Identifying pathological subtypes of non-small-cell lung cancer by using the radiomic features of 18F-fluorodeoxyglucose positron emission computed tomography
Introduction
Lung cancer (LC) is one of the most common malignancies and exhibits the highest morbidity and mortality rates worldwide (1). Non-small-cell lung cancer (NSCLC) comprises 85–90% of all LC and is characterized by three types, which was squamous cell carcinoma (SqCC), adenocarcinoma (ADC), and large-cell carcinoma. Furthermore, the first two types accounts for approximately 80% of all LCs (2,3). Histologic subtype represents tumor cells from different cellular origins that are known to have different genomic compositions and biological behaviors, and preclinical and subsequent clinical data have suggested histology-specific efficacy of chemotherapy in NSCLC. Therefore, it is significant to identify the histological subtype of NSCLC to determine the treatment and therapeutic strategies.
In conventional clinical practice, analyzing the tumor tissues obtained via puncture biopsies was commonly used to classify tumor histology (3,4). However, pathological specimens cannot be obtained from patients who cannot undergo surgery, and random samples of tumor tissues acquired through invasive puncture biopsy for molecular characterization may not accurately reflect the overall characteristics of tumor. Considered one of the fundamental building blocks of clinical oncology, medical imaging play vital roles in cancer staging, treatment planning, and response monitoring, especially in identifying tumor subtypes (5). One of the disadvantages of imaging examination is closely related to the experience of the physician. Thus, automatic, noninvasive, cost-effective, and reproducible alternatives that are unaffected by individual variability are desired.
Radiomics provides promising opportunities in cancer diagnosis, endowing medical imaging with an increasingly important role in analyzing tumor phenotypes. It uses high-throughput extraction of advanced quantitative features to objectively and quantitatively describe tumor phenotypes (6). Moreover, this technique has great potential for capturing important phenotypic information, such as intratumor heterogeneity, which will provide valuable information for personalized therapy.
In the past decade, positron emission computed tomography (PET) has become a routine method for evaluating solid tumors. A series of publications have reported PET radiomic features used in tumor therapy (7-9). PET imaging can detect functional changes before they become morphologically evident on computed tomography (CT) imaging. Differences between histological subtypes have also been discovered in the expression of glycolysis- and hypoxia-related markers, thus indicating histology-specific glucose metabolism in NSCLC (10-12). Recent advancements in NSCLC therapy have been characterized by the found of targeted mutations and histology-based treatments (13,14). PET, which does not rely on dimensional criteria, is more accurate than conventional imaging for the assessment pathological subtypes. Consequently, we developed and validated an optimal 18F-FDG PET radiomic model to distinguish between ADC and SqCC in the present study.
Methods
Patient cohorts
One hundred NSCLC patients (mean age 62 years, range 26–85 years) with pathologically confirmed ADC (n=61) or SqCC (n=39), who underwent 18F-FDG PET examination before treatment at Shandong Cancer Hospital from October 2014 to May 2017, were enrolled. According to the World Health Organization (WHO) classification of malignant lung tumors, the tumors were categorize as ADC or SqCC based on hematoxylin and eosin (HE) staining. Patients with two or more lesions in one or both lungs and those with the presence of other tumors and metastatic lesions were excluded from this study. The primary tumors were all solid tumor, and necrosis in the lesion was excluded from this study. This work was a retrospective study and was approved by the ethics committee at Shandong Cancer Hospital and Institute.
18F-FDG PET/CT scanning and gross tumor volume (GTV) delineation
18F-FDG PET images were obtained using a Philips Gemini TF PET/CT system (Phillips Medical Systems, Holland). The patients fasted for more than 6 hours, and their blood glucose was measured to ensure a level of <140 mg/dL. Patients were intravenously injected with 18F-FDG at 4.4 MBq/kg, and chest CT and PET scans were performed 1 hour later. All images were acquired with the respiratory gating technique. PET images were attenuated, corrected, reconstructed in multiple layers and imported into MIM Maestro version 6.8.2 (MIM software, Cleveland, OH). The GTV was delineated according to the metabolic information of the PET images and the anatomic information of the CT images. The target images were then reviewed by two senior radiologists, and differences in the findings were resolved by consensus.
Feature extraction
In this study, radiomic features were extracted using Chang-Gung Image Texture Analysis (CGITA) software, which is based on MATLAB (Mathworks, Natick, MA, USA) version 8.3 (15,16). In total, we extracted 107 quantitative features (comprising 59 texture features, 45 SUV statistic features and 5 shape features), and we provided the definitions and interpretation of these features in the supplement file. Then, we added six clinical features (smoking, age, sex, tumor size, T stage and N stage) for further feature selection. The longest dimension of the primary tumor was defined as the tumor size.
Statistical analysis
Statistical data was performed using SPSS 19.0 and R software (version 3.3.0, http://www.R-project.org). Based on the least absolute shrinkage and selection operator (LASSO) method, the “glmnet” software package reduces feature dimensionality and selects features with discriminative significance. P values <0.05 were considered statistically significant. The flow chart of this study is depicted in Figure 1.
Results
Patient characteristics
One hundred patients who met the inclusion criteria were divided into two independent groups; 74 patients treated between October 2014 and June 2016 constituted the training cohort, and 26 patients treated between July 2016 and May 2017 constituted the validation cohort. The characteristics of the patients in both the training and validation cohorts are displayed in Table 1. In the training cohort, ADC patients comprised 61% (45/74) of the cases and SqCC patients comprised 39% (29/74) of the cases. Male patients and female patients accounted for 61% (45/74) and 39% (29/74) in the training cohort, respectively. Smokers comprised 53% (39/74) of the patients, and non-smokers comprised 47% (35/74) of the patients. In the validation cohort, ADC patients comprised 62% (16/26) of the cases; SqCC patients comprised 38% (10/26) of the cases. Male patients and female patients accounted for 81% (21/26) and 19% (5/26) in the validation cohort, respectively. Smokers accounted for 65% (17/26) of the patients, whereas non-smokers accounted for 35% (9/26) of the patients.
Table 1
Characteristics | Training cohort | Validation cohort | |||||
---|---|---|---|---|---|---|---|
ADC | SqCC | P | ADC | SqCC | P | ||
Number (n) | 45 | 29 | 16 | 10 | 0.002 | ||
Age, mean ± SD (years) | 53.9±10.2 | 64.1±6.7 | 0.363 | 65.6±10.2 | 60.3±7.8 | 0.341 | |
Gender (n) | 0.025 | 0.317 | |||||
Male | 25 (56%) | 20 (69%) | 12 (75%) | 9 (90%) | |||
Female | 20 (44%) | 9 (31%) | 4 (25%) | 1 (10%) | |||
Smoking (n) | 0.003 | 0.317 | |||||
Yes | 15 (33%) | 24 (83%) | 9 (56%) | 8 (80%) | |||
No | 30 (67%) | 5 (17%) | 7 (44%) | 2 (20%) | |||
T stage (n) | <0.001 | 0.011 | |||||
T1 | 7 | 4 | 4 | 1 | |||
T2 | 24 | 12 | 9 | 5 | |||
T3 | 8 | 8 | 3 | 3 | |||
T4 | 6 | 5 | 0 | 1 | |||
N stage (n) | <0.001 | 0.008 | |||||
N1 | 10 | 6 | 3 | 2 | |||
N2 | 8 | 4 | 5 | 2 | |||
N3 | 13 | 13 | 6 | 4 | |||
N4 | 14 | 6 | 2 | 2 | |||
Tumor size (n) | 0.010 | 0.046 | |||||
≤3 cm | 30 | 18 | 13 | 6 | |||
>3 cm | 15 | 11 | 3 | 4 |
The P value represents the univariate association between each of the clinical variables and NSCLC subtypes using the Wilcoxon rank-sum test. A P<0.05 indicates significance. ADC, adenocarcinoma; SqCC, squamous cell carcinoma; SD, standard deviation; NSCLC, non-small-cell lung cancer.
Radiomic feature selection
Based on the elastic-net method in the training cohort, we chose the features with non-zero coefficients. The result showed that 5 radiomic features were selected from 107 features, which included short-zone emphasis, high-intensity short-run, code entropy, surface SUV NSR 3, and entropy. Figure 2 illustrates the parameter tuning procedure for the regression model and feature space reduction, and Table 2 lists the name and description of the selected features. The paired t-test results showed that the five radiomic features selected by the LASSO model differed significantly between lung ADC and lung SqCC patients (P<0.05). In the univariate analysis, the short-zone emphasis of gray-level features showed the best discriminative performance, and the area under the curve (AUC) reached 0.754. The texture parameter, code entropy, was used as the predictor, and its sensitivity reached 0.897, which was higher than those of the other predictors. The metabolic parameter surface SUV NSR 3 had the highest specificity of 0.821. In Figure 3, we depict the features selected to develop the radiomic model in ADC and SqCC.

Table 2
Feature name | Mean ± SD | Weighted | AUC | SEN | SPE | P value | |
---|---|---|---|---|---|---|---|
ADC | SqCC | ||||||
Short-zone emphasis | 0.832±0.070 | 0.764±0.067 | 4.65 | 0.754 | 0.821 | 0.639 | 0.020 |
High-intensity short-run | 1,048.320±463.592 | 709.554±379.995 | 0.01 | 0.717 | 0.639 | 0.769 | 0.009 |
Code entropy | 3.000±0.331 | 3.313±0.314 | −0.05 | 0.753 | 0.897 | 0.557 | 0.001 |
Surface SUV NSR 3 | 15.224±7.828 | 21.180±9.257 | −0.09 | 0.690 | 0.508 | 0.821 | 0.023 |
Entropy | −0.919±0.599 | −1.500±0.719 | −0.38 | 0.744 | 0.718 | 0.738 | 0.020 |
SD, standard deviation; ADC, adenocarcinoma; SqCC, squamous cell carcinoma; AUC, area under the curve; SEN, sensitivity; SPE, specificity.
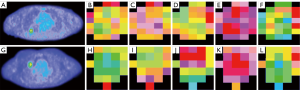
Development of the radiomic model and receiver operating characteristic (ROC) curve analysis
The ROC curves showed good performance and generalization for the model built using radiomic features. The AUCs for the radiomic model were 0.803 in the training cohort and 0.700 in the validation cohort (Figure 4). The result revealed that the sensitivity, specificity, positive predictive value, negative predictive value and accuracy of the radiomic model in the validation cohort were 0.938, 0.600, 0.789, 0.857, and 0.808, respectively (Table 3).
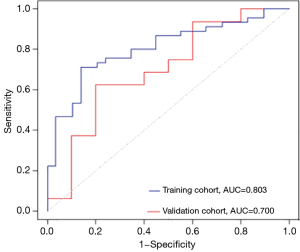
Table 3
Type | Group | Sensitivity | Specificity | PPV | NPV | Accuracy | AUC |
---|---|---|---|---|---|---|---|
Radiomic features | Training group | 0.889 (40/45) | 0.586 (17/29) | 0.769 (40/52) | 0.773 (17/22) | 0.770 (57/74) | 0.804 |
Validation group | 0.938 (15/16) | 0.600 (6/10) | 0.789 (15/19) | 0.857 (6/7) | 0.808 (21/26) | 0.700 | |
Total | 0.902 (55/61) | 0.590 (23/39) | 0.775 (55/71) | 0.793 (23/29) | 0.780 (78/100) | – |
PPV, positive predictive value; NPV, negative predictive value; AUC, area under the curve.
Development of the clinical model and ROC curve analysis
A clinical features classification model was built by multivariable logistic regression analysis with smoking status, sex, age, tumor size, T stage and N stage. The performance of the clinical model for the classification NSCLC subtypes presented by ROC curves is depicted in Figure 5, which yielded AUCs of 0.702 in the training dataset and 0.728 in the validation dataset. Compared with the radiomic model, the specificity reached from 0.600 to 1.000 in the validation group. However, the sensitivity, positive predictive value, negative predictive value and accuracy were reduced in the clinical validation dataset. Similarly, we describe these indicators in Table 4.
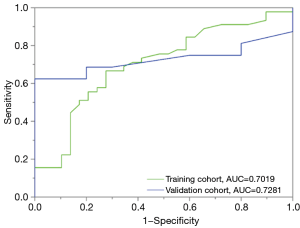
Table 4
Type | Group | Sensitivity | Specificity | PPV | NPV | Accuracy | AUC |
---|---|---|---|---|---|---|---|
Clinical features | Training group | 0.667 (30/45) | 0.724 (21/29) | 0.789 (30/38) | 0.583 (21/36) | 0.689 (51/74) | 0.702 |
Validation group | 0.625 (10/16) | 1.000 (10/10) | 1.000 (10/10) | 0.625 (10/16) | 0.769 (20/26) | 0.728 | |
Total | 0.656 (40/61) | 0.795 (31/39) | 0.816 (40/49) | 0.608 (31/51) | 0.710 (71/100) | – |
PPV, positive predictive value; NPV, negative predictive value; AUC, area under the curve.
Development of the radiomic features combined with the clinical features model and ROC curve analysis
Multivariable logistic regression analysis identified smoking status and five radiomic features as independent predictors. For the model built with radiomic features and clinical variables, the AUCs were 0.822 in the training cohort and 0.781 in the validation cohort, which showed better performance for differentiating ADC and SqCC than the models incorporating radiomic features or clinical variables only (Figure 6). The performance of the combined model (sensitivity 1.000, specificity 0.700, accuracy 0.885) in the validation cohort is described in Table 5.
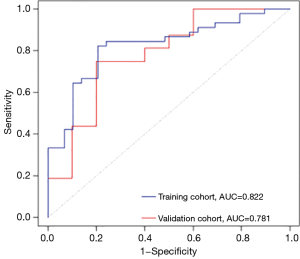
Table 5
Type | Group | Sensitivity | Specificity | PPV | NPV | Accuracy | AUC |
---|---|---|---|---|---|---|---|
Radiomic features & clinical features | Training group | 0.889 (40/45) | 0.690 (20/29) | 0.816 (40/49) | 0.800 (20/25) | 0.811 (60/74) | 0.822 |
Validation group | 1.000 (16/16) | 0.700 (7/10) | 0.842 (16/19) | 1.000 (7/7) | 0.885 (23/26) | 0.781 | |
Total | 0.918 (56/61) | 0.692 (27/39) | 0.824 (56/68) | 0.844 (27/32) | 0.830 (83/100) | – |
PPV, positive predictive value; NPV, negative predictive value; AUC, area under the curve.
Discussion
Identifying tumor subtypes has an important impact on patients with initially diagnosed NSCLC to select the most appropriate therapeutic option. Studies have confirmed the effectiveness of immunotherapy due to the benefit of overall survival for cancer patients (17). Lung ADC and lung SqCC have distinctly different oncogenic mutations and divergent therapeutic responses, especially for mutations in EGFR and PD1-targeted antibody status which drive the heightened emphasis on accurate pathological NSCLC subtyping (18).
Currently, histological and cytological examinations are the two main methods for determining NSCLC subtypes. However, 70% to 80% of NSCLC patients are diagnosed at an advanced stage, and pathological specimens cannot be obtained surgically. Consequently, puncture biopsy is the most commonly used pathological test for LC. However, puncture biopsies are susceptible to lesion size, wall thickness, and location, which may affect diagnostic accuracy. Priola et al. (19) analyzed clinical data from 612 patients who underwent CT-guided biopsies to confirm the diagnosis of NSCLC, and compared to the surgical pathology results, the pathological type determined by puncture was only 83.3% accurate. Moreover, qualitative detection methods are subjectively influenced by the observer. Steinfort et al. (20) collected specimens from 60 patients who underwent endobronchial ultrasound (EBUS)-guided transbronchial needle aspiration (TBNA) for the diagnosis/staging of suspected/known NSCLC, and three pathologists with more than 10 years of diagnostic experience independently reviewed the specimens. The agreement in determining NSCLC subtype by smear, H&E staining and immunohistochemistry (IHC) of the specimens was slight (κ=50.095, 95% CI: 0.164–0.355), fair (κ=50.278, 95% CI: 0.075–0.481) and moderate (κ=50.564, 95% CI: 0.338–0.740), respectively, which may have occurred because some clinical features are defined by pathologists whose evaluation standards may differ based on subjective experiential judgment. The observer variability of many diagnostic decisions related to the characteristics of pulmonary lesions also affects the sensitivity of diagnosis. Therefore, clinicians are constantly seeking better methods for making an accurate diagnosis.
Radiomics has recently become an important auxiliary tool for precision medicine, and several studies have reported an association between radiomic features and tumor pathophysiological properties based on CT and magnetic resonance imaging (MRI) (21,22). As a functional imaging method, 18F-FDG PET indicates the specific distribution of 18F-FDG uptake by tissues, and 18F-FDG PET images have important clinical value in differentiating benign and malignant tumors, confirming pathological subtypes, clinical staging, evaluating efficacy, monitoring recurrence and metastasis, and searching for primary tumors (23-25). The pathological subtypes of LC are complex, and the pathogenesis of NSCLC subtypes differ significantly, as reflected by PET images (26). Medical imaging enables the noninvasive assessment of tissue characteristics and is therefore routinely used to diagnose and treat tumors. Furthermore, determining the relationship between pretreatment NSCLC images and histological subtypes has great implications in choosing clinical treatment options.
This study explored the potential of pretreatment 18F-FDG PET radiomic features to identify NSCLC subtypes. The results showed that the sensitivity and accuracy in the validation model were 0.938 and 0.808, respectively. These results might be attributed to the fact that texture analysis can indirectly capture the microscopic features of these lesions, which was completely different from macroscopic morphological characteristics. These pathological features cannot be determined by visual examination of the tissue images, however, they might be ascertained by inspecting the changes in the arrangement of pixels via a texture analysis of medical images (27,28). When clinical features were integrated, the sensitivity and accuracy of the validation model reached 1 and 0.885, respectively. One reason is that SqCC is mainly associated with a smoking history and tends to form large tumors in the center of the lung. In the present study, the accuracy of the radiomic model is superior to the puncture results, and the method proposed herein has the advantages of being noninvasive and reproducible and reflects the overall tumor information. Thus, the radiomic method is an improvement over the puncture biopsy results.
The texture features can quantify the arrangement of tumor cells in different pathological types, which were related to heterogeneity in tumor gene expression and biological behavior. Our study provides a new method for differentiating NSCLC pathological types using 18F-FDG PET images. Radiomic feature analysis is a new image postprocessing technique that may play an important role in describing tumor characteristics (29,30). One limitation of the present study was that we did not evaluate radiomic features of lymph nodes in this study due to feature stability and reproducibility. Another limitation is that to acquire a reliable result, the noninvasive identification of NSCLC pathological subtypes using imaging biomarker should involve sufficient patient data. As an emerging research field of medical image processing, radiomic research requires a large amount of data for confirmation. With multicenter cohort expansion and prospective studies, we expect higher accuracy and stability of subtype classification in future works.
Conclusions
Our study reveals that a model including radiomic features combined with clinical features is superior to models that contain either radiomic features or clinical features alone. Therefore, it is a promising and applicable alternative approach for identifying subtypes of NSCLC, which may serve as a complementary tool to help doctors make clinical decisions.
Supplementary
Gray level co-occurrence matrix (GLCM) features
For GLCM features, they describe the joint distribution of two pixels with a certain spatial position relationship, which can be regarded as a joint histogram of two pixel gray pairs, and belong to the second order statistics.
1. Angular Second Moment (ASM):
2. Contrast:
3. Entropy:
4. Dissimilarity:
5. Inverse difference moment (IDM):
6. Correlation:
Normalized gray level co-occurrence matrix (NGLCM) features
For NGLCM features, they were the normalized version of the GLCM features.
- Angular second moment;
- Contrast;
- Entropy;
- Dissimilarity;
- Inverse difference moment;
- Correlation.
Voxel-alignment features
The voxel-alignment features were computed with the length of voxels that have the same gray intensity. They were only the measurement and statistics of image pixel information. In the process of practical use, it is necessary to calculate the generated grayscale run-length matrix and get the image feature information based on grayscale co-occurrence matrix.
1. Short run emphasis (SRE):
2. Long run emphasis (LRE):
3. Intensity variability (IV):
4. Run-length variability (RLV):
5. Run percentage (RP):
6. Low-intensity run emphasis (LIRE):
7. High-intensity run emphasis (HIRE):
8. Low-intensity short-run emphasis (LISRE):
9. High-intensity short-run emphasis (HISRE):
10. Low-intensity long-run emphasis (LILRE):
11. High-intensity long-run emphasis (HILRE):
Intensity size zone matrix (ISZM) features
ISZM features were defined as the numbers of neighbor voxels with same gray value, and it shows remarkable effect on texture consistency and non-periodicity.
1. Short-zone emphasis (SZE):
2. Large-zone emphasis (LZE):
3. Intensity variance (IV):
Here, .
4. Size-zone variability (SZV):
5. Zone percentage (ZP):
6. Low-intensity zone emphasis (LIZE):
7. High-intensity zone emphasis (HIZE):
8. Low-intensity short-zone emphasis (LISZE):
9. High-intensity short-zone emphasis (HISZE):
10. Low-intensity large-zone emphasis (LILZE):
11. High-intensity large-zone emphasis (HILZE):
Neighborhood intensity-difference matrix (NIDM) features
The indices measured the sum of average difference between a pixel value and the pixel value of its neighbors with a specific distance.
1. Coarseness:
2. Contrast:
3. Busyness:
4. Complexity:
5. Strength:
Neighboring gray level dependence (NGLD) features
GLDM features quantify the intensity dependency which was defined as the number of connected voxels within specific distance that are dependent on the center voxel.
1. Small dependence emphasis (SDE):
2. Large dependence emphasis (LDE):
3. Dependence non-uniformity (DN):
4. Dependence entropy (DE):
5. Gray level non-uniformity (GLN):
SUV statistics
The standardized uptake value (SUV) refer to the ratio of the image derived radioactivity concentration and the whole body concentration of the injected radioactivity, particularly common in the analysis of [18F]fluorodeoxyglucose {[18F]FDG} images of cancer patients.
1. SUVmin: The minimal standardized uptake value of ROI.
2. SUVmax: The maximal standardized uptake value of ROI.
3. SUVmax_prod_asphericity
4. SUVmax_prod_surface_area
5. SUVmean: The mean standardized uptake value of ROI.
6. SUVmean_prod_asphericity
7. SUVmean_prod_surface_area
8. SUV variance:
9. Surface SUV variance 1
10. Surface SUV variance 2
11. Surface SUV variance 3
12. Surface SUV variance 4
13. SUV standard deviation (SD)
14. Surface SUV SD 1
15. Surface SUV SD 2
16. Surface SUV SD 3
17. Surface SUV SD 4
18. SUV skewness
19. SUV bias-corrected skewness
20. SUV kurtosis:
21. SUV bias-corrected kurtosis
22. Total lesion glycolysis (TLG):
TLG=MTV* SUVmean
23. Tumor volume
24. Entropy
25. Entropy_prod_asphericity
26. Entropy_prod_surface_area
27. Surface SUV entropy 1
28. Surface SUV entropy 2
29. Surface SUV entropy 3
30. Surface SUV entropy 4
31. SULpeak: It was defined as the highest SUVmean of a 1 cm3 spherical lesion/target.
32. SULpeak_prod_asphericity
33. SULpeak_prod_surface_area
34. Surface area
35. Surface total SUV 1
36. Surface total SUV 2
37. Surface total SUV 3
38. Surface total SUV 4
39. Surface mean SUV 1
40. Surface mean SUV 2
41. Surface mean SUV 3
42. Surface mean SUV 4
43. Surface SUV NSR 1
44. Surface SUV NSR 2
45. Surface SUV NSR 3
46. Surface SUV NSR 4
47. Perimeter
48. Asphericity 2D
49. Asphericity 3D
Texture spectrum features
1. Max spectrum
2. Black-white symmetry
Texture coding features
1. Coarseness
2. Homogeneity
3. Mean convergence
4. Variance
Texture coding co-occurance features
1. Second angular moment (SAM)
2. Contrast
3. Entropy
4. Homogeneity
5. Intensity
6. Inverse difference moment (IDM)
7. Code entropy
8. Code similarity
Supplementary
Acknowledgments
Funding: This work was supported by
Footnote
Conflicts of Interest: All authors have completed the ICMJE uniform disclosure form (available at http://dx.doi.org/10.21037/tcr.2019.08.20). The authors have no conflicts of interest to declare.
Ethical Statement: The authors are accountable for all aspects of the work in ensuring that questions related to the accuracy or integrity of any part of the work are appropriately investigated and resolved. The study was conducted in accordance with the Declaration of Helsinki (as revised in 2013). This retrospective study was approved by the Institutional Review Board of Shandong Cancer Hospital and Institute (No. 201903021). Medical record review was performed in accordance with Institutional Ethics Review Board guidelines.
Open Access Statement: This is an Open Access article distributed in accordance with the Creative Commons Attribution-NonCommercial-NoDerivs 4.0 International License (CC BY-NC-ND 4.0), which permits the non-commercial replication and distribution of the article with the strict proviso that no changes or edits are made and the original work is properly cited (including links to both the formal publication through the relevant DOI and the license). See: https://creativecommons.org/licenses/by-nc-nd/4.0/.
References
- Torre LA, Bray F, Siegel RL, et al. Global cancer statistics, 2012. CA Cancer J Clin 2015;65:87-108. [Crossref] [PubMed]
- Risch A, Plass C. Lung cancer epigenetics and genetics. Int J Cancer 2008;123:1-7. [Crossref] [PubMed]
- West H, Harpole D, Travis W. Histologic considerations for individualized systemic therapy approaches for the management of non-small cell lung cancer. Chest 2009;136:1112-8. [Crossref] [PubMed]
- Travis WD. Classification of lung cancer. Semin Roentgenol 2011;46:178-86. [Crossref] [PubMed]
- Qiu Q, Duan J, Gong G, et al. Reproducibility of radiomic features with GrowCut and GraphCut semiautomatic tumor segmentation in hepatocellular carcinoma. Transl Cancer Res 2017;6:940-8. [Crossref]
- Yip SS, Aerts HJ. Applications and limitations of radiomics. Phys Med Biol 2016;61:R150-66. [Crossref] [PubMed]
- Scrivener M, de Jong EE, van Timmeren JE, et al. Radiomics applied to lung cancer: a review. Transl Cancer Res 2016;5:398-409. [Crossref]
- Kirienko M, Cozzi L, Antunovic L, et al. Prediction of disease-free survival by the PET/CT radiomic signature in non-small cell lung cancer patients undergoing surgery. Eur J Nucl Med Mol Imaging 2018;45:207-17. [Crossref] [PubMed]
- Lee JW, Sang ML. Radiomics in Oncological PET/CT: Clinical Applications. Nucl Med Mol Imaging 2018;52:170-89. [Crossref] [PubMed]
- Rekhtman N, Ang DC, Sima CS, et al. Immunohistochemical algorithm for differentiation of lung adenocarcinoma and squamous cell carcinoma based on large series of whole-tissue sections with validation in small specimens. Mod Pathol 2011;24:1348-59. [Crossref] [PubMed]
- Schuurbiers OC, Meijer TW, Kaanders JH, et al. Glucose metabolism in NSCLC is histology-specific and diverges the prognostic potential of 18FDG-PET for adenocarcinoma and squamous cell carcinoma. J Thorac Oncol 2014;9:1485-93. [Crossref] [PubMed]
- Meijer TW, Schuurbiers OC, Kaanders JH, et al. Differences in metabolism between adeno- and squamous cell non-small cell lung carcinomas: spatial distribution and prognostic value of GLUT1 and MCT4. Lung Cancer 2012;76:316-23. [Crossref] [PubMed]
- Manegold C. Treatment algorithm in 2014 for advanced non-small cell lung cancer: therapy selection by tumour histology and molecular biology. Adv Med Sci 2014;59:308-13. [Crossref] [PubMed]
- Cufer T, Ovcaricek T, O’Brien ME. Systemic therapy of advanced non-small cell lung cancer: major-developments of the last 5-years. Eur J Cancer 2013;49:1216-25. [Crossref] [PubMed]
- Fang YH, Lin CY, Shih MJ, et al. Development and evaluation of an open-source software package ‘‘CGITA’’ for quantifying tumor heterogeneity with molecular images. Biomed Res Int 2014;2014:248505. [Crossref] [PubMed]
- Aerts H J, Velazquez E R, Leijenaar R T, et al. Decoding tumour phenotype by noninvasive imaging using a quantitative radiomics approach. Nat Commun 2014;5:4006. [Crossref] [PubMed]
- Davies M. New modalities of cancer treatment for NSCLC: focus on immunotherapy. Cancer Manag Res 2014;6:63-75. [Crossref] [PubMed]
- Zhang L, Chen B, Liu X, et al. Quantitative biomarkers for prediction of epidermal growth factor receptor mutation in non-small cell lung cancer. Transl Oncol 2018;11:94-101. [Crossref] [PubMed]
- Priola AM, Priola SM, Cataldi A, et al. Accuracy of CT-guided transthoracic needle biopsy of lung lesions: Factors affecting diagnostic yield. Radiol Med 2007;112:1142-59. [Crossref] [PubMed]
- Steinfort DP, Russell PA, Tsui A, et al. Interobserver agreement in determining non-small cell lung cancer subtype in specimens acquired by EBUS-TBNA. Eur Respir J 2012;40:699-705. [Crossref] [PubMed]
- Liu H, Wang XY, Long XY. Research progress and clinical application of tumor heterogeneity based on CT texture analysis. International Journal of Medical Radiology 2016;39:543-48.
- Li H, Zhu Y, Burnside ES, et al. Quantitative MRI radiomics in the prediction of molecular classifications of breast cancer subtypes in the TCGA/TCIA data set. NPJ Breast Cancer 2016;2:16012. [Crossref] [PubMed]
- Lee BE, von Haag D, Lown T, et al. Advances in positron emission tomography technology have increased the need for surgical staging in non-small cell lung cancer. J Thorac Cardiovasc Surg 2007;133:746-52. [Crossref] [PubMed]
- Perigaud C, Bridji B, Roussel JC, et al. Prospective preoperative mediastinal lymph node staging by integrated positron emission tomography computerised tomography in patients with non-small-cell lung cancer. Eur J Cardiothorac Surg 2009;36:731-6. [Crossref] [PubMed]
- Pyka T, Bundschuh RA, Andratschke N, et al. Textural features in pre-treatment [F18]-FDG-PET/CT are correlated with risk of local recurrence and disease-specific survival in early stage NSCLC patients receiving primary stereotactic radiation therapy. Radiat Oncol 2015;10:100. [Crossref] [PubMed]
- Ren H, Xu W, You J, et al. Analysis of the role of PET/CT SUVmax in prognosis and its correlation with clinicopathological characteristics in resectable lung squamous cell carcinoma. Zhongguo Fei Ai Za Zhi 2016;19:192-9. [PubMed]
- Yang F, Young LA, Johnson PB. Quantitative radiomics: Validating image textural features for oncological PET in lung cancer. Radiother Oncol 2018;129:209-17. [Crossref] [PubMed]
- Hatt M, Tixier F, Pierce L, et al. Characterization of PET/CT images using texture analysis: the past, the present… any future? Eur J Nucl Med Mol Imaging 2017;44:151-65. [Crossref] [PubMed]
- Court LE, Rao A, Krishnan S. Radiomics in cancer diagnosis, cancer staging, and prediction of response to treatment. Transl Cancer Res 2016;5:337-9. [Crossref]
- Acharya UR, Hagiwara Y, Sudarshan VK, et al. Precision medical imaging in big data: radiomics. Chin J Bases Clin General Surg 2016;752-5.