Down-regulated hsa_circ_0067934 facilitated the progression of gastric cancer by sponging hsa-mir-4705 to downgrade the expression of BMPR1B
Introduction
Gastric cancer, whose incidence accounts for 5.7% of total cancer cases worldwide, is the fifth most commonly diagnosed cancer, lagging just behind lung cancer (11.6%), breast cancer (11.6%), colorectal cancer (10.2%), and prostate cancer (7.1%). Moreover, up to 8.2% mortality of the total cancer-related deaths makes gastric cancer the third leading cause of cancer-related death, ranking next only to lung cancer (18.4%) and colorectal cancer (9.2%) (1). As the only curative treatment for gastric cancer, adequate surgical resection can make the overall 5-year survival rate of patients with early-stage gastric cancer reach 98%. Unfortunately, most patients with gastric cancer were diagnosed at an advanced stage. The lack of an apparent syndrome and practical screening approach at an early stage are likely responsible for the high mortality of gastric cancer (2). In the past few decades, we have also developed adjuvant and neoadjuvant therapies applied according to the stage and risk assessment of gastric cancer, such as pre-/peri- or postoperative chemotherapy and chemoradiotherapy, as well as targeted therapy. Nevertheless, the overall prognosis of advanced gastric cancer remains poor (3,4). We must find new diagnostic approaches to detect gastric cancer in the early stage along with efficient therapies to improve the prognosis of gastric cancer.
Circular RNAs, also known as circRNAs, used to be thought of as the byproducts of the normal splicing process (5), and were first discovered nearly 43 years ago (6) and found pervasive expression in eukaryotic genes in 2012 (7). The critically biological functions of circRNAs were identified in 2013 (8). In recent years, there have been new research hotspots in the field of cancer, and the understandings about circRNAs have been deepened. Most circRNAs are non-coding RNA and are located in the cytoplasm. Different from linear RNAs, the downstream 3’ splice site and the upstream 5’ splice site of circRNAs are complementary. The formation of the unique “backsplice” causes circRNAs to become a highly conserved and stably covalently closed RNA circlea (9). Recent works have revealed that circRNAs mostly generated from exons of protein-coding genes have numerous functions. For instance, they can act as miRNA sponges to regulate the expression of miRNA; binding tumor suppressor proteins by protein-binding sites on circRNAs to inhibit oncogenesis (10); the exons of the circRNAs may translate protein to influence the function of cell; changing mRNA expression from the host gene (11). Also, increasing reports have found that circRNAs are aberrantly expressed in various cancers, such as breast cancer (12), colorectal carcinoma (CRC) (13), ovarian cancer (14), hepatocellular carcinoma (HCC) (15) and so on. More importantly, circRNAs with a highly stable structure are enriched in blood, plasma, and platelets. Meanwhile, changes in circRNA expression level (downregulation or upregulation) were found to be tightly related to tumorigenesis, progression, invasion, and cell proliferation. Given the circRNA properties mentioned above, we speculated that circRNAs might be promising cancer biomarkers that could be detected at an early stage of cancer and a therapeutic target to improve the prognosis of cancer (11).
This study aimed to screen the significantly cancer-related circRNAs in gastric cancer and find potential mechanisms among oncogenesis. First, microarray datasets of gastric cancer tissue were downloaded from Gene Expression Omnibus (GEO) database. Secondly, integrated bioinformatics analysis methods were applied to filter markedly differently expressed circRNAs (DECs). Thirdly, the targeted miRNAs and mRNAs were predicted. Subsequently, Gene Oncology (GO), Kyoto Encyclopedia of Genes and Genomes (KEGG) analyses about the DECs were performed to identify the functions of DECs. To validate the predictions about DECs targets, we also downloaded the expression data of miRNAs and mRNAs from The Cancer Genome Atlas (TCGA). Aberrantly expressed miRNAs and mRNAs were obtained by the edgeR package. Overlapped miRNAs and mRNAs in predicted results and outputs from the TCGA database were obtained by taking the intersection. Lastly, we discovered the hsa_circ_0067934/hsa-mir-4705/BMPR1B axis, which promoted the occurrence and progression of gastric cancer by regulating the Hippo pathway and the signaling pathways regulating pluripotency of stem cells.
Methods
Acquisition of expression data from GEO
We screened circRNA expression profiles from Gene Expression Omnibus (GEO), a regularly updated database storing numerous circRNAs expression profiles (16), by searching the keywords “circRNA and gastric cancer.” As a result, 8 datasets were identified. Further screening criteria were set as the following: (I) the specimens must be human gastric cancer tissue; (II) the type of datasets must be expression profiling by array; (III) samples must be able to be divided into a normal group and cancer group; (IV) no special treatments, such as radiotherapy and chemotherapy, were applied on patients; (V) the name of circRNAs in the data of platforms must be a standard name or named by Agilent Arraystar. The raw expression profiles and platforms were retrieved.
Screening of aberrantly expressed circRNAs by integrated bioinformatics analysis
Normalization and log2 transformation for raw expression data of all datasets were performed. Then, the limma package in R software was used to analyze differently expressed circRNAs (DECs) in each dataset (17). |logFoldChange| >1 and P value <0.05 were set as cut-off criteria. In order to find more significant DECs, RobustRankAggreg was applied to integrate and rank all DECs (18). Statistical significance was defined as |logFoldChange| >1 and adj. P value <0.05.
Prediction of miRNAs interacted with circRNAs
Cancer-specific circRNA database (CSCD) is a powerful web tool to annotate circRNAs functions, including the prediction of microRNA response element sites (MRE), RNA-binding protein sites, and potential open reading frames (19). Hence, we made use of CSCD to forecast the miRNAs which bind to differently expressed circRNAs.
Prediction of miRNA target genes
The public databases, such as miRDB (20), miRTar Base (21), Target Scan (22), are practical online resources that can carry on functional annotations of miRNAs and predict miRNA-targeted genes. We took miRNAs overlapped in the three databases as significant targets.
Functional enrichment analysis
Database for Annotation, Visualization, and Integrated Discovery (DAVID), an online software that can conduct Gene Ontology (GO) analysis, can perform concretely functional annotations [the molecular function (MF), biological process (BP), and cellular component (CC)] of genes (23). Then, we took DAVID to implement GO analysis about predicted genes, and the P value <0.01 was statistically significant. As an R software package that enables us to carry on biological-term classification and the enrichment analysis of gene clusters, the clusterProfiler package was run for the Kyoto Encyclopedia of Genes and Genomes (KEGG) analysis to discover the potential pathways that DECs may participate in (24). P value <0.01 was set as the cut-off criterion. The R package ggplot2 was utilized to present the results.
Collection of miRNAs and genes expression data from TCGA
TCGA is a publicly accessible database with thousands of tumor samples and contains a variety of molecular data such as gene expression, gene methylation, copy number variation (CNV), and so on (25,26). miRNAs and mRNA expression data tested by experiments in gastric cancer were retrieved from TCGA. The miRNA data met the following criteria: “Disease Type is Adenomas” AND “Primary site is stomach” AND “Project id is TCGA-STAD” AND “Data Category is Transcriptome Profiling” AND “Data Type is miRNA Expression Quantification” AND “Experimental Strategy is miRNA-Seq”, were available. Similarly, the standards set to filter the datasets of mRNAs were as follows: “Disease Type is Adenomas” AND “Primary site is stomach” AND “Project id is TCGA-STAD” AND “Workflow Type is HTseq-Counta” AND “Data Category is Transcriptome Profiling” AND “Data Type is Gene Expression Quantification” AND “Experimental Strategy is miRNA-Seq”.
Selection of differently expressed miRNAs and genes in gastric cancer from TCGA
The aberrantly expressed miRNAs and genes were identified using edgeR package, a software used to analyze differential expression in RNA-seq datasets (27,28), and |logFoldChange| >1 and P value <0.05 were defined as screening standards.
Results
Identification of DECs in gastric cancer
According to the above limitations, four datasets, including GSE78092, GSE83521, GSE89143, and GSE93541, were screened, and the details are shown in Table 1. The expression profiles of the four datasets were normalized and log2-transformed. Based on these standardized data, we conducted the aberrantly expressed analysis to screen the differentially expressed circRNAs (DECs) among the four datasets by limma package with |logFoldChange| >1 and P value <0.05, respectively. Eventually, a total of 196 DECs, consisting of 144 down-regulated circRNAs and 52 up-regulated circRNAs, were identified in GSE78092. In total, 148 DECs were obtained from GSE83521, including 66 down-regulated circRNAs and 82 up-regulated circRNAs. In total, 122 DECs with 94 down-regulated circRNAs and 28 up-regulated circRNAs were selected in GSE89143. Also, we screened 410 DECs consisting of 202 down-regulated circRNAs and 208 up-regulated circRNAs from GSE93541. The aberrant expression of four datasets was expressed in a volcano plot, as shown in Figure 1.
Table 1
GEO | Platform | Sample | Normal | Tumor | Submission | Update | Author |
---|---|---|---|---|---|---|---|
GSE78092 | GPL21485 | Tissue | 3 | 3 | Feb 19, 2016 | Oct 26, 2017 | Huang YS |
GSE83521 | GPL19978 | Tissue | 6 | 6 | Jun 20, 2016 | Dec 15, 2017 | Zhang Y |
GSE89143 | GPL19978 | Tissue | 3 | 3 | Oct 25, 2016 | Jun 27, 2017 | Guo J |
GSE93541 | GPL19978 | Plasma | 3 | 3 | Jan 12, 2017 | Jan 16, 2017 | Guo J |
GEO, Gene Expression Omnibus.
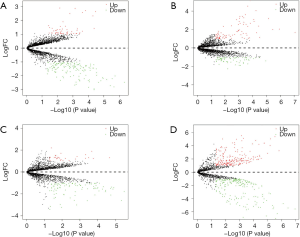
Integrated analysis based on RobustRankAggreg
The analysis outcome of the single dataset was highly likely to increase the ratio of false-positive results. Taking this into account, we decided to adopt the RobustRankAggreg algorithm to integrate the DECs of four datasets, to improve the authenticity of the results. Through rank analysis, two DECs, which were most likely to be linked to gastric cancer, were screened with an adjusted P value <0.05, namely hsa_circ_0007991 and hsa_circ_0067934. Figure 2 displays the expression of two DECs among the four datasets and structural patterns of two DECs. The characteristics of two DECs are described in Table 2.
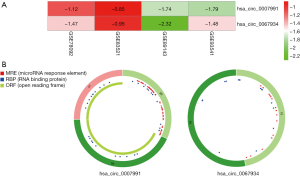
Table 2
CircRNA ID | Position | Strand | Best transcript | Gene symbol | CircRNA study |
---|---|---|---|---|---|
hsa_circ_0007991 | chr1:21329205-21415706 | − | NM_001198801 | EIF4G3 | Jeck2013, Maass2017, Rybak2015, alzman2013 |
hsa_circ_0067934 | chr3:170013698-170015181 | + | NM_002740 | PRKCI | Rybak2015, alzman2013 |
DECs, differently expressed circRNAs.
Identification of miRNAs and genes at the downstream of DECs
As an online web tool that could assist us in obtaining the MREs of circRNAs, the CSCD was utilized to forecast the miRNAs binding to the DECs. As a consequence, a total of 60 miRNAs were discovered at the downstream of hsa_circ_0007991, and 36 miRNAs were selected at the downstream of hsa_circ_0067934. Furthermore, we took advantage of three online resources, miRDB, miRTarBase, and TargetScan, to predict the miRNA-targeted genes. Genes simultaneously existing in three databases and the relevant miRNAs were included in our study.
GO enrichment analysis of DECs
GO enrichment analysis mainly consists of three parts, CC, BP, and MF, and was performed using the DAVID, a powerful online tool. The results are displayed in Figure 3. In the cell composition group, the predicted genes at the downstream of hsa_circ_0007991 were primarily enriched in the nucleus, cytoplasm, and focal adhesion. The predicted genes at the downstream of hsa_circ_0067934 were chiefly enriched in nucleoplasm, nucleus, and cytoplasm. In the MF group, the predicted genes at the downstream of hsa_circ_0007991 were primarily concentrated in poly (A) RNA binding, protein binding, and nucleic acid binding. The predicted genes at the downstream of hsa_circ_0067934 were mainly enriched in protein binding, poly (A) RNA binding, and ubiquitin-protein ligase binding. In the BP group, the predicted genes at the downstream of hsa_circ_0007991 were primarily enriched in regulation of transcription, DNA-templated, transcription, and positive regulation of protein insertion into mitochondrial membrane involved in the apoptotic signaling pathway. The predicted genes at the downstream of hsa_circ_0067934 were mainly enriched in Wnt signaling pathway, calcium modulating pathway, negative regulation of transcription from RNA polymerase II promoter. It illustrated that DECs existed in the nucleus and cytoplasm and could bind to protein and RNA, and therein participated in the regulation of transcription and signaling pathway, ultimately leading to the occurrence of diseases. These results are consistent with other reported conclusions.
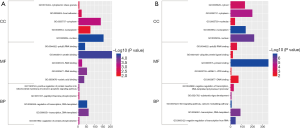
Pathway analysis of DECs
KEGG pathway analysis was conducted with a clusterProfiler package in R software. The results are shown in Figure 4. The signaling pathways of genes at the downstream of hsa_circ_0007991 were involved in two pathways: signaling pathways regulating the pluripotency of stem cells and the p53 signaling pathway. The signaling pathways of genes at the downstream of hsa_circ_0067934 were primarily concentrated in signaling pathways regulating pluripotency of stem cells, autophagy–animal, and FoxO signaling pathway. The results proved that hsa_circ_0007991 and hsa_circ_0067934 had a close relationship with cancer-related pathways and were the initiators of gastric cancer.
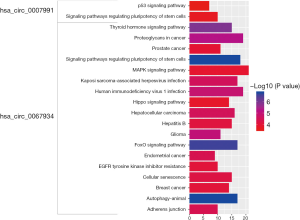
Validation of miRNAs and genes at downstream of hsa_circ_0007991 and hsa_circ_0067934 through the data from TCGA
The miRNAs expression profiles of 42 gastric cancer samples and 410 normal controls were retrieved from TCGA. The expression of genes among 30 gastric cancer samples and 343 normal controls were downloaded from TCGA. All of them were analyzed with edgeR package from the Bioconductor project, independently. Volcano plots are shown in Figure 5. As a result, a total of 338 aberrantly expressed miRNAs were determined. Among them, 104 down-regulated miRNAs and 234 up-regulated miRNAs were included. A total of 7042 differentially expressed genes, containing 2828 down-regulated genes and 4214 up-regulated genes, were detected. To examine whether the predicted miRNAs and genes at the downstream of hsa_circ_0007991 and hsa_circ_0067934 obtained through bioinformatics methods were verified in the experiment, we took the intersection between the predicted miRNAs and genes and the aberrantly expressed miRNAs and genes in the expression data from TCGA. No overlapped miRNAs at the downstream of hsa_circ_0007991 were found, and hsa-mir-4705 at the downstream of hsa_circ_0067934 was identified in both datasets (Figure 5C). Therefore, we took further analysis to study the genes at the downstream of hsa-mir-4705. Among four hsa-mir-4705-targeted genes (TMEM68, BMPR1B, MCFD2, and ASH1L), only BMPR1B was verified in the expression data from TCGA (Figure 5D). In summary, we speculate that there may be a hsa_circ_0067934/hsa-mir-4705/BMPR1B axis, which plays a vital role in the occurrence and development of gastric cancer.
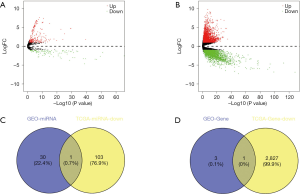
Discussion
Gastric cancer is the third most lethal cancer in the world (1,29), and the most common type of gastric cancer is adenocarcinoma, which can be divided into two subtypes, including intestinal and diffuse types, based on the Lauren classification (30). Also, gastric cancer is a complicated disease attributed to the interaction of environmental and host-associated factors. The biological and genetic characteristics may play leading roles in the initiation and development of gastric cancer (4,31). Reports have revealed that the poor outcome of patients with gastric cancer is mainly caused by the lack of diagnosed approach at the early-stage gastric cancer; thus, the gene diagnosis (32) and targeted therapy (33,34) might represent a new dawn for curing gastric cancer. Over the past few decades, the bioinformatics approach has been widely used to detect potential therapeutic targets for gastric cancer with the boom of microarray and high-throughput sequencing technologies. Trastuzumab, the inhibitor of HER-2, has been demonstrated to be effective in patients with HER2-positive gastric cancer (35,36). However, anti-EGFR and anti-MET/HGF therapies do not remarkably improve the overall survival (OS) in gastric cancer (37-40). The emerging PD-1inhibitors, pembrolizumab and nivolumab may be useful in suppressing the development of gastric cancer (41,42). There is still much room for exploration in the field of targeted therapy for gastric cancer.
As the highlights of cancers in recent years, circRNAs have been held to have the potential to become diagnostic and prognostic biomarkers due to the highly conserved and stable loop structure and tissue-specificity (11,43,44). An abundance of research has illustrated the importance of circRNAs in the progression of cancers. For instance, Han et al. discovered that circMTO1 is down-regulated in HCC tissues and can repress the development of HCC by sponging miR-9 to increase p21 expression (45). A recent publication in the Journal of Hepatology discovered that cSMARCA5 behaved as a tumor suppressor in HCC, and was able to interact with miR-17-3p and miR-181b-5p to promote the expression of an anti-oncogene called TIMP3 (46). Furthermore, circ-SHPRH could code tumor suppressor protein, SHPRH-146aa, to regulate the progression of glioblastomas (47). Based on existing research results, we concluded that circRNAs were critical molecules to understand the underlying mechanism of carcinoma progression.
In our study, four expression profiles (GSE78092, GSE83521, GSE89143, and GSE93541) were retrieved from the GEO database and integrated to analyze the aberrantly expressed circRNAs using the RobustRankAggreg algorithm. Through integrated analysis, two circRNAs, namely hsa_circ_0007991 and hsa_circ_0067934, were screened, and all of them were dysregulated in gastric cancer tissue. According to the theory of MREs, we utilized CSCD, a web tool, to predict the miRNAs interacting with DECs. Sixty miRNAs were forecasted at the downstream of hsa_circ_0007991, and 36 miRNAs were hsa_circ_0067934-targeted. Then, three online resources, miRDB, miRTarBase, and TargetScan, were combined to predict the miRNA-targeted genes. Genes simultaneously existed in three databases and relevant miRNAs were included in our study. Forty miRNAs and 371 genes were determined at the downstream of hsa_circ_0007991, and 31miRNAs and 530 genes were predicted at the downstream of hsa_circ_0067934.
Furthermore, to understand the functions of hsa_circ_0007991 and hsa_circ_0067934 in gastric cancer, we performed the GO and KEGG analyses. CircRNA hsa_circ_0007991 acted on genes enriched in the nucleus and cytoplasm. These genes could bind to RNA, protein, and nucleic acid, and regulated transcription and protein insertion into mitochondrial membrane involved in the apoptotic signaling pathway, leading to abnormal signal transductions of signaling pathways regulating pluripotency of stem cells and p53 signaling pathway.
Similarly, the hsa_circ_0067934-targeted genes were concentrated in the nucleoplasm, nucleus, and cytoplasm, and interacted with RNA, protein, and ubiquitin-protein ligase. Finally, it could have obstructed the signal conduction of the Wnt signaling pathway and calcium modulating pathway. This may be a potent mechanism in gastric cancer. These discoveries indicate that hsa_circ_0007991 and hsa_circ_0067934 may be pivotal molecules in the development mechanism of gastric cancer and could be a potential target for gastric cancer.
To ascertain the expression of predicted miRNAs and genes at the downstream of DECs, we obtained the expression profiles of miRNAs and mRNAs from TCGA database. The differently expressed analyses were performed separately by edgeR package in R software. In total, 104 down-regulated miRNAs and 234 up-regulated miRNAs were identified. A total of 7,042 differentially expressed genes, including 2,828 down-regulated genes and 4,214 up-regulated genes, were detected. Subsequently, we obtained overlapped miRNAs and genes in the predicted results and outputs from TCGA database by the intersection method. The predicted miRNAs at the downstream of hsa_circ_0007991 were not found in expression data from TCGA.
Nevertheless, hsa-mir-4705, a hsa_circ_0067934-targeted miRNA, was overlapped in two datasets, and the expression of it in data from TCGA was down-regulated. Accordingly, we took hsa-mir-4705 as the focus. BMPR1B, one of the four dysregulated genes (TMEM68, BMPR1B, MCFD2, and ASH1L) at the downstream of hsa_circ_0067934 was confirmed in DEGs from TCGA database. As mentioned above, we deduced that hsa_circ_0067934 could regulate the expression of BMPR1B by interacting with hsa-mir-4705, and all of them functioned as a tumor suppressor in gastric cancer. In other words, there may be a hsa_circ_0067934/hsa-mir-4705/BMPR1B axis to regulate the occurrence, progression, and prognosis of gastric cancer. To study the potential pathway related to the hsa_circ_0067934/hsa-mir-4705/BMPR1B axis, we researched the predicted pathways that BMPR1B at the downstream of hsa_circ_0067934 participated in. Three pathways, including signaling pathways regulating pluripotency of stem cells, the Hippo signaling pathway, and axon guidance, were included. Among them, signaling pathways regulating the pluripotency of stem cells and the Hippo signaling pathway was involved with carcinomas (Figure 6). This implies that the hsa_circ_0067934/hsa-mir-4705/BMPR1B axis regulated the differentiation of gastric cells and the progression of gastric cancer by impacting signaling pathways regulating pluripotency of stem cells and Hippo signaling pathway.
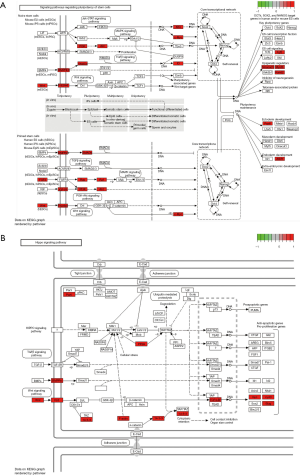
A previous study had reported that mechanisms mediated by circRNAs are liable for the progression of gastric cancer (48). hsa_circ_002059 (49) and hsa_circ_0014717 (50) were found significantly dysregulated in gastric cancer tissues and could be detected in body fluid. In other analyses, the low expression of hsa_circ_0001649 (51), hsa_circ_104916 (52), and hsa_circ_100269 (53) were closely related to the development of gastric cancer. As for hsa_circ_0067934 in our study, Xia et al. demonstrated that hsa_circ_0067934 is up-regulated in ESCC and promoted the proliferation of ESCC cells. The reason why the result in the study of Xia et al. is contrary to our conclusion might be that circRNAs are tissue-specific (54). In the same way, the hsa_circ_0067934 could also be detected in plasma (the samples of gastric cancer were plasma in the GSE93541 dataset) and have a low expression.
Consequently, we inferred that hsa_circ_0067934 might be a novel biomarker and therapeutic target in gastric cancer. The hsa-mir-4705 was firstly discovered in breast cancer through the next-generation sequencing approach (55). Not much is known about how hsa-mir-4705 regulates the progression of carcinoma. Our study disclosed that the dysregulated hsa-mir-4705 suppressed the expression of BMPR1B in gastric cancer.
Further experiments are needed to illustrate the role of hsa-mir-4705 in gastric cancer. BMPR1B, as the target of hsa-mir-4705, is a transmembrane serine-threonine kinase receptor among the bone morphogenetic protein (BMP) receptor family (56). The responsibility of it is to transduce the BMPs signal by binding to transforming growth factor-β (TGF-β) and activin (57). Recent studies indicated that BMPR1B acted as an oncogenesis factor, and the declined expression of it was highly connected with the poor prognosis of breast cancer (58,59). These discoveries are consistent with our results. Chapellier et al. demonstrated the vital role of BMPRIB in the initiation of stem cell transformation (60). The Hippo pathway is conserved, and the pivotal signaling pathway functions as a regulator to modulate cell proliferation, differentiation, and apoptosis, leading to the alteration of tissue growth and homeostasis. The processes are mainly mediated by YAP and TAZ. Moreover, BMPRIB can possibly integrate with the Hippo pathway through crossing talks with TGF-β signaling pathways (61). Evidence is available that elucidates the connections between BMPRIB and signaling pathways regulating the pluripotency of stem cells and the Hippo pathway.
Conclusions
In summary, we detected two DECs, hsa_circ_0007991, and hsa_circ_0067934, which were involved in the progression mechanisms of gastric cancer and could be novel diagnostic or prognostic markers, as well as therapeutic targets for gastric cancer. Also, a hsa_circ_0067934/hsa-mir-4705/BMPR1B regulatory axis was identified in gastric cancer. No matter which molecules among hsa_circ_0067934, hsa-mir-4705, or BMPR1B were abnormally expressed, the signal transduction of signaling pathways regulating pluripotency of stem cells and Hippo pathway would be disturbed and accompany the occurrence and progression of gastric cancer. This may be one of the underlying mechanisms by which gastric cancer develops. Indeed, the absence of experiments is the most significant limitation in our study. We do realize the problem, and further experiments are on the schedule. We will knock down the hsa_circ_0067934 in the normal gastric cell line to examine whether the changes of hsa-mir-4705 and BMPR1B expression are accordant with the results we predicted in this study. More functional assays will also be conducted to validate the effects of the hsa_circ_0067934/hsa-mir-4705/BMPR1B regulatory axis on the biological behavior of gastric cancer cells.
Acknowledgments
Funding: None.
Footnote
Conflicts of Interest: All authors have completed the ICMJE uniform disclosure form (available at http://dx.doi.org/10.21037/tcr.2019.10.32). The authors have no conflicts of interest to declare.
Ethical Statement: The authors are accountable for all aspects of the work in ensuring that questions related to the accuracy or integrity of any part of the work are appropriately investigated and resolved.
Open Access Statement: This is an Open Access article distributed in accordance with the Creative Commons Attribution-NonCommercial-NoDerivs 4.0 International License (CC BY-NC-ND 4.0), which permits the non-commercial replication and distribution of the article with the strict proviso that no changes or edits are made and the original work is properly cited (including links to both the formal publication through the relevant DOI and the license). See: https://creativecommons.org/licenses/by-nc-nd/4.0/.
References
- Bray F, Ferlay J, Soerjomataram I, et al. Global cancer statistics 2018: GLOBOCAN estimates of incidence and mortality worldwide for 36 cancers in 185 countries. CA Cancer J Clin 2018;68:394-424. [Crossref] [PubMed]
- Van Cutsem E, Sagaert X, Topal B, et al. Gastric cancer. Lancet 2016;388:2654-64. [Crossref] [PubMed]
- Fornaro L, Vasile E, Aprile G, et al. Locally advanced gastro-oesophageal cancer: Recent therapeutic advances and research direc-tions. Cancer Treat Rev 2018;69:90-100. [Crossref] [PubMed]
- Smyth EC, Verheij M, Allum W, et al. Gastric cancer: ESMO Clinical Practice Guidelines for diagnosis, treatment and follow-up†. Ann Oncol 2016;27:v38-v49. [Crossref] [PubMed]
- Cocquerelle C, Mascrez B, Hetuin D, et al. Mis-splicing yields circular RNA molecules. FASEB J 1993;7:155-60. [Crossref] [PubMed]
- Sanger HL, Klotz G, Riesner D, et al. Viroids are single-stranded covalently closed circular RNA molecules existing as highly base-paired rod-like structures. Proc Natl Acad Sci U S A 1976;73:3852-6. [Crossref] [PubMed]
- Salzman J, Gawad C, Wang PL, et al. Circular RNAs are the predominant transcript isoform from hundreds of human genes in di-verse cell types. PLoS One 2012;7:e30733. [Crossref] [PubMed]
- Memczak S, Jens M, Elefsinioti A, et al. Circular RNAs are a large class of animal RNAs with regulatory potency. Nature 2013;495:333. [Crossref] [PubMed]
- Szabo L, Salzman J. Detecting circular RNAs: bioinformatic and experimental challenges. Nat Rev Genet 2016;17:679. [Crossref] [PubMed]
- Kristensen LS, Hansen T, Venø M, et al. Circular RNAs in cancer: opportunities and challenges in the field. Oncogene 2018;37:555. [Crossref] [PubMed]
- Patop IL, Kadener S. circRNAs in Cancer. Curr Opin Genet Dev 2018;48:121-7. [Crossref] [PubMed]
- Nair AA, Niu N, Tang X, et al. Circular RNAs and their associations with breast cancer subtypes. Oncotarget 2016;7:80967. [Crossref] [PubMed]
- Bachmayr-Heyda A, Reiner AT, Auer K, et al. Correlation of circular RNA abundance with proliferation–exemplified with colorectal and ovarian cancer, idiopathic lung fibrosis, and normal human tissues. Sci Rep 2015;5:8057. [Crossref] [PubMed]
- Ahmed I, Karedath T, Andrews SS, et al. Altered expression pattern of circular RNAs in primary and metastatic sites of epithelial ovarian carcinoma. Oncotarget 2016;7:36366. [Crossref] [PubMed]
- Qin M, Liu G, Huo X, et al. Hsa_circ_0001649: a circular RNA and potential novel biomarker for hepatocellular carcinoma. Cancer Biomark 2016;16:161-9. [Crossref] [PubMed]
- Barrett T, Troup DB, Wilhite SE, et al. NCBI GEO: mining tens of millions of expression profiles—database and tools update. Nucleic Acids Res 2007;35:D760-5. [Crossref] [PubMed]
- Ritchie ME, Phipson B, Wu D, et al. limma powers differential expression analyses for RNA-sequencing and microarray studies. Nucleic Acids Res 2015;43:e47. [Crossref] [PubMed]
- Kolde R, Laur S, Adler P, et al. Robust rank aggregation for gene list integration and meta-analysis. Bioinformatics 2012;28:573-80. [Crossref] [PubMed]
- Xia S, Feng J, Chen K, et al. CSCD: a database for cancer-specific circular RNAs. Nucleic Acids Res 2018;46:D925-9. [Crossref] [PubMed]
- Wong N, Wang X. miRDB: an online resource for microRNA target prediction and functional annotations. Nucleic Acids Res 2015;43:D146-52. [Crossref] [PubMed]
- Chou CH, Shrestha S, Yang C-D, et al. miRTarBase update 2018: a resource for experimentally validated microRNA-target interac-tions. Nucleic Acids Res 2018;46:D296-302. [Crossref] [PubMed]
- Lewis BP, Burge CB, Bartel DP. Conserved seed pairing, often flanked by adenosines, indicates that thousands of human genes are microRNA targets. Cell 2005;120:15-20. [Crossref] [PubMed]
- Dennis G, Sherman BT, Hosack DA, et al. DAVID: database for annotation, visualization, and integrated discovery. Genome Biol 2003;4:3. [Crossref] [PubMed]
- Yu G, Wang L-G, Han Y, et al. clusterProfiler: an R package for comparing biological themes among gene clusters. OMICS 2012;16:284-7. [Crossref] [PubMed]
- Deng M, Brägelmann J, Schultze JL, et al. Web-TCGA: an online platform for integrated analysis of molecular cancer data sets. BMC Bioinformatics 2016;17:72. [Crossref] [PubMed]
- BITGDA C. Analysis-ready standardized TCGA data from Broad GDAC Firehose 2016_01_28 run. Broad Institute of MIT and Harvard. Dataset 2016;
- Robinson MD, McCarthy DJ, Smyth GK. edgeR: a Bioconductor package for differential expression analysis of digital gene expression data. Bioinformatics 2010;26:139-40. [Crossref] [PubMed]
- McCarthy DJ, Chen Y, Smyth GK. Differential expression analysis of multifactor RNA-Seq experiments with respect to biological variation. Nucleic Acids Res 2012;40:4288-97. [Crossref] [PubMed]
- Venerito M, Vasapolli R, Rokkas T, et al. Gastric cancer: epidemiology, prevention, and therapy. Helicobacter 2018;23:e12518. [Crossref] [PubMed]
- Cancer Genome Atlas Research Network. Comprehensive molecular characterization of gastric adenocarcinoma. Nature 2014;513:202. [Crossref] [PubMed]
- Tan P, Yeoh KG. Genetics and molecular pathogenesis of gastric adenocarcinoma. Gastroenterology 2015;149:1153-62.e3. [Crossref] [PubMed]
- Kahroba H, Hejazi MS, Samadi N. Exosomes: from carcinogenesis and metastasis to diagnosis and treatment of gastric cancer. Cell Mol Life Sci 2019;76:1747-58. [Crossref] [PubMed]
- Duarte HO, Gomes J, Machado JC, et al. Gastric cancer: Basic aspects. Helicobacter 2018;23:e12523. [Crossref] [PubMed]
- Kumar V, Soni P, Garg M, et al. Emerging Therapies in the Management of Advanced-Stage Gastric Cancer. Front Pharmacol 2018;9:404. [Crossref] [PubMed]
- Bartley AN, Washington MK, Colasacco C, et al. HER2 Testing and Clinical Decision Making in Gastroesophageal Adenocarcinoma: Guideline From the College of American Pathologists, American Society for Clinical Pathology, and the American Society of Clinical Oncology. J Clin Oncol 2017;35:446-64. [Crossref] [PubMed]
- Ock CY, Lee KW, Kim JW, et al. Optimal patient selection for trastuzumab treatment in HER2-positive advanced gastric cancer. Clin Cancer Res 2015;21:2520-9. [Crossref] [PubMed]
- Waddell T, Chau I, Cunningham D, et al. Epirubicin, oxaliplatin, and capecitabine with or without panitumumab for patients with previously untreated advanced oesophagogastric cancer (REAL3): a randomised, open-label phase 3 trial. Lancet Oncol 2013;14:481-9. [Crossref] [PubMed]
- Lordick F, Kang YK, Chung HC, et al. Capecitabine and cisplatin with or without cetuximab for patients with previously untreated advanced gastric cancer (EXPAND): a randomised, open-label phase 3 trial. Lancet Oncol 2013;14:490-9. [Crossref] [PubMed]
- Cunningham D, Tebbutt NC, Davidenko I, et al. Phase III, randomized, double-blind, multicenter, placebo (P)-controlled trial of rilotumumab (R) plus epirubicin, cisplatin and capecitabine (ECX) as first-line therapy in patients (pts) with advanced MET-positive (pos) gastric or gastroesophageal junction (G/GEJ) cancer: RILOMET-1 study. J Clin Oncol 2015;33:4000. [Crossref]
- Shah MA, Bang YJ, Lordick F, et al. METGastric: A phase III study of onartuzumab plus mFOLFOX6 in patients with metastatic HER2-negative (HER2-) and MET-positive (MET+) adenocarcinoma of the stomach or gastroesophageal junction (GEC). J Clin Oncol 2015;33:4012. [Crossref]
- Le DT, Bendell JC, Calvo E, et al. Safety and activity of nivolumab monotherapy in advanced and metastatic (A/M) gastric or gastroesophageal junction cancer (GC/GEC): results from the CheckMate-032 study. J Clin Oncol 2016;34:6. [Crossref]
- Muro K, Chung HC, Shankaran V, et al. Pembrolizumab for patients with PD-L1-positive advanced gastric cancer (KEY-NOTE-012): a multicentre, open-label, phase 1b trial. Lancet Oncol 2016;17:717-26. [Crossref] [PubMed]
- Chen Y, Li C, Tan C, et al. Circular RNAs: a new frontier in the study of human diseases. J Med Genet 2016;53:359-65. [Crossref] [PubMed]
- Hsiao KY, Lin YC, Gupta SK, et al. Noncoding effects of circular RNA CCDC66 promote colon cancer growth and metastasis. Cancer Res 2017;77:2339-50. [Crossref] [PubMed]
- Han D, Li J, Wang H, et al. Circular RNA circMTO1 acts as the sponge of microRNA-9 to suppress hepatocellular carcinoma progression. Hepatology 2017;66:1151-64. [Crossref] [PubMed]
- Yu J, Xu QG, Wang ZG, et al. Circular RNA cSMARCA5 inhibits growth and metastasis in hepatocellular carcinoma. J Hepatol 2018;68:1214-27. [Crossref] [PubMed]
- Begum S, Yiu A, Stebbing J, et al. Novel tumour suppressive protein encoded by circular RNA, circ-SHPRH, in glioblastomas. Oncogene 2018;37:4055-7. [Crossref] [PubMed]
- Lei B, Tian Z, Fan W, et al. Circular RNA: a novel biomarker and therapeutic target for human cancers. Int J Med Sci 2019;16:292-301. [Crossref] [PubMed]
- Li P, Chen S, Chen H, et al. Using circular RNA as a novel type of biomarker in the screening of gastric cancer. Clin Chim Acta 2015;444:132-6. [Crossref] [PubMed]
- Shao Y, Li J, Lu R, et al. Global circular RNA expression profile of human gastric cancer and its clinical significance. Cancer Med 2017;6:1173-80. [Crossref] [PubMed]
- Li WH, Song YC, Zhang H, et al. Decreased expression of Hsa_circ_00001649 in gastric cancer and its clinical significance. Dis Markers 2017;2017:4587698. [Crossref] [PubMed]
- Li J, Zhen L, Zhang Y, et al. Circ-104916 is downregulated in gastric cancer and suppresses migration and invasion of gastric cancer cells. Onco Targets Ther 2017;10:3521. [Crossref] [PubMed]
- Zhang Y, Liu H, Li W, et al. CircRNA_100269 is downregulated in gastric cancer and suppresses tumor cell growth by targeting miR-630. Aging (Albany NY) 2017;9:1585. [Crossref] [PubMed]
- Xia W, Qiu M, Chen R, et al. Circular RNA has_circ_0067934 is upregulated in esophageal squamous cell carcinoma and promoted proliferation. Sci Rep 2016;6:35576. [Crossref] [PubMed]
- Persson H, Kvist A, Rego N, et al. Identification of new microRNAs in paired normal and tumor breast tissue suggests a dual role for the ERBB2/Her2 gene. Cancer Res 2011;71:78-86. [Crossref] [PubMed]
- Hardwick JC, Van Den Brink GR, Bleuming SA, et al. Bone morphogenetic protein 2 is expressed by, and acts upon, mature epithelial cells in the colon. Gastroenterology 2004;126:111-21. [Crossref] [PubMed]
- ten Dijke P, Yamashita H, Ichijo H, et al. Characterization of type I receptors for transforming growth factor-beta and activin. Science 1994;264:101-4. [Crossref] [PubMed]
- Dai K, Qin F, Zhang H, et al. Low expression of BMPRIB indicates poor prognosis of breast cancer and is insensitive to taxane-anthracycline chemotherapy. Oncotarget 2016;7:4770. [PubMed]
- Bokobza SM, Ye L, Kynaston HE, et al. Reduced expression of BMPR-IB correlates with poor prognosis and increased proliferation of breast cancer cells. Cancer Genomics Proteomics 2009;6:101-8. [PubMed]
- Chapellier M, Bachelard-Cascales E, Schmidt X, et al. Disequilibrium of BMP2 levels in the breast stem cell niche launches epithelial transformation by overamplifying BMPR1B cell response. Stem Cell Reports 2015;4:239-54. [Crossref] [PubMed]
- Yu FX, Meng Z, Plouffe SW, et al. Hippo pathway regulation of gastrointestinal tissues. Annu Rev Physiol 2015;77:201-27. [Crossref] [PubMed]