigned to a training set and a validation set, the training set (N=129) was used to screen for key miRNAs, and a validation set (N=129) was used to develop miRNA signatures. The expression abundance (FPKM) of miRNAs of all samples was converted as log2(X+1) to make the data consistent with the normal distribution (X means the FPKM value of miRNA). If the log2(X+1) value of miRNA expression is greater than 0 in more than 50% samples, it would be defined as high expressed miRNA.
Identification and screening of prognosis-related miRNAs
The training set data was used to identify miRNAs related to the OS by the univariable cox regression of the R language’s survival package. miRNAs of P<0.05 were selected as candidate. To improve the accuracy and reliability of prognostic analysis based on miRNA, the relationship between candidate miRNAs and survival was further analyzed. The data of all 259 cases were randomly assigned to the training set and test set again. The training data set was N*(1–P), and the test set data was N*P (P=1/3). Robust likelihood-based survival analysis was taken by the rbsurv package of R language. Each of the identified prognostic-related miRNAs in the training set was screened using univariate cox proportional regression model and the correlation parameters for each miRNA were generated. In the validation set, the log likelihood of each miRNA corresponding parameter estimate is further evaluated in the validation set. This process was repeated 10 times independently. Each miRNA generated 10 log-likelihoods, and the gene with the largest log-likelihood average was used as the optimal miRNA, abbreviated as mi(a). Then mi(a) and other miRNAs form a 2-miRNA model, and each 2-miRNA model is evaluated, and the miRNA with the largest log likelihood average is selected as the second optimal miRNA, mi(b). The above steps were repeated to obtain a series of predictive models. The candidate miRNAs were fitted to the Akaike Information Standard (AIC), and the miRNA with the lowest AIC value were selected to establish the prediction model.
Establish and verify risk score formula
According to the method as Mao et al. (20) established, the multivariate Cox regression were used to analyze the risk scores of each patient according to the prognosis-related miRNAs. According to the median risk score, patients in the training set were divided into high-risk and low-risk groups. The receiver operating characteristic (ROC) curve was drawn using the survival ROC package of R language, and the threshold with the highest sensitivity and specificity was selected. Based on this threshold, the Kaplan-Meier (KM) curve was used to analyze the survival of patients in the high-risk group and the low-risk group. Differences were compared using log-rank detection. Finally, the meaning risk scoring formula is verified by using the verification set and all data sets.
Prediction of miRNA target genes and function annotation
MirTissue (21) is a web service that based on validated miRNA-target interactions linked to a specific tissue context, specifically related to cancer pathologies, which was also used to screen the targets of the key miRNAs. The mirTissue database includes 15 projects, which are bladder urothelial carcinoma (BLCA), breast invasive carcinoma (BRCA), cervical squamous cell carcinoma & endocervical adenocarcinoma (CESC), cholangiocarcinoma (CHOL), colon adenocarcinoma (COAD), lymphoid neoplasm diffuse large B-cell lymphoma (DLBC), head and neck squamous cell carcinoma (HNSC), kidney chromophobe (KICH), kidney renal clear cell carcinoma (KIRC), kidney renal papillary cell carcinoma (KIRP), liver hepatocellular carcinoma (LIHC), lung adenocarcinoma (LUAD), prostate adenocarcinoma (PRAD), uterine corpus endometrial carcinoma (UCEC), adrenocortical carcinoma (ACC). In this study, the target genes of the key miRNAs were analyzed by mirTissue, the targets were filtered if the interaction between miRNA and targets was inhibition or degradation (P<0.01). The interactions of the target genes were analyzed by CytoScape (22).
The functional annotation of the target genes was analyzed by DAVID 6.8 (23,24), a web service of functional annotation tool. The pathways of the target genes involved in the biological pathways were analyzed by KEGG_PATHWAY tool in DAVID.
Results
Screening and identification of prognosis-associated miRNAs
The clinical data and expression value of miRNAs of 259 Sarcoma samples were downloaded from Genomic Data Commons Data Portal by Data transfer tool. The clinical features, primary site and disease type of sarcoma and number of samples were shown in Table 1. According to criteria of the log2(FPKM + 1)>0 in more than 50% samples, 499 high expressed miRNAs were identified from the 1,881 downloaded miRNAs. The list of the miRNAs was shown in supplementary Table 2. The 259 samples were randomly divided into a training set and a validation set. The univariate Cox proportional hazards regression method revealed that a total of 50 miRNAs (P<0.01) had significant prognostic value for sarcomas (http://fp.amegroups.cn/cms/tcr.2019.07.46-3.pdf). The top 20 miRNA with the lowest P value were further analyzed by robust likelihood-based survival model, and 4 miRNAs (hsa-miR-190b, hsa-miR-3170, hsa-miR-4762 and hsa-miR-18a) were identified as independent prognostic indicators of sarcoma (Table 2).
Table 1
Clinical characteristic | Number of patients |
---|---|
Primary site | |
Connective, subcutaneous and other soft tissues | 116 |
Retroperitoneum and peritoneum | 101 |
Uterus, NOS | 26 |
Corpus uteri | 4 |
Bones, joints and articular cartilage of limbs | 2 |
Kidney | 2 |
Stomach | 2 |
Colon | 1 |
Meninges | 1 |
Other and unspecified male genital organs | 1 |
Other and unspecified parts of tongue | 1 |
Ovary | 1 |
Peripheral nerves and autonomic nervous system | 1 |
Disease type | |
Myomatous neoplasms | 104 |
Lipomatous neoplasms | 61 |
Fibromatous neoplasms | 39 |
Soft tissue tumors and sarcomas, NOS | 35 |
Synovial-like Neoplasms | 10 |
Nerve sheath tumors | 10 |
Gender | |
Female | 140 |
Male | 119 |
Race | |
White | 227 |
Black or African American | 18 |
Not reported | 8 |
Asian | 6 |
Vital status | |
Alive | 161 |
Dead | 98 |
Ages at diagnosis | |
≤60 | 126 |
>60 | 132 |
The age of one patient (ID: TCGA-WP-A9GB) was missing.
Table 2
ID | nloglik | AIC |
---|---|---|
hsa-mir-190b | 198.69 | 399.38* |
hsa-mir-3170 | 191.83 | 387.66* |
hsa-mir-4762 | 187.16 | 380.31* |
hsa-mir-18a | 185.11 | 378.21* |
hsa-mir-1288 | 184.32 | 378.65 |
hsa-mir-744 | 184.27 | 380.55 |
hsa-mir-3677 | 183.56 | 381.12 |
hsa-mir-6783 | 183.3 | 382.59 |
hsa-mir-130b | 182.98 | 383.95 |
hsa-mir-19b-1 | 181.23 | 382.45 |
hsa-mir-19a | 180.88 | 383.77 |
hsa-mir-361 | 179.97 | 383.94 |
hsa-mir-581 | 178.34 | 382.68 |
hsa-mir-19b-2 | 178.34 | 384.68 |
hsa-mir-92a-2 | 177.28 | 384.57 |
hsa-mir-17 | 176.58 | 385.15 |
hsa-mir-29c | 173.89 | 381.77 |
hsa-mir-20a | 172.82 | 381.65 |
hsa-mir-92a-1 | 171.47 | 380.94 |
*P<0.05.
Risk score based on 4-miRNA signatures and prognosis of sarcomas
To comprehensively analyze the relationship between 4-miRNA and Sarcoma prognosis, a 4-miRNA-based risk score was developed, based on Cox coefficients:
The survival status of each patient and the expression level of the 4 miRNAs in each patient was shown in Figure 1A. It was shown that the survival time of Sarcoma patients was negatively correlated with the risk score. Most deceased patients had higher risk scores, while those with longer survival times reported lower risk scores. Hsa-miR-190b, hsa-miR-3170, hsa-miR-4762 and hsa-miR-18a4 miRNAs were highly expressed in high-risk patients, especially hsa-miR-18a. While the expression of hsa-miR-190b, hsa-miR-3170 and hsa-miR-4762 were higher in the low-risk patients.
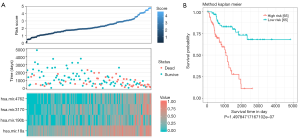
According to this formula, all patients in the train set were given a risk score and were divided into a high-risk group (n=65) and a low risk group (n=65) by using the corresponding median risk score as the cut-off. According to the Kaplan-Meier curve and log-rank analysis, patients in the low-risk group had a longer survival (P<0.0001) (Figure 1B).
4-miRNA features can be used as effective predictive markers for sarcomas
ROC was used to analyze the sensitivity and specificity in survival prediction of the risk score based on 4-miRNA signatures. As shown in Figure 2, the AUC (area under curve) value was 0.722, and most of the cut-off points were able to reach a decent classification. At the threshold of 2.574, both sensitivity and specificity were the highest. Based on this threshold, patients in the validation set were divided into a high-risk group (n=39) and low-risk group (n=91). According to the Kaplan-Meier curve and log-rank analysis, the survival of patients in the high-risk group and low-risk group was significant different (P<0.0001).
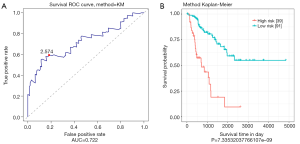
Verify the role of 4-miRNA signatures in survival prediction
The risk scores of 4-miRNA signatures were analyzed using validation set data and the entire data collection. According to the optimal threshold determined by the ROC curve, patients in all data sets were divided into a high-risk group (n=80) and a low-risk group (n=179). Similar to previous results, survival of high-risk groups and low-risk groups were significantly different (P<0.0001) (Figure 3A). In the validation data set, patients were divided into a high-risk group (n=41) and a low-risk group (n=88), and there was a significant difference in survival between the high-risk group and the low-risk group (P<0.01) (Figure 3B). The optimal cut-off point selected by ROC analysis could divided the patients into high-risk and low-risk groups effectively both in all data set and validation data set.
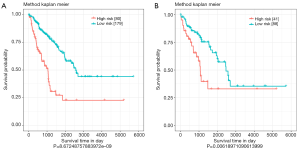
Target genes prediction and function analysis
The target genes of the 4 miRNAs was analyzed in mirTissue database. There was no SARC-project data in the mirTissue, so the pan-cancers including 15 projects (ACC, BLCA, BRCA, CESC, CHOL, COAD, DLBC, HNSC, KICH, KIRC, KIRP, LIHC, LUAD, PRAD, UCEC) were used to analyze the targets of the 4 miRNAs. The targets were filtered if the interaction was degradation or inhibition (P<0.01) and the miRNA-gene interactions were detected in one cancer at least. As shown in http://fp.amegroups.cn/cms/tcr.2019.07.46-4.pdf, 324 genes were filtered as targets of hsa-miR-18a, 77 genes were filtered as targets of hsa-miR-3170, 41genes were filtered as targets of hsa-miR-4762, and 31 genes were filtered as targets of hsa-miR-190b. The network of these 4 miRNAs and their targets were analyzed by CytoScape (Figure 4). It was found that, MEF2D, RAB5C, CDKN1A, DNASE2, APOL6, RAB13, and HACD4 genes were targets of both hsa-miR-18a and hsa-miR-3170, RUNX1 was target of hsa-miR-18a and hsa-miR-4762, BCL2 was target of hsa-miR-18a and hsa-miR-190b, POLL was target of hsa-miR-3170 and hsa-miR-4762.
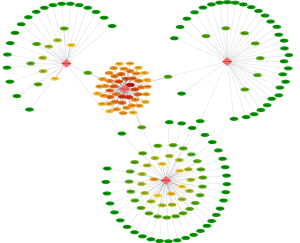
To further analyze the function of the miRNAs and these target genes, the function of the target genes was annotated by DAVID 6.8. As shown in Table 3, the target genes were involved in 20 biological pathways annotated by KEGG_pathway database, most of the signaling pathway were cancer related.
Table 3
Term | Genes | Gene count | P value |
---|---|---|---|
FoxO signaling pathway | GRB2, MAP2K2, TGFBR2, SMAD4, SMAD3, IGF1, SMAD2, MAPK10, IL7R, PTEN, ATM, CDKN1A, CCND1, KRAS, MAPK13, PIK3R3 | 16 | 2.62E-06 |
Pathways in cancer | GNA13, COL4A1, MSH3, GRB2, MAP2K2, TGFBR2, MITF, SMAD4, EGLN3, FGF11, ITGA2, SMAD3, IGF1, SMAD2, MAPK10, GLI2, PTEN, MAX, CCND1, CDKN1A, KRAS, HIF1A, BCL2, TGFA, FAS, RUNX1, PIK3R3 | 27 | 1.59E-05 |
Colorectal cancer | CCND1, KRAS, MSH3, BCL2, TGFBR2, SMAD4, SMAD3, SMAD2, MAPK10, PIK3R3 | 10 | 3.59E-05 |
Prolactin signaling pathway | CCND1, KRAS, TNFSF11, MAPK13, GRB2, MAP2K2, ESR1, MAPK10, SOCS5, PIK3R3 | 10 | 1.07E-04 |
Thyroid hormone signaling pathway | MED4, CCND1, KRAS, HIF1A, THRA, NCOA3, MAP2K2, NOTCH4, ESR1, ATP1A1, MED13L, PIK3R3 | 12 | 2.47E-04 |
Proteoglycans in cancer | GRB2, MAP2K2, ESR1, IGF1, ITGA2, DDX5, SDC4, PPP1CB, CDKN1A, CCND1, HIF1A, KRAS, EZR, MAPK13, FAS, PIK3R3 | 16 | 2.96E-04 |
Glioma | CDKN1A, CCND1, KRAS, GRB2, MAP2K2, TGFA, IGF1, PIK3R3, PTEN | 9 | 3.20E-04 |
Pancreatic cancer | CCND1, KRAS, TGFBR2, SMAD4, TGFA, SMAD3, SMAD2, MAPK10, PIK3R3 | 9 | 3.20E-04 |
Renal cell carcinoma | KRAS, HIF1A, PAK3, GRB2, MAP2K2, EGLN3, TGFA, RAP1A, PIK3R3 | 9 | 3.56E-04 |
Focal adhesion | VAV3, COL4A1, DIAPH1, GRB2, ITGA2, IGF1, MAPK10, PPP1CB, PTEN, CCND1, PAK3, BCL2, RAP1A, PIK3R3, THBS2, MYLK | 16 | 4.07E-04 |
Prostate cancer | CDKN1A, CCND1, KRAS, GRB2, MAP2K2, BCL2, TGFA, IGF1, PIK3R3, PTEN | 10 | 5.55E-04 |
Melanoma | CDKN1A, CCND1, KRAS, MAP2K2, MITF, FGF11, IGF1, PIK3R3, PTEN | 9 | 5.88E-04 |
Chronic myeloid leukemia | CDKN1A, CCND1, KRAS, GRB2, MAP2K2, TGFBR2, SMAD4, RUNX1, PIK3R3 | 9 | 6.46E-04 |
Hippo signaling pathway | PARD6B, CCND1, CTGF, BTRC, TGFBR2, SMAD4, WWC1, SMAD3, SMAD2, GLI2, WWTR1, PPP1CB, BMPR1A | 13 | 7.20E-04 |
Signaling pathways regulating pluripotency of stem cells | KRAS, MAPK13, GRB2, MAP2K2, IL6ST, SMAD4, SMAD3, IGF1, SMAD2, ID4, PIK3R3, BMPR1A | 12 | 0.001312184 |
Hepatitis B | CDKN1A, CCND1, KRAS, GRB2, MAP2K2, BCL2, MAP3K1, SMAD4, FAS, MAPK10, PIK3R3, PTEN | 12 | 0.001743177 |
Sphingolipid signaling pathway | GNA13, KRAS, SGMS2, MAPK13, MAP2K2, BCL2, MAPK10, PIK3R3, PTEN, DEGS1 | 10 | 0.004867618 |
Neurotrophin signaling pathway | RPS6KA5, KRAS, MAPK13, GRB2, MAP2K2, BCL2, MAP3K1, RAP1A, MAPK10, PIK3R3 | 10 | 0.004867618 |
ErbB signaling pathway | CDKN1A, KRAS, PAK3, GRB2, MAP2K2, TGFA, MAPK10, PIK3R3 | 8 | 0.008674856 |
Fc epsilon RI signaling pathway | VAV3, KRAS, MAPK13, GRB2, MAP2K2, MAPK10, PIK3R3 | 7 | 0.009595195 |
Discussion
Sarcomas occur in multiple sites of the body, although the molecular mechanism of its pathogenesis is not identical, there are still some genetic changes in various sarcomas. The study found that TP53, ATRX and RB1 genes are mutated in a variety of sarcoma types, and gene copy number changes occur in a variety of sarcomas, affecting the cell cycle and other biological pathways. The genetic changes in sarcomas have their own unique features, which are not the same as other cancers (25). Clinically, due to the low incidence of sarcomas, it is often misdiagnosed and not easy to be found in the early stage. Therefore, the development of molecular markers for screening and monitoring sarcomas would be important in their clinical application.
This study used the clinical data and miRNA expression data from the TCGA Sarcoma patients to identify miRNA related to prognosis. First, we identified 499 miRNAs with a high abundance in sarcoma tissue samples. Then based on the Cox risk proportional regression model, a prognostic prediction model of 4-miRNA (hsa-miR-190b, hsa-miR-3170, hsa-miR-4762, hsa-miR-18a) expression signatures was established. Based on this model, patients were divided into a high-risk and a low-risk group; the high-risk patients had significantly lower survival times than low-risk patients. The ROC curve was used to optimize the model to further improve the accuracy and specificity. The patients were regrouped based on the new threshold, and the same results were found in both the validation set and the entire data set. This prediction model has a high value in predicting the survival of sarcoma patients.
The four miRNAs identified in this study, hsa-miR-190b, hsa-miR-3170, hsa-miR-4762, hsa-miR-18a, were enriched in sarcoma tissue samples. The current study found that hsa-miR-18a plays a role in a variety of tumors; in the hepatocellular carcinoma patients, hsa-mi-18a was involved in the regulation of EGFR and IL-6 pathway, and it can be used as a prognostic factor (26). In basal cell carcinoma patients, the expression abundance of hsa-miR-18a was much higher (27). In lung squamous cell carcinoma patients with a higher abundance of hsa-mi-18a often had a poor prognosis (28). Also, hsa-miR-18a was associated with the prognosis of esophageal cancer (29). Hsa-mir-3170 can be used to predict survival in patients with endometrial cancer (30). At present, no reports on hsa-miR-190b and hsa-miR-4762 in tumor research have been found. This provides a new research direction for sarcomas.
The target genes of the 4 miRNAs were investigated by miRTissue database, which includes expression values of both miRNA and mRNA belonging to 15 types of tumor tissues, however there was no sarcoma data. The interaction between miRNA and mRNA were annotated as degradation, inhibition and no interaction. It was found that 324 genes,77 genes, 41 genes and 31 genes were filtered as degradation targets of hsa-miR-18a, hsa-miR-3170, 41 hsa-miR-4762, and hsa-miR-190b, respectively (http://fp.amegroups.cn/cms/tcr.2019.07.46-4.pdf). According to the function annotation and KEGG pathway analysis, some target genes were involved in cancer signaling pathways. This may indicate that the 4 miRNAs were involved in cancer progression by regulating their target genes. On the other hand, the interaction network of miRNAs and their target genes were analyzed, hsa-miR-18a targeted the same genes with hsa-miR-3170, hsa-miR-4762 or hsa-miR-190b. The weight of hsa-miR-18a was the lowest in the prediction model, however, it has the most target genes and crosstalks with other miRNAs. Overall, hsa-miR-18a, hsa-miR-3170, 41 hsa-miR-4762, and hsa-miR-190b played important roles in cancer prognosis, this study may suggest the roles of the 4 miRNAs in sarcoma, which need to be verified by experiment in future.
Conclusions
Based on data pertaining to TCGA sarcoma miRNA and survival rates, this study developed a 4-miRNA signature risk prediction model that can effectively predict patient prognosis. These 4 miRNAs may regulate the progression of sarcoma by cross-talks between their target genes.
Acknowledgments
We thank Ms. Zhao Junya for her assistance in language editing.
Funding: None.
Footnote
Conflicts of Interest: All authors have completed the ICMJE uniform disclosure form (available at http://dx.doi.org/10.21037/tcr.2019.07.46). The authors have no conflicts of interest to declare.
Ethical Statement: The authors are accountable for all aspects of the work in ensuring that questions related to the accuracy or integrity of any part of the work are appropriately investigated and resolved. The data used in this study were downloaded from TCGA, additional approval by ethics committee was not obligatory. Data was processed according to the TCGA human subject protection and data access policies. The study was conducted in accordance with the Declaration of Helsinki (as revised in 2013). Informed consent was waived.
Open Access Statement: This is an Open Access article distributed in accordance with the Creative Commons Attribution-NonCommercial-NoDerivs 4.0 International License (CC BY-NC-ND 4.0), which permits the non-commercial replication and distribution of the article with the strict proviso that no changes or edits are made and the original work is properly cited (including links to both the formal publication through the relevant DOI and the license). See: https://creativecommons.org/licenses/by-nc-nd/4.0/.
References
- Chen H, Shen J, Choy E, et al. Targeting protein kinases to reverse multidrug resistance in sarcoma. Cancer Treat Rev 2016;43:8-18. [Crossref] [PubMed]
- Ito M, Barys L, O'Reilly T, et al. Comprehensive mapping of p53 pathway alterations reveals an apparent role for both SNP309 and MDM2 amplification in sarcomagenesis. Clin Cancer Res 2011;17:416-26. [Crossref] [PubMed]
- Toguchida J, Yamaguchi T, Ritchie B, et al. Mutation spectrum of the p53 gene in bone and soft tissue sarcomas. Cancer Res 1992;52:6194-9. [PubMed]
- Chen X, Bahrami A, Pappo A, et al. Recurrent somatic structural variations contribute to tumorigenesis in pediatric osteosarcoma. Cell Rep 2014;7:104-12. [Crossref] [PubMed]
- Courtney KD, Corcoran RB, Engelman JA. The PI3K pathway as drug target in human cancer. J Clin Oncol 2010;28:1075-83. [Crossref] [PubMed]
- Fukao A, Fujiwara T. The coupled and uncoupled mechanisms by which trans-acting factors regulate mRNA stability and translation. J Biochem 2017;161:309-14. [PubMed]
- Hemmatzadeh M, Mohammadi H, Karimi M, et al. Differential role of microRNAs in the pathogenesis and treatment of Esophageal cancer. Biomed Pharmacother 2016;82:509-19. [Crossref] [PubMed]
- Cantara S, Pilli T, Sebastiani G, et al. Circulating miRNA95 and miRNA190 are sensitive markers for the differential diagnosis of thyroid nodules in a Caucasian population. J Clin Endocrinol Metab 2014;99:4190-8. [Crossref] [PubMed]
- Duroux-Richard I, Pers YM, Fabre S, et al. Circulating miRNA-125b is a potential biomarker predicting response to rituximab in rheumatoid arthritis. Mediators Inflamm 2014;2014:342524. [Crossref] [PubMed]
- El-Abd NE, Fawzy NA, El-Sheikh SM, et al. Circulating miRNA-122, miRNA-199a, and miRNA-16 as biomarkers for early detection of hepatocellular carcinoma in egyptian patients with chronichepatitis C virus infection. Mol Diagn Ther 2015;19:213-20. [Crossref] [PubMed]
- Fujiwara T, Kunisada T, Takeda K, et al. MicroRNAs in Soft Tissue Sarcomas: Overview of the Accumulating Evidence and Importance as Novel Biomarkers. BioMed Research International 2014;2014:1-15. [PubMed]
- Guled M, Pazzaglia L, Borze I, et al. Differentiating soft tissue leiomyosarcoma and undifferentiated pleomorphic sarcoma: a miRNA analysis. Genes Chromosomes Cancer 2014;53:693-702. [Crossref] [PubMed]
- Gonzalez Dos Anjos L, Almeida BC, Gomes AT, et al. Could miRNA Signatures be Useful for Predicting Uterine Sarcoma and Carcinosarcoma Prognosis and Treatment. Cancers (Basel) 2018;10: [Crossref] [PubMed]
- Wang L, Hu K, Chao Y. MicroRNA-1301 inhibits migration and invasion of osteosarcoma cells by targeting BCL9. Gene 2018;679:100-7. [Crossref] [PubMed]
- Lu G, Wu X, Zhao ZF, et al. MicroRNA-126 regulates the phosphatidylinositol-3 kinase (PI3K)/protein kinase B (AKT) pathway in SLK cells in vitro and the expression of its pathway members in Kaposi's sarcoma tissue. Medicine (Baltimore) 2018;97:e11855. [Crossref] [PubMed]
- Tiram G, Segal E, Krivitsky A, et al. Identification of Dormancy-Associated MicroRNAs for the Design of Osteosarcoma-Targeted Dendritic Polyglycerol Nanopolyplexes. ACS Nano 2016;10:2028-45. [Crossref] [PubMed]
- Shi W, Dong F, Jiang Y, et al. Construction of prognostic microRNA signature for human invasive breast cancer by integrated analysis. Onco Targets Ther 2019;12:1979-2010. [Crossref] [PubMed]
- Luo Y, Chen L, Wang G, et al. Identification of a three-miRNA signature as a novel potential prognostic biomarker in patients with clear cell renal cell carcinoma. J Cell Biochem 2019;120:13751-64. [Crossref] [PubMed]
- Shi C, Yang Y, Zhang L, et al. Optimal subset of signature miRNAs consisting of 7 miRNAs that can serve as a novel diagnostic and prognostic predictor for the progression of cervical cancer. Oncol Rep 2019;41:3167-78. [PubMed]
- Mao X, Qin X, Li L, et al. A 15-long non-coding RNA signature to improve prognosis prediction of cervical squamous cell carcinoma. Gynecol Oncol 2018;149:181-7. [Crossref] [PubMed]
- Fiannaca A, La Rosa M, La Paglia L, et al. miRTissue: a web application for the analysis of miRNA-target interactions in human tissues. BMC Bioinformatics 2018;19:434. [Crossref] [PubMed]
- Shannon P, Markiel A, Ozier O, et al. Cytoscape: a software environment for integrated models of biomolecular interaction networks. Genome Research 2003;13:2498-504. [Crossref] [PubMed]
- Huang da W. Sherman BT, Lempicki RA. Systematic and integrative analysis of large gene lists using DAVID Bioinformatics Resources. Nat Protoc 2009;4:44-57. [Crossref] [PubMed]
- Huang DW, Sherman BT, Lempicki RA. Bioinformatics enrichment tools: paths toward the comprehensive functional analysis of large gene lists. Nucleic Acids Res 2009;37:1-13. [Crossref] [PubMed]
- Cancer Genome Atlas Research Network. Comprehensive and Integrated Genomic Characterization of Adult Soft Tissue Sarcomas. Cell 2017;171:950-65.e28. [Crossref] [PubMed]
- Awan FM, Naz A, Obaid A, et al. MicroRNA pharmacogenomics based integrated model of miR-17-92 cluster in sorafenib resistant HCC cells reveals a strategy to forestall drug resistance. Sci Rep 2017;7:11448. [Crossref] [PubMed]
- Sand M, Skrygan M, Sand D, et al. Expression of microRNAs in basal cell carcinoma. Br J Dermatol 2012;167:847-55. [Crossref] [PubMed]
- Gao W, Shen H, Liu L, et al. MiR-21 overexpression in human primary squamous cell lung carcinoma is associated with poor patient prognosis. J Cancer Res Clin Oncol 2011;137:557-66. [Crossref] [PubMed]
- Zhao JY, Wang F, Li Y, et al. Five miRNAs Considered as Molecular Targets for Predicting Esophageal Cancer. Med Sci Monit 2015;21:3222-30. [Crossref] [PubMed]
- Wang Y, Xu M, Yang Q, et al. A six-microRNA signature predicts survival of patients with uterine corpus endometrial carcinoma. Curr Probl Cancer 2019;43:167-76. [Crossref] [PubMed]