Bioinformatic analysis of long non-coding RNA-associated competing endogenous RNA network in adrenocortical carcinoma
Introduction
Adrenocortical carcinoma (ACC) is a malignant tumor that occurs in the adrenal cortex, with an annual incidence of 0.7–2.0 per million (1). Due to the high degree of aggressiveness and propensity of metastasis, ACC patients present advanced disease since the diagnosis and do not benefit from surgery. At present, the treatment of ACC includes surgery (2), mitotane (3), standard chemotherapy, including the scheme Etoposide, Doxorubicin and Cisplatin, or steptozotocin (4), or gemcitabine plus capecitabine (5), radiotherapy (6), and target therapies (7-11). However, a complete resection remains the most effective treatment (2).
Inoperable patients can choose adjuvant therapy such as cytotoxic drugs, and targeted drugs, but the current curative effect is unsatisfactory. Five-year survival is for tumors confined to the adrenal space 60–80%, 35–50% for locally advanced disease, and much lower in case of metastatic disease with reported percentages ranging from 0% to 28% (1). Although diagnostic techniques for adrenocortical carcinoma have improved greatly in recent decades, the exact pathogenesis of the disease is still not fully understood. A better understanding of the pathogenesis of ACC may help to develop new diagnostic, therapeutic, and preventive strategies.
In recent years, long-chain non-coding RNAs (lncRNAs) have received increasing attention, and are thought to participate in almost all steps of tumor progression including cell growth, survival, and metastasis (12). LncRNAs are RNAs containing more than 200 nucleotides, and collectively constitute at least 80% of the human genome. LncRNAs do not have protein coding capacity due to lack of an open reading frame (ORF), and can only regulate expression of genes in the form of RNA (13). LncRNAs have a wide range of biological functions, and can participate in regulation of gene expression through transcription, post-transcriptional translation, and epigenetics, thereby affecting apoptosis, cell cycle, cell proliferation, and differentiation (14). Many studies have confirmed that lncRNAs are involved in the development of tumors, such as breast cancer, lung cancer, ovarian cancer, colorectal cancer, and lymphoid malignancies (15-19).
In recent years, research has focused on regulatory mechanisms of lncRNA-miRNA-mRNA, or so-called competing endogenous RNA (ceRNA). In 2011, researchers at Harvard University proposed a competitive endogenous RNA hypothesis. The hypothesis states that lncRNAs regulate other RNA transcripts by competing for shared miRNAs, affecting tumorigenesis and development (20). Many studies have confirmed this view. For example, in esophageal squamous cell carcinoma, lncRNA MALAT1 interacts with miR-101 and miR-217 to upregulate the downstream genes p21, p27, and B-MYB, then blocking G2/M phase cells and inhibiting tumor cell proliferation (21). LncRNA UCA1 can competitively bind to miR-204, which in turn affects the expression of SOX4 and ultimately promotes tumor cell proliferation (22). However, to date, a large-scale sequencing database to investigate the regulatory mechanisms of specific lncRNAs and lncRNA-mediated ceRNA network in adrenocortical carcinoma remains lacking.
In the present study, we successfully constructed the lncRNA-miRNA-mRNA (ceRNA) network in ACC using the lncRNA expression profiles between 10 ACC samples and 6 normal tissue samples obtained from the Gene Expression Omnibus (GEO) database, and then conducted a functional enrichment analysis of mRNAs in the ceRNA network to reveal their underlying mechanisms. Finally, we analyzed the relationship between lncRNAs in the ceRNA network and the prognosis of patients with ACC.
Methods
Data acquisition and processing
GEO (23) is a public functional genomics data repository of array- and sequence-based data. The GSE61359 dataset (24) was downloaded from GEO [Affymetrix GPL16956 platform, Agilent-045997 Arraystar human lncRNA microarray V3 (Probe Name Version)]. The GSE61359 dataset contained 10 ACC samples and 6 normal adrenal cortex samples. TCGA is a database of clinical information and clinical pathology information collected from approximately 11,000 cancer patient samples. The clinical data for 79 ACC patients were downloaded from the TCGA Database (25). This study was conducted according to the publication guidelines provided by the GEO and TCGA; therefore, further ethics committee approval was not required.
Identification of differentially expressed RNAs
We identified differentially expressed lncRNAs and mRNAs between ACC samples and normal adrenal cortex samples using the “limma” packages in R. |Log2 fold change (FC)| >1 and false discovery rate adjusted to P<0.05 were defined as the thresholds. In addition, differentially expressed RNAs were depicted in the heat map by the limma package in R.
Construction of the ceRNA network
Construction of the ceRNA network included two steps: (I) lncRNA-miRNA interactions were predicted by miRcode (http://www.mircode.org/); (II) the miRNA-targeted mRNAs were retrieved from miRDB, miRTarBase, and TargetScan databases (26-28). Using the above data, we then built and visualized the lncRNA-miRNA-mRNA network using Cytoscape 3.7.0 (29).
Functional enrichment analysis
In order to fully investigate the specific functions of genes in the ceRNA network, we performed Gene Ontology (GO) and Kyoto Encyclopedia of Genes and Genomes (KEGG) pathway analysis of mRNAs in the ceRNA network by using the clusterProfiler package of R. P value <0.05 and q values <0.05 were considered statistically significant and representative of statistically significant enrichment. The bubble chart of the GO and KEGG analysis results was implemented using the clusterProfiler package in R language.
Establishment of protein-protein interaction (PPI) network
Analysis of the interaction between genes may help us better understand the underlying mechanisms of ACC disease. We built a PPI network using the STRING (https://string-db.org/cgi/input.pl) online tool and visualized it using Cytoscape 3.7.0. Then, the hub genes were identified using the cytoHubba app of Cytoscape.
Survival analysis
To evaluate the relationship of lncRNAs in the ceRNA network to prognosis, we performed survival analysis using the combined clinical data of ACC patients in TCGA. R package “survival” was used to implement log-rank tests and plot Kaplan-Meier survival curves. P value <0.001 was regarded as the threshold.
Results
Identification of differentially expressed lncRNAs (DElncRNAs) and mRNAs (DEmRNAs) between ACC and normal adrenal glands
Using logFC >1 and P<0.05 as thresholds, we used the “limma” package in R to identify DElncRNAs and DEmRNAs between adrenocortical carcinoma samples and normal control samples. A total of 391 differentially expressed lncRNAs and 1,313 differentially expressed mRNAs were identified. 265 DElncRNAs were upregulated and 126 were downregulated. Five hundred and eleven DEmRNAs were upregulated and 802 were downregulated. All differentially expressed lncRNAs and differentially expressed mRNAs are shown in the heat map of Figure 1 (http://fp.amegroups.cn/cms/24f41f127c4a0fc425131c0741e158a8/tcr.2019.09.34-1.pdf; http://fp.amegroups.cn/cms/f7cf804a1bfdb64ddc0d64a166ce5335/tcr.2019.09.34-2.pdf.
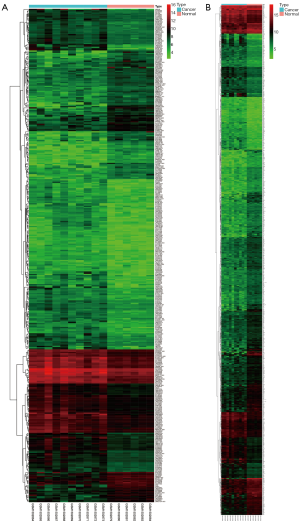
Building a ceRNA network
In order to further understand how lncRNA affects mRNA and miRNA binding in adrenocortical carcinoma, we constructed and visualized the lncRNA-miRNA-mRNA (ceRNA) network using Cytoscape v3.7.0 software. First, we used the 391 DElncRNAs to obtain 3,277 pairs of interacting lncRNA-miRNAs (including 94 lncRNAs and 207 miRNAs) by using the miRcode database and applying the Perl program. After that, we used the 207 miRNAs previously aligned to predicted target mRNAs from miRDB, miRTarBase, and TargetScan databases. One thousand nine hundred thirty-five miRNA-mRNA pairs (including 42 miRNAs and 1,269 mRNAs) were predicted by all three databases. The 1,269 mRNAs predicted by miRNA targeting were compared with the 1,313 DEmRNAs, and mRNAs not included among the DEmRNAs were excluded to obtain 78 mRNAs. Altogether, we identified 87 lncRNAs, 31 miRNAs, and 78 mRNA nodes as differential expression profiles in the ceRNA network (Figure 2). Then, we identified the top 5 lncRNAs and miRNAs with highest degree score from the ceRNA network. Five lncRNAs include: AL022344, AL592494, DLX6-AS1, AC131025, and AC015818.
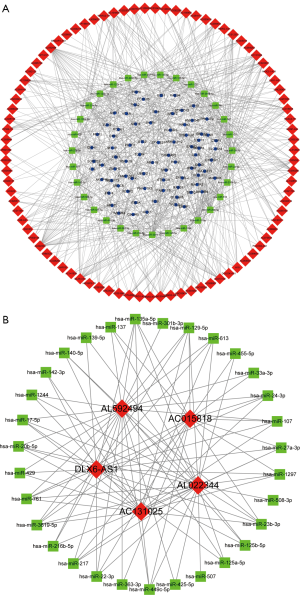
Prediction of mRNA function in the ceRNA network
LncRNAs are typically the center of the ceRNA network and are linked to one or more mRNAs. For a better understanding of the role of lncRNAs, we performed a GO and KEGG enrichment analysis on the 78 mRNAs in the ceRNA network to infer the role of each lncRNA. Using P<0.05 and q <0.05 as thresholds, we identified 9 significantly enriched GO terms (Figure 3 and Table 1). The most prominent GO terms included transcription factor activity, RNA polymerase II proximal promoter sequence-specific DNA binding, and RNA polymerase II proximal promoter sequence-specific DNA binding. Similarly, using P<0.05 and q <0.05 as thresholds, 21 significantly enriched pathways were obtained from the KEGG pathway analysis (Figure 4 and Table 2). The most significant enrichments included MAPK signaling pathway, microRNAs in cancer, and human cytomegalovirus infection.
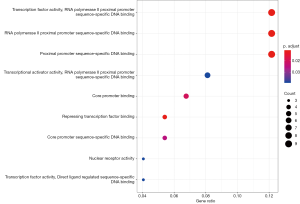
Table 1
ID | Description | P value | q value | Gene symbols |
---|---|---|---|---|
GO:0000982 | Transcription factor activity, RNA polymerase II proximal promoter sequence-specific DNA binding | 6.02E-05 | 0.01001 | FOS/STAT3/BTG2/IRF4/ZNF217/SIRT1/ETS1/ARNTL/MYC |
GO:0000978 | RNA polymerase II proximal promoter sequence-specific DNA binding | 8.79E-05 | 0.01001 | FOS/STAT3/IRF4/EZH2/ZNF217/ETS1/NR1H3/ARNTL/MYC |
GO:0000987 | Proximal promoter sequence-specific DNA binding | 0.000114 | 0.01001 | FOS/STAT3/IRF4/EZH2/ZNF217/ETS1/NR1H3/ARNTL/MYC |
GO:0070491 | Repressing transcription factor binding | 0.000153 | 0.010097 | STAT3/ARNTL/MYC/CTBP1 |
GO:0001047 | Core promoter binding | 0.000333 | 0.020093 | FOS/EZH2/SFPQ/SIRT1/ARNTL |
GO:0001046 | Core promoter sequence-specific DNA binding | 0.000498 | 0.021921 | FOS/EZH2/SIRT1/ARNTL |
GO:0001077 | Transcriptional activator activity, RNA polymerase II proximal promoter sequence-specific DNA binding | 0.001147 | 0.033874 | FOS/STAT3/IRF4/SIRT1/ETS1/MYC |
GO:0004879 | Nuclear receptor activity | 0.001154 | 0.033874 | STAT3/NR1H3/NR2F2 |
GO:0098531 | Transcription factor activity, direct ligand regulated sequence-specific DNA binding | 0.001154 | 0.033874 | STAT3/NR1H3/NR2F2 |
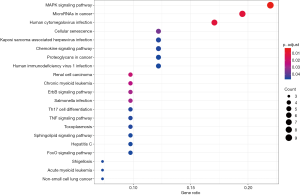
Table 2
ID | Description | P value | q value | Gene symbols |
---|---|---|---|---|
hsa04010 | MAPK signaling pathway | 2.38E-05 | 0.002975 | FOS/MAPK1/CRKL/MAP3K5/ERBB3/MYC/MAP3K8/GNG12/HSPA1B |
hsa05206 | MicroRNAs in cancer | 0.000181 | 0.008028 | STAT3/MAPK1/CRKL/EZH2/ERBB3/SIRT1/ROCK1/MYC |
hsa05163 | Human cytomegalovirus infection | 0.000192 | 0.008028 | STAT3/MAPK1/CRKL/TMEM173/ROCK1/MYC/GNG12 |
hsa05211 | Renal cell carcinoma | 0.000522 | 0.016344 | EGLN3/MAPK1/CRKL/ETS1 |
hsa05220 | Chronic myeloid leukemia | 0.000753 | 0.018874 | MAPK1/CRKL/MYC/CTBP1 |
hsa04012 | ErbB signaling pathway | 0.001147 | 0.02145 | MAPK1/CRKL/ERBB3/MYC |
hsa05132 | Salmonella infection | 0.001199 | 0.02145 | FOS/MAPK1/DYNC1LI2/ROCK1 |
hsa04218 | Cellular senescence | 0.001702 | 0.026651 | MAPK1/ZFP36L2/SIRT1/ETS1/MYC |
hsa04659 | Th17 cell differentiation | 0.002681 | 0.0339 | FOS/STAT3/IRF4/MAPK1 |
hsa04668 | TNF signaling pathway | 0.002964 | 0.0339 | FOS/MAPK1/MAP3K5/MAP3K8 |
hsa05145 | Toxoplasmosis | 0.003267 | 0.0339 | LDLR/STAT3/MAPK1/HSPA1B |
hsa05167 | Kaposi sarcoma-associated herpesvirus infection | 0.003284 | 0.0339 | FOS/STAT3/MAPK1/MYC/GNG12 |
hsa04062 | Chemokine signaling pathway | 0.003518 | 0.0339 | STAT3/MAPK1/CRKL/ROCK1/GNG12 |
hsa04071 | Sphingolipid signaling pathway | 0.003818 | 0.034157 | MAPK1/MAP3K5/S1PR1/ROCK1 |
hsa05205 | Proteoglycans in cancer | 0.004576 | 0.034176 | STAT3/MAPK1/ERBB3/ROCK1/MYC |
hsa05131 | Shigellosis | 0.005296 | 0.034176 | MAPK1/CRKL/ROCK1 |
hsa05221 | Acute myeloid leukemia | 0.005527 | 0.034176 | STAT3/MAPK1/MYC |
hsa05223 | Non-small cell lung cancer | 0.005527 | 0.034176 | STAT3/MAPK1/EML4 |
hsa05160 | Hepatitis C | 0.005538 | 0.034176 | LDLR/STAT3/MAPK1/NR1H3 |
hsa04068 | FoxO signaling pathway | 0.005688 | 0.034176 | STAT3/MAPK1/S1PR1/SIRT1 |
hsa05170 | Human immunodeficiency virus 1 infection | 0.00573 | 0.034176 | FOS/MAPK1/CRKL/TMEM173/GNG12 |
Construction of PPI network
To identify the possible key genes that may play a central role in the development of ACC, we used the STRING online tool and Cytoscape v3.7.0 software to construct a PPI network consisting of 34 mRNAs with a median confidence score of >0.40. Then, we then used the cytoHubba app to identify the top 10 key genes from the PPI network. These key genes include: MYC, FOS, MAPK1, STAT3, ERBB3, SIRT1, ETS1, ARNTL, EZH2, and MAP3K5 (Figure 5).
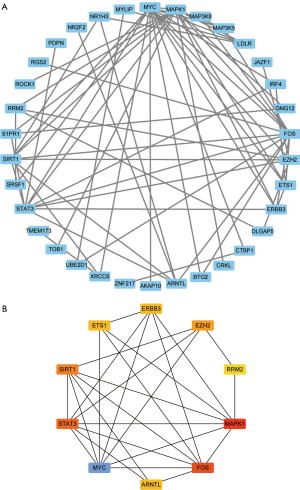
Survival-associated lncRNAs in the ceRNA network
To evaluate the association of lncRNAs in the ceRNA network with the prognosis of patients with ACC, a Kaplan-Meier analysis was performed. Using P value <0.001 as the threshold, we identified five lncRNAs significantly associated with overall survival (OS). Three of the lncRNAs (LINC00887, MEIS1-AS2, MIR29B2CHG) were positively correlated with OS, while the remaining two (MIR503HG, SREBF2-AS1) were negatively correlated with OS (Figure 6).
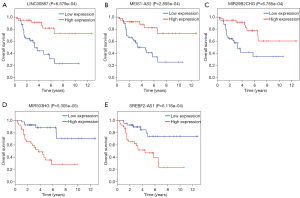
Discussion
Despite significant advances in the understanding of adrenocortical carcinoma and its molecular mechanisms, survival has improved for only some patients (30,31). To date, early diagnosis and surgical resection of the tumor remains the key to treatment. Therefore, improved understanding of the pathogenesis of ACC is essential for preventing the occurrence and progression of ACC.
With the development of genome-wide and transcriptome sequencing technologies, lncRNAs have received increasing attention. However, the role of lncRNAs in adrenocortical carcinoma remains unclear compared to other malignancies. Our study explored the relationship between specific lncRNAs in the ceRNA network and adrenocortical carcinoma using bioinformatics methods, opening up an exciting field of research aimed at better understanding the pathogenesis of adrenocortical carcinoma. LncRNAs play a key role in the development of tumors, and their expression is involved in the growth and metastasis of tumor cells (32).
In the present study, we first identified 391 lncRNAs, 1,313 mRNAs that were aberrantly expressed between the adrenocortical carcinoma samples and normal control samples from GEO. Subsequently, we used bioinformatics tools to establish a ceRNA coexpression network with 87 lncRNAs, 31 miRNAs and 78 mRNA. Then, Using GO and KEGG analyses, we determined the functions and signaling pathways of mRNAs in ceRNA networks. We further identified 5 key lncRNA from the ceRNA network that was related to OS.
The GO and KEGG pathway analyses of the 78 DEmRNAs in the ceRNA network identified the important BPs and pathways in ACC, most of which were classic pathways and BPs that play critical roles in ACC, such as the MAPK signaling pathway and MicroRNAs in cancer. Interestingly, some of the other novel BPs and pathways involved in ACC progression and development, such as Human cytomegalovirus infection, ErbB signaling pathway, Cellular senescence and transcription factor activity, supplemented the findings of previous studies.
Here, we constructed a lncRNA-miRNA-mRNA regulatory network in ACC. Our findings indicate that several specific lncRNAs play an important role in regulating the occurrence and progression of ACC by regulating a series of miRNAs and mRNAs. To the best of our knowledge, this study is the first to identify the ceRNA network in ACC. We identified 391 differentially expressed lncRNAs and 1313 differentially expressed mRNAs in ACC by analyzing publicly available datasets [GSE61359 (24), Glover et al.]. Because of the differences in the versions of the software used in previous literature reports, the differentially expressed genes obtained from the analysis differ. However, the limma package we use is currently the most widely used package for analyzing differential expression. Limma is an R package for the analysis of gene expression microarray data, especially the assessment of differential expression. Empirical Bayesian methods are used to provide stable results even when the number of arrays is small. The differential expression functions apply to all microarray technologies including Affymetrix and other single-channel oligonucleotide platforms.
We further identified 79 differentially expressed lncRNAs in the ceRNA network. By analyzing the association of specific lncRNAs in the ceRNA network with the prognosis of patients with ACC, we identified five lncRNAs that are significantly associated with ACC survival, including three lncRNAs (LINC00887, MEIS1-AS2, MIR29B2CHG) positively correlated with OS and two lncRNAs (MIR503HG, SREBF2-AS1) negatively correlated with OS. Searching for relevant literature found that LINC00887 can reduce the invasiveness and metastasis of non-small cell lung cancer (32). MIR503HG is highly expressed in anaplastic lymphoma kinase (ALK)-negative cell lines, and overexpression of MIR503HG enhances tumor cell growth (33). Our study also suggests that high expression of MIR503HG and low expression of LINC00887 is associated with poor overall survival. There are no related literatures to study the remaining three lncRNAs. Therefore, further research is needed to verify the function of these lncRNAs in ACC and other cancers.
Similar to our investigation, Glover et al. presented a group of differentially expressed lncRNAs (such as H19, GAS5, MALAT1, and PRINS) in ACC and verified some lncRNAs through external validation; and confirmed that the lncRNA PRINS is a possible tumour suppressor in ACC (24). Hassan et al. revealed that down-regulation of MALAT1 inhibits tumor cell proliferation and tumorigenesis (34). Guo et al. found that UCA1 was highly expressed in ACC and closely associated with the TNM stage and metastasis of ACC patients. Overexpression of UCA1 significantly promoted the proliferation and suppressed the apoptosis of ACC cells (35). Our study also found differential expression of H19 between ACC and normal adrenal glands. Better than previous studies, our study established a lncRNA-miRNA-mRNA ceRNA network among the DEGs in ACC and normal adrenal glands.
However, some limitations of this study should be noted. First, although the dataset used in this study has been reported and validated, further experiments are needed to verify the difference in specific lncRNA expression in ACC. Second, the public dataset for analyzing ACC-related lncRNAs is limited, and further expansion of ACC-related lncRNA datasets is required through high-throughput sequencing technology.
Conclusions
In conclusion, we constructed a ceRNA network and proposed a new method for lncRNA research in ACC. Our findings provide important new clues for further exploration of lncRNAs in the pathogenesis of ACC.
Acknowledgments
The authors appreciate the valuable suggestions from other members of their team. This abstract of this paper was presented at the SUI conference as a conference talk with interim findings.
Funding: The present work was supported by
Footnote
Conflicts of Interest: All authors have completed the ICMJE uniform disclosure form (available at http://dx.doi.org/10.21037/tcr.2019.09.34). The authors have no conflicts of interest to declare.
Ethical Statement: The authors are accountable for all aspects of the work in ensuring that questions related to the accuracy or integrity of any part of the work are appropriately investigated and resolved. This study was conducted according to the publication guidelines provided by the GEO and TCGA; therefore, further ethics committee approval was not required.
Open Access Statement: This is an Open Access article distributed in accordance with the Creative Commons Attribution-NonCommercial-NoDerivs 4.0 International License (CC BY-NC-ND 4.0), which permits the non-commercial replication and distribution of the article with the strict proviso that no changes or edits are made and the original work is properly cited (including links to both the formal publication through the relevant DOI and the license). See: https://creativecommons.org/licenses/by-nc-nd/4.0/.
References
- Fassnacht M, Dekkers O, Else T, et al. European Society of Endocrinology Clinical Practice Guidelines on the Management of Adrenocortical Carcinoma in Adults, in collaboration with the European Network for the Study of Adrenal Tumors. Eur J Endocrinol 2018;179:G1-46. [Crossref] [PubMed]
- Gaujoux S, Mihai R. European Society of Endocrine Surgeons (ESES) and European Network for the Study of Adrenal Tumours (ENSAT) recommendations for the surgical management of adrenocortical carcinoma. Br J Surg 2017;104:358-76. [Crossref] [PubMed]
- Terzolo M, Angeli A, Fassnacht M, et al. Adjuvant mitotane treatment for adrenocortical carcinoma. N Engl J Med 2007;356:2372-80. [Crossref] [PubMed]
- Fassnacht M, Terzolo M, Allolio B, et al. Combination chemotherapy in advanced adrenocortical carcinoma. N Engl J Med 2012;366:2189-97. [Crossref] [PubMed]
- Henning JEK, Deutschbein T, Altieri B, et al. Gemcitabine-Based Chemotherapy in Adrenocortical Carcinoma: A Multicenter Study of Efficacy and Predictive Factors. J Clin Endocrinol Metab 2017;102:4323-32. [Crossref] [PubMed]
- Gharzai LA, Green MD, Griffith KA, et al. Adjuvant Radiation Improves Recurrence-Free Survival and Overall Survival in Adrenocortical Carcinoma. J Clin Endocrinol Metab 2019;104:3743-50. [Crossref] [PubMed]
- Altieri B, Colao A, Faggiano A. The role of insulin-like growth factor system in the adrenocortical tumors. Minerva Endocrinol 2019;44:43-57. [PubMed]
- Berruti A, Sperone P, Ferrero A, et al. Phase II study of weekly paclitaxel and sorafenib as second/third-line therapy in patients with adrenocortical carcinoma. Eur J Endocrinol 2012;166:451-8. [Crossref] [PubMed]
- Casey RT, Giger O, Seetho I, et al. Rapid disease progression in a patient with mismatch repair-deficient and cortisol secreting adrenocortical carcinoma treated with pembrolizumab. Semin Oncol 2018;45:151-5. [Crossref] [PubMed]
- Kroiss M, Quinkler M, Johanssen S, et al. Sunitinib in refractory adrenocortical carcinoma: a phase II, single-arm, open-label trial. J Clin Endocrinol Metab 2012;97:3495-503. [Crossref] [PubMed]
- O'Sullivan C, Edgerly M, Velarde M, et al. The VEGF inhibitor axitinib has limited effectiveness as a therapy for adrenocortical cancer. J Clin Endocrinol Metab 2014;99:1291-7. [Crossref] [PubMed]
- Prensner JR, Chinnaiyan AM. The emergence of lncRNAs in cancer biology. Cancer Discov 2011;1:391-407. [Crossref] [PubMed]
- Fatica A, Bozzoni I. Long non-coding RNAs: new players in cell differentiation and development. Nat Rev Genet 2014;15:7-21. [Crossref] [PubMed]
- Li CH, Chen Y. Targeting long non-coding RNAs in cancers: progress and prospects. Int J Biochem Cell Biol 2013;45:1895-910. [Crossref] [PubMed]
- Zhou M, Xu W, Yue X, et al. Relapse-related long non-coding RNA signature to improve prognosis prediction of lung adenocarcinoma. Oncotarget 2016;7:29720-38. [PubMed]
- Sun J, Chen X, Wang Z, et al. A potential prognostic long non-coding RNA signature to predict metastasis-free survival of breast cancer patients. Sci Rep 2015;5:16553. [Crossref] [PubMed]
- Zhou M, Sun Y, Sun Y, et al. Comprehensive analysis of lncRNA expression profiles reveals a novel lncRNA signature to discriminate nonequivalent outcomes in patients with ovarian cancer. Oncotarget 2016;7:32433-48. [PubMed]
- Hu Y, Chen HY, Yu CY, et al. A long non-coding RNA signature to improve prognosis prediction of colorectal cancer. Oncotarget 2014;5:2230-42. [Crossref] [PubMed]
- Zhou M, Zhao H, Xu W, et al. Discovery and validation of immune-associated long non-coding RNA biomarkers associated with clinically molecular subtype and prognosis in diffuse large B cell lymphoma. Mol Cancer 2017;16:16. [Crossref] [PubMed]
- Salmena L, Poliseno L, Tay Y, et al. A ceRNA hypothesis: the Rosetta Stone of a hidden RNA language? Cell 2011;146:353-8. [Crossref] [PubMed]
- Wang X, Li M, Wang Z, et al. Silencing of long noncoding RNA MALAT1 by miR-101 and miR-217 inhibits proliferation, migration, and invasion of esophageal squamous cell carcinoma cells. J Biol Chem 2015;290:3925-35. [Crossref] [PubMed]
- Jiao C, Song Z, Chen J, et al. lncRNA-UCA1 enhances cell proliferation through functioning as a ceRNA of Sox4 in esophageal cancer. Oncol Rep 2016;36:2960-6. [Crossref] [PubMed]
- Edgar R, Domrachev M, Lash AE. Gene Expression Omnibus: NCBI gene expression and hybridization array data repository. Nucleic Acids Res 2002;30:207-10. [Crossref] [PubMed]
- Glover AR, Zhao JT, Ip JC, et al. Long noncoding RNA profiles of adrenocortical cancer can be used to predict recurrence. Endocr Relat Cancer 2015;22:99-109. [Crossref] [PubMed]
- Zheng S, Cherniack AD, Dewal N, et al. Comprehensive Pan-Genomic Characterization of Adrenocortical Carcinoma. Cancer Cell 2016;29:723-36. [Crossref] [PubMed]
- Wong N, Wang X. miRDB: an online resource for microRNA target prediction and functional annotations. Nucleic Acids Res 2015;43:D146-52. [Crossref] [PubMed]
- Chou CH, Chang NW, Shrestha S, et al. miRTarBase 2016: updates to the experimentally validated miRNA-target interactions database. Nucleic Acids Res 2016;44:D239-47. [Crossref] [PubMed]
- Fromm B, Billipp T, Peck LE, et al. A Uniform System for the Annotation of Vertebrate microRNA Genes and the Evolution of the Human microRNAome. Annu Rev Genet 2015;49:213-42. [Crossref] [PubMed]
- Shannon P, Markiel A, Ozier O, et al. Cytoscape: a software environment for integrated models of biomolecular interaction networks. Genome Res 2003;13:2498-504. [Crossref] [PubMed]
- Assie G, Jouinot A, Fassnacht M, et al. Value of Molecular Classification for Prognostic Assessment of Adrenocortical Carcinoma. JAMA Oncol 2019; [Epub ahead of print]. [Crossref] [PubMed]
- Lippert J, Appenzeller S, Liang R, et al. Targeted Molecular Analysis in Adrenocortical Carcinomas: A Strategy Toward Improved Personalized Prognostication. J Clin Endocrinol Metab 2018;103:4511-23. [Crossref] [PubMed]
- Tian Y, Yu M, Sun L, et al. Long noncoding RNA00887 reduces the invasion and metastasis of nonsmall cell lung cancer by causing the degradation of miRNAs. Oncol Rep 2019;42:1173-82. [PubMed]
- Huang PS, Chung IH, Lin YH, et al. The Long Non-Coding RNA MIR503HG Enhances Proliferation of Human ALK-Negative Anaplastic Large-Cell Lymphoma. Int J Mol Sci 2018;19: [Crossref] [PubMed]
- Hassan N, Zhao J, Glover AR, et al. Reciprocal interplay of miR-497 and MALAT1 promotes tumourigenesis of adrenocortical cancer. Endocr Relat Cancer 2019; [Epub ahead of print]. [Crossref] [PubMed]
- Guo N, Sun Q, Fu D, et al. Long non-coding RNA UCA1 promoted the growth of adrenocortical cancer cells via modulating the miR-298-CDK6 axis. Gene 2019;703:26-34. [Crossref] [PubMed]