Screening of differential expressed genes from gene chip and sequencing data and evaluate its prognostic values in males with digestive system neoplasms
Introduction
Tumors are hereditary diseases whose biological basis is genetic abnormality. Oncogenic factors lead to somatic cell gene mutations, resulting in dysfunction and disorder of gene expression, affecting the biological activity and genetic characteristics of cells, and forming tumor cells with different morphology, metabolism and function from normal cells. Genetic abnormalities of different genes lead to different tumors (1). The incidence and mortality rate of tumors in male is higher than that in female, and half of tumors in male occur in the respiratory tract and digestive tract (2). We sought to identify genes that are differential expressed in male with digestive system tumors (MDSN).
Recently, gene expression profiling and bioinformatics analysis have been widely used to identify potential biomarkers for various cancers (3,4). LncRNA CTB-193M12.5 was found associated with prognosis of lung adenocarcinoma by integrated The Cancer Genome Atlas (TCGA) analysis (5). Similarly, an in-depth understanding of the notable signaling pathway was achieved through the analysis of pan-carcinoma using TCGA data (6). Besides, approximately 895 genes and their associated pathways were identified by analyzing the Gene Expression Omnibus (GEO) datasets of 7,168 tumour samples from 16 independent cancer types (7). However, there are still limited studies on MDSN.
DNA methylation is a chemically modified form of DNA that alters hereditary properties without altering DNA sequences and is one of the earliest epigenetic regulatory mechanisms that have been discovered. DNA methylation causes changes in chromatin structure, DNA conformation, DNA stability and DNA-protein interactions, thereby controlling gene expression (8). In general, hypermethylation inhibits gene expression, while hypomethylation leads to gene over-expression. The N6-methyladenosine (m6A) modification is the most common methylation modification in transcripts and involves almost all aspects of mRNA regulation (9). Documents revealed that the modification of m6A is involved in all processes of mRNA transcription and translation, involving various cancers (10,11).
In these studies, the raw gene expression data of TCGA database and GEO database were analyzed, and 46 shared differential expressed genes (DEGs) were found in MDSN tumour tissue samples compared with adjacent normal samples. Therein, a hub of 7 genes (CCNB1, MAD2L1, BUB1, CHEK1, MCM2, CCNA2 and CDC25B) were found to be enriched in cell cycle pathway and the 7 genes were found to be interacted with each other in protein level. Furthermore, MAD2L1, BUB1 and MCM2 was hypomethylation via m6A modification. Survival analysis of MAD2L1, BUB1 and MCM2 suggested that these three genes have a promising prognostic values in MDSN. Particular, in stomach adenocarcinoma (STAD), there was a race difference between white and Asian people. Medicine molecules related analysis shown that medicine molecules, I-threonine (ID: PA451673) and enzymes (ID: PA164712734) might be efficient medicines in these three genes up-regulated MDSN patients.
Methods
TCGA database
RNA sequence expression profiles of digestive system neoplasms in males were obtained from TCGA database, consists of 976 male tumour tissues and 81 normal tissues, including esophageal carcinoma (ESCA), STAD, liver hepatocellular carcinoma (LIHC), colon adenocarcinoma and rectum adenocarcinoma (COAD and READ) (Table S1).
GEO database
Microarray expression profiles of GSE41258, GSE23400, GSE3335 and GSE84402 were obtained from GEO database and consisted of 277 tumour tissues and 143 normal tissues (Table S1). Methylation expression profiles of GSE73003 were downloaded in GEO database, containing 20 paired tumour tissues and non-tumour tissues.
Identification of DEGs
The DEGs between tumour tissues and adjacent non-tumorous tissues in MDSN in TCGA were identified using limma package by R software 3.5.1. While the DEGs in GEO were performed by GEO2R (https://www.ncbi.nlm.nih.gov/geo/geo2r/). |log FC|>2 and P<0.01 was selected as significant.
Bioinformatics analysis
DAVID (https://david.ncifcrf.gov/home.jsp) was used to conducted Gene ontology (GO) and Kyoto Encyclopedia of Genes and Genomes (KEGG) pathway enrichment analysis of DEGs. The threshold of FDR <0.05, P value<0.05 was regarded as significantly enrichment. STRING (https://string-db.org/) was performed to construct a protein-protein interaction (PPI) network for DEGs. P<0.001 and combined score >0.9 were selected as significant.
The RMBase (http://rna.sysu.edu.cn/rmbase) and m6AVar (http://m6avar.renlab.org/index.html) was used to investigate modification type of genes.
Statistic analysis and clinical relevance
Kaplan-Meier plotter was used to predict the prognostic values of genes in MDSN and the race difference between white and Asian people. Correlation analysis of genes was performed in Graphpad Prism 5 software. GEPIA (http://gepia.cancer-pku.cn/) was used for clinical relevance analysis. The data are presented as the mean ± standard deviation. P<0.05 was set as the cutoff criterion.
DEGs related medicine analysis
WebGestalt (http://www.webgestalt.org/option.php) is a functional web tool for enrichment analysis. Information in this web was collected from NCBI GEO, DrugBank, KEGG and dbSNP. In Gene Set Enrichment Analysis part, medicine molecules related DEGs were screened. P value <0.05 was regarded as significant.
Results
Identification of DEGs in MDSN
A total of 976 cancerous and 81 para-cancerous raw mRNA expression data of MDSN were downloaded from the TCGA database. R language software was used to screen DEGs in MDSN, including mRNAs and lncRNAs. In MDSN, 4 cancer types were included, ESCA, STAD, LIHC, COAD and READ. All DEGs were shown in volcano plots (Figure 1A). A total of 195 shared DEGs are common in MDSN in TCGA dataset (Figure 1B). We further screened DEGs in GEO dataset by GEO2R, 289 shared DEGs were found (Figure 1C). In TCGA and GEO database, 46 common DEGs were found in MDSN, including 29 up-regulated genes and 17 down-regulated genes (Figure 1D).
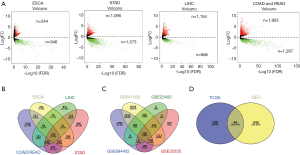
Bioinformatics analysis of 46 DEGs in MDSN
GO and KEGG analysis was conducted on DAVID's website tool and it was found that these 46 DEGs significantly affected GO function and KEGG signaling pathways (Figure 2). In GO terms, as to molecular function, the DEGs were significantly enriched in DNA clamp loader activity, cell cycle, ATPase activity, single-stranded DNA-dependent ATPase activity, microtubule motor activity, etc. About cellular component, DNA replication factor C complex, ATP binding, microtubule, proteinaceous extracellular matrix, condensed chromosome kinetochore, etc., were significantly enriched with these DEGs. In addition, the most enriched GO terms in biological process were DNA unwinding involved in DNA replication, positive regulation of DNA-directed DNA polymerase activity, G2/M transition of mitotic cell cycle, positive regulation of mitotic cell cycle, positive regulation of apoptotic process, etc. In KEGG pathway terms, DEGs were enriched in cell cycle, DNA replication and mismatch repair. Cell cycle is the most DEGs enrichment pathway and a hub of 7 genes (CCNB1, MAD2L1, BUB1, CHEK1, MCM2, CCNA2 and CDC25B) were included.
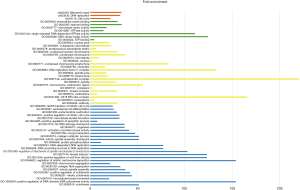
Then, PPI network of STRING result was constructed to predict the interaction between these 46 DEGs. A total of 153 protein interaction nodes were shown in the PPI network, among them CCNB1, MAD2L1, BUB1, CHEK1, MCM2, CCNA2 and CDC25B were found to be interaction with each other in protein level (Figure 3A). The connected nodes in the network are at required interaction score >0.9. The top 20 combined score genes were selected (Figure 3B) and CCNB1, MAD2L1, BUB1, CHEK1, MCM2 and CCNA2 were included in the top 20 genes. Combined with KEGG and PPI analysis, we selected the cell cycle pathway for further study.
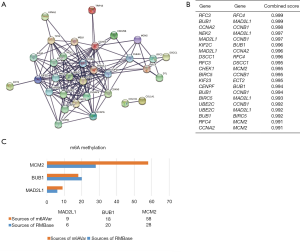
Methylation status analysis of cell cycle pathway related 7 genes
We downloaded the raw mRNA methylation expression data from the GEO database, and R language was used to found differential expressed CpG methylation sites. In GSE73003, a total of 13,660 CpG methylation sites were found differential expressed, of which MAD2L1, BUB1 and MCM2 was hypomethylation. Further investigation in RMBase and m6AVar, we found that MAD2L1, BUB1 and MCM2 was hypomethylation via m6A modification (Figure 3C).
Survival analysis and clinical relevance
After verifying BUB1, MAD2L1 and MCM2 were up-regulation in MDSN than adjacent normal tissues (Figure S1). We predicted the prognostic value of BUB1, MAD2L1 and MCM2 in Kaplan-Meier plotter. Over-expression of BUB1, MAD2L1 and MCM2 significantly lead to a worse prognostic in liver hepatocellular carcinoma (Figure 4A), while causing a better prognostic in STAD (Figure 4B). Due to the number limited of males patient, BUB1, MAD2L1 and MCM2 tends to be better prognostic in rectum adenocarcinoma, however the difference is not statistically significant.
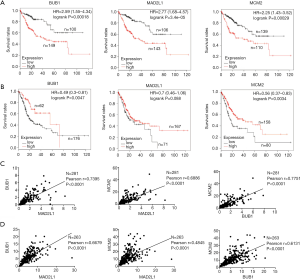
For ESCA, there is no significantly prognostic value of BUB1, MAD2L1 and MCM2 (Figure S2). Further co-expression analysis were performed, we found that BUB1, MAD2L1 and MCM2 co-expression with each other in mRNA level (Figure 4C,D). The co-expression and interaction (Figure 3A) of BUB1, MAD2L1 and MCM2 might increase the prognostic value in liver hepatocellular carcinoma and STAD. Further, clinical relevance analysis was performed in Kaplan-Meier plotter and GEPIA. For survival analysis of different race, high expression of BUB1, MAD2L1 and MCM2 leading a poorer prognostic in hepatocellular carcinoma in both Asian and white people (Figure 5A,B). In STAD, up-regulation of BUB1, MAD2L1 and MCM2 causing a better prognostic in Asian (Figure 5C); so did MCM2 in white people, while BUB1 and MAD2L1 has no significant prognostic value (Figure 5D). Besides, in GEPIA, we found that BUB1, MAD2L1 and MCM2 expression elevated in stage I to stage III, while down-regulated in stage IV (Figure 5E).
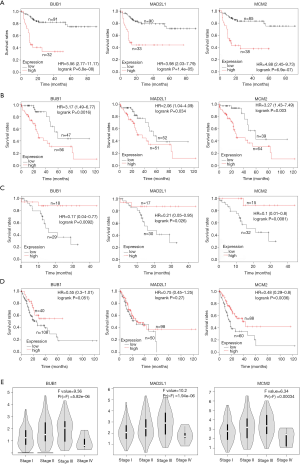
Medicine molecules related to BUB1, MAD2L1 and MCM2
Based on the above studies, molecule drugs of BUB1, MAD2L1 and MCM2 was identified in WebGestalt (Table 1). It shown that both I-threonine (ID: PA451673) and enzymes (ID: PA164312734) are closely related to BUB1, MAD2L1 and MCM2. For BUB1 and MAD2L1, they are significantly enriched with taxanes (ID: PA150481189), paclitaxel (ID: PA450761), calcium (ID: PA164712582) and I-serine (ID: PA451330). However, tumor detection (ID: PA164713367) and dactinomycin (ID: PA151917012) were only correlated with MCM2, while cisplatin (ID: PA449014) and vinblastine (ID: PA451877) were correlated with MAD2L1, respectively.
Table 1
Drug | ID | Parameters | Genes |
---|---|---|---|
Taxanes | PA150481189 | R=76.66; P value =2.23e-04; FDR =1.71e-01 | MAD2L1; BUB1 |
Paclitaxel | PA450761 | R=76.66; P value =2.23e-04; FDR =1.71e-01 | MAD2L1; BUB1 |
l-threonine | PA451673 | R=14.98; P value =2.96e-04; FDR =1.71e-01 | MAD2L1; BUB1; MCM2 |
Enzymes | PA164712734 | R=6.19; P value =4.2e-03; FDR =1e+00 | MAD2L1; BUB1; MCM2 |
Vinblastine | PA451877 | R=98.16; P value =1.02e-02; FDR =1e+00 | MAD2L1 |
Calcium | PA164712582 | R=10.23; P value =1.22e-02; FDR =1e+00 | MAD2L1; BUB1 |
l-serine | PA451330 | R=8.85; P value =1.62e-02; FDR =1e+00 | MAD2L1; BUB1 |
Cisplatin | PA449014 | R=27.01; P value =3.66e-02; FDR =1e+00 | MAD2L1 |
Tumour detection | PA164713367 | R=21.99; P value =4.48e-02; FDR =1e+00 | MCM2 |
Dactinomycin | PA151917012 | R=20.53; P value =4.79e-02; FDR =1e+00 | MCM2 |
Discussion
Colorectal cancer is the third most common cancer diagnosed in men worldwide, while liver, stomach and esophageal cancers are the fourth, fifth and ninth cancers, respectively. Liver cancer is the second leading cause of death, while gastric, colorectal and esophageal cancers rank third, fourth and sixth globally (2). These four types of cancers all belonged to digestive system neoplasms. So we committed to identifying the commonality in MDSN and give an insight to its mechanism.
TCGA database contains a variety of original expression data of tumors, including mRNA expression data, methylation data, gene mutation data, and related clinical data (12). Increasing literature report that through the analysis of the TCGA database, a large number of new research directions emerge. Previous researchers analyzed the TCGA database to determine a targeted treatment trial and a roadmap for stratifying gastric adenocarcinoma patients (13). Through systematic analysis of the TCGA database, biomarkers and transgenes were identified to point to relevant cellular processes and new therapeutic targets (14). With the development of scientific research and detection technology, the GEO database has become an important data tool to make up for the defects of the TCGA database. GEO has more accurate expression data, more precise experimental conditions, and further advancing the development of bioinformatics analysis. The combination of TCGA and GEO will provide more accurate and reliable clinical guidance for scientific research (15). We downloaded raw gene expression profiles from the TCGA database and GEO database, then DEGs analysis was performed, and 46 shared DEGs were found in the MDSN. Combined with bioinformatics analysis, we found that these 46 DEGs significantly enriched in GO and KEGG pathways. Of which, a hub of 7 genes (CCNB1, MAD2L1, BUB1, CHEK1, MCM2, CCNA2 and CDC25B) were interacted with each other in protein level and significantly enriched in cell cycle pathway. We hypothesized that the dysregulation of the downstream cell cycle pathway may play an important role in the tumorigenesis and development of MDSN.
DNA methylation changes in promoter and genomic regions produce cancer phenotypes by affecting gene transcription (16). Previous studies have shown that DNA methylation of its promoter significantly down-regulated miR-486-5p and promoted cell proliferation and invasion in rectal cancer by activating the PLAGL2/IGF2/beta-catenin signaling pathway (17). Compared with radiation-sensitive cells in squamous cell carcinoma of the head and neck, DNA methylation increased in radiation-resistant cells, so as to further understand the DNA methylation status as a biomarker of radiation-resistant cells (18). We downloaded DNA methylation expression data from the GEO database to investigate differential methylated CPG sites and found that MAD2L1, BUB1and MCM2 was hypomethylation in DMSN tumour tissues. Among the more than 100 chemical modifications that may occur in various types of RNA molecules, m6A is the most abundant internal chemical modification of mRNA, which has the greatest influence on its dynamic regulation (19-21). Ubiquitous m6A modification plays a fundamental regulatory role in gene expression. Besides, studies have shown that binding proteins selectively recognize dynamic m6A modifications to regulate mRNA translation status and longevity (22). Changes in m6A modification are observed to be involved in multiple cellular processes, which might have impacts on several human diseases (23). In order to study the m6A modification state of MAD2L1, BUB1 and MCM2, RMbase and m6AVar were used for analysis, and it was found that MAD2L1, BUB1 and MCM2 were hypomethylated by m6A modification. Therefore, we speculate that the up-regulation of MAD2L1, BUB1 and MCM2 in MDSN may be the result of m6A-modified hypomethylation.
Shi et al. reported (24) that MAD2L1 was up-regulated in cancer tissue, elevated expression of MAD2L1 increased the risk of cancer recurrence and caused a worse survival in lung adenocarcinoma. Up-regulated MAD2L1 enhanced the proliferation, invasion and migration of hepatocellular carcinoma cells and inhibited apoptosis and cell cycle arrest (25). As for BUB1, it is elevated expressed in non-small cell lung cancer and has a poor prognosis (26) similar to prostate cancer (27). However, in gastric adenocarcinoma, elevated BUB1 expression can predict prognosis well (28). In terms of MCM2, high expression of MCM2 leading a worse prognostic in hepatocellular carcinoma (29), while causing a better prognostic in cervical cancer (30). Although MAD2L1, BUB1 and MCM2 up-regulation in various cancers, they play opposite prognostic in different cancer. In this study, we revealed that up-regulation of MAD2L1, BUB1 and MCM2 lead to a worse prognostic in liver hepatocellular carcinoma, while causing a better prognostic in STAD, which provide a exact prognostic for clinical biomarkers. Not only that, we further estimate the survival rates in white and Asian people, we found in hepatocellular carcinoma, elevated MAD2L1, BUB1 and MCM2 function as the poorer prognostic in both races. However, in STAD, elevated MAD2L1, BUB1 and MCM2 signify a better prognostic only in Asian patients but not in white persons.
Chemotherapy resistance is one of the major obstacle in cancer treatment (31-34). We investigated the MAD2L1, BUB1 and MCM2 related anti-cancer drugs and found I-threonine, enzymes, taxanes, paclitaxel, calcium, I-serine, cisplatin and vinblastine are closely related to BUB1, MAD2L1 and MCM2. It is speculated that increased expression of BUB1, MAD2L1 and MCM2 can provide guidance for the use of these drug molecules.
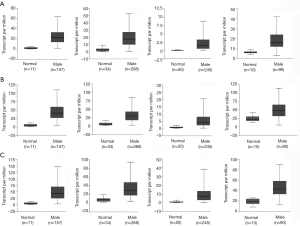
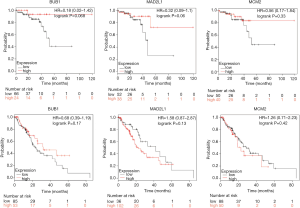
Table S1
TCGA | Tumor tissues (n) | Normal tissues (n) | GEO | Tumor tissues (n) | Normal tissues (n) |
---|---|---|---|---|---|
ESCA | 139 | 8 | GSE41258 | 185 | 54 |
STAD | 241 | 22 | GSE23400 | 53 | 50 |
LIHC | 253 | 28 | GSE3335 | 25 | 25 |
COAD and READ | 343 | 23 | GSE84402 | 14 | 14 |
Acknowledgments
Funding: This work was supported by Grants from
Footnote
Conflicts of Interest: All authors have completed the ICMJE uniform disclosure form (available at http://dx.doi.org/10.21037/tcr.2019.09.58). The authors have no conflicts of interest to declare.
Ethical Statement: The authors are accountable for all aspects of the work in ensuring that questions related to the accuracy or integrity of any part of the work are appropriately investigated and resolved. The study was conducted in accordance with the Declaration of Helsinki (as revised in 2013). The institutional ethical approval and individual informed consent were waived due to the nature of the study.
Open Access Statement: This is an Open Access article distributed in accordance with the Creative Commons Attribution-NonCommercial-NoDerivs 4.0 International License (CC BY-NC-ND 4.0), which permits the non-commercial replication and distribution of the article with the strict proviso that no changes or edits are made and the original work is properly cited (including links to both the formal publication through the relevant DOI and the license). See: https://creativecommons.org/licenses/by-nc-nd/4.0/.
References
- Xia LC, Bell JM, Wood-Bouwens C, et al. Identification of large rearrangements in cancer genomes with barcode linked reads. Nucleic Acids Res 2018;46:e19. [Crossref] [PubMed]
- Bray F, Ferlay J, Soerjomataram I, et al. Global cancer statistics 2018: GLOBOCAN estimates of incidence and mortality worldwide for 36 cancers in 185 countries. CA Cancer J Clin 2018;68:394-424. [Crossref] [PubMed]
- Barrett T, Wilhite SE, Ledoux P, et al. NCBI GEO: archive for functional genomics data sets--update. Nucleic Acids Res 2013;41:D991-5. [Crossref] [PubMed]
- Ryan M, Wong WC, Brown R, et al. TCGASpliceSeq a compendium of alternative mRNA splicing in cancer. Nucleic Acids Res 2016;44:D1018-22. [Crossref] [PubMed]
- Wang X, Li G, Luo Q, et al. Integrated TCGA analysis implicates lncRNA CTB-193M12.5 as a prognostic factor in lung adenocarcinoma. Cancer Cell Int 2018;18:27. [Crossref] [PubMed]
- Neapolitan R, Horvath CM, Jiang X. Pan-cancer analysis of TCGA data reveals notable signaling pathways. BMC Cancer 2015;15:516. [Crossref] [PubMed]
- Cava C, Bertoli G, Colaprico A, et al. Integration of multiple networks and pathways identifies cancer driver genes in pan-cancer analysis. BMC Genomics 2018;19:25. [Crossref] [PubMed]
- Estève PO, Zhang G, Ponnaluri VK, et al. Binding of 14-3-3 reader proteins to phosphorylated DNMT1 facilitates aberrant DNA methylation and gene expression. Nucleic Acids Res 2016;44:1642-56. [Crossref] [PubMed]
- Huang J, Yin P. Structural Insights into N(6)-methyladenosine (m(6)A) Modification in the Transcriptome. Genomics Proteomics Bioinformatics 2018;16:85-98. [Crossref] [PubMed]
- Roundtree IA, Evans ME, Pan T, et al. Dynamic RNA Modifications in Gene Expression Regulation. Cell 2017;169:1187-200. [Crossref] [PubMed]
- Wang S, Sun C, Li J, et al. Roles of RNA methylation by means of N(6)-methyladenosine (m(6)A) in human cancers. Cancer Lett 2017;408:112-20. [Crossref] [PubMed]
- Tomczak K, Czerwinska P, Wiznerowicz M. The Cancer Genome Atlas (TCGA): an immeasurable source of knowledge. Contemp Oncol (Pozn) 2015;19:A68-77. [Crossref] [PubMed]
- Cancer Genome Atlas Research Network. Comprehensive molecular characterization of gastric adenocarcinoma. Nature 2014;513:202-9. [Crossref] [PubMed]
- Rykunov D, Beckmann ND, Li H, et al. A new molecular signature method for prediction of driver cancer pathways from transcriptional data. Nucleic Acids Res 2016;44:e110. [Crossref] [PubMed]
- Zhang H, Liu T, Zhang Z, et al. Integrated Proteogenomic Characterization of Human High-Grade Serous Ovarian Cancer. Cell 2016;166:755-65. [Crossref] [PubMed]
- Molnár B, Galamb O, Peterfia B, et al. Gene promoter and exon DNA methylation changes in colon cancer development - mRNA expression and tumour mutation alterations. BMC Cancer 2018;18:695. [Crossref] [PubMed]
- Liu X, Chen X, Zeng K, et al. DNA-methylation-mediated silencing of miR-486-5p promotes colorectal cancer proliferation and migration through activation of PLAGL2/IGF2/beta-catenin signal pathways. Cell Death Dis 2018;9:1037. [Crossref] [PubMed]
- Chen X, Liu L, Mims J, et al. Analysis of DNA methylation and gene expression in radiation-resistant head and neck tumors. Epigenetics 2015;10:545-61. [Crossref] [PubMed]
- Ianniello Z, Fatica A. N6-Methyladenosine Role in Acute Myeloid Leukaemia. Int J Mol Sci 2018; [Crossref] [PubMed]
- Golovina AY, Dzama MM, Petriukov KS, et al. Method for site-specific detection of m6A nucleoside presence in RNA based on high-resolution melting (HRM) analysis. Nucleic Acids Res 2014;42:e27. [Crossref] [PubMed]
- Bartosovic M, Molares HC, Gregorova P, et al. N6-methyladenosine demethylase FTO targets pre-mRNAs and regulates alternative splicing and 3'-end processing. Nucleic Acids Res 2017;45:11356-70. [Crossref] [PubMed]
- Huang Y, Yan J, Li Q, et al. Meclofenamic acid selectively inhibits FTO demethylation of m6A over ALKBH5. Nucleic Acids Res 2015;43:373-84. [Crossref] [PubMed]
- He L, Li J, Wang X, et al. The dual role of N6-methyladenosine modification of RNAs is involved in human cancers. J Cell Mol Med 2018;22:4630-9. [Crossref] [PubMed]
- Shi YX, Zhu T, Zou T, et al. Prognostic and predictive values of CDK1 and MAD2L1 in lung adenocarcinoma. Oncotarget 2016;7:85235-43. [Crossref] [PubMed]
- Li Y, Bai W, Zhang J. MiR-200c-5p suppresses proliferation and metastasis of human hepatocellular carcinoma (HCC) via suppressing MAD2L1. Biomed Pharmacother 2017;92:1038-44. [Crossref] [PubMed]
- Huang R, Gao L. Identification of potential diagnostic and prognostic biomarkers in non-small cell lung cancer based on microarray data. Oncol Lett 2018;15:6436-42. [PubMed]
- Fu X, Chen G, Cai ZD, et al. Overexpression of BUB1B contributes to progression of prostate cancer and predicts poor outcome in patients with prostate cancer. Onco Targets Ther 2016;9:2211-20. [PubMed]
- Stahl D, Braun M, Gentles AJ, et al. Low BUB1 expression is an adverse prognostic marker in gastric adenocarcinoma. Oncotarget 2017;8:76329-39. [Crossref] [PubMed]
- Liu Z, Li J, Chen J, et al. MCM family in HCC: MCM6 indicates adverse tumour features and poor outcomes and promotes S/G2 cell cycle progression. BMC Cancer 2018;18:200. [Crossref] [PubMed]
- Wang M, Li L, Liu J, et al. A gene interaction network based method to measure the common and heterogeneous mechanisms of gynecological cancer. Mol Med Rep 2018;18:230-42. [PubMed]
- Chen J, Yang X, Huang L, et al. Development of dual-drug-loaded stealth nanocarriers for targeted and synergistic anti-lung cancer efficacy. Drug Deliv 2018;25:1932-42. [Crossref] [PubMed]
- Huang H, Li T, Chen M, et al. Identification and validation of NOLC1 as a potential target for enhancing sensitivity in multidrug resistant non-small cell lung cancer cells. Cell Mol Biol Lett 2018;23:54. [Crossref] [PubMed]
- Zeng L, Liao Q, Zou Z, et al. Long Non-Coding RNA XLOC_006753 Promotes the Development of Multidrug Resistance in Gastric Cancer Cells Through the PI3K/AKT/mTOR Signaling Pathway. Cell Physiol Biochem 2018;51:1221-36. [Crossref] [PubMed]
- Leonetti A, Assaraf YG, Veltsista PD, et al. MicroRNAs as a drug resistance mechanism to targeted therapies in EGFR-mutated NSCLC: Current implications and future directions. Drug Resist Updat 2019;42:1-11. [Crossref] [PubMed]