Identification of key protein-coding genes in lung adenocarcinomas based on bioinformatic analysis
Introduction
Nowadays, lung cancer remains a major type of malignancies with high death rate all around the word (1). Small cell lung cancer and non-small cell lung cancer are two histologic subtypes of lung carcinoma, and non-small cell carcinoma accounts for >85% of lung cancer (2). Lung adenocarcinomas is featured with frequent recurrence, and even at the first diagnosis, metastasis is easily detected in the patients (3). The prognosis is still poor and not satisfying, although many therapeutic modalities have been applied to the treatment of lung adenocarcinomas in recent years (4). The identification of biomarkers is of great significance for lung adenocarcinomas. Therefore, it is greatly needed to identify biomarkers and therapeutic targets in lung adenocarcinomas for better diagnose and treatment. The bioinformatics analyses is an effective way for detecting genome-wide gene expression, and The Cancer Genome Atlas (TCGA) and the Gene Expression Omnibus (GEO) are Cancer-related public genome data databases used to re-analyze gene expression data from different research institute. So the bioinformatics analysis is effective for finding potential biomarker closely related to disease by studying some expression profile datasets of some disease online, using different bioinformatics analysis tools (such as GO analysis and KEGG analysis). In this study, we extracted data from GSE32863, GSE43458 and GSE63459 datasets, and screened 242 common differentially expressed genes between lung adenocarcinomas and normal pulmonary tissues. The function and pathway enrichment of DEGS were analyzed on GO and KEGG online databases, and their protein-protein interaction network was constructed by STRING online database. Furthermore, quantitative real-time PCR was used to identify the bioinformatic analysis.
Methods
Our study was approved by the Ethics Committee of the Affiliated Hospital of Qingdao University (No.2018015). Each patient was informed of the use of their tissue specimens.
Identification of feature genes
The expression profile datasets were gained from the Gene Expression Omnibus (GEO; http://www.ncbi.nlm.nih.gov/geo/), which is a public genomics data repository (5,6). We selected three gene expression profile datasets (GSE32863, GSE43458 and GSE63459). GES63459 consists of 32 normal samples and 33 lung adenocarcinoma samples. GSE43458 consists of 30 normal samples and 80 lung adenocarcinoma samples, GSE32863 consists of 58 normal samples and 58 lung adenocarcinoma samples. The GEO2R (http://www.ncbi.nlm.nih.gov/geo/geo2r/) is a tool which can compare different groups of samples and identify DEGs in a GEO database. To identify the DEGs in lung adenocarcinomas, GEO data were divided into two groups, and GEO2R was used to compare them. P-value (adj. P-value) was adjusted using Benjamini-Hochberg method. |logFC| >1 and <0.05 were considered as the criteria to identify DEGs between normal tissue samples and lung adenocarcinoma samples.
Co-expression network construction
The online tool STRNG database and Cytoscape software (www.cytoscape.org) (7) were used to establish the protein-protein interaction (PPI) network. Input of differentially expressed genes on the STRNG database to predict their interactions. Hub genes were identified from PPIs, and then we gained the edge length between nodes from these hub genes. Hub genes are a small part of a protein that interacts with other proteins
Bioinformatic analysis
Based on the Database for Annotation, Visualization and Integrated Discovery (DAVID) (https://david.ncifcrf.gov), Gene Ontology (GO) and Kyoto Encyclopedia of Genes and Genomes (KEGG) were used to analyze the biological function of DEGs. The enrichment threshold values were considered significant at P<0.01.
Survival analysis of patients
To identify the clinical value of differentially expressed genes, Kaplan-Meier curve and Cox regression analysis were performed. Kaplan-Meier is an online tool, and can predicts the effect of genes on survival while submit interested genes. The samples were divided into high expression group and low expression group. The ONCOMINE database (http://www.cytoscape.org) and Kaplan-Meier Plotter database are employed to perform all of the statistical analysis (8). Statistically significant was assessed using student’s t-test, P<0.05 was considered significance.
Quantitative real-time PCR
The expression levels of ASPM, CCNB2, CDC20, CDC45, MELK, PRC1, TOP2A, UBE2T and KIAA0101 in lung adenocarcinoma tissues were detected by qRT-PCR. We collected seven pairs of lung cancer and adjacent normal tissues. The specimens were excised and immediately frozen in liquid nitrogen and stored at −80 °C. Total RNA was retrieved into cDNA by reverse transcription kit (Tiangen, Beijing). qRT-PCR was performed using a SYBR Green PCR Kit (Tiangen, Beijing) and ABI StepOne Plus thermal circulator. The primer sequences are shown in Table 1. All specimens were analyzed in three copies. Data were calculated based on the comparative 2-ΔΔCT method.
Table 1
Gene and direction | Sequence |
---|---|
GAPDH-F | ATCAC TGCCACCCAGAAGAC |
GAPDH-R | TTTCTAGAC GGCAGGTCAGG |
CDC45-F | CTTGAAGTTCCCGCCTATGAAG |
CDC45-R | GCATGGTTTGCTCCACTATCTC |
PRC1- F | ATCACCTTCGGGAAATATGGGA |
PRC1-R | TCTTTCTGACAGACGGATATGCT |
CCNB2-F | TTGGCTGGTACAAGTCCACTC |
CCNB2-R | TGGGAACTGGTATAAGCATTGTC |
KIAA0101-F | ATGGTGCGGACTAAAGCAGAC |
KIAA0101-R | CCTCGATGAAACTGATGTCGAAT |
ASPM-F | TGCAGTGGGTGAACATGAAAA |
ASPM-R | CGAAGAGGGTGTTACCTCGTTT |
UBE2T-F | ATCCCTCAACATCGCAACTGT |
UBE2T-R | CAGCCTCTGGTAGATTATCAAGC |
MELK-F | AACTCCAGCCTTATGCAGAAC |
MELK-R | AACGATTTGGCGTAGTGAGTATT |
TOP2A-F | ACCATTGCAGCCTGTAAATGA |
TOP2A-R | GGGCGGAGCAAAATATGTTCC |
CDC20-F | GCTTTGAACCTGAACGGTTTTG |
CDC20-R | TCTGGCGCATTTTGTGGTTTT |
Results
Identification of DEGs and bioinformatic analysis
We obtained 1,264, 896 and 408 DGEs in the datasets GSE32863, GES43458 and GSE63459, respectively, identified 242 genes expressed in all the three datasets (Figure 1), and the up-regulated and down-regulated genes were shown in the volcano plot (Figure 2), logFC >1 and P<0.05 were considered as significantly up-regulated genes, logFC <−1 and P<0.05 were considered as significantly down-regulated genes. Gene symbols of the three databases are list in the http://cdn.amegroups.cn/static/application/bb0fb2c8a974b80b905d240f0bb48d8d/tcr.2019.10.45-1.pdf. The KEGG analysis showed that these differential genes were enriched in many biological pathways, including cell adhesion molecules, ECM-receptor interaction, leukocyte transendothelial migration and protein digestion, absorption, malaria and PPAR signaling pathway (Figure 3). The result of GO analysis demonstrated that DEGs were associated with many molecular function, such as calcium ion binding, heparin binding, collagen binding, glycosaminoglycan binding, extracellular matrix binding and transforming growth factor beta binding. In cellular component ontology, DEGs were most enriched in extracellular space, extracellular region, proteinaceous extracellular matrix, extracellular matrix, transforming matrix, membrane raft and collagen trimer. These DEGs were further analyzed in the biological, and they are mainly enriched in cell adhesion, extracellular matrix organization, angiogenesis transforming growth factor beta receptor signaling pathway, cellular response to hormone stimulus and response to cAMP (Figure 4).
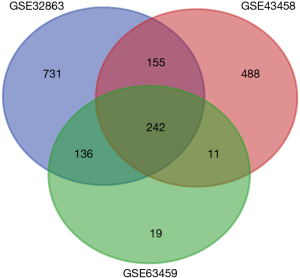
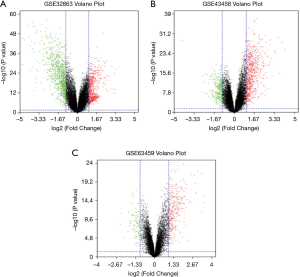
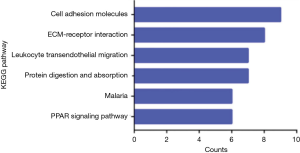
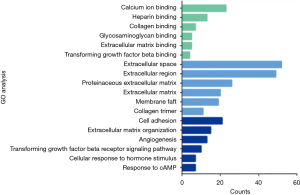
Hub gene analysis
Two hundred forty-two common DEGs of three datasets were uploaded to STRING online database, and then Cytoscape software was used to identify the cluster. Among the 242 differentially expressed genes, ASPM, CCNB2, CDC20, CDC45, CDCA7, MELK, PRC1, TOP2A, UBE2T and KIAA0101 had the closest correlation. Figure 5 showed the PPI work of the ten genes.
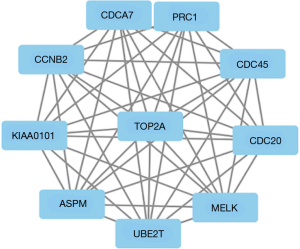
Survival analysis
In order to further evaluate the prognostic value of the ten significantly interacted genes, we conducted Kaplan-Meier curve using the Kaplan-Meier-plotter database. The results showed that ASPM, CCNB2, CDC20, CDC45, MELK, PRC1, TOP2A, UBE2T and KIAA0101 were all significantly associated with the survival of patients with lung adenocarcinomas (hazard ratio =1.76, 1.78, 2.13, 1.67, 1.95, 2.05, 1.86, 1.59, 1.71 respectively) (Figure 6). However, CDCA7 was not associated with poor survival in patients. Furthermore, the ONCOMINE database showed that the expression of ASPM, CCNB2, CDC20, CDC45, MELK, PRC1, TOP2A, UBE2T and KIAA0101 were higher in lung adenocarcinoma tissues, compared with normal counterparts (Figure 7). Although the analysis with online database revealed that the 9-gene signature was differentially expressed in pulmonary malignancy and associated with the prognosis, the above predicted genes were required for further confirmation in clinical specimen derived from lung adenocarcinoma.
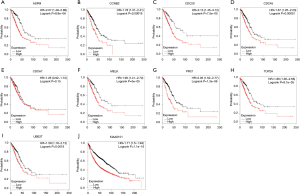
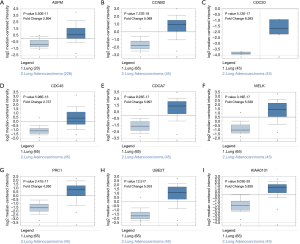
Clinical verification based on qRT-PCR
Subsequently, to verify our bioinformatic analysis, qRT-PCR was used to confirm the expression profile of ASPM, CCNB2, CDC20, CDC45, MELK, PRC1, TOP2A, UBE2T and KIAA0101 in lung adenocarcinoma patients. The result of qRT-RCR showed, as compared to normal tissues, ASPM, CCNB2, CDC20, CDC45, MELK, TOP2A, UBE2T were obviously upregulated in lung adenocarcinoma tissues (Figure 8). However, there was no distinct difference in the expression of PRC1 and KIAA0101 between lung adenocarcinoma and normal tissues. Therefore, these genes may be potential biomarkers for predicting survival rates in patients with lung adenocarcinomas.
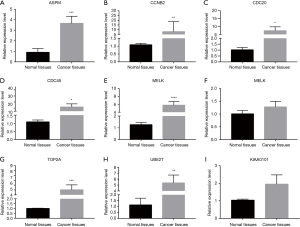
Discussion
Although chemotherapy and targeted therapy have made great benefits on patients, lung cancer remains a major cause for cancer-related deaths. Our study is aimed at identifying reliable biomarkers and therapeutic targets in lung adenocarcinomas to improve diagnosis and survival. In our study, we obtained three datasets from GEO database (GSE32863, GSE43458 and GSE63459), and then, compared gene expression profiles between lung cancer and normal tissues (n=291). Two hundred forty-two common DEGs were obtained in three datasets. The result of GO analysis showed that DEGs were associated with many the molecular functions, the cellular component ontology and the biological process. The KEGG analysis indicated that the DEGs were enriched in many biological pathways. In our PPI network, ten key hub genes (ASPM, CCNB2, CDC20, CDC45, CDCA7, MELK, PRC1, TOP2A, UBE2T and KIAA0101) were identified markedly interacted. Survival analysis also showed these nine hug genes (ASPM, CCNB2, CDC20, CDC45, MELK, PRC1, TOP2A, UBE2T and KIAA0101) were associated with the survival rate of lung adenocarcinoma patients. The result of qRT-PCR displayed that seven-key protein-coding gene (ASPM, CCNB2, CDC20, CDC45, MELK, TOP2A, and UBE2T) were higher expression in lung adenocarcinoma tissues. The small sample size may be one of the major reasons the profile of PRC1 and KIAA0101 were barely noticeable difference. Therefore, ASPM, CCNB2, CDC20, CDC45, MELK, TOP2A, and UBE2T may be responsible for lung adenocarcinomas, and they may be potential molecular targets for the treatment of lung adenocarcinomas. CCNB2, also called cyclin B2, is a member of cell cycle regulator family. CCNB2 interacted with cyclin-dependent protein kinase (CDK) family in eukaryotic cell cycle regulation. CCNB2 is mainly distributed in the Golgi apparatus (9), and is expressed during the all stages of cell division. Moreover, CCNB2 lays a key role in regulating eukaryotic cell cycle in during G2/M transition and mitosis by activating CDK1 kinase (10). The overexpression of CCNB2 has been verified in many human tumors (11), and its overexpression affects the development and prognosis of non-small cell lung cancer.
Cell division cycle associated 7 (CDCA7) is a nuclear protein which contains a zinc finger domain in the C-terminus (12,13), and is a myc-regulated target associated with cancer transformation (14). Overexpression of CDCA7 may promote myc-mediated tumorigenesis, which is often found in human tumors (15). One study has shown that mutations in CDCA7 and HELLS can lead to immunodeficiency-centromeric instability-facial anomalies syndrome (16). CDCA7 participates in hematopoietic stem cell emergence by recruiting the RBPJ factor, especially Notch1 in aorta-gonad-mesonephros cells (17).
Abnormal spindle microtubule assembly (ASPM) is a microtubule-related protein found in the mitotic spindle pole, and is essential for cell division. ASPM plays an important role in the orientation and maintenance of mitotic spindle, especially in nerve stem/progenitor cells (18). Its mutation may promote apoptosis of cells and deplete self-renewal of neural stem cells and progenitor cells, perhaps causing microcephaly (19). ASPM has recently been found as an important modulator of Wnt signaling transduction and cancer stem cells in hormone-refractory prostate cancer (PCA) (20). Its overexpression in glioblastoma cell accelerates the proliferation of the tumor cells (21).
DNA topoisomerase II alpha (TOP2A) is an enzyme that regulates DNA topology during transcription. The overexpression and mutation of TOP2A, and its interaction with other protein partners result in the progression of tumors (22). The expression of TOP2A, which peaks at G2 phase of cell cycle (23), is related to the growth of tumors (24). A study indicated that TOP2A is expressed in more than 70% of oncocytomas, and the expression of TOP2A is related to the size and invasiveness of tumors (25). In pancreatic adenocarcinoma, TOP2A may affect the prognosis and progression of cancer by regulating cell cycle and p53 signaling pathway (26). Since the expression of TOP2A is closely related to the prognosis of hepatocellular carcinoma, TOP2A may be an important biomarker of hepatocellular carcinoma (27).
Cell division cycle 20 (CDC20) is an essential regulatory protein for mitotic cell cycle, and interacts with several other proteins at multiple points during the cell cycle (28). And CDC20 is a key co-factor for promoting E3 ubiquitin ligase of compounds or late bodies (APC/C), so as to regulate the APC/C activity of ubiquitin on specific substrates (29). CDC20, which is significantly overexpressed while the development of malignant tumors, is related to the poor prognosis of various carcinomas. It has been proved that CDC20 may be a potential biomarker of pancreatic ductal adenocarcinoma (PDAC) (30).
Protein regulator of cytokinesis 1 (PRC1) is an important substrate of tubulin (31), and participates in the formation of the spindle through interacting with multiple proteins (32). PRC1 plays an important role in cytoplasmic separation, and is closely related to the occurrence of various tumors (33), especially in breast cancer (34). The TCGA database suggests that the expression of PRC1 is significantly higher in breast cancer tissues than in adjacent tissues.
KIAA0101 is a key biological factor regulating cell proliferation, and belongs to proliferating cell nuclear antigen binding factor family (35). Studies showed that KIAA0101 is overexpressed in cancer, and is involved in regulating DNA replication and repair, cell cycle progression, and cell proliferation (36). Silencing KIAA0101 gene in breast could promote the formation of P35/Sp1 complex and the inhibition of cell cycle (36).
Ubiquitin conjugating enzyme E2 T (UBE2T) is a member of the E2 family of ubiquitin-binding enzymes. UBE2T has attracted more and more attention due to its repairing effect on DNA damage in Fanconi anemia (37). UBE2T regulates the level of mono-ubiquitination of FANCD2 and affects the repairing process of DNA damage within cells (38). This enzyme plays its oncogenic role by inhibiting the expression of BRCA1 gene (39). UBE2T also participates in cell proliferation, and apoptosis, and is up-regulated in osteosarcoma (40), bladder cancer (41), prostate cancer (42), nasopharyngeal cancer (41) and breast cancer (39).
Maternal embryonic leucine zipper kinase (MELK), cell cycle-dependent protein kinase, belongs to the KIN1/PAR-1/MARK family (43). MELK is an indispensable protein kinase of regulating cell cycle, cell proliferation, apoptosis and embryonic development (44). MELK is involved in many protein-protein interactions, and affects many stages of tumorigenesis (45).
Cell division cycle 45 (CDC45) is an important component of DNA helicase complex in eukaryotic DNA replication to initiate DNA synthesis (46). It has been found that increased origin firing by up-regulate CDC45 could ultimately lead to cell apoptosis (47) Mutations in CDC45 may be a major cause for Meier-Gorlin syndrome and skull syndromes (48).
In our study, we identified 242 genes expressed in all the three datasets, and nine key genes (ASPM, CCNB2, CDC20, CDC45, MELK, PRC1, TOP2A, UBE2T and KIAA0101) were associated with survival of lung adenocarcinoma patients by bioinformatics analysis, because there are many other factors (age and comorbidity) that affect the survival rate of lung adenocarcinoma patients. The result of our study may be a new indicators for further research on lung adenocarcinoma. We used qRT-PCR to further verify the relationship between these nine hug genes and lung adenocarcinoma. As shown Figure 8, ASPM, CCNB2, CDC20, CDC45, MELK, TOP2A and UBE2T were more significantly upregulated than PRC1 and KIAA0101 in lung adenocarcinoma patients tissue. Except for the effect of sample size, which cannot cover total races and regions, which may affect gene expression in lung adenocarcinoma. And another limitation is that we did not observes further the protein expression level of these genes via Western Blot or immunohistochemistry to validate the transcriptomic finding. Therefore, we will further study their relationship at the protein level in the further study.
At present, some studies about key genes related to lung adenocarcinoma in the database have been published. For example, Gao et al. identified 11 key genes, among which 10 genes were associated with development of NSCLC patients, from 4 GEO datasets by a bioinformatics method incorporating functional enrichment analysis, PPI network, the module analysis and overall survival analysis (49). Piao et al. found 14 hub genes play an important role in prognosis of NSCLC patients from 3 Gene expression profiles and 1 miRNA expression profile via using data preprocessing, functional and pathway enrichment, the protein-protein interaction network and overall survival analysis. Compared to previous works, we not only screened out the key genes and analyzed the functional enrichment, but verified the relationship between the expression of these genes and lung adenocarcinoma patients at the transcriptional level based on qRT-PCR.
Conclusions
In summary, we screened out seven key genes (ASPM, CCNB2, CDC20, CDC45, MELK, TOP2A and UBE2T) out of 242 DEGs from GSE32863, GSE43458 and GSE63459, which may play significant role during the progression of lung adenocarcinomas, and be potential biomarkers and therapeutic targets for lung adenocarcinomas. Nevertheless, further studies are needed to elucidate the molecular mechanisms underlying the identified genes in the pathogenesis of lung adenocarcinomas.
Acknowledgments
Funding: This work was supported by
Footnote
Conflicts of Interest: All authors have completed the ICMJE uniform disclosure form (available at http://dx.doi.org/10.21037/tcr.2019.10.45). The authors have no conflicts of interest to declare.
Ethical Statement: The authors are accountable for all aspects of the work in ensuring that questions related to the accuracy or integrity of any part of the work are appropriately investigated and resolved. Our study was approved by the Ethics Committee of the Affiliated Hospital of Qingdao University (No.2018015). Each patients was informed of the use of their tissue specimens.
Open Access Statement: This is an Open Access article distributed in accordance with the Creative Commons Attribution-NonCommercial-NoDerivs 4.0 International License (CC BY-NC-ND 4.0), which permits the non-commercial replication and distribution of the article with the strict proviso that no changes or edits are made and the original work is properly cited (including links to both the formal publication through the relevant DOI and the license). See: https://creativecommons.org/licenses/by-nc-nd/4.0/.
References
- Gao C, Zhuang J, He Li, et al. Exploration of methylation-driven genes for monitoring and prognosis of patients with lung adenocarcinoma. Cancer Cell Int 2018;18:194. [Crossref] [PubMed]
- Wang Y, Zhang F, Wang J, et al. lncRNA LOC100132354 promotes angiogenesis through VEGFA/VEGFR2 signaling pathway in lung adenocarcinoma. Cancer Manag Res 2018;10:4257-66. [Crossref] [PubMed]
- Koo HK, Jin SM, Lee CH, et al. Factors associated with recurrence in patients with curatively resected stage I-II lung cancer. Lung Cancer 2011;73:222-9. [Crossref] [PubMed]
- Gridelli C, Rossi A, Maione P. Treatment of non-small-cell lung cancer: state of the art and development of new biologic agents. Oncogene 2003;22:6629-38. [Crossref] [PubMed]
- Barrett T, Edgar R. Mining Microarray Data at NCBI’s Gene Expression Omnibus (GEO)*. Methods Mol Biol 2006;338:175-90. [PubMed]
- Edgar R, Lash A. The Gene Expression Omnibus (GEO): A Gene Expression and Hybridization Repository. 2002 Oct 9 [Updated 2003 Aug 13]. In: McEntyre J, Ostell J, editors. The NCBI Handbook [Internet]. Bethesda (MD): National Center for Biotechnology Information (US); 2002-. Chapter 6.
- Demchak B, Hull T, Reich M, et al. Cytoscape: the network visualization tool for GenomeSpace workflows. F1000research 2014;3:151. [Crossref] [PubMed]
- Dennis G Jr, Sherman BT, Hosack DA, et al. DAVID: Database for Annotation, visualization, and Integrated Discovery. Genome Biol 2003;4:3. [Crossref] [PubMed]
- Draviam VM, Orrechia S, Lowe M, et al. The localization of human cyclins B1 and B2 determines CDK1 substrate specificity and neither enzyme requires MEK to disassemble the Golgi apparatus. J Cell Biol 2001;152:945-58. [Crossref] [PubMed]
- Takashima S, Saito H, Takahashi N, et al. Strong expression of cyclin B2 mRNA correlates with a poor prognosis in patients with non-small cell lung cancer. Tumor Biology 2014;35:4257-65. [Crossref] [PubMed]
- Lei CY, Wang W, Zhu YT, et al. The decrease of cyclin B2 expression inhibits invasion and metastasis of bladder cancer. Urol Oncol 2016;34:237.e1-237.e10. [Crossref] [PubMed]
- Goto Y, Hayashi R, Muramatsu T, et al. JPO1/CDCA7, a novel transcription factor E2F1-induced protein, possesses intrinsic transcriptional regulator activity. Biochimica Et Biophysica Acta 2006;1759:60-8. [Crossref] [PubMed]
- Thijssen PE, Grillo G, Wang J, et al. Mutations in CDCA7 and HELLS cause immunodeficiency-centromeric instability-facial anomalies syndrome. Nat Commun 2015;6:7870. [Crossref] [PubMed]
- Gill RM, Gabor TV, Couzens AL, et al. The MYC-associated protein CDCA7 is phosphorylated by AKT to regulate MYC-dependent apoptosis and transformation. Mol Cell Biol 2013;33:498-513. [Crossref] [PubMed]
- Osthus RC, Karim B, Prescott JE, et al. The Myc target gene JPO1/CDCA7 is frequently overexpressed in human tumors and has limited transforming activity in vivo. Cancer Res 2005;65:5620. [Crossref] [PubMed]
- Thijssen PE, Ito Y, Grillo G, et al. Corrigendum: Mutations in CDCA7 and HELLS cause immunodeficiency-centromeric instability-facial anomalies syndrome. Nat Commun 2016;7:12003. [Crossref] [PubMed]
- Guiu J, Berqen DJ, De Pater E, et al. Identification of Cdca7 as a novel Notch transcriptional target involved in hematopoietic stem cell emergence. J Exp Med 2014;211:2411-23. [Crossref] [PubMed]
- Lang PY, Gershon TR. A New Way to Treat Brain Tumors: Targeting Proteins Coded by Microcephaly Genes?: Brain tumors and microcephaly arise from opposing derangements regulating progenitor growth. Drivers of microcephaly could be attractive brain tumor targets. Bioessays 2018;40:e1700243. [Crossref] [PubMed]
- Létard P, Drunat S, Vial Y, et al. Autosomal recessive primary microcephaly due to ASPM mutations: An update. Human Mutation 2018;39:319-32. [Crossref] [PubMed]
- Pai VC, Hsu CC, Chan TS, et al. ASPM promotes prostate cancer stemness and progression by augmenting Wnt−Dvl-3−β-catenin signaling. Oncogene 2019;38:1340-53. [Crossref] [PubMed]
- Hagemann C, Anacker J, Gerngras S, et al. Expression analysis of the autosomal recessive primary microcephaly genes MCPH1 (microcephalin) and MCPH5 (ASPM, abnormal spindle-like, microcephaly associated) in human malignant gliomas. Oncol Rep 2008;20:301-8. [PubMed]
- Pei YF, Yin XM, Liu XQ. TOP2A induces malignant character of pancreatic cancer through activating beta-catenin signaling pathway. Biochim Biophys Acta Mol Basis Dis 2018;1864:197-207. [Crossref] [PubMed]
- Adachi N, Nomoto M, Kohno K, et al. Cell-cycle regulation of the DNA topoisomerase IIα promoter is mediated by proximal CCAAT boxes: Possible involvement of acetylation. Gene 2000;245:49-57. [Crossref] [PubMed]
- Mano MS, Rosa DD, De Azasmbuja E, et al. The 17q12-q21 amplicon: Her2 and topoisomerase-IIalpha and their importance to the biology of solid tumours. Cancer Treat Rev 2007;33:64-77. [Crossref] [PubMed]
- Baldys-Waligorska A, Wierzbicka L, Sokolowski G, et al. Markers of proliferation and invasiveness in somatotropinomas. Endokrynol Pol 2018;69:182-9. [PubMed]
- Zhou Z, Liu S, Zhang M, et al. Overexpression of Topoisomerase 2-Alpha Confers a Poor Prognosis in Pancreatic Adenocarcinoma Identified by Co-Expression Analysis. Dig Dis Sci 2017;62:2790-800. [Crossref] [PubMed]
- Shen S, Kong J, Qiu Y, et al. Identification of core genes and outcomes in hepatocellular carcinoma by bioinformatics analysis. J Cell Biochem 2019;120:10069-81. [Crossref] [PubMed]
- Zhuang L, Yang Z, Meng Z. Upregulation of BUB1B, CCNB1, CDC7, CDC20, and MCM3 in Tumor Tissues Predicted Worse Overall Survival and Disease-Free Survival in Hepatocellular Carcinoma Patients. Biomed Res Int 2018;2018:7897346. [Crossref] [PubMed]
- Kapanidou M, Curtis NL, Bolanos-Garcia VM. Cdc20: At the Crossroads between Chromosome Segregation and Mitotic Exit. Trends Biochem Sci 2017;42:193-205. [Crossref] [PubMed]
- Chang DZ, Ma Y, Ji B, et al. Increased CDC20 expression is associated with pancreatic ductal adenocarcinoma differentiation and progression. J Hematol Oncol 2012;5:15. [Crossref] [PubMed]
- Jiang W, Jimenez G, Wells NJ, et al. PRC1: A Human Mitotic Spindle–Associated CDK Substrate Protein Required for Cytokinesis. Molecular Cell 1998;2:877-85. [Crossref] [PubMed]
- Liu J, Wang Z, Jiang K, et al. PRC1 cooperates with CLASP1 to organize central spindle plasticity in mitosis. J Biol Chem 2009;284:23059. [Crossref] [PubMed]
- Kurasawa Y, Earnshaw WC, Mochizuki Y, et al. Essential roles of KIF4 and its binding partner PRC1 in organized central spindle midzone formation. EMBO J 2004;23:3237-48. [Crossref] [PubMed]
- Shimo A, Nishidate T, Ohta T, et al. Elevated expression of protein regulator of cytokinesis 1, involved in the growth of breast cancer cells. Cancer Sci 2007;98:174-81. [Crossref] [PubMed]
- De Biasio A, Opakua AI, Mortuza GB, et al. Structure of p15PAF–PCNA complex and implications for clamp sliding during DNA replication and repair. Nat Commun 2015;6:6439. [Crossref] [PubMed]
- Lv W, Su B, Li Y, et al. KIAA0101 inhibition suppresses cell proliferation and cell cycle progression by promoting the interaction between p53 and Sp1 in breast cancer. Biochem Biophys Res Commun 2018;503:600-6. [Crossref] [PubMed]
- Morreale FE, Bortoluzzi A, Chauqule VK, et al. Allosteric Targeting of the Fanconi Anemia Ubiquitin-Conjugating Enzyme Ube2T by Fragment Screening. J Med Chem 2017;60:4093-8. [Crossref] [PubMed]
- Stanley EC, Azzinaro PA, Vierra DA, et al. The Simple ChordateCiona intestinalisHas a Reduced Complement of Genes Associated with Fanconi Anemia. Evolutionary Bioinformatics Online 2016;12:133-48. [Crossref] [PubMed]
- Ueki T, Park JH, Nishidate T, et al. Ubiquitination and downregulation of BRCA1 by ubiquitin-conjugating enzyme E2T overexpression in human breast cancer cells. Cancer Res 2009;69:8752-60. [Crossref] [PubMed]
- Wang Y, Leng H, Chen H, et al. Knockdown of UBE2T Inhibits Osteosarcoma Cell Proliferation, Migration, and Invasion by Suppressing the PI3K/Akt Signaling Pathway. Oncol Res 2016;24:361. [Crossref] [PubMed]
- Hu W, Xiao L, Cao C, et al. UBE2T promotes nasopharyngeal carcinoma cell proliferation, invasion, and metastasis by activating the AKT/GSK3β/β-catenin pathway. Oncotarget 2016;7:15161-72. [PubMed]
- Wen M, Kwon Y, Wang Y, et al. Elevated expression of UBE2T exhibits oncogenic properties in human prostate cancer. Oncotarget 2015;6:25226-39. [Crossref] [PubMed]
- Blot J, Chartrain I, Roqhi C, et al. Cell cycle regulation of pEg3, a new Xenopus protein kinase of the KIN1/PAR-1/MARK family. Dev Biol 2002;241:327-38. [Crossref] [PubMed]
- Li G, Yang M, Zou L, et al. MELK as a potential target to control cell proliferation in triple-negative breast cancer MDA-MB-231 cells. Oncol Lett 2018;15:9934. [PubMed]
- Liu H, Sun Y, Zhang J, et al. MELK and EZH2 Cooperate to Regulate Medulloblastoma Cancer Stem-like Cell Proliferation and Differentiation. Mol Cancer Res 2017;15:1275-86. [Crossref] [PubMed]
- Simon AC, Sannino V, Costanzo V, et al. Structure of human Cdc45 and implications for CMG helicase function. Nat Commun 2016;7:11638. [Crossref] [PubMed]
- Köhler C, Koalick D, Fabricius A, et al. Cdc45 is limiting for replication initiation in humans. Cell Cycle 2016;15:974-85. [Crossref] [PubMed]
- Fenwick AL, Kliszczak M, Cooper F, et al. Mutations in CDC45, Encoding an Essential Component of the Pre-initiation Complex, Cause Meier-Gorlin Syndrome and Craniosynostosis. Am J Hum Genet 2016;99:125-38. [Crossref] [PubMed]
- Guo T, Ma H, Zhou Y. Bioinformatics analysis of microarray data to identify the candidate biomarkers of lung adenocarcinoma. Peer J 2019;7:e7313. [Crossref] [PubMed]