Pancreatic cancer differential methylation atlas in blood, peri-carcinomatous and diseased tissue
Introduction
Pancreatic cancer is more common in elderly persons than in younger persons, and less than 20% of patients present with localized, potentially curable tumors (1). The estimated incidence of pancreatic cancer in the United States was 37,700 cases, and an estimated 34,300 patients died from the disease in 2008. The overall 5-year survival rate among patients with pancreatic cancer is <5% (2). Several environmental factors have been implicated, but evidence of a causative role exists only for tobacco use. The risk of pancreatic cancer in smokers is 2.5 to 3.6 times that in nonsmokers (3) Some studies have shown an increased incidence of pancreatic cancer among patients with a history of diabetes or chronic pancreatitis, and there is also evidence that chronic cirrhosis, a high-fat, high-cholesterol diet, and previous cholecystectomy are associated with an increased incidence (4,5).
Presently, there is no valid diagnostic marker for pancreatic cancer. Carbohydrate antigen 19-9 (CA 19-9) levels are elevated in pancreatic cancer but frequently only in advanced disease. It can also be elevated in other cancers, chronic pancreatitis, and autoimmune diseases such as rheumatoid arthritis. Approximately 10% of the population lacks expression of Lewis antigen, which is required to produce CA 19-9. Furthermore, CA 19-9 is used in a clinical setting based on response to treatment (6,7). Up to now, a combination of complex and advanced imaging modalities, such as positron emission tomography scanning, 3-phase computed tomography scanning, endoscopic ultrasound, laparoscopic ultrasound, endoscopic retrograde cholangiopancreatography, and trans-abdominal ultrasound, are necessary for the diagnosis of pancreatic cancer. However, several of these methods are invasive and thus risk complications. Consequently, a minimally or noninvasive marker for pancreatic cancer is urgently needed.
Epigenetics is defined as the study of mitotically or meiotically heritable variations in gene function that cannot be explained by changes in DNA sequence (8). Epigenetic modifications, such as DNA promoter hypermethylation, are known to be aspects of early carcinogenesis and have shown significant potential in the development of a useful diagnostic marker (9,10). Recently, attention to its role in pancreatic cancer has recently increased. DNA methylation has gained much recent interest for its role in cancer biology. Aberrant patterns of DNA methylation can be associated with carcinogenesis and affect the regulation of genome stability and gene transcription (11). Genome wide studies of CpG islands have uncovered thousands of loci where differential methylation can segregate pancreatic tumor tissue from normal tissue (12).
Cancer-linked global genomic hypomethylation in tumor tissue is a common characteristic in a wide variety of malignancies, ranging from solid tumors, such as breast, colon, oral, and lung cancers, to cancers of the blood (13,14). In this study, in order to identify candidate liquid biopsy methylation markers in pancreatic cancer, we have employed a global methylation profiling platform to comprehensively survey a large scale of CpG sites between blood and cancer tissues versus pericarcinous tissues. We compared pericarcinous tissues vs. cancer tissue and blood vs. pericarcinous tissues in order to harvest methylation markers for diagnostic purposes. These genes could be the most likely candidate methylation markers for future liquid biopsies in pancreatic cancer.
Methods
Subjects
Six patients with pancreatic cancer (2 males and 4 females, mean age: 58.83±14.95 y), without radiation, chemotherapy and immunotherapy treatment, were recruited from the Chinese General Hospital of PLA in China (Table S1). The diagnosis of pancreatic cancer was made by at least two experienced oncologists. Sample collection was carried out accorded to the following criteria: (I) the minimum diameter of tumor was greater than 2 cm. Meanwhile, pancreatic cancer was identified by Hematoxylin and Eosin (H&E) staining and the ratio of cancer cells in the whole cells section was over 80%. (II) Tissue adjacent to cancer was collected as far as possible from the cancer tissue in order to avoid the mistake sampling. (III) Blood samples were collected before surgery. Pancreatic cancer tissue and tissue adjacent to cancer of each patient were collected and stored in liquid nitrogen immediately for DNA extraction. All specimens were subjected to autolysis for 4 to 8 h and then snap-frozen at −80 °C until use in analysis. DNA was extracted from 25 mg samples of the tissue specimens using the QIAamp DNA Mini Kit (Qiagen) according to the manufacturer's instructions. gDNA of Blood samples were extracted by FitAmp™ Plasma/Serum DNA Isolation Kit (Epigentek, USA) according to the manufacturer's instructions. The DNA yield and purity were determined spectrophotometrically (NanoDrop® ND1000; Thermo Fisher Scientific Inc., Waltham, MA, USA) and by gel electrophoresis, respectively. DNA of sample was stored at −20 °C for further study. This study was approved by the Ethics Committee of Chinese General Hospital of PLA (No. S2018-013-02). All patients provided signed informed consent.
DNA methylation methods
Bisulfite conversion of 500 ng genomic DNA was performed using the EZ DNA methylation kit (Zymo Research). DNA methylation level was assessed according to the manufacturer’s instructions using Infinium-HumanMethylation450 Beadchips (Illumina Inc.). The technical schemes, the accuracy, and the high reproducibility of this array have been described previously (15). Quantitative measurements of DNA methylation were determined for 485,577 CpG dinucleotides, which covered 99% of the RefSeq genes and were distributed across the whole gene regions, including promoter, gene body, and 30-untranslated regions (UTRs). They also covered 96% of CGIs from the UCSC database with additional coverage in CGI shores (0–2 kb from CGI) and CGI shelves (2–4 kb from CGI). Detailed information on the contents of the array is available in the Infinium HumanMethylation450 User Guide and Human-Methylation 450 manifest (www.illumina.com) and in recent papers (16). DNA methylation data were analyzed with the methylation analysis module within the BeadStudio software (Illumina Inc.). DNA methylation status of the CpG sites was calculated as the ratio of the signal from a methylated probe relative to the sum of both methylated and unmethylated probes. This value, known as b, ranges from 0 (completely unmethylated) to 1 (fully methylated). Given the batch effects normally associated with this platform and especially for small sample sizes as in the current study, we performed batch effect correction as described previously (17). For intra-chip normalization of probe intensities, colored balance and background corrections in every set of ten samples from the same chip were performed using internal control probes. X chromosome CpG sites in the CGIs in the AR gene in this array as well as the internal control probes were checked to validate the DNA methylation measurements.
Bioinformatics
GO enrichment analysis was performed using GOEAST (http://omicslab.genetics.ac.cn/GOEAST/index.php). Hypergeometric distribution was used to calculate the P value of GOID enrichment, and P<1E−4 cut-off value was applied (18). The graph size was reduced by condensing non-significant nodes to points. The smaller the P value is, the more significant the GO term is enriched in the dataset. And the graph size was reduced by condensing non-significant nodes to points. KEGG is a database resource for understanding high-level functions and utilities of the biological system, such as the cell, the organism and the ecosystem, from molecular-level information, especially large-scale molecular datasets generated by genome sequencing and other high-through put experimental technologies (http://www.genome.jp/kegg/). We used KOBAS software to test the statistical enrichment of differentially methylated genes in KEGG pathways.
Results
Overlap of differential DNA methylation sites between pericarcinous tissues vs. cancer tissue and blood vs. pericarcinous tissues
DNA methylation levels were compared between four pericarcinous tissues (B) vs. six pancreatic cancer tissues (C) and six blood samples (A) vs. four pericarcinous tissues (B) using Infinium HumanMethylation450 Bead Chips (Table 1). Sites simultaneously present in B versus C and A versus B group comparisons were group defined as hypermethylation sites. Same was done to define hypomethylation sites. Meanwhile, hypomethylation sits simultaneously existed in B vs. C group and A vs. B group was defined as hypomethylation sits. Of 485,577 CpG sites, significant diagnostic differences in DNA methylation were observed at 15,397 CpG sites representing 7,440 genes at FDR 5% correction (Figure 1 and http://fp.amegroups.cn/cms/9614487675fcbfcb574c6af25b586775/tcr.2019.11.26-1.pdf). Of these sites, 5,605 (36.4%, 5,605 of 15,397) CpG sites were hypomethylated and 5,870 (38.12%, 5,870 of 15,397) CpG sites were hypermethylated. Functional distribution of 5,870 hypermethylated CpG sites suggested that 47.4% of these sites were located in promoter regions, 38.86% of these sites were located in gene bodies, 12.42% of these sites were located in intergenic regions and 6.01% of these sites were located in the 3’-untranslated regions (UTRs). Furthermore, sublocation analysis of 2,659 CpG sites in promoter region with hypermethylated indicated that 31.74% of these sites were located in regions from −200 to −1,500 nt upstream of the transcription start site (TSS1500), 28.43% of these sites were located in regions from −200 nt upstream to the TSS itself (TSS200), 27.15% of these sites were located in 1st Exon regions and 12.67% of these sites were located in the 5’-untranslated regions (UTRs). These hypermethylated CpG sites were mostly located in gene bodies and promoter regions. Meanwhile, Functional distribution of 5,605 hypomethylated CpG sites suggested that 20.43% of these sites were located in promoter regions, 39.64% of these sites were located in gene bodies, 36.24% of these sites were located in intergenic regions and 3.69% of these sites were located in 3’UTR regions. Furthermore, sublocation analysis of 5,605 hypomethylated CpG sites in promoter regions indicated that 48.38% of these sites were located in TSS1500 regions, 15.46% of these sites were located in TSS200 regions, 11.35% of these sites were located in 1st Exon regions and 24.8% of these sites were located in 5’UTR regions. These hypomethylated CpG sites were mostly located in gene bodies, promoter regions and intergenic regions. The results above seem to be in apparent contradiction to widely held belief that promoter hypomethylation is correlated to increased transcription and vice versa. This also indicates the possibility that transcription factors are modified which dictate their regulation of anomalous transcription in the cancer cells.
Table 1
Patient | Age | A (blood) | B (pericarcinous tissue) | C (pancreatic cancer tissue) |
---|---|---|---|---|
Patient 1 (F) | 74 | A1& | B1& | C1& |
Patient 2 (M) | 36 | A2& | B2* | C2& |
Patient 3 (M) | 66 | A3& | B3& | C3& |
Patient 4 (F) | 60 | A4& | B4* | C4& |
Patient 5 (F) | 46 | A5& | B5& | C5& |
Patient 6 (F) | 71 | A6& | B6& | C6& |
&, represents qualified sample; *, represents unqualified samples. F, female; M, male.
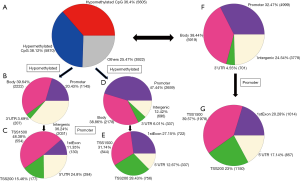
Because the 15,397 methylated CpG sites corresponded to 7,440 genes, some of the methylated genes must contain more than one methylated site. Further analysis showed that among the 7,440 methylated genes, 4,962 (67%) possessed only one methylated site, 1,590 (21%) contained two methylated sites, and 888 (12%) contained three or more methylated sites (Figure 2 and http://fp.amegroups.cn/cms/3adcaa480666f581911c4ab936783571/tcr.2019.11.26-2.pdf). In particular, one methylated gene (PTPRN2) possessed 40 methylated sites in overlap. Meanwhile, the MAD1 mitotic arrest deficient-like 1 (yeast) (MAD1L1, ENSG00000002822) possessed over 25 methylated sites (Figure 3). Of note, number of methylation sites can be correlated to gene length and mere presence of more methylation sites does not mean increased methylation-based regulation. Instead, methylation sites normalized over gene length is a better indicator of propensity to regulation by methylation.
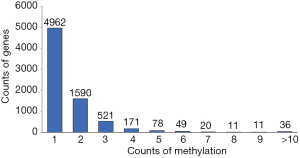
Gene Ontology (GO) and KEGG pathway analysis of differentially methylated genes in overlap group
In order to improve the credibility of this research, the genes with counts of methylation sites were equal or greater than 15 were selected to perform intensive study. After such screening, 10 genes with more than three counts of differentially methylated CpG sites were harvested. GO terms were further assigned to Homo sapiens differentially methylated genes based on their sequence similarities to known proteins in the UniProt database annotated with GO terms as well as InterPro and Pfam domains they contain. GO annotation and enrichment analysis of ten significantly differentially methylated genes was implemented by GOEAST software (http://omicslab.genetics.ac.cn/GOEAST/index.php), in which gene length bias was corrected. GO terms with corrected P value less than 10-4 were considered significantly enriched (Figure 4). Biological processes, cellular components, and molecular functions are shown in Figure 4 and Table S2. From the perspective of biological processes, there are 75 GO terms were assigned under this catalogues. Among these terms, spindle checkpoint (GO: 0031577, P value: 8.98E−21), mitotic spindle assembly checkpoint (GO: 0007094, P value: 3.5E−21) and negative regulation of mitotic sister chromatid segregation (GO: 0033048, P value: 3.5E−21) were the top three significantly enriched terms. From the cellular component perspective, there are 3 GO terms were assigned under this catalogues. Among these terms, A band (GO: 0031672, P value: 1.1E−05) was the top significantly enriched terms. From the molecular function perspective, there are 4 GO terms were assigned under this catalogues. Among these terms, G-protein beta/gamma-subunit complex binding (GO: 0031683, P value: 2.4E−11) was the top significantly over-represented terms.
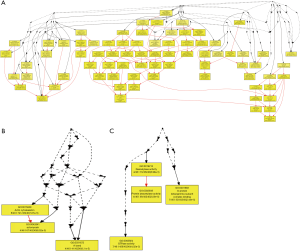
In vivo, various biological functions were implemented by cooperation of different genes. Pathways enrichment analysis can give some clues to the biochemical and signal transduction pathways that differentially expressed genes may participate in. KEGG is a database resource for understanding high-level functions and utilities of the biological system, such as the cell, the organism and the ecosystem, from molecular-level information, especially large-scale molecular datasets generated by genome sequencing and other high-through put experimental technologies (http://www.genome.jp/kegg/). We used KOBAS software to test the statistical enrichment of differentially methylated genes in KEGG pathways (19). In this study, ten significantly differentially methylated genes involve 52 pathways (Table S3). It was worthy noticed that 43 pathways owned the same corrected P value (0.31). Table S3 shows the results of pathways enrichment, it clearly displays that vibrio cholerae infection were the top enriched term. Two differentially methylated genes that identified in our study participate in this pathway. Moreover, it is worth noting that pancreatic secretion, type I diabetes mellitus, Insulin secretion and Adrenergic signaling in cardiomyocytes were also significant enriched in this study. The pathways mentioned above were adopted with the function that pancreas played.
GO and KEGG pathway analysis of differentially methylated sites located in promoter regions of genes in overlap group
The promoter contains specific DNA sequences that are recognized by proteins known as transcription factors. These factors bind to the promoter sequences, recruiting RNA polymerase, the enzyme that synthesizes the RNA from the coding region of the gene. Eukaryotic promoters are extremely diverse and are difficult to characterize. They typically lie upstream of the gene and can have regulatory elements several kilobases away from the transcriptional start site. In eukaryotes, the transcriptional complex can cause the DNA to bend back on itself, which allows for placement of regulatory sequences far from the actual site of transcription. Many eukaryotic promoters, contain a TATA box (sequence TATAAA), which in turn binds a TATA binding protein which assists in the formation of the RNA polymerase transcriptional complex. Of this study, we identified 4,999 differentially methylated sites located in promoter regions in overlap group (http://fp.amegroups.cn/cms/24bc751fdb7f41b7ce54e74bde803221/tcr.2019.11.26-3.pdf). Moreover, we picked out 30 genes with significantly hypermethylation and 30 genes with significantly hypomethylation in the overlap group (Figure 5 and Table S4). GO and KEGG analysis were performed with these 60 aberrant methylation genes. Of the GO analysis (Figure 6 and Table S5), GO terms with corrected P value less than 10−4 were considered significantly enriched. From the perspective of biological processes, there are three GO terms were assigned under this catalogues. Among these terms, autophagosome assembly (GO: 0000045, P value: 5.8E−05), autophagy (GO: 0006914, P value: 3.8E−10) and autophagosome organization (GO: 1905037, P value: 5.8E−05) were the top three significantly enriched terms. From the cellular component perspective, there are three GO terms were assigned under this catalogues. Among these terms, mitochondrial fatty acid beta-oxidation multienzyme complex (GO: 0016507, P value: 6.4E−07), fatty acid beta-oxidation multienzyme complex (GO: 0036125, P value: 6.4E−07) and glycine cleavage complex (GO: 0005960, P value: 6.4E−07) were the top three significantly enriched terms. From the molecular function perspective, there are five GO terms were assigned under this catalogues. Among these terms, long-chain-3-hydroxyacyl-CoA dehydrogenase activity (GO: 0016509, P value: 6.4E−07) was the top significantly over-represented terms. Of the KEGG analysis (Table S6), it clearly displays that Regulation of autophagy were the top enriched term. Two differentially methylated genes that identified in our study participate in this pathway. Moreover, it is worth noting that Non-small cell lung cancer, Glioma, ErbB signaling pathway and Fc gamma R-mediated phagocytosis were also significant enriched in this study.
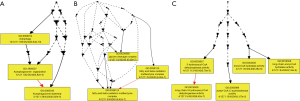
Methylation status of key genes related to pancreatic cancer
To pinpoint the methylation status of pancreatic cancer related genes (Table 2). We check out 22 pancreatic cancer related genes, including ERBB2, AKT1, CDC42, KRAS, RAC1, RALB, RALA, PIK3R3, PIK3R2, AKT2, PLD1, RALBP1, SMAD4, RAF1, SMAD3, SMAD2, RB1, MAPK10, BAD, CDK4, STAT3 and CCND1, which has been reported before. The results indicated that ERBB2, KRAS, PIK3R3, PLD1, RALBP1, RB1 and MAPK10 all showed hypomethylation status. On the contrary, the other genes all showed hypermethylation status. Of note, effect size estimation was not calculated in this case.
Table 2
Series number | Gene | Methylation status | |
---|---|---|---|
Hypermethylation | Hypomethylation | ||
1 | ERBB2 | N/A | Yes |
2 | AKT1 | Yes | N/A |
3 | CDC42 | Yes | N/A |
4 | KRAS | N/A | Yes |
5 | RAC1 | Yes | N/A |
6 | RALB | Yes | N/A |
7 | RALA | Yes | N/A |
8 | PIK3R3 | N/A | Yes |
9 | AKT2 | Yes | N/A |
10 | PIK3R2 | Yes | N/A |
11 | PLD1 | N/A | Yes |
12 | RALBP1 | N/A | Yes |
13 | SMAD4 | Yes | N/A |
14 | RAF1 | Yes | N/A |
15 | SMAD3 | Yes | N/A |
16 | SMAD2 | Yes | N/A |
17 | RB1 | N/A | Yes |
18 | MAPK10 | N/A | Yes |
19 | BAD | Yes | N/A |
20 | CDK4 | Yes | N/A |
21 | STAT3 | Yes | N/A |
22 | CCND1 | Yes | N/A |
Discussion
It is now evident that epigenetic abnormalities are extremely common in cancers, and these abnormalities provide an alternative mechanism of transcriptional silencing. Epigenetic abnormalities in cancer predominantly encompass methylation of CG dinucleotides (CpG islands) in the 5’ regulatory region of tumor suppressor genes, which abrogates RNA polymerase from binding and initiating transcription. In cancers, there is preferential methylation of the gene promoter, but not in the corresponding normal cells within the tissue of origin. Methylome sequencing, without a priori bias to known CpG islands, yielded novel highly discriminant methylation markers for pancreatic cancer. Importantly, these findings were confirmed using an independent sample set of tumor and control tissues, showing that the method used in this study successfully identify pancreatic cancer markers with low background levels. Many of the markers with the strongest association to pancreatic cancer also showed greater than 10-fold increases in the median copies per sample compared with controls; this observation is critical to the application of these markers in diagnostic test development where assays must detect tumor signal against the background biologic milieu. Novel candidates identified by this method were clinically piloted by assay from pancreatic juice, demonstrating utility for the detection of pancreatic cancer in blinded comparisons, even to diseased controls with chronic pancreatitis.
In this study, genome-wide DNA methylation profiling was conducted between four pericarcinous tissues vs. six pancreatic cancer tissues and six blood samples vs. four pericarcinous tissues using Infinium HumanMethyla-tion450 Beadchips. Sampling from pancreatic cancer tissues, pericarcinous tissues and blood of one patient is a useful method for investigating DNA methylation biomarkers without the influence of genetic discordance. Actually, the approach used in this study has identified vagarious epigenetic differences, including non-small cell lung cancer (20), colorectal carcinoma (21) and hepatocellular carcinoma (22), etc. Of this study, a total of 15,397 differentially methylated CpG sites (3.2%, of 485,577 CpG sites,) corresponding 7,440 genes that were identified in overlap. Of these 15,397 CpG sites with significant diagnostic differences in DNA methylation, 5,605 (36.4%, 5,605 of 15,397) CpG sites were hypomethylated and 5,870 (38.12%, 5,870 of 15,397) CpG sites were hypermethylated. Functional distribution of 5,870 hypermethylated CpG sites suggested that 47.4% of these sites were located in promoter regions, 38.86% of these sites were located in gene bodies, 12.42% of these sites were located in intergenic regions and 6.01% of these sites were located in the 3’-untranslated regions (UTRs). Furthermore, sublocation analysis of 2,659 CpG sites in promoter region with hypermethylated indicated that 31.74% of these sites were located in regions from −200 to −1,500 nt upstream of the transcription start site (TSS1500), 28.43% of these sites were located in regions from −200 nt upstream to the TSS itself (TSS200), 27.15% of these sites were located in 1st Exon regions and 12.67% of these sites were located in the 5’-untranslated regions (UTRs). These hypermethylated CpG sites were mostly located in gene bodies and promoter regions. Meanwhile, Functional distribution of 5,605 hypomethylated CpG sites suggested that 20.43% of these sites were located in promoter regions, 39.64% of these sites were located in gene bodies, 36.24% of these sites were located in intergenic regions and 3.69% of these sites were located in 3’UTR regions. Furthermore, sublocation analysis of 5,605 hypomethylated CpG sites in promoter regions indicated that 48.38% of these sites were located in TSS1500 regions, 15.46% of these sites were located in TSS200 regions, 11.35% of these sites were located in 1st Exon regions and 24.8% of these sites were located in 5’UTR regions. This seems to be consistent with previous findings that methylation of these regions inhibits transcription. For example, Irizarry et al. demonstrated that altered DNA methylation in cancer occurred in CGI shores rather than in the CGIs, and DNA methylation changes in CGI shores were strongly related to gene expression (23). In addition, we had noticed that numerous differential CpG sites were located in gene bodies. Recently, it became apparent that CGIs in gene bodies act as alternative promoters (24,25) and that tissue-specific or cell type-specific CGI methylation is prevalent in gene bodies (26). GO analysis of these significantly differentially methylated genes revealed that spindle checkpoint, mitotic spindle assembly checkpoint and negative regulation of mitotic sister chromatid segregation were the top three significantly enriched terms from perspective of biological processes. Meanwhile, from the cellular component perspective, there are 3 GO terms were assigned under this catalogues. Among these terms, A band was the top significantly enriched terms. In addition, from the molecular function perspective, there are 4 GO terms were assigned under this catalogues. Among these terms, G-protein beta/gamma-subunit complex binding was the top significantly over-represented terms. KEGG analysis showed that vibrio cholerae infection was the top enriched term. Moreover, pancreatic secretion, Type I diabetes mellitus, Insulin secretion and Adrenergic signaling in cardiomyocytes were also significant enriched in this study. Furthermore, GO analysis of differentially methylated sites located in promoter regions of genes showed that autophagosome assembly, autophagy and autophagosome organization were the top three significantly enriched terms from the perspective of biological processes. From the cellular component perspective, there are three GO terms were assigned under this catalogues. Among these terms, mitochondrial fatty acid beta-oxidation multienzyme complex, fatty acid beta-oxidation multienzyme complex and glycine cleavage complex were the top three significantly enriched terms. From the molecular function perspective, long-chain-3-hydroxyacyl-CoA dehydrogenase activity was the top significantly over-represented terms. Of the KEGG analysis, it clearly displays that Regulation of autophagy were the top enriched term. It is worth noting that Non-small cell lung cancer, Glioma, ErbB signaling pathway and Fc gamma R-mediated phagocytosis were also significant enriched in this study. Meanwhile, we have invested methylation status of 22 pancreatic cancer related key genes, and revealed the aberrant methylation status. For example, Cyclin D1 (CCND1) has been showed to be over-expressed in human pancreatic cancer (27). Here, CCND1 was identified as hypermethylated candidate gene that is inconsistent with a previous study (28), which suggested that over-expression of cyclin D1 in pancreatic cancer is associated with the loss of methylation.
There are several limitations to the present study. First, the sample size was not large. Further validation in studies encompassing more samples is warranted in the future. Second, the analyzed CpG sites were limited in number, although the 450 K microarray is one of the most powerful and cost-effective tools currently available for assessing methylation changes. Third, it is not possible to differentiate methylation from 5-hydroxymethylation of cytosine, which also plays a critical role in gene regulation (29). In summary, aberrant DNA methylation in pancreatic cancer tissues was identified at numerous CpG sites across the whole genome in using two independent sets of samples. Of the differently methylated CpG sites in the CGIs, most of them were located in the promoter regions. These findings support the hypothesis that altered DNA methylation could be involved in the pathophysiology of pancreatic cancer. Although the number of analyzed individuals was limited, the analysis was sufficient to provide DNA methylation distribution patterns across different genomic regions that were largely in agreement with patterns previously observed. The methylome data alone was sufficient for correctly distinguishing between all the ten tissues studied, collectively demonstrating that tissues are characterized by distinctive methylation patterns that reflect their tissue-specific functions. Our study provoked the question, of how differentially methylated CpG sites mechanistically contribute to the gene functions, especially for the numerous methylation regions that were found in gene body areas. In addition, it remains unclear, however, how the gene body differentially methylated CpG sites may function as regulators of gene expression, and this question should be addressed in the future epigenetic studies.
In conclusion, previous studies have demonstrated that DNA methylation play important roles in the regulation of developmental processes of several cancers. The identification of differentially methylated genes in this study provides information valuable to the in-depth study of pancreatic cancer. Moreover, the results of this study will not only improve our understanding of the differentially methylated genes but will also help to enhance methylome studies of pancreatic cancer.
Table S1
Patient No. | Age | Gender | Histology |
---|---|---|---|
1 | F | 74 | Highly differentiated ductal adenocarcinoma |
2 | M | 36 | Poorly differentiated adenocarcinoma |
3 | M | 66 | Moderately differentiated adenocarcinoma |
4 | F | 60 | Moderately differentiated ductal adenocarcinoma |
5 | F | 46 | Moderately differentiated ductal adenocarcinoma |
6 | F | 71 | Moderately-poorly differentiated adenocarcinoma |
F, female; M, male.
Table S2
GOID | Ontology | Term | Level | q | m | t | k | Gene IDs | Symbols | Log odds ratio | P |
---|---|---|---|---|---|---|---|---|---|---|---|
GO: 0048519 | Biological process | Negative regulation of biological process | 2 | 19 | 4,961 | 45,240 | 46 | Q92932, B3KR41, C9J9H5, C9JIR0, C9JJ38, C9JKI7, C9JP81, C9JPS1, C9JTA2, C9JX80, C9K086, Q9Y6D9, Q9HAZ2, P51787, C9J0X4, F5GX36, F5H0B1, P56524, Q9BTW9 | PTPRN2, MAD1L1, MAD1L1, MAD1L1, MAD1L1, MAD1L1, MAD1L1, MAD1L1, MAD1L1, MAD1L1, MAD1L1, MAD1L1, PRDM16, KCNQ1, HDAC4, HDAC4, HDAC4, HDAC4, TBCD | 1.913262 | 1.42711E−05 |
GO: 0000075 | Biological process | Cell cycle checkpoint | 3 | 11 | 264 | 45,240 | 46 | B3KR41, C9J9H5, C9JIR0, C9JJ38, C9JKI7, C9JP81, C9JPS1, C9JTA2, C9JX80, C9K086, Q9Y6D9 | MAD1L1, MAD1L1, MAD1L1, MAD1L1, MAD1L1, MAD1L1, MAD1L1, MAD1L1, MAD1L1, MAD1L1, MAD1L1 | 5.356787 | 5.86265E−13 |
GO: 0000278 | Biological process | Mitotic cell cycle | 2 | 11 | 810 | 45,240 | 46 | B3KR41, C9J9H5, C9JIR0, C9JJ38, C9JKI7, C9JP81, C9JPS1, C9JTA2, C9JX80, C9K086, Q9Y6D9 | MAD1L1, MAD1L1, MAD1L1, MAD1L1, MAD1L1, MAD1L1, MAD1L1, MAD1L1, MAD1L1, MAD1L1, MAD1L1 | 3.739403 | 6.84714E−08 |
GO: 0007049 | Biological process | Cell cycle | 2 | 11 | 1,636 | 45,240 | 46 | B3KR41, C9J9H5, C9JIR0, C9JJ38, C9JKI7, C9JP81, C9JPS1, C9JTA2, C9JX80, C9K086, Q9Y6D9 | MAD1L1, MAD1L1, MAD1L1, MAD1L1, MAD1L1, MAD1L1, MAD1L1, MAD1L1, MAD1L1, MAD1L1, MAD1L1 | 2.725224 | 5.43394E−05 |
GO: 0007088 | Biological process | Regulation of mitotic nuclear division | 4 | 11 | 154 | 45,240 | 46 | B3KR41, C9J9H5, C9JIR0, C9JJ38, C9JKI7, C9JP81, C9JPS1, C9JTA2, C9JX80, C9K086, Q9Y6D9 | MAD1L1, MAD1L1, MAD1L1, MAD1L1, MAD1L1, MAD1L1, MAD1L1, MAD1L1, MAD1L1, MAD1L1, MAD1L1 | 6.134394 | 2.24109E−15 |
GO: 0007093 | Biological process | Mitotic cell cycle checkpoint | 7 | 11 | 166 | 45,240 | 46 | B3KR41, C9J9H5, C9JIR0, C9JJ38, C9JKI7, C9JP81, C9JPS1, C9JTA2, C9JX80, C9K086, Q9Y6D9 | MAD1L1, MAD1L1, MAD1L1, MAD1L1, MAD1L1, MAD1L1, MAD1L1, MAD1L1, MAD1L1, MAD1L1, MAD1L1 | 6.026142 | 4.53154E−15 |
GO: 0007094 | Biological process | Mitotic spindle assembly checkpoint | 13 | 11 | 42 | 45,240 | 46 | B3KR41, C9J9H5, C9JIR0, C9JJ38, C9JKI7, C9JP81, C9JPS1, C9JTA2, C9JX80, C9K086, Q9Y6D9 | MAD1L1, MAD1L1, MAD1L1, MAD1L1, MAD1L1, MAD1L1, MAD1L1, MAD1L1, MAD1L1, MAD1L1, MAD1L1 | 8.008864 | 3.49706E−21 |
GO: 0007346 | Biological process | Regulation of mitotic cell cycle | 2 | 11 | 388 | 45,240 | 46 | B3KR41, C9J9H5, C9JIR0, C9JJ38, C9JKI7, C9JP81, C9JPS1, C9JTA2, C9JX80, C9K086, Q9Y6D9 | MAD1L1, MAD1L1, MAD1L1, MAD1L1, MAD1L1, MAD1L1, MAD1L1, MAD1L1, MAD1L1, MAD1L1, MAD1L1 | 4.801268 | 3.6196E−11 |
GO: 0010564 | Biological process | Regulation of cell cycle process | 2 | 11 | 462 | 45,240 | 46 | B3KR41, C9J9H5, C9JIR0, C9JJ38, C9JKI7, C9JP81, C9JPS1, C9JTA2, C9JX80, C9K086, Q9Y6D9 | MAD1L1, MAD1L1, MAD1L1, MAD1L1, MAD1L1, MAD1L1, MAD1L1, MAD1L1, MAD1L1, MAD1L1, MAD1L1 | 4.549432 | 2.32842E−10 |
GO: 0010639 | Biological process | Negative regulation of organelle organization | 5 | 12 | 264 | 45,240 | 46 | B3KR41, C9J9H5, C9JIR0, C9JJ38, C9JKI7, C9JP81, C9JPS1, C9JTA2, C9JX80, C9K086, Q9Y6D9, Q9BTW9 | MAD1L1, MAD1L1, MAD1L1, MAD1L1, MAD1L1, MAD1L1, MAD1L1, MAD1L1, MAD1L1, MAD1L1, MAD1L1, TBCD | 5.482318 | 1.24116E−14 |
GO: 0010948 | Biological process | Negative regulation of cell cycle process | 3 | 11 | 226 | 45,240 | 46 | B3KR41, C9J9H5, C9JIR0, C9JJ38, C9JKI7, C9JP81, C9JPS1, C9JTA2, C9JX80, C9K086, Q9Y6D9 | MAD1L1, MAD1L1, MAD1L1, MAD1L1, MAD1L1, MAD1L1, MAD1L1, MAD1L1, MAD1L1, MAD1L1, MAD1L1 | 5.581002 | 1.20706E−13 |
GO: 0010965 | Biological process | Regulation of mitotic sister chromatid separation | 5 | 11 | 80 | 45,240 | 46 | B3KR41, C9J9H5, C9JIR0, C9JJ38, C9JKI7, C9JP81, C9JPS1, C9JTA2, C9JX80, C9K086, Q9Y6D9 | MAD1L1, MAD1L1, MAD1L1, MAD1L1, MAD1L1, MAD1L1, MAD1L1, MAD1L1, MAD1L1, MAD1L1, MAD1L1 | 7.079253 | 1.5405E−18 |
GO: 0022402 | Biological process | Cell cycle process | 3 | 11 | 1,278 | 45,240 | 46 | B3KR41, C9J9H5, C9JIR0, C9JJ38, C9JKI7, C9JP81, C9JPS1, C9JTA2, C9JX80, C9K086, Q9Y6D9 | MAD1L1, MAD1L1, MAD1L1, MAD1L1, MAD1L1, MAD1L1, MAD1L1, MAD1L1, MAD1L1, MAD1L1, MAD1L1 | 3.081509 | 6.23166E−06 |
GO: 0030071 | Biological process | Regulation of mitotic metaphase/anaphase transition | 7 | 11 | 76 | 45,240 | 46 | B3KR41, C9J9H5, C9JIR0, C9JJ38, C9JKI7, C9JP81, C9JPS1, C9JTA2, C9JX80, C9K086, Q9Y6D9 | MAD1L1, MAD1L1, MAD1L1, MAD1L1, MAD1L1, MAD1L1, MAD1L1, MAD1L1, MAD1L1, MAD1L1, MAD1L1 | 7.153253 | 9.65074E−19 |
GO: 0031577 | Biological process | Spindle checkpoint | 3 | 11 | 50 | 45,240 | 46 | B3KR41, C9J9H5, C9JIR0, C9JJ38, C9JKI7, C9JP81, C9JPS1, C9JTA2, C9JX80, C9K086, Q9Y6D9 | MAD1L1, MAD1L1, MAD1L1, MAD1L1, MAD1L1, MAD1L1, MAD1L1, MAD1L1, MAD1L1, MAD1L1, MAD1L1 | 7.757325 | 8.97641E−21 |
GO: 0033043 | Biological process | Regulation of organelle organization | 2 | 12 | 854 | 45,240 | 46 | B3KR41, C9J9H5, C9JIR0, C9JJ38, C9JKI7, C9JP81, C9JPS1, C9JTA2, C9JX80, C9K086, Q9Y6D9, Q9BTW9 | MAD1L1, MAD1L1, MAD1L1, MAD1L1, MAD1L1, MAD1L1, MAD1L1, MAD1L1, MAD1L1, MAD1L1, MAD1L1, TBCD | 3.78862 | 7.71321E−09 |
GO: 0033044 | Biological process | Regulation of chromosome organization | 2 | 11 | 239 | 45,240 | 46 | B3KR41, C9J9H5, C9JIR0, C9JJ38, C9JKI7, C9JP81, C9JPS1, C9JTA2, C9JX80, C9K086, Q9Y6D9 | MAD1L1, MAD1L1, MAD1L1, MAD1L1, MAD1L1, MAD1L1, MAD1L1, MAD1L1, MAD1L1, MAD1L1, MAD1L1 | 5.500314 | 2.01867E−13 |
GO: 0033045 | Biological process | Regulation of sister chromatid segregation | 4 | 11 | 91 | 45,240 | 46 | B3KR41, C9J9H5, C9JIR0, C9JJ38, C9JKI7, C9JP81, C9JPS1, C9JTA2, C9JX80, C9K086, Q9Y6D9 | MAD1L1, MAD1L1, MAD1L1, MAD1L1, MAD1L1, MAD1L1, MAD1L1, MAD1L1, MAD1L1, MAD1L1, MAD1L1 | 6.893386 | 6.4978E−18 |
GO: 0033046 | Biological process | Negative regulation of sister chromatid segregation | 7 | 11 | 47 | 45,240 | 46 | B3KR41, C9J9H5, C9JIR0, C9JJ38, C9JKI7, C9JP81, C9JPS1, C9JTA2, C9JX80, C9K086, Q9Y6D9 | MAD1L1, MAD1L1, MAD1L1, MAD1L1, MAD1L1, MAD1L1, MAD1L1, MAD1L1, MAD1L1, MAD1L1, MAD1L1 | 7.846592 | 4.66045E−21 |
GO: 0033047 | Biological process | Regulation of mitotic sister chromatid segregation | 5 | 11 | 80 | 45,240 | 46 | B3KR41, C9J9H5, C9JIR0, C9JJ38, C9JKI7, C9JP81, C9JPS1, C9JTA2, C9JX80, C9K086, Q9Y6D9 | MAD1L1, MAD1L1, MAD1L1, MAD1L1, MAD1L1, MAD1L1, MAD1L1, MAD1L1, MAD1L1, MAD1L1, MAD1L1 | 7.079253 | 1.5405E−18 |
GO: 0033048 | Biological process | Negative regulation of mitotic sister chromatid segregation | 8 | 11 | 45 | 45,240 | 46 | B3KR41, C9J9H5, C9JIR0, C9JJ38, C9JKI7, C9JP81, C9JPS1, C9JTA2, C9JX80, C9K086, Q9Y6D9 | MAD1L1, MAD1L1, MAD1L1, MAD1L1, MAD1L1, MAD1L1, MAD1L1, MAD1L1, MAD1L1, MAD1L1, MAD1L1 | 7.909328 | 3.49706E−21 |
GO: 0045786 | Biological process | Negative regulation of cell cycle | 3 | 11 | 481 | 45,240 | 46 | B3KR41, C9J9H5, C9JIR0, C9JJ38, C9JKI7, C9JP81, C9JPS1, C9JTA2, C9JX80, C9K086, Q9Y6D9 | MAD1L1, MAD1L1, MAD1L1, MAD1L1, MAD1L1, MAD1L1, MAD1L1, MAD1L1, MAD1L1, MAD1L1, MAD1L1 | 4.491288 | 3.49572E−10 |
GO: 0045839 | Biological process | Negative regulation of mitotic nuclear division | 7 | 11 | 53 | 45,240 | 46 | B3KR41, C9J9H5, C9JIR0, C9JJ38, C9JKI7, C9JP81, C9JPS1, C9JTA2, C9JX80, C9K086, Q9Y6D9 | MAD1L1, MAD1L1, MAD1L1, MAD1L1, MAD1L1, MAD1L1, MAD1L1, MAD1L1, MAD1L1, MAD1L1, MAD1L1 | 7.673261 | 1.66166E−20 |
GO: 0045841 | Biological process | Negative regulation of mitotic metaphase/anaphase transition | 9 | 11 | 45 | 45,240 | 46 | B3KR41, C9J9H5, C9JIR0, C9JJ38, C9JKI7, C9JP81, C9JPS1, C9JTA2, C9JX80, C9K086, Q9Y6D9 | MAD1L1, MAD1L1, MAD1L1, MAD1L1, MAD1L1, MAD1L1, MAD1L1, MAD1L1, MAD1L1, MAD1L1, MAD1L1 | 7.909328 | 3.49706E−21 |
GO: 0045930 | Biological process | Negative regulation of mitotic cell cycle | 3 | 11 | 204 | 45,240 | 46 | B3KR41, C9J9H5, C9JIR0, C9JJ38, C9JKI7, C9JP81, C9JPS1, C9JTA2, C9JX80, C9K086, Q9Y6D9 | MAD1L1, MAD1L1, MAD1L1, MAD1L1, MAD1L1, MAD1L1, MAD1L1, MAD1L1, MAD1L1, MAD1L1, MAD1L1 | 5.728756 | 4.01676E−14 |
GO: 0048523 | Biological process | Negative regulation of cellular process | 3 | 18 | 4,555 | 45,240 | 46 | B3KR41, C9J9H5, C9JIR0, C9JJ38, C9JKI7, C9JP81, C9JPS1, C9JTA2, C9JX80, C9K086, Q9Y6D9, Q9HAZ2, P51787, C9J0X4, F5GX36, F5H0B1, P56524, Q9BTW9 | MAD1L1, MAD1L1, MAD1L1, MAD1L1, MAD1L1, MAD1L1, MAD1L1, MAD1L1, MAD1L1, MAD1L1, MAD1L1, PRDM16, KCNQ1, HDAC4, HDAC4, HDAC4, HDAC4, TBCD | 1.958439 | 2.17828E−05 |
GO: 0051128 | Biological process | Regulation of cellular component organization | 2 | 12 | 2,006 | 45,240 | 46 | B3KR41, C9J9H5, C9JIR0, C9JJ38, C9JKI7, C9JP81, C9JPS1, C9JTA2, C9JX80, C9K086, Q9Y6D9, Q9BTW9 | MAD1L1, MAD1L1, MAD1L1, MAD1L1, MAD1L1, MAD1L1, MAD1L1, MAD1L1, MAD1L1, MAD1L1, MAD1L1, TBCD | 2.556606 | 5.28453E−05 |
GO: 0051129 | Biological process | Negative regulation of cellular component organization | 4 | 12 | 524 | 45,240 | 46 | B3KR41, C9J9H5, C9JIR0, C9JJ38, C9JKI7, C9JP81, C9JPS1, C9JTA2, C9JX80, C9K086, Q9Y6D9, Q9BTW9 | MAD1L1, MAD1L1, MAD1L1, MAD1L1, MAD1L1, MAD1L1, MAD1L1, MAD1L1, MAD1L1, MAD1L1, MAD1L1, TBCD | 4.493289 | 3.19493E−11 |
GO: 0051726 | Biological process | Regulation of cell cycle | 2 | 11 | 966 | 45,240 | 46 | B3KR41, C9J9H5, C9JIR0, C9JJ38, C9JKI7, C9JP81, C9JPS1, C9JTA2, C9JX80, C9K086, Q9Y6D9 | MAD1L1, MAD1L1, MAD1L1, MAD1L1, MAD1L1, MAD1L1, MAD1L1, MAD1L1, MAD1L1, MAD1L1, MAD1L1 | 3.485302 | 4.03765E−07 |
GO: 0051783 | Biological process | Regulation of nuclear division | 2 | 11 | 186 | 45,240 | 46 | B3KR41, C9J9H5, C9JIR0, C9JJ38, C9JKI7, C9JP81, C9JPS1, C9JTA2, C9JX80, C9K086, Q9Y6D9 | MAD1L1, MAD1L1, MAD1L1, MAD1L1, MAD1L1, MAD1L1, MAD1L1, MAD1L1, MAD1L1, MAD1L1, MAD1L1 | 5.862022 | 1.49082E−14 |
GO: 0051784 | Biological process | Negative regulation of nuclear division | 5 | 11 | 71 | 45,240 | 46 | B3KR41, C9J9H5, C9JIR0, C9JJ38, C9JKI7, C9JP81, C9JPS1, C9JTA2, C9JX80, C9K086, Q9Y6D9 | MAD1L1, MAD1L1, MAD1L1, MAD1L1, MAD1L1, MAD1L1, MAD1L1, MAD1L1, MAD1L1, MAD1L1, MAD1L1 | 7.251434 | 5.05177E−19 |
GO: 0051983 | Biological process | Regulation of chromosome segregation | 2 | 11 | 103 | 45,240 | 46 | B3KR41, C9J9H5, C9JIR0, C9JJ38, C9JKI7, C9JP81, C9JPS1, C9JTA2, C9JX80, C9K086, Q9Y6D9 | MAD1L1, MAD1L1, MAD1L1, MAD1L1, MAD1L1, MAD1L1, MAD1L1, MAD1L1, MAD1L1, MAD1L1, MAD1L1 | 6.71468 | 2.42944E−17 |
GO: 0051985 | Biological process | Negative regulation of chromosome segregation | 4 | 11 | 47 | 45,240 | 46 | B3KR41, C9J9H5, C9JIR0, C9JJ38, C9JKI7, C9JP81, C9JPS1, C9JTA2, C9JX80, C9K086, Q9Y6D9 | MAD1L1, MAD1L1, MAD1L1, MAD1L1, MAD1L1, MAD1L1, MAD1L1, MAD1L1, MAD1L1, MAD1L1, MAD1L1 | 7.846592 | 4.66045E−21 |
GO: 0071173 | Biological process | Spindle assembly checkpoint | 3 | 11 | 45 | 45,240 | 46 | B3KR41, C9J9H5, C9JIR0, C9JJ38, C9JKI7, C9JP81, C9JPS1, C9JTA2, C9JX80, C9K086, Q9Y6D9 | MAD1L1, MAD1L1, MAD1L1, MAD1L1, MAD1L1, MAD1L1, MAD1L1, MAD1L1, MAD1L1, MAD1L1, MAD1L1 | 7.909328 | 3.49706E−21 |
GO: 0071174 | Biological process | Mitotic spindle checkpoint | 11 | 11 | 45 | 45,240 | 46 | B3KR41, C9J9H5, C9JIR0, C9JJ38, C9JKI7, C9JP81, C9JPS1, C9JTA2, C9JX80, C9K086, Q9Y6D9 | MAD1L1, MAD1L1, MAD1L1, MAD1L1, MAD1L1, MAD1L1, MAD1L1, MAD1L1, MAD1L1, MAD1L1, MAD1L1 | 7.909328 | 3.49706E−21 |
GO:1901987 | Biological process | Regulation of cell cycle phase transition | 2 | 11 | 237 | 45,240 | 46 | B3KR41, C9J9H5, C9JIR0, C9JJ38, C9JKI7, C9JP81, C9JPS1, C9JTA2, C9JX80, C9K086, Q9Y6D9 | MAD1L1, MAD1L1, MAD1L1, MAD1L1, MAD1L1, MAD1L1, MAD1L1, MAD1L1, MAD1L1, MAD1L1, MAD1L1 | 5.512438 | 1.9028E−13 |
GO:1901988 | Biological process | Negative regulation of cell cycle phase transition | 3 | 11 | 160 | 45,240 | 46 | B3KR41, C9J9H5, C9JIR0, C9JJ38, C9JKI7, C9JP81, C9JPS1, C9JTA2, C9JX80, C9K086, Q9Y6D9 | MAD1L1, MAD1L1, MAD1L1, MAD1L1, MAD1L1, MAD1L1, MAD1L1, MAD1L1, MAD1L1, MAD1L1, MAD1L1 | 6.079253 | 3.13253E−15 |
GO:1901990 | Biological process | Regulation of mitotic cell cycle phase transition | 3 | 11 | 234 | 45,240 | 46 | B3KR41, C9J9H5, C9JIR0, C9JJ38, C9JKI7, C9JP81, C9JPS1, C9JTA2, C9JX80, C9K086, Q9Y6D9 | MAD1L1, MAD1L1, MAD1L1, MAD1L1, MAD1L1, MAD1L1, MAD1L1, MAD1L1, MAD1L1, MAD1L1, MAD1L1 | 5.530816 | 1.71147E−13 |
GO:1901991 | Biological process | Negative regulation of mitotic cell cycle phase transition | 5 | 11 | 157 | 45,240 | 46 | B3KR41, C9J9H5, C9JIR0, C9JJ38, C9JKI7, C9JP81, C9JPS1, C9JTA2, C9JX80, C9K086, Q9Y6D9 | MAD1L1, MAD1L1, MAD1L1, MAD1L1, MAD1L1, MAD1L1, MAD1L1, MAD1L1, MAD1L1, MAD1L1, MAD1L1 | 6.10656 | 2.65226E−15 |
GO:1902099 | Biological process | Regulation of metaphase/anaphase transition of cell cycle | 4 | 11 | 76 | 45,240 | 46 | B3KR41, C9J9H5, C9JIR0, C9JJ38, C9JKI7, C9JP81, C9JPS1, C9JTA2, C9JX80, C9K086, Q9Y6D9 | MAD1L1, MAD1L1, MAD1L1, MAD1L1, MAD1L1, MAD1L1, MAD1L1, MAD1L1, MAD1L1, MAD1L1, MAD1L1 | 7.153253 | 9.65074E−19 |
GO:1902100 | Biological process | Negative regulation of metaphase/anaphase transition of cell cycle | 4 | 11 | 45 | 45,240 | 46 | B3KR41, C9J9H5, C9JIR0, C9JJ38, C9JKI7, C9JP81, C9JPS1, C9JTA2, C9JX80, C9K086, Q9Y6D9 | MAD1L1, MAD1L1, MAD1L1, MAD1L1, MAD1L1, MAD1L1, MAD1L1, MAD1L1, MAD1L1, MAD1L1, MAD1L1 | 7.909328 | 3.49706E−21 |
GO:1903047 | Biological process | Mitotic cell cycle process | 4 | 11 | 726 | 45,240 | 46 | B3KR41, C9J9H5, C9JIR0, C9JJ38, C9JKI7, C9JP81, C9JPS1, C9JTA2, C9JX80, C9K086, Q9Y6D9 | MAD1L1, MAD1L1, MAD1L1, MAD1L1, MAD1L1, MAD1L1, MAD1L1, MAD1L1, MAD1L1, MAD1L1, MAD1L1 | 3.897355 | 2.31135E−08 |
GO:2000816 | Biological process | Negative regulation of mitotic sister chromatid separation | 8 | 11 | 45 | 45,240 | 46 | B3KR41, C9J9H5, C9JIR0, C9JJ38, C9JKI7, C9JP81, C9JPS1, C9JTA2, C9JX80, C9K086, Q9Y6D9 | MAD1L1, MAD1L1, MAD1L1, MAD1L1, MAD1L1, MAD1L1, MAD1L1, MAD1L1, MAD1L1, MAD1L1, MAD1L1 | 7.909328 | 3.49706E−21 |
GO:2001251 | Biological process | Negative regulation of chromosome organization | 5 | 11 | 99 | 45,240 | 46 | B3KR41, C9J9H5, C9JIR0, C9JJ38, C9JKI7, C9JP81, C9JPS1, C9JTA2, C9JX80, C9K086, Q9Y6D9 | MAD1L1, MAD1L1, MAD1L1, MAD1L1, MAD1L1, MAD1L1, MAD1L1, MAD1L1, MAD1L1, MAD1L1, MAD1L1 | 6.771824 | 1.62527E−17 |
GO: 0015629 | Cellular component | Actin cytoskeleton | 4 | 8 | 741 | 45,240 | 46 | B3KR41, C9JKI7, C9JP81, Q9Y6D9, C9J0X4, F5GX36, F5H0B1, P56524 | MAD1L1, MAD1L1, MAD1L1, MAD1L1, HDAC4, HDAC4, HDAC4, HDAC4 | 3.40842 | 7.07259E−05 |
GO: 0043467 | Biological process | Regulation of generation of precursor metabolites and energy | 3 | 5 | 71 | 45,240 | 46 | Q9HAZ2, C9J0X4, F5GX36, F5H0B1, P56524 | PRDM16, HDAC4, HDAC4, HDAC4, HDAC4 | 6.11393 | 1.58738E−06 |
GO: 0003924 | Molecular function | Gtpase activity | 1 | 7 | 456 | 45,240 | 46 | A2A2R6, H0Y7E8, H0Y7F4, P63092, Q5JWD1, Q5JWE9, Q5JWF2 | GNAS, GNAS, GNAS, GNAS, GNAS, GNAS, GNAS | 3.916214 | 4.02427E−05 |
GO: 0031683 | Molecular function | G-protein beta/gamma-subunit complex binding | 2 | 7 | 53 | 45,240 | 46 | A2A2R6, H0Y7E8, H0Y7F4, P63092, Q5JWD1, Q5JWE9, Q5JWF2 | GNAS, GNAS, GNAS, GNAS, GNAS, GNAS, GNAS | 7.021184 | 2.43723E−11 |
GO: 0045667 | Biological process | Regulation of osteoblast differentiation | 3 | 5 | 157 | 45,240 | 46 | Q5JWF2, C9J0X4, F5GX36, F5H0B1, P56524 | GNAS, HDAC4, HDAC4, HDAC4, HDAC4 | 4.969057 | 5.56829E−05 |
GO: 0008016 | Biological process | Regulation of heart contraction | 2 | 5 | 178 | 45,240 | 46 | P51787, C9J0X4, F5GX36, F5H0B1, P56524 | KCNQ1, HDAC4, HDAC4, HDAC4, HDAC4 | 4.787944 | 9.84102E−05 |
GO: 0002076 | Biological process | Osteoblast development | 6 | 4 | 33 | 45,240 | 46 | C9J0X4, F5GX36, F5H0B1, P56524 | HDAC4, HDAC4, HDAC4, HDAC4 | 6.897355 | 5.03412E−06 |
GO: 0006942 | Biological process | Regulation of striated muscle contraction | 2 | 4 | 63 | 45,240 | 46 | C9J0X4, F5GX36, F5H0B1, P56524 | HDAC4, HDAC4, HDAC4, HDAC4 | 5.964469 | 5.28453E−05 |
GO: 0010882 | Biological process | Regulation of cardiac muscle contraction by calcium ion signaling | 5 | 4 | 25 | 45,240 | 46 | C9J0X4, F5GX36, F5H0B1, P56524 | HDAC4, HDAC4, HDAC4, HDAC4 | 7.297893 | 1.65414E−06 |
GO: 0055117 | Biological process | Regulation of cardiac muscle contraction | 3 | 4 | 52 | 45,240 | 46 | C9J0X4, F5GX36, F5H0B1, P56524 | HDAC4, HDAC4, HDAC4, HDAC4 | 6.24131 | 2.70438E−05 |
GO: 0014854 | Biological process | Response to inactivity | 1 | 4 | 10 | 45,240 | 46 | C9J0X4, F5GX36, F5H0B1, P56524 | HDAC4, HDAC4, HDAC4, HDAC4 | 8.619821 | 3.27042E−08 |
GO: 0014870 | Biological process | Response to muscle inactivity | 1 | 4 | 7 | 45,240 | 46 | C9J0X4, F5GX36, F5H0B1, P56524 | HDAC4, HDAC4, HDAC4, HDAC4 | 9.134394 | 6.30331E−09 |
GO: 0014874 | Biological process | Response to stimulus involved in regulation of muscle adaptation | 3 | 4 | 11 | 45,240 | 46 | C9J0X4, F5GX36, F5H0B1, P56524 | HDAC4, HDAC4, HDAC4, HDAC4 | 8.482318 | 5.02378E−08 |
GO: 0014877 | Biological process | Response to muscle inactivity involved in regulation of muscle adaptation | 5 | 4 | 7 | 45,240 | 46 | C9J0X4, F5GX36, F5H0B1, P56524 | HDAC4, HDAC4, HDAC4, HDAC4 | 9.134394 | 6.30331E−09 |
GO: 0014894 | Biological process | Response to denervation involved in regulation of muscle adaptation | 5 | 4 | 7 | 45,240 | 46 | C9J0X4, F5GX36, F5H0B1, P56524 | HDAC4, HDAC4, HDAC4, HDAC4 | 9.134394 | 6.30331E−09 |
GO: 0043502 | Biological process | Regulation of muscle adaptation | 3 | 4 | 44 | 45,240 | 46 | C9J0X4, F5GX36, F5H0B1, P56524 | HDAC4, HDAC4, HDAC4, HDAC4 | 6.482318 | 1.42711E−05 |
GO: 0031672 | Cellular component | A band | 7 | 4 | 41 | 45,240 | 46 | C9J0X4, F5GX36, F5H0B1, P56524 | HDAC4, HDAC4, HDAC4, HDAC4 | 6.584197 | 1.10087E−05 |
GO: 0019213 | Molecular function | Deacetylase activity | 1 | 4 | 73 | 45,240 | 46 | C9J0X4, F5GX36, F5H0B1, P56524 | HDAC4, HDAC4, HDAC4, HDAC4 | 5.751925 | 8.98652E−05 |
GO: 0033558 | Molecular function | Protein deacetylase activity | 1 | 4 | 56 | 45,240 | 46 | C9J0X4, F5GX36, F5H0B1, P56524 | HDAC4, HDAC4, HDAC4, HDAC4 | 6.134394 | 3.5536E−05 |
GO: 0042641 | Cellular component | Actomyosin | 6 | 4 | 67 | 45,240 | 46 | C9J0X4, F5GX36, F5H0B1, P56524 | HDAC4, HDAC4, HDAC4, HDAC4 | 5.87566 | 6.51755E−05 |
GO: 0045668 | Biological process | Negative regulation of osteoblast differentiation | 5 | 4 | 57 | 45,240 | 46 | C9J0X4, F5GX36, F5H0B1, P56524 | HDAC4, HDAC4, HDAC4, HDAC4 | 6.108859 | 3.76518E−05 |
GO: 0006110 | Biological process | Regulation of glycolytic process | 7 | 4 | 25 | 45,240 | 46 | C9J0X4, F5GX36, F5H0B1, P56524 | HDAC4, HDAC4, HDAC4, HDAC4 | 7.297893 | 1.65414E−06 |
GO: 0009118 | Biological process | Regulation of nucleoside metabolic process | 4 | 4 | 30 | 45,240 | 46 | C9J0X4, F5GX36, F5H0B1, P56524 | HDAC4, HDAC4, HDAC4, HDAC4 | 7.034859 | 3.44042E−06 |
GO: 0010677 | Biological process | Negative regulation of cellular carbohydrate metabolic process | 6 | 4 | 36 | 45,240 | 46 | C9J0X4, F5GX36, F5H0B1, P56524 | HDAC4, HDAC4, HDAC4, HDAC4 | 6.771824 | 6.98124E−06 |
GO: 0043470 | Biological process | Regulation of carbohydrate catabolic process | 3 | 4 | 37 | 45,240 | 46 | C9J0X4, F5GX36, F5H0B1, P56524 | HDAC4, HDAC4, HDAC4, HDAC4 | 6.732296 | 7.68907E−06 |
GO: 0045820 | Biological process | Negative regulation of glycolytic process | 12 | 4 | 8 | 45,240 | 46 | C9J0X4, F5GX36, F5H0B1, P56524 | HDAC4, HDAC4, HDAC4, HDAC4 | 8.941749 | 1.14254E−08 |
GO: 0045912 | Biological process | Negative regulation of carbohydrate metabolic process | 4 | 4 | 40 | 45,240 | 46 | C9J0X4, F5GX36, F5H0B1, P56524 | HDAC4, HDAC4, HDAC4, HDAC4 | 6.619821 | 1.01024E−05 |
GO: 0045978 | Biological process | Negative regulation of nucleoside metabolic process | 7 | 4 | 12 | 45,240 | 46 | C9J0X4, F5GX36, F5H0B1, P56524 | HDAC4, HDAC4, HDAC4, HDAC4 | 8.356787 | 7.06904E−08 |
GO: 0045980 | Biological process | Negative regulation of nucleotide metabolic process | 8 | 4 | 62 | 45,240 | 46 | C9J0X4, F5GX36, F5H0B1, P56524 | HDAC4, HDAC4, HDAC4, HDAC4 | 5.987553 | 5.08282E−05 |
GO: 0051193 | Biological process | Regulation of cofactor metabolic process | 3 | 4 | 39 | 45,240 | 46 | C9J0X4, F5GX36, F5H0B1, P56524 | HDAC4, HDAC4, HDAC4, HDAC4 | 6.656347 | 9.24809E−06 |
GO: 0051195 | Biological process | Negative regulation of cofactor metabolic process | 6 | 4 | 8 | 45,240 | 46 | C9J0X4, F5GX36, F5H0B1, P56524 | HDAC4, HDAC4, HDAC4, HDAC4 | 8.941749 | 1.14254E−08 |
GO: 0051196 | Biological process | Regulation of coenzyme metabolic process | 3 | 4 | 39 | 45,240 | 46 | C9J0X4, F5GX36, F5H0B1, P56524 | HDAC4, HDAC4, HDAC4, HDAC4 | 6.656347 | 9.24809E−06 |
GO: 0051198 | Biological process | Negative regulation of coenzyme metabolic process | 7 | 4 | 8 | 45,240 | 46 | C9J0X4, F5GX36, F5H0B1, P56524 | HDAC4, HDAC4, HDAC4, HDAC4 | 8.941749 | 1.14254E−08 |
GO:1900543 | Biological process | Negative regulation of purine nucleotide metabolic process | 9 | 4 | 60 | 45,240 | 46 | C9J0X4, F5GX36, F5H0B1, P56524 | HDAC4, HDAC4, HDAC4, HDAC4 | 6.034859 | 4.51066E−05 |
GO:1903578 | Biological process | Regulation of ATP metabolic process | 5 | 4 | 30 | 45,240 | 46 | C9J0X4, F5GX36, F5H0B1, P56524 | HDAC4, HDAC4, HDAC4, HDAC4 | 7.034859 | 3.44042E−06 |
GO:1903579 | Biological process | Negative regulation of ATP metabolic process | 11 | 4 | 12 | 45,240 | 46 | C9J0X4, F5GX36, F5H0B1, P56524 | HDAC4, HDAC4, HDAC4, HDAC4 | 8.356787 | 7.06904E−08 |
GO: 0010830 | Biological process | Regulation of myotube differentiation | 3 | 4 | 50 | 45,240 | 46 | C9J0X4, F5GX36, F5H0B1, P56524 | HDAC4, HDAC4, HDAC4, HDAC4 | 6.297893 | 2.33836E−05 |
GO: 0048742 | Biological process | Regulation of skeletal muscle fiber development | 5 | 3 | 13 | 45,240 | 46 | C9J0X4, F5GX36, F5H0B1 | HDAC4, HDAC4, HDAC4 | 7.826272 | 3.04774E−05 |
Statistical test method: hypergeometric test/Fisher’s exact test. FDR correction method: Benjamini and Hochberg.
Table S3
#Term | Database | ID | Input number | Background number | P value | Corrected P value | Input | Hyperlink | |
---|---|---|---|---|---|---|---|---|---|
Vibrio cholerae infection | KEGG PATHWAY | hsa05110 | 2 | 50 | 0.006229 | 0.308577 | 0.510637 | ENSG00000087460, ENSG00000053918 | http://www.genome.jp/kegg-bin/show_pathway?hsa05110/hsa:2778%09red/hsa:3784%09red |
Gastric acid secretion | KEGG PATHWAY | hsa04971 | 2 | 74 | 0.012932 | 0.308577 | 0.510637 | ENSG00000087460, ENSG00000053918 | http://www.genome.jp/kegg-bin/show_pathway?hsa04971/hsa:2778%09red/hsa:3784%09red |
Pancreatic secretion | KEGG PATHWAY | hsa04972 | 2 | 96 | 0.020893 | 0.308577 | 0.510637 | ENSG00000087460, ENSG00000053918 | http://www.genome.jp/kegg-bin/show_pathway?hsa04972/hsa:2778%09red/hsa:3784%09red |
Adrenergic signaling in cardiomyocytes | KEGG PATHWAY | hsa04261 | 2 | 151 | 0.047242 | 0.308577 | 0.510637 | ENSG00000087460, ENSG00000053918 | http://www.genome.jp/kegg-bin/show_pathway?hsa04261/hsa:2778%09red/hsa:3784%09red |
Alcoholism | KEGG PATHWAY | hsa05034 | 2 | 180 | 0.064213 | 0.308577 | 0.510637 | ENSG00000087460, ENSG00000068024 | http://www.genome.jp/kegg-bin/show_pathway?hsa05034/hsa:9759%09red/hsa:2778%09red |
Viral carcinogenesis | KEGG PATHWAY | hsa05203 | 2 | 207 | 0.08156 | 0.308577 | 0.510637 | ENSG00000002822, ENSG00000068024 | http://www.genome.jp/kegg-bin/show_pathway?hsa05203/hsa:9759%09red/hsa:8379%09red |
Type I diabetes mellitus | KEGG PATHWAY | hsa04940 | 1 | 42 | 0.093054 | 0.308577 | 0.510637 | ENSG00000155093 | http://www.genome.jp/kegg-bin/show_pathway?hsa04940/hsa:5799%09red |
Vasopressin-regulated water reabsorption | KEGG PATHWAY | hsa04962 | 1 | 45 | 0.099219 | 0.308577 | 0.510637 | ENSG00000087460 | http://www.genome.jp/kegg-bin/show_pathway?hsa04962/hsa:2778%09red |
Endocrine and other factor-regulated calcium reabsorption | KEGG PATHWAY | hsa04961 | 1 | 47 | 0.103306 | 0.308577 | 0.510637 | ENSG00000087460 | http://www.genome.jp/kegg-bin/show_pathway?hsa04961/hsa:2778%09red |
Cocaine addiction | KEGG PATHWAY | hsa05030 | 1 | 49 | 0.107375 | 0.308577 | 0.510637 | ENSG00000087460 | http://www.genome.jp/kegg-bin/show_pathway?hsa05030/hsa:2778%09red |
Ovarian steroidogenesis | KEGG PATHWAY | hsa04913 | 1 | 52 | 0.113444 | 0.308577 | 0.510637 | ENSG00000087460 | http://www.genome.jp/kegg-bin/show_pathway?hsa04913/hsa:2778%09red |
Regulation of lipolysis in adipocytes | KEGG PATHWAY | hsa04923 | 1 | 58 | 0.12546 | 0.308577 | 0.510637 | ENSG00000087460 | http://www.genome.jp/kegg-bin/show_pathway?hsa04923/hsa:2778%09red |
MicroRNAs in cancer | KEGG PATHWAY | hsa05206 | 2 | 273 | 0.128856 | 0.308577 | 0.510637 | ENSG00000068024, ENSG00000168477 | http://www.genome.jp/kegg-bin/show_pathway?hsa05206/hsa:9759%09red/hsa:7148%09red |
Long-term depression | KEGG PATHWAY | hsa04730 | 1 | 61 | 0.131408 | 0.308577 | 0.510637 | ENSG00000087460 | http://www.genome.jp/kegg-bin/show_pathway?hsa04730/hsa:2778%09red |
Renin secretion | KEGG PATHWAY | hsa04924 | 1 | 64 | 0.137317 | 0.308577 | 0.510637 | ENSG00000087460 | http://www.genome.jp/kegg-bin/show_pathway?hsa04924/hsa:2778%09red |
Amphetamine addiction | KEGG PATHWAY | hsa05031 | 1 | 67 | 0.143185 | 0.308577 | 0.510637 | ENSG00000087460 | http://www.genome.jp/kegg-bin/show_pathway?hsa05031/hsa:2778%09red |
Bile secretion | KEGG PATHWAY | hsa04976 | 1 | 71 | 0.150949 | 0.308577 | 0.510637 | ENSG00000087460 | http://www.genome.jp/kegg-bin/show_pathway?hsa04976/hsa:2778%09red |
Thyroid hormone synthesis | KEGG PATHWAY | hsa04918 | 1 | 71 | 0.150949 | 0.308577 | 0.510637 | ENSG00000087460 | http://www.genome.jp/kegg-bin/show_pathway?hsa04918/hsa:2778%09red |
Aldosterone synthesis and secretion | KEGG PATHWAY | hsa04925 | 1 | 80 | 0.168167 | 0.308577 | 0.510637 | ENSG00000087460 | http://www.genome.jp/kegg-bin/show_pathway?hsa04925/hsa:2778%09red |
ECM-receptor interaction | KEGG PATHWAY | hsa04512 | 1 | 83 | 0.173829 | 0.308577 | 0.510637 | ENSG00000168477 | http://www.genome.jp/kegg-bin/show_pathway?hsa04512/hsa:7148%09red |
Insulin secretion | KEGG PATHWAY | hsa04911 | 1 | 87 | 0.18132 | 0.308577 | 0.510637 | ENSG00000087460 | http://www.genome.jp/kegg-bin/show_pathway?hsa04911/hsa:2778%09red |
Gap junction | KEGG PATHWAY | hsa04540 | 1 | 88 | 0.183182 | 0.308577 | 0.510637 | ENSG00000087460 | http://www.genome.jp/kegg-bin/show_pathway?hsa04540/hsa:2778%09red |
Salivary secretion | KEGG PATHWAY | hsa04970 | 1 | 90 | 0.186894 | 0.308577 | 0.510637 | ENSG00000087460 | http://www.genome.jp/kegg-bin/show_pathway?hsa04970/hsa:2778%09red |
Dilated cardiomyopathy | KEGG PATHWAY | hsa05414 | 1 | 90 | 0.186894 | 0.308577 | 0.510637 | ENSG00000087460 | http://www.genome.jp/kegg-bin/show_pathway?hsa05414/hsa:2778%09red |
Protein digestion and absorption | KEGG PATHWAY | hsa04974 | 1 | 90 | 0.186894 | 0.308577 | 0.510637 | ENSG00000053918 | http://www.genome.jp/kegg-bin/show_pathway?hsa04974/hsa:3784%09red |
Morphine addiction | KEGG PATHWAY | hsa05032 | 1 | 91 | 0.188744 | 0.308577 | 0.510637 | ENSG00000087460 | http://www.genome.jp/kegg-bin/show_pathway?hsa05032/hsa:2778%09red |
GnRH signaling pathway | KEGG PATHWAY | hsa04912 | 1 | 92 | 0.19059 | 0.308577 | 0.510637 | ENSG00000087460 | http://www.genome.jp/kegg-bin/show_pathway?hsa04912/hsa:2778%09red |
Circadian entrainment | KEGG PATHWAY | hsa04713 | 1 | 95 | 0.196102 | 0.308577 | 0.510637 | ENSG00000087460 | http://www.genome.jp/kegg-bin/show_pathway?hsa04713/hsa:2778%09red |
ProgesteronE−mediated oocyte maturation | KEGG PATHWAY | hsa04914 | 1 | 97 | 0.199757 | 0.308577 | 0.510637 | ENSG00000002822 | http://www.genome.jp/kegg-bin/show_pathway?hsa04914/hsa:8379%09red |
Endocrine resistance | KEGG PATHWAY | hsa01522 | 1 | 99 | 0.203395 | 0.308577 | 0.510637 | ENSG00000087460 | http://www.genome.jp/kegg-bin/show_pathway?hsa01522/hsa:2778%09red |
Melanogenesis | KEGG PATHWAY | hsa04916 | 1 | 100 | 0.205207 | 0.308577 | 0.510637 | ENSG00000087460 | http://www.genome.jp/kegg-bin/show_pathway?hsa04916/hsa:2778%09red |
Amoebiasis | KEGG PATHWAY | hsa05146 | 1 | 100 | 0.205207 | 0.308577 | 0.510637 | ENSG00000087460 | http://www.genome.jp/kegg-bin/show_pathway?hsa05146/hsa:2778%09red |
Inflammatory mediator regulation of TRP channels | KEGG PATHWAY | hsa04750 | 1 | 101 | 0.207016 | 0.308577 | 0.510637 | ENSG00000087460 | http://www.genome.jp/kegg-bin/show_pathway?hsa04750/hsa:2778%09red |
Estrogen signaling pathway | KEGG PATHWAY | hsa04915 | 1 | 101 | 0.207016 | 0.308577 | 0.510637 | ENSG00000087460 | http://www.genome.jp/kegg-bin/show_pathway?hsa04915/hsa:2778%09red |
Glucagon signaling pathway | KEGG PATHWAY | hsa04922 | 1 | 102 | 0.208821 | 0.308577 | 0.510637 | ENSG00000087460 | http://www.genome.jp/kegg-bin/show_pathway?hsa04922/hsa:2778%09red |
Chagas disease (American trypanosomiasis) | KEGG PATHWAY | hsa05142 | 1 | 106 | 0.216 | 0.308577 | 0.510637 | ENSG00000087460 | http://www.genome.jp/kegg-bin/show_pathway?hsa05142/hsa:2778%09red |
Cholinergic synapse | KEGG PATHWAY | hsa04725 | 1 | 113 | 0.228409 | 0.308577 | 0.510637 | ENSG00000053918 | http://www.genome.jp/kegg-bin/show_pathway?hsa04725/hsa:3784%09red |
Serotonergic synapse | KEGG PATHWAY | hsa04726 | 1 | 113 | 0.228409 | 0.308577 | 0.510637 | ENSG00000087460 | http://www.genome.jp/kegg-bin/show_pathway?hsa04726/hsa:2778%09red |
Glutamatergic synapse | KEGG PATHWAY | hsa04724 | 1 | 115 | 0.231919 | 0.308577 | 0.510637 | ENSG00000087460 | http://www.genome.jp/kegg-bin/show_pathway?hsa04724/hsa:2778%09red |
Vascular smooth muscle contraction | KEGG PATHWAY | hsa04270 | 1 | 123 | 0.245803 | 0.308577 | 0.510637 | ENSG00000087460 | http://www.genome.jp/kegg-bin/show_pathway?hsa04270/hsa:2778%09red |
Cell cycle | KEGG PATHWAY | hsa04110 | 1 | 124 | 0.247521 | 0.308577 | 0.510637 | ENSG00000002822 | http://www.genome.jp/kegg-bin/show_pathway?hsa04110/hsa:8379%09red |
Platelet activation | KEGG PATHWAY | hsa04611 | 1 | 125 | 0.249235 | 0.308577 | 0.510637 | ENSG00000087460 | http://www.genome.jp/kegg-bin/show_pathway?hsa04611/hsa:2778%09red |
Dopaminergic synapse | KEGG PATHWAY | hsa04728 | 1 | 129 | 0.256053 | 0.309646 | 0.509135 | ENSG00000087460 | http://www.genome.jp/kegg-bin/show_pathway?hsa04728/hsa:2778%09red |
Phospholipase D signaling pathway | KEGG PATHWAY | hsa04072 | 1 | 146 | 0.284358 | 0.336059 | 0.473584 | ENSG00000087460 | http://www.genome.jp/kegg-bin/show_pathway?hsa04072/hsa:2778%09red |
Oxytocin signaling pathway | KEGG PATHWAY | hsa04921 | 1 | 160 | 0.306871 | 0.354606 | 0.450254 | ENSG00000087460 | http://www.genome.jp/kegg-bin/show_pathway?hsa04921/hsa:2778%09red |
Calcium signaling pathway | KEGG PATHWAY | hsa04020 | 1 | 179 | 0.336314 | 0.380181 | 0.42001 | ENSG00000087460 | http://www.genome.jp/kegg-bin/show_pathway?hsa04020/hsa:2778%09red |
cAMP signaling pathway | KEGG PATHWAY | hsa04024 | 1 | 201 | 0.368872 | 0.399073 | 0.398948 | ENSG00000087460 | http://www.genome.jp/kegg-bin/show_pathway?hsa04024/hsa:2778%09red |
Epstein-Barr virus infection | KEGG PATHWAY | hsa05169 | 1 | 204 | 0.373188 | 0.399073 | 0.398948 | ENSG00000068024 | http://www.genome.jp/kegg-bin/show_pathway?hsa05169/hsa:9759%09red |
Focal adhesion | KEGG PATHWAY | hsa04510 | 1 | 206 | 0.376049 | 0.399073 | 0.398948 | ENSG00000168477 | http://www.genome.jp/kegg-bin/show_pathway?hsa04510/hsa:7148%09red |
Rap1 signaling pathway | KEGG PATHWAY | hsa04015 | 1 | 216 | 0.390165 | 0.405771 | 0.391719 | ENSG00000087460 | http://www.genome.jp/kegg-bin/show_pathway?hsa04015/hsa:2778%09red |
PI3K-Akt signaling pathway | KEGG PATHWAY | hsa04151 | 1 | 343 | 0.544312 | 0.554985 | 0.255719 | ENSG00000168477 | http://www.genome.jp/kegg-bin/show_pathway?hsa04151/hsa:7148%09red |
Pathways in cancer | KEGG PATHWAY | hsa05200 | 1 | 399 | 0.599439 | 0.599439 | 0.222255 | ENSG00000087460 | http://www.genome.jp/kegg-bin/show_pathway?hsa05200/hsa:2778%09red |
Statistical test method: hypergeometric test/Fisher’s exact test. FDR correction method: Benjamini and Hochberg.
Table S4
Genes | Diff score | Chromosome | Position | Methylation status | P value (B vs. C) | P value (A vs. B) |
---|---|---|---|---|---|---|
ZNF323 | 131.89468 | 6 | 28431998 | High-GpG | 4.16001E−07 | 1.55395E−07 |
ASAH1 | 130.81353 | 8 | 17986262 | High-GpG | 2.01169E−07 | 4.1218E−07 |
FAM111A | 130.45708 | 11 | 58666572 | High-GpG | 1.21744E−05 | 7.39343E−09 |
MAD2L1 | 129.33632 | 4 | 121207392 | High-GpG | 5.45286E−07 | 2.1367E−07 |
ATG12 | 127.27827 | 5 | 115205360 | High-GpG | 8.27127E−07 | 2.26256E−07 |
ZNF28 | 127.26429 | 19 | 58016010 | High-GpG | 1.55587E−06 | 1.2067E−07 |
HADHB | 125.49116 | 2 | 26320955 | High-GpG | 4.79904E−06 | 5.88478E−08 |
PRKCG | 125.39503 | 19 | 59077027 | High-GpG | 2.97625E−05 | 9.70126E−09 |
PTGES2 | 124.99208 | 9 | 129930459 | High-GpG | 8.51945E−07 | 3.7186E−07 |
AK2 | 124.18006 | 1 | 33275020 | High-GpG | 1.48229E−07 | 2.57668E−06 |
MAGOHB | 123.44346 | 12 | 10657370 | High-GpG | 8.11057E−05 | 5.57958E−09 |
NFXL1 | 122.4017 | 4 | 47611255 | High-GpG | 1.88006E−06 | 3.05955E−07 |
MOBKL3 | 120.93052 | 2 | 198088826 | High-GpG | 2.86446E−06 | 2.81777E−07 |
FAM76A | 120.92863 | 1 | 27925162 | High-GpG | 1.06657E−06 | 7.57092E−07 |
SUPT4H1 | 119.87514 | 17 | 53784566 | High-GpG | 1.36289E−05 | 7.55137E−08 |
PFN4 | 118.79988 | 2 | 24199741 | High-GpG | 5.71282E−06 | 2.30761E−07 |
NTAN1 | 117.88967 | 16 | 15057701 | High-GpG | 1.57434E−06 | 1.03261E−06 |
ETNK1 | 117.59242 | 12 | 22669361 | High-GpG | 3.17717E−06 | 5.47921E−07 |
RALY | 117.18969 | 20 | 32046086 | High-GpG | 9.3884E−06 | 2.03442E−07 |
CCND1 | 116.8124 | 11 | 69164711 | High-GpG | 1.57231E−06 | 1.32502E−06 |
ASAP3 | 116.03716 | 1 | 23683861 | High-GpG | 7.38714E−05 | 3.37139E−08 |
ERCC4 | 115.92696 | 16 | 13921604 | High-GpG | 2.69769E−07 | 9.46918E−06 |
UBE2K | 115.73845 | 4 | 39375775 | High-GpG | 0.000128084 | 2.08286E−08 |
KIAA1324L | 115.34069 | 7 | 86526859 | High-GpG | 3.39017E−06 | 8.62402E−07 |
AP1AR | 115.30214 | 4 | 113372285 | High-GpG | 4.0348E−06 | 7.31079E−07 |
C1GALT1 | 114.78336 | 7 | 7188867 | High-GpG | 4.95185E−06 | 6.71268E−07 |
NCAPH | 114.77977 | 2 | 96365157 | High-GpG | 6.31399E−05 | 5.26889E−08 |
GCSH | 114.74176 | 16 | 79687498 | High-GpG | 4.30184E−07 | 7.80136E−06 |
DHCR24 | 114.43711 | 1 | 55125751 | High-GpG | 1.87238E−05 | 1.92263E−07 |
HNRNPA1 | 114.07533 | 12 | 52960808 | High-GpG | 0.000139304 | 2.80869E−08 |
GPR109A | −90.42935 | 12 | 121755189 | Low-CpG | 7.59402E−06 | 0.000119287 |
RGPD3 | −91.08637 | 2 | 106451234 | Low-CpG | 4.72388E−05 | 1.64841E−05 |
C17orf98 | −92.50327 | 17 | 34251672 | Low-CpG | 0.00010681 | 5.2609E−06 |
AP1B1 | −93.62296 | 22 | 28115355 | Low-CpG | 2.05317E−05 | 2.11484E−05 |
DSCR8 | −93.67115 | 21 | 38415359 | Low-CpG | 0.002349546 | 1.82768E−07 |
SNORD89 | −94.64155 | 2 | 101256138 | Low-CpG | 2.65111E−05 | 1.29544E−05 |
MTL5 | −95.11474 | 11 | 68275537 | Low-CpG | 8.07261E−06 | 3.81515E−05 |
WRB | −95.16274 | 21 | 39672973 | Low-CpG | 1.04278E−05 | 2.92101E−05 |
NALCN | −95.55199 | 13 | 100866990 | Low-CpG | 0.000157631 | 1.76669E−06 |
ZNF100 | −97.47202 | 19 | 21725295 | Low-CpG | 1.15163E−05 | 1.55412E−05 |
PHACTR4 | −97.70953 | 1 | 28567843 | Low-CpG | 7.41887E−06 | 2.28407E−05 |
GSTM4 | −97.92513 | 1 | 109998812 | Low-CpG | 9.94493E−06 | 1.62138E−05 |
CIB4 | −99.87754 | 2 | 26718375 | Low-CpG | 0.000282136 | 3.64575E−07 |
TMBIM4 | −100.22459 | 12 | 64851491 | Low-CpG | 3.88671E−06 | 2.4432E−05 |
LOC339535 | −103.632 | 1 | 236716068 | Low-CpG | 5.41422E−06 | 8.0032E−06 |
SNORD115-38 | −103.94379 | 15 | 23034641 | Low-CpG | 3.08714E−06 | 1.30636E−05 |
HCCA2 | −105.64957 | 11 | 1601150 | Low-CpG | 1.64093E−05 | 1.65941E−06 |
PARP4 | −105.90702 | 13 | 23979063 | Low-CpG | 2.30641E−05 | 1.11266E−06 |
DLGAP2 | −106.78054 | 8 | 1442761 | Low-CpG | 1.34894E−06 | 1.5558E−05 |
TMEM22 | −108.01178 | 3 | 138039519 | Low-CpG | 8.62848E−06 | 1.83184E−06 |
TCAM1 | −109.0501 | 17 | 59288076 | Low-CpG | 3.68175E−06 | 3.38015E−06 |
CEP63 | −111.64598 | 3 | 135690134 | Low-CpG | 0.000347835 | 1.96801E−08 |
PAK2 | −112.77409 | 3 | 197954174 | Low-CpG | 5.13802E−06 | 1.02753E−06 |
FAM9A | −112.86534 | X | 8729344 | Low-CpG | 2.79992E−06 | 1.84638E−06 |
CCDC83 | −114.29396 | 11 | 85246352 | Low-CpG | 5.92682E−06 | 6.27745E−07 |
SNORD114-15 | −115.87882 | 14 | 100508183 | Low-CpG | 9.26565E−06 | 2.78767E−07 |
CDCA7L | −120.37022 | 7 | 21930929 | Low-CpG | 2.20559E−06 | 4.16346E−07 |
WDR27 | −120.65469 | 6 | 169839513 | Low-CpG | 5.12794E−06 | 1.67721E−07 |
BECN1 | −124.31615 | 17 | 38230497 | Low-CpG | 6.43061E−06 | 5.75617E−08 |
AGAP11 | −138.8236 | 10 | 88746560 | Low-CpG | 8.04749E−08 | 1.62922E−07 |
Table S5
GOID | Ontology | Term | Level | q | m | t | k | Gene IDs | Symbols | Log odds ratio | P |
---|---|---|---|---|---|---|---|---|---|---|---|
GO: 0000045 | Biological process | Autophagosome assembly | 4 | 5 | 38 | 45,240 | 127 | C1IDX9, O94817, K7EPZ0, K7EQQ7, Q14457 | ATG12, ATG12, BECN1, BECN1, BECN1 | 5.550627 | 5.82E−05 |
GO: 0006914 | Biological process | Autophagy | 1 | 12 | 159 | 45,240 | 127 | C1IDX9, O94817, E7EV84, K7ELY9, K7EMA2, K7EN35, K7EPZ0, K7EQQ7, K7ER46, K7ERY0, K7ESG3, Q14457 | ATG12, ATG12, BECN1, BECN1, BECN1, BECN1, BECN1, BECN1, BECN1, BECN1, BECN1, BECN1 | 4.748706 | 3.84E−10 |
GO:1905037 | Biological process | Autophagosome organization | 2 | 5 | 38 | 45,240 | 127 | C1IDX9, O94817, K7EPZ0, K7EQQ7, Q14457 | ATG12, ATG12, BECN1, BECN1, BECN1 | 5.550627 | 5.82E−05 |
GO: 0003857 | Molecular function | 3-hydroxyacyl-CoA dehydrogenase activity | 1 | 4 | 13 | 45,240 | 127 | C9JE81, C9JEY0, C9K0M0, P55084 | HADHB, HADHB, HADHB, HADHB | 6.776187 | 3.76E−05 |
GO: 0003988 | Molecular function | Acetyl-CoA C-acyltransferase activity | 1 | 4 | 10 | 45,240 | 127 | C9JE81, C9JEY0, C9K0M0, P55084 | HADHB, HADHB, HADHB, HADHB | 7.154699 | 1.67E−05 |
GO: 0004300 | Molecular function | Enoyl-CoA hydratase activity | 1 | 4 | 8 | 45,240 | 127 | C9JE81, C9JEY0, C9K0M0, P55084 | HADHB, HADHB, HADHB, HADHB | 7.476627 | 6.38E−06 |
GO: 0016507 | Cellular component | Mitochondrial fatty acid beta-oxidation multienzyme complex | 8 | 4 | 5 | 45,240 | 127 | C9JE81, C9JEY0, C9K0M0, P55084 | HADHB, HADHB, HADHB, HADHB | 8.154699 | 6.42E−07 |
GO: 0036125 | Cellular component | Fatty acid beta-oxidation multienzyme complex | 1 | 4 | 5 | 45,240 | 127 | C9JE81, C9JEY0, C9K0M0, P55084 | HADHB, HADHB, HADHB, HADHB | 8.154699 | 6.42E−07 |
GO: 0016508 | Molecular function | Long-chain-enoyl-CoA hydratase activity | 1 | 4 | 6 | 45,240 | 127 | C9JE81, C9JEY0, C9K0M0, P55084 | HADHB, HADHB, HADHB, HADHB | 7.891664 | 1.6E−06 |
GO: 0016509 | Molecular function | Long-chain-3-hydroxyacyl-CoA dehydrogenase activity | 1 | 4 | 5 | 45,240 | 127 | C9JE81, C9JEY0, C9K0M0, P55084 | HADHB, HADHB, HADHB, HADHB | 8.154699 | 6.42E−07 |
GO: 0005960 | Cellular component | Glycine cleavage complex | 5 | 4 | 5 | 45,240 | 127 | H3BNV1, H3BQ30, H3BUG8, P23434 | GCSH, GCSH, GCSH, GCSH | 8.154699 | 6.42E−07 |
Statistical test method: hypergeometric test/Fisher’s exact test. FDR correction method: Benjamini and Hochberg.
Table S6
#Term | Database | ID | Input number | Background number | P value | Corrected P value | Input | Hyperlink |
---|---|---|---|---|---|---|---|---|
Regulation of autophagy | KEGG PATHWAY | hsa04140 | 2 | 40 | 0.023689 | 0.583858 | ENSG00000126581, ENSG00000145782 | http://www.genome.jp/kegg-bin/show_pathway?hsa04140/hsa:9140%09red/hsa:8678%09red |
Non-small cell lung cancer | KEGG PATHWAY | hsa05223 | 2 | 58 | 0.045599 | 0.583858 | ENSG00000126583, ENSG00000110092 | http://www.genome.jp/kegg-bin/show_pathway?hsa05223/hsa:595%09red/hsa:5582%09red |
Glioma | KEGG PATHWAY | hsa05214 | 2 | 67 | 0.058495 | 0.583858 | ENSG00000126583, ENSG00000110092 | http://www.genome.jp/kegg-bin/show_pathway?hsa05214/hsa:595%09red/hsa:5582%09red |
ErbB signaling pathway | KEGG PATHWAY | hsa04012 | 2 | 90 | 0.096076 | 0.583858 | ENSG00000126583, ENSG00000180370 | http://www.genome.jp/kegg-bin/show_pathway?hsa04012/hsa:5062%09red/hsa:5582%09red |
Fc gamma R-mediated phagocytosis | KEGG PATHWAY | hsa04666 | 2 | 96 | 0.106773 | 0.583858 | ENSG00000088280, ENSG00000126583 | http://www.genome.jp/kegg-bin/show_pathway?hsa04666/hsa:55616%09red/hsa:5582%09red |
Steroid biosynthesis | KEGG PATHWAY | hsa00100 | 1 | 20 | 0.112548 | 0.583858 | ENSG00000116133 | http://www.genome.jp/kegg-bin/show_pathway?hsa00100/hsa:1718%09red |
Focal adhesion | KEGG PATHWAY | hsa04510 | 3 | 206 | 0.11596 | 0.583858 | ENSG00000126583, ENSG00000180370, ENSG00000110092 | http://www.genome.jp/kegg-bin/show_pathway?hsa04510/hsa:595%09red/hsa:5062%09red/hsa:5582%09red |
Fatty acid elongation | KEGG PATHWAY | hsa00062 | 1 | 25 | 0.137434 | 0.583858 | ENSG00000138029 | http://www.genome.jp/kegg-bin/show_pathway?hsa00062/hsa:3032%09red |
Glyoxylate and dicarboxylate metabolism | KEGG PATHWAY | hsa00630 | 1 | 28 | 0.152032 | 0.583858 | ENSG00000140905 | http://www.genome.jp/kegg-bin/show_pathway?hsa00630/hsa:2653%09red |
Thyroid hormone signaling pathway | KEGG PATHWAY | hsa04919 | 2 | 121 | 0.154242 | 0.583858 | ENSG00000126583, ENSG00000110092 | http://www.genome.jp/kegg-bin/show_pathway?hsa04919/hsa:595%09red/hsa:5582%09red |
Thyroid cancer | KEGG PATHWAY | hsa05216 | 1 | 29 | 0.156843 | 0.583858 | ENSG00000110092 | http://www.genome.jp/kegg-bin/show_pathway?hsa05216/hsa:595%09red |
Sphingolipid signaling pathway | KEGG PATHWAY | hsa04071 | 2 | 123 | 0.1582 | 0.583858 | ENSG00000126583, ENSG00000104763 | http://www.genome.jp/kegg-bin/show_pathway?hsa04071/hsa:427%09red/hsa:5582%09red |
Cell cycle | KEGG PATHWAY | hsa04110 | 2 | 124 | 0.160187 | 0.583858 | ENSG00000110092, ENSG00000164109 | http://www.genome.jp/kegg-bin/show_pathway?hsa04110/hsa:595%09red/hsa:4085%09red |
Lysosome | KEGG PATHWAY | hsa04142 | 2 | 124 | 0.160187 | 0.583858 | ENSG00000104763, ENSG00000100280 | http://www.genome.jp/kegg-bin/show_pathway?hsa04142/hsa:162%09red/hsa:427%09red |
Mucin type O-Glycan biosynthesis | KEGG PATHWAY | hsa00512 | 1 | 31 | 0.166384 | 0.583858 | ENSG00000106392 | http://www.genome.jp/kegg-bin/show_pathway?hsa00512/hsa:56913%09red |
Base excision repair | KEGG PATHWAY | hsa03410 | 1 | 33 | 0.175818 | 0.583858 | ENSG00000102699 | http://www.genome.jp/kegg-bin/show_pathway?hsa03410/hsa:143%09red |
Apoptosis-multiple species | KEGG PATHWAY | hsa04215 | 1 | 33 | 0.175818 | 0.583858 | ENSG00000126581 | http://www.genome.jp/kegg-bin/show_pathway?hsa04215/hsa:8678%09red |
African trypanosomiasis | KEGG PATHWAY | hsa05143 | 1 | 34 | 0.180495 | 0.583858 | ENSG00000126583 | http://www.genome.jp/kegg-bin/show_pathway?hsa05143/hsa:5582%09red |
FoxO signaling pathway | KEGG PATHWAY | hsa04068 | 2 | 135 | 0.18232 | 0.583858 | ENSG00000110092, ENSG00000145782 | http://www.genome.jp/kegg-bin/show_pathway?hsa04068/hsa:9140%09red/hsa:595%09red |
Spliceosome | KEGG PATHWAY | hsa03040 | 2 | 136 | 0.184355 | 0.583858 | ENSG00000111196, ENSG00000135486 | http://www.genome.jp/kegg-bin/show_pathway?hsa03040/hsa:55110%09red/hsa:3178%09red |
Wnt signaling pathway | KEGG PATHWAY | hsa04310 | 2 | 142 | 0.196631 | 0.583858 | ENSG00000126583, ENSG00000110092 | http://www.genome.jp/kegg-bin/show_pathway?hsa04310/hsa:595%09red/hsa:5582%09red |
Glycine, serine and threonine metabolism | KEGG PATHWAY | hsa00260 | 1 | 40 | 0.20801 | 0.583858 | ENSG00000140905 | http://www.genome.jp/kegg-bin/show_pathway?hsa00260/hsa:2653%09red |
Hepatitis B | KEGG PATHWAY | hsa05161 | 2 | 148 | 0.209005 | 0.583858 | ENSG00000126583, ENSG00000110092 | http://www.genome.jp/kegg-bin/show_pathway?hsa05161/hsa:595%09red/hsa:5582%09red |
Bladder cancer | KEGG PATHWAY | hsa05219 | 1 | 41 | 0.212505 | 0.583858 | ENSG00000110092 | http://www.genome.jp/kegg-bin/show_pathway?hsa05219/hsa:595%09red |
AldosteronE−regulated sodium reabsorption | KEGG PATHWAY | hsa04960 | 1 | 41 | 0.212505 | 0.583858 | ENSG00000126583 | http://www.genome.jp/kegg-bin/show_pathway?hsa04960/hsa:5582%09red |
Fatty acid degradation | KEGG PATHWAY | hsa00071 | 1 | 45 | 0.230236 | 0.583858 | ENSG00000138029 | http://www.genome.jp/kegg-bin/show_pathway?hsa00071/hsa:3032%09red |
Oxytocin signaling pathway | KEGG PATHWAY | hsa04921 | 2 | 160 | 0.233969 | 0.583858 | ENSG00000126583, ENSG00000110092 | http://www.genome.jp/kegg-bin/show_pathway?hsa04921/hsa:595%09red/hsa:5582%09red |
Hedgehog signaling pathway | KEGG PATHWAY | hsa04340 | 1 | 46 | 0.234606 | 0.583858 | ENSG00000110092 | http://www.genome.jp/kegg-bin/show_pathway?hsa04340/hsa:595%09red |
Nucleotide excision repair | KEGG PATHWAY | hsa03420 | 1 | 46 | 0.234606 | 0.583858 | ENSG00000175595 | http://www.genome.jp/kegg-bin/show_pathway?hsa03420/hsa:2072%09red |
Endocrine and other factor-regulated calcium reabsorption | KEGG PATHWAY | hsa04961 | 1 | 47 | 0.238952 | 0.583858 | ENSG00000126583 | http://www.genome.jp/kegg-bin/show_pathway?hsa04961/hsa:5582%09red |
Sphingolipid metabolism | KEGG PATHWAY | hsa00600 | 1 | 47 | 0.238952 | 0.583858 | ENSG00000104763 | http://www.genome.jp/kegg-bin/show_pathway?hsa00600/hsa:427%09red |
Valine, leucine and isoleucine degradation | KEGG PATHWAY | hsa00280 | 1 | 48 | 0.243273 | 0.583858 | ENSG00000138029 | http://www.genome.jp/kegg-bin/show_pathway?hsa00280/hsa:3032%09red |
Fatty acid metabolism | KEGG PATHWAY | hsa01212 | 1 | 49 | 0.24757 | 0.583858 | ENSG00000138029 | http://www.genome.jp/kegg-bin/show_pathway?hsa01212/hsa:3032%09red |
Glutathione metabolism | KEGG PATHWAY | hsa00480 | 1 | 51 | 0.256091 | 0.583858 | ENSG00000168765 | http://www.genome.jp/kegg-bin/show_pathway?hsa00480/hsa:2948%09red |
RNA transport | KEGG PATHWAY | hsa03013 | 2 | 171 | 0.257003 | 0.583858 | ENSG00000153165, ENSG00000111196 | http://www.genome.jp/kegg-bin/show_pathway?hsa03013/hsa:653489%09red/hsa:55110%09red |
Endometrial cancer | KEGG PATHWAY | hsa05213 | 1 | 54 | 0.268693 | 0.583858 | ENSG00000110092 | http://www.genome.jp/kegg-bin/show_pathway?hsa05213/hsa:595%09red |
Fanconi anemia pathway | KEGG PATHWAY | hsa03460 | 1 | 56 | 0.276976 | 0.583858 | ENSG00000175595 | http://www.genome.jp/kegg-bin/show_pathway?hsa03460/hsa:2072%09red |
Viral myocarditis | KEGG PATHWAY | hsa05416 | 1 | 57 | 0.281083 | 0.583858 | ENSG00000110092 | http://www.genome.jp/kegg-bin/show_pathway?hsa05416/hsa:595%09red |
Acute myeloid leukemia | KEGG PATHWAY | hsa05221 | 1 | 59 | 0.289227 | 0.583858 | ENSG00000110092 | http://www.genome.jp/kegg-bin/show_pathway?hsa05221/hsa:595%09red |
Long-term depression | KEGG PATHWAY | hsa04730 | 1 | 61 | 0.297279 | 0.583858 | ENSG00000126583 | http://www.genome.jp/kegg-bin/show_pathway?hsa04730/hsa:5582%09red |
VEGF signaling pathway | KEGG PATHWAY | hsa04370 | 1 | 64 | 0.309188 | 0.583858 | ENSG00000126583 | http://www.genome.jp/kegg-bin/show_pathway?hsa04370/hsa:5582%09red |
Arachidonic acid metabolism | KEGG PATHWAY | hsa00590 | 1 | 64 | 0.309188 | 0.583858 | ENSG00000148334 | http://www.genome.jp/kegg-bin/show_pathway?hsa00590/hsa:80142%09red |
Colorectal cancer | KEGG PATHWAY | hsa05210 | 1 | 64 | 0.309188 | 0.583858 | ENSG00000110092 | http://www.genome.jp/kegg-bin/show_pathway?hsa05210/hsa:595%09red |
Shigellosis | KEGG PATHWAY | hsa05131 | 1 | 66 | 0.317016 | 0.583858 | ENSG00000176732 | http://www.genome.jp/kegg-bin/show_pathway?hsa05131/hsa:375189%09red |
Long-term potentiation | KEGG PATHWAY | hsa04720 | 1 | 66 | 0.317016 | 0.583858 | ENSG00000126583 | http://www.genome.jp/kegg-bin/show_pathway?hsa04720/hsa:5582%09red |
Amphetamine addiction | KEGG PATHWAY | hsa05031 | 1 | 67 | 0.320896 | 0.583858 | ENSG00000126583 | http://www.genome.jp/kegg-bin/show_pathway?hsa05031/hsa:5582%09red |
Pancreatic cancer | KEGG PATHWAY | hsa05212 | 1 | 68 | 0.324755 | 0.583858 | ENSG00000110092 | http://www.genome.jp/kegg-bin/show_pathway?hsa05212/hsa:595%09red |
Drug metabolism-cytochrome P450 | KEGG PATHWAY | hsa00982 | 1 | 68 | 0.324755 | 0.583858 | ENSG00000168765 | http://www.genome.jp/kegg-bin/show_pathway?hsa00982/hsa:2948%09red |
Renal cell carcinoma | KEGG PATHWAY | hsa05211 | 1 | 69 | 0.328592 | 0.583858 | ENSG00000180370 | http://www.genome.jp/kegg-bin/show_pathway?hsa05211/hsa:5062%09red |
p53 signaling pathway | KEGG PATHWAY | hsa04115 | 1 | 69 | 0.328592 | 0.583858 | ENSG00000110092 | http://www.genome.jp/kegg-bin/show_pathway?hsa04115/hsa:595%09red |
RIG-I-like receptor signaling pathway | KEGG PATHWAY | hsa04622 | 1 | 70 | 0.332408 | 0.583858 | ENSG00000145782 | http://www.genome.jp/kegg-bin/show_pathway?hsa04622/hsa:9140%09red |
Proteoglycans in cancer | KEGG PATHWAY | hsa05205 | 2 | 208 | 0.33428 | 0.583858 | ENSG00000126583, ENSG00000110092 | http://www.genome.jp/kegg-bin/show_pathway?hsa05205/hsa:595%09red/hsa:5582%09red |
Thyroid hormone synthesis | KEGG PATHWAY | hsa04918 | 1 | 71 | 0.336201 | 0.583858 | ENSG00000126583 | http://www.genome.jp/kegg-bin/show_pathway?hsa04918/hsa:5582%09red |
Metabolism of xenobiotics by cytochrome P450 | KEGG PATHWAY | hsa00980 | 1 | 72 | 0.339974 | 0.583858 | ENSG00000168765 | http://www.genome.jp/kegg-bin/show_pathway?hsa00980/hsa:2948%09red |
Melanoma | KEGG PATHWAY | hsa05218 | 1 | 73 | 0.343725 | 0.583858 | ENSG00000110092 | http://www.genome.jp/kegg-bin/show_pathway?hsa05218/hsa:595%09red |
Gastric acid secretion | KEGG PATHWAY | hsa04971 | 1 | 74 | 0.347455 | 0.583858 | ENSG00000126583 | http://www.genome.jp/kegg-bin/show_pathway?hsa04971/hsa:5582%09red |
Prolactin signaling pathway | KEGG PATHWAY | hsa04917 | 1 | 74 | 0.347455 | 0.583858 | ENSG00000110092 | http://www.genome.jp/kegg-bin/show_pathway?hsa04917/hsa:595%09red |
Rap1 signaling pathway | KEGG PATHWAY | hsa04015 | 2 | 216 | 0.350752 | 0.583858 | ENSG00000176732, ENSG00000126583 | http://www.genome.jp/kegg-bin/show_pathway?hsa04015/hsa:375189%09red/hsa:5582%09red |
Chronic myeloid leukemia | KEGG PATHWAY | hsa05220 | 1 | 75 | 0.351164 | 0.583858 | ENSG00000110092 | http://www.genome.jp/kegg-bin/show_pathway?hsa05220/hsa:595%09red |
Platinum drug resistance | KEGG PATHWAY | hsa01524 | 1 | 76 | 0.354852 | 0.583858 | ENSG00000168765 | http://www.genome.jp/kegg-bin/show_pathway?hsa01524/hsa:2948%09red |
Regulation of actin cytoskeleton | KEGG PATHWAY | hsa04810 | 2 | 219 | 0.356894 | 0.583858 | ENSG00000176732, ENSG00000180370 | http://www.genome.jp/kegg-bin/show_pathway?hsa04810/hsa:375189%09red/hsa:5062%09red |
Aldosterone synthesis and secretion | KEGG PATHWAY | hsa04925 | 1 | 80 | 0.369397 | 0.583858 | ENSG00000126583 | http://www.genome.jp/kegg-bin/show_pathway?hsa04925/hsa:5582%09red |
Chemical carcinogenesis | KEGG PATHWAY | hsa05204 | 1 | 82 | 0.376547 | 0.583858 | ENSG00000168765 | http://www.genome.jp/kegg-bin/show_pathway?hsa05204/hsa:2948%09red |
EGFR tyrosine kinase inhibitor resistance | KEGG PATHWAY | hsa01521 | 1 | 83 | 0.380091 | 0.583858 | ENSG00000126583 | http://www.genome.jp/kegg-bin/show_pathway?hsa01521/hsa:5582%09red |
Ras signaling pathway | KEGG PATHWAY | hsa04014 | 2 | 231 | 0.381248 | 0.583858 | ENSG00000126583, ENSG00000180370 | http://www.genome.jp/kegg-bin/show_pathway?hsa04014/hsa:5062%09red/hsa:5582%09red |
Salmonella infection | KEGG PATHWAY | hsa05132 | 1 | 86 | 0.390606 | 0.583858 | ENSG00000176732 | http://www.genome.jp/kegg-bin/show_pathway?hsa05132/hsa:375189%09red |
Insulin secretion | KEGG PATHWAY | hsa04911 | 1 | 87 | 0.394071 | 0.583858 | ENSG00000126583 | http://www.genome.jp/kegg-bin/show_pathway?hsa04911/hsa:5582%09red |
GABAergic synapse | KEGG PATHWAY | hsa04727 | 1 | 88 | 0.397517 | 0.583858 | ENSG00000126583 | http://www.genome.jp/kegg-bin/show_pathway?hsa04727/hsa:5582%09red |
Small cell lung cancer | KEGG PATHWAY | hsa05222 | 1 | 88 | 0.397517 | 0.583858 | ENSG00000110092 | http://www.genome.jp/kegg-bin/show_pathway?hsa05222/hsa:595%09red |
Gap junction | KEGG PATHWAY | hsa04540 | 1 | 88 | 0.397517 | 0.583858 | ENSG00000126583 | http://www.genome.jp/kegg-bin/show_pathway?hsa04540/hsa:5582%09red |
Salivary secretion | KEGG PATHWAY | hsa04970 | 1 | 90 | 0.40435 | 0.583858 | ENSG00000126583 | http://www.genome.jp/kegg-bin/show_pathway?hsa04970/hsa:5582%09red |
Prostate cancer | KEGG PATHWAY | hsa05215 | 1 | 91 | 0.407737 | 0.583858 | ENSG00000110092 | http://www.genome.jp/kegg-bin/show_pathway?hsa05215/hsa:595%09red |
Morphine addiction | KEGG PATHWAY | hsa05032 | 1 | 91 | 0.407737 | 0.583858 | ENSG00000126583 | http://www.genome.jp/kegg-bin/show_pathway?hsa05032/hsa:5582%09red |
mRNA surveillance pathway | KEGG PATHWAY | hsa03015 | 1 | 91 | 0.407737 | 0.583858 | ENSG00000111196 | http://www.genome.jp/kegg-bin/show_pathway?hsa03015/hsa:55110%09red |
Metabolic pathways | KEGG PATHWAY | hsa01100 | 8 | 1240 | 0.412411 | 0.583858 | ENSG00000139163, ENSG00000004455, ENSG00000148334, ENSG00000116133, ENSG00000106392, ENSG00000104763, ENSG00000138029, ENSG00000140905 | http://www.genome.jp/kegg-bin/show_pathway?hsa01100/hsa:2653%09red/hsa:427%09red/hsa:56913%09red/hsa:55500%09red/hsa:3032%09red/hsa:80142%09red/hsa:1718%09red/hsa:204%09red |
Circadian entrainment | KEGG PATHWAY | hsa04713 | 1 | 95 | 0.421098 | 0.583858 | ENSG00000126583 | http://www.genome.jp/kegg-bin/show_pathway?hsa04713/hsa:5582%09red |
Pancreatic secretion | KEGG PATHWAY | hsa04972 | 1 | 96 | 0.424391 | 0.583858 | ENSG00000126583 | http://www.genome.jp/kegg-bin/show_pathway?hsa04972/hsa:5582%09red |
Glycerophospholipid metabolism | KEGG PATHWAY | hsa00564 | 1 | 96 | 0.424391 | 0.583858 | ENSG00000139163 | http://www.genome.jp/kegg-bin/show_pathway?hsa00564/hsa:55500%09red |
Progesterone-mediated oocyte maturation | KEGG PATHWAY | hsa04914 | 1 | 97 | 0.427665 | 0.583858 | ENSG00000164109 | http://www.genome.jp/kegg-bin/show_pathway?hsa04914/hsa:4085%09red |
MAPK signaling pathway | KEGG PATHWAY | hsa04010 | 2 | 257 | 0.432595 | 0.583858 | ENSG00000126583, ENSG00000180370 | http://www.genome.jp/kegg-bin/show_pathway?hsa04010/hsa:5062%09red/hsa:5582%09red |
Endocrine resistance | KEGG PATHWAY | hsa01522 | 1 | 99 | 0.434158 | 0.583858 | ENSG00000110092 | http://www.genome.jp/kegg-bin/show_pathway?hsa01522/hsa:595%09red |
HTLV-I infection | KEGG PATHWAY | hsa05166 | 2 | 259 | 0.436454 | 0.583858 | ENSG00000110092, ENSG00000164109 | http://www.genome.jp/kegg-bin/show_pathway?hsa05166/hsa:595%09red/hsa:4085%09red |
Amoebiasis | KEGG PATHWAY | hsa05146 | 1 | 100 | 0.437378 | 0.583858 | ENSG00000126583 | http://www.genome.jp/kegg-bin/show_pathway?hsa05146/hsa:5582%09red |
Melanogenesis | KEGG PATHWAY | hsa04916 | 1 | 100 | 0.437378 | 0.583858 | ENSG00000126583 | http://www.genome.jp/kegg-bin/show_pathway?hsa04916/hsa:5582%09red |
Inflammatory mediator regulation of TRP channels | KEGG PATHWAY | hsa04750 | 1 | 101 | 0.440579 | 0.583858 | ENSG00000126583 | http://www.genome.jp/kegg-bin/show_pathway?hsa04750/hsa:5582%09red |
Retrograde endocannabinoid signaling | KEGG PATHWAY | hsa04723 | 1 | 101 | 0.440579 | 0.583858 | ENSG00000126583 | http://www.genome.jp/kegg-bin/show_pathway?hsa04723/hsa:5582%09red |
Phosphatidylinositol signaling system | KEGG PATHWAY | hsa04070 | 1 | 101 | 0.440579 | 0.583858 | ENSG00000126583 | http://www.genome.jp/kegg-bin/show_pathway?hsa04070/hsa:5582%09red |
AGE−RAGE signaling pathway in diabetic complications | KEGG PATHWAY | hsa04933 | 1 | 103 | 0.446926 | 0.583858 | ENSG00000110092 | http://www.genome.jp/kegg-bin/show_pathway?hsa04933/hsa:595%09red |
Choline metabolism in cancer | KEGG PATHWAY | hsa05231 | 1 | 104 | 0.450074 | 0.583858 | ENSG00000126583 | http://www.genome.jp/kegg-bin/show_pathway?hsa05231/hsa:5582%09red |
HIF-1 signaling pathway | KEGG PATHWAY | hsa04066 | 1 | 105 | 0.453203 | 0.583858 | ENSG00000126583 | http://www.genome.jp/kegg-bin/show_pathway?hsa04066/hsa:5582%09red |
T cell receptor signaling pathway | KEGG PATHWAY | hsa04660 | 1 | 107 | 0.459408 | 0.583858 | ENSG00000180370 | http://www.genome.jp/kegg-bin/show_pathway?hsa04660/hsa:5062%09red |
MicroRNAs in cancer | KEGG PATHWAY | hsa05206 | 2 | 273 | 0.46306 | 0.583858 | ENSG00000126583, ENSG00000110092 | http://www.genome.jp/kegg-bin/show_pathway?hsa05206/hsa:595%09red/hsa:5582%09red |
Serotonergic synapse | KEGG PATHWAY | hsa04726 | 1 | 113 | 0.477608 | 0.589389 | ENSG00000126583 | http://www.genome.jp/kegg-bin/show_pathway?hsa04726/hsa:5582%09red |
Cholinergic synapse | KEGG PATHWAY | hsa04725 | 1 | 113 | 0.477608 | 0.589389 | ENSG00000126583 | http://www.genome.jp/kegg-bin/show_pathway?hsa04725/hsa:5582%09red |
Glutamatergic synapse | KEGG PATHWAY | hsa04724 | 1 | 115 | 0.483539 | 0.590426 | ENSG00000126583 | http://www.genome.jp/kegg-bin/show_pathway?hsa04724/hsa:5582%09red |
Oocyte meiosis | KEGG PATHWAY | hsa04114 | 1 | 120 | 0.498074 | 0.595635 | ENSG00000164109 | http://www.genome.jp/kegg-bin/show_pathway?hsa04114/hsa:4085%09red |
Leukocyte transendothelial migration | KEGG PATHWAY | hsa04670 | 1 | 120 | 0.498074 | 0.595635 | ENSG00000126583 | http://www.genome.jp/kegg-bin/show_pathway?hsa04670/hsa:5582%09red |
Vascular smooth muscle contraction | KEGG PATHWAY | hsa04270 | 1 | 123 | 0.506599 | 0.599648 | ENSG00000126583 | http://www.genome.jp/kegg-bin/show_pathway?hsa04270/hsa:5582%09red |
AMPK signaling pathway | KEGG PATHWAY | hsa04152 | 1 | 125 | 0.512203 | 0.600157 | ENSG00000110092 | http://www.genome.jp/kegg-bin/show_pathway?hsa04152/hsa:595%09red |
Dopaminergic synapse | KEGG PATHWAY | hsa04728 | 1 | 129 | 0.523221 | 0.604046 | ENSG00000126583 | http://www.genome.jp/kegg-bin/show_pathway?hsa04728/hsa:5582%09red |
Natural killer cell mediated cytotoxicity | KEGG PATHWAY | hsa04650 | 1 | 130 | 0.525936 | 0.604046 | ENSG00000126583 | http://www.genome.jp/kegg-bin/show_pathway?hsa04650/hsa:5582%09red |
Ubiquitin mediated proteolysis | KEGG PATHWAY | hsa04120 | 1 | 137 | 0.54452 | 0.613122 | ENSG00000078140 | http://www.genome.jp/kegg-bin/show_pathway?hsa04120/hsa:3093%09red |
Measles | KEGG PATHWAY | hsa05162 | 1 | 138 | 0.547116 | 0.613122 | ENSG00000110092 | http://www.genome.jp/kegg-bin/show_pathway?hsa05162/hsa:595%09red |
Tight junction | KEGG PATHWAY | hsa04530 | 1 | 139 | 0.549696 | 0.613122 | ENSG00000126583 | http://www.genome.jp/kegg-bin/show_pathway?hsa04530/hsa:5582%09red |
Apoptosis | KEGG PATHWAY | hsa04210 | 1 | 142 | 0.55735 | 0.615739 | ENSG00000102699 | http://www.genome.jp/kegg-bin/show_pathway?hsa04210/hsa:143%09red |
Hippo signaling pathway | KEGG PATHWAY | hsa04390 | 1 | 153 | 0.584325 | 0.638598 | ENSG00000110092 | http://www.genome.jp/kegg-bin/show_pathway?hsa04390/hsa:595%09red |
mTOR signaling pathway | KEGG PATHWAY | hsa04150 | 1 | 155 | 0.589052 | 0.638598 | ENSG00000126583 | http://www.genome.jp/kegg-bin/show_pathway?hsa04150/hsa:5582%09red |
Jak-STAT signaling pathway | KEGG PATHWAY | hsa04630 | 1 | 160 | 0.600635 | 0.645126 | ENSG00000110092 | http://www.genome.jp/kegg-bin/show_pathway?hsa04630/hsa:595%09red |
Purine metabolism | KEGG PATHWAY | hsa00230 | 1 | 177 | 0.637645 | 0.670677 | ENSG00000004455 | http://www.genome.jp/kegg-bin/show_pathway?hsa00230/hsa:204%09red |
Axon guidance | KEGG PATHWAY | hsa04360 | 1 | 178 | 0.639713 | 0.670677 | ENSG00000180370 | http://www.genome.jp/kegg-bin/show_pathway?hsa04360/hsa:5062%09red |
Calcium signaling pathway | KEGG PATHWAY | hsa04020 | 1 | 179 | 0.641769 | 0.670677 | ENSG00000126583 | http://www.genome.jp/kegg-bin/show_pathway?hsa04020/hsa:5582%09red |
Pathways in cancer | KEGG PATHWAY | hsa05200 | 2 | 399 | 0.665937 | 0.689721 | ENSG00000126583, ENSG00000110092 | http://www.genome.jp/kegg-bin/show_pathway?hsa05200/hsa:595%09red/hsa:5582%09red |
cAMP signaling pathway | KEGG PATHWAY | hsa04024 | 1 | 201 | 0.684166 | 0.702329 | ENSG00000182782 | http://www.genome.jp/kegg-bin/show_pathway?hsa04024/hsa:338442%09red |
Viral carcinogenesis | KEGG PATHWAY | hsa05203 | 1 | 207 | 0.694837 | 0.707027 | ENSG00000110092 | http://www.genome.jp/kegg-bin/show_pathway?hsa05203/hsa:595%09red |
Endocytosis | KEGG PATHWAY | hsa04144 | 1 | 264 | 0.779936 | 0.786718 | ENSG00000088280 | http://www.genome.jp/kegg-bin/show_pathway?hsa04144/hsa:55616%09red |
PI3K-Akt signaling pathway | KEGG PATHWAY | hsa04151 | 1 | 343 | 0.860284 | 0.860284 | ENSG00000110092 | http://www.genome.jp/kegg-bin/show_pathway?hsa04151/hsa:595%09red |
Statistical test method: hypergeometric test/Fisher’s exact test. FDR correction method: Benjamini and Hochberg.
Acknowledgments
We also thanks for native English expert in Medical Communications Department in Fresta Technologies Co, Ltd. (www.4upub.com) to polish the language.
Funding: This study was supported by
Footnote
Conflicts of Interest: The authors have completed the ICMJE uniform disclosure form (available at http://dx.doi.org/10.21037/tcr.2019.11.26). The authors have no conflicts of interest to declare.
Ethical Statement: The authors are accountable for all aspects of the work in ensuring that questions related to the accuracy or integrity of any part of the work are appropriately investigated and resolved. The study was conducted in accordance with the Declaration of Helsinki (as revised in 2013). This study was approved by the Ethics Committee of Chinese General Hospital of PLA. All patients provided signed informed consent.
Open Access Statement: This is an Open Access article distributed in accordance with the Creative Commons Attribution-NonCommercial-NoDerivs 4.0 International License (CC BY-NC-ND 4.0), which permits the non-commercial replication and distribution of the article with the strict proviso that no changes or edits are made and the original work is properly cited (including links to both the formal publication through the relevant DOI and the license). See: https://creativecommons.org/licenses/by-nc-nd/4.0/.
References
- Jemal A, Siegel R, Ward E, et al. Cancer statistics, 2008. CA Cancer J Clin 2008;58:71-96. [Crossref] [PubMed]
- Li D, Xie K, Wolff R, et al. Pancreatic cancer. Lancet 2004;363:1049-57. [Crossref] [PubMed]
- Hassan MM, Bondy ML, Wolff RA, et al. Risk factors for pancreatic cancer: case-control study. Am J Gastroenterol 2007;102:2696-707. [Crossref] [PubMed]
- Batty GD, Kivimaki M, Morrison D, et al. Risk factors for pancreatic cancer mortality: extended follow-up of the original Whitehall Study. Cancer Epidemiol Biomarkers Prev 2009;18:673-5. [Crossref] [PubMed]
- Landi S. Genetic predisposition and environmental risk factors to pancreatic cancer: A review of the literature. Mutat Res 2009;681:299-307. [Crossref] [PubMed]
- Hartwig W, Strobel O, Hinz U, et al. CA19-9 in potentially resectable pancreatic cancer: perspective to adjust surgical and perioperative therapy. Ann Surg Oncol 2013;20:2188-96. [Crossref] [PubMed]
- Kim JE, Lee KT, Lee JK, et al. Clinical usefulness of carbohydrate antigen 19-9 as a screening test for pancreatic cancer in an asymptomatic population. J Gastroenterol Hepatol 2004;19:182-6. [Crossref] [PubMed]
- Petronis A, Gottesman II, Crow TJ, et al. Psychiatric epigenetics: a new focus for the new century. Mol Psychiatry 2000;5:342-6. [Crossref] [PubMed]
- Yi JM, Guzzetta AA, Bailey VJ, et al. Novel methylation biomarker panel for the early detection of pancreatic cancer. Clin Cancer Res 2013;19:6544-55. [Crossref] [PubMed]
- Jiao L, Zhu J, Hassan MM, et al. K-ras mutation and p16 and preproenkephalin promoter hypermethylation in plasma DNA of pancreatic cancer patients: in relation to cigarette smoking. Pancreas 2007;34:55-62. [Crossref] [PubMed]
- Alvarez H, Opalinska J, Zhou L, et al. Widespread hypomethylation occurs early and synergizes with gene amplification during esophageal carcinogenesis. PLoS Genet 2011;7:e1001356. [Crossref] [PubMed]
- Nones K, Waddell N, Song S, et al. Genome-wide DNA methylation patterns in pancreatic ductal adenocarcinoma reveal epigenetic deregulation of SLIT-ROBO, ITGA2 and MET signaling. Int J Cancer 2014;135:1110-8. [Crossref] [PubMed]
- Ehrlich M. DNA methylation in cancer: too much, but also too little. Oncogene 2002;21:5400-13. [Crossref] [PubMed]
- Feinberg AP, Gehrke CW, Kuo KC, et al. Reduced genomic 5-methylcytosine content in human colonic neoplasia. Cancer Res 1988;48:1159-61. [PubMed]
- Dedeurwaerder S, Defrance M, Calonne E, et al. Evaluation of the Infinium Methylation 450K technology. Epigenomics 2011;3:771-84. [Crossref] [PubMed]
- Sandoval J, Heyn H, Moran S, et al. Validation of a DNA methylation microarray for 450,000 CpG sites in the human genome. Epigenetics 2011;6:692-702. [Crossref] [PubMed]
- Sun Z, Chai HS, Wu Y, et al. Batch effect correction for genome-wide methylation data with Illumina Infinium platform. BMC Med Genomics 2011;4:84. [Crossref] [PubMed]
- Zheng Q, Wang XJ. GOEAST: a web-based software toolkit for Gene Ontology enrichment analysis. Nucleic Acids Res 2008;36:W358-63. [Crossref] [PubMed]
- Mao X, Cai T, Olyarchuk JG, et al. Automated genome annotation and pathway identification using the KEGG Orthology (KO) as a controlled vocabulary. Bioinformatics 2005;21:3787-93. [Crossref] [PubMed]
- Wang LG, Ni Y, Su BH, et al. MicroRNA-34b functions as a tumor suppressor and acts as a nodal point in the feedback loop with Met. Int J Oncol 2013;42:957-62. [Crossref] [PubMed]
- Jin S, Mu Y, Wang X, et al. Overexpressed RACK1 is positively correlated with malignant degree of human colorectal carcinoma. Mol Biol Rep 2014;41:3393-9. [Crossref] [PubMed]
- Wang Z, Zhang L, Zhang D, et al. Glycolysis inhibitor 2-deoxy-D-glucose suppresses carcinogen-induced rat hepatocarcinogenesis by restricting cancer cell metabolism. Mol Med Rep 2015;11:1917-24. [Crossref] [PubMed]
- Irizarry RA, Ladd-Acosta C, Wen B, et al. The human colon cancer methylome shows similar hypo- and hypermethylation at conserved tissue-specific CpG island shores. Nat Genet 2009;41:178-86. [Crossref] [PubMed]
- Illingworth RS, Gruenewald-Schneider U, Webb S, et al. Orphan CpG islands identify numerous conserved promoters in the mammalian genome. PLoS Genet 2010;6:e1001134. [Crossref] [PubMed]
- Maunakea AK, Nagarajan RP, Bilenky M, et al. Conserved role of intragenic DNA methylation in regulating alternative promoters. Nature 2010;466:253-7. [Crossref] [PubMed]
- Deaton AM, Webb S, Kerr AR, et al. Cell type-specific DNA methylation at intragenic CpG islands in the immune system. Genome Res 2011;21:1074-86. [Crossref] [PubMed]
- Ebert MP, Hernberg S, Fei G, et al. Induction and expression of cyclin D3 in human pancreatic cancer. J Cancer Res Clin Oncol 2001;127:449-54. [Crossref] [PubMed]
- Gansauge S, Gansauge F, Ramadani M, et al. Overexpression of cyclin D1 in human pancreatic carcinoma is associated with poor prognosis. Cancer Res 1997;57:1634-7. [PubMed]
- Bhutani N, Burns DM, Blau HM. DNA demethylation dynamics. Cell 2011;146:866-72. [Crossref] [PubMed]