Relationship between histogram metrics of pharmacokinetic parameters of DCE-MRI and histological phenotype in breast cancer
Introduction
Breast cancer is one of the most common cancers that endanger the health of women worldwide. In recent years, the incidence rate of breast cancer has been increased, and the age of onset tends to be younger (1,2). Molecular target therapy for breast cancer is primarily based on the histological phenotypes, such as estrogen receptor (ER), progesterone receptor (PR), human epidermal growth factor receptor 2 (HER2), and MiB1(Ki-67). All these factors exhibit synergistic effects in the progression of breast cancer, and significantly affect the therapeutic efficacy and clinical prognosis of patients (3,4). Therefore, it is of significance to identify these molecular markers preoperatively in molecular target therapy.
High-spatial resolution magnetic resonance imaging (MRI) has been frequently applied in the diagnosis of breast cancer due to higher sensitivity compared with mammography or ultrasound (5). In addition, functional MRI, such as dynamic contrast-enhanced MRI (DCE-MRI), has been routinely incorporated into standard MRI protocols (6,7). Investigators have demonstrated the added value of DCE-MRI in the differential diagnosis, staging, prognosis, and prediction of breast cancer treatment responses (8-10). The reason why DCE-MRI has achieved great success in breast cancer is that it provides a non-invasive and accurate tool to evaluate the blood supply and vascular permeability of tumors. Angiogenesis is a natural and vital process for nutrient supply of tumor growth. However, newly-developed vessels of a tumor are always immature, tortuous, and hyper-permeable (11,12). With DCE-MRI, tumor angiogenesis and permeability can be qualitatively or quantitatively studied in a non-invasive manner (13,14). Recently, significant correlations have been detected between ER, PR, HER2, and parameters derived from DCE-MRI (15,16).
In essence, tumors are markedly heterogeneous in the cell density and vascular structures (17). Tumor heterogeneity is intrinsically a consequence of the imperfection DNA replication: whenever a normal or cancerous cell divides, and a few mutations are acquired, leading to a diverse population of cancer cells (18,19). Furthermore, tumor heterogeneity is highly correlated with tumor staging, therapeutic efficacy and clinical prognosis, etc. (20-22). DCE-MRI can display the heterogeneous features of tumors (23,24).
Histogram analysis has become an emerging quantitative technique to enhance the clinical efficacy and diagnostic accuracy (25,26). Histogram can reflect the changes of the voxel distribution and obtain the mean and percentile values, as well as parameters that can directly represent tumor heterogeneity. Hence, the calculation results of voxel values can be more accurate and comprehensive (27). Breast cancer is biologically heterogeneous, even with the same histological type. Li et al. [2018] have found that histogram parameters detected by DCE-MRI are correlated with breast cancer malignancy (23). In this study, the relationship between pharmacokinetic parameters of DCE-MRI and histological phenotype of breast cancer was investigated by histogram analysis.
Methods
Patients
This retrospective study was approved by the institutional review board and informed consent was waived. From September 2014 to June 2016, a total of 144 clinically or histologically confirmed breast cancer patients (all women, aged 18–79 years) who underwent MRI examination in our hospital were reviewed from the picture archiving and communication system (PACS). Inclusion criteria included: (I) availability of diagnostic quality MR images including DCE-MRI; (II) completion of histopathological examination results of ER/PR/HER2 and Ki-67. Exclusion criteria were: (I) patients underwent biopsy, radiotherapy, chemotherapy, or surgery before MRI examination; (II) patients in menstrual and lactation period; (III) patients with ill-defined margins of the tumors (non-mass like enhancement lesions) on MRI images. Eventually, 44 cases were excluded for the following reasons: 30 cases for non-availability of histological biomarkers, and 14 cases for low image quality. Therefore, the final dataset consisted of 100 patients.
Breast MRI technique
MRI examinations were conducted using a 1.5T (Signa HDxt 1.5T, GE Healthcare, Milwaukee, WI, USA) MRI system equipped with a dedicated 8-channel breast coil. The patients were in a prone position with their bilateral breasts naturally hanged in the two coil holes. After acquiring the scout images in three orthogonal orientations, the axial bilateral fat-suppressed T2-weighted fast-spin echo sequence (repetition time/echo time, 6,600 ms/42 ms; slice thickness, 5 mm; field of view, 330 mm; matrix, 330×330) in the transverse orientation was acquired. The imaging protocol of DCE-MRI consisted of a pre-contrast T1 mapping sequence and a DCE sequence. The two sequences possessed the same spatial resolution and field of view (FOV), and the series of echo sequences were called by the pre-contrast three-dimensional spoiled gradient by using variable flip angle T1 mapping method with flip angles of 5°, 15°, and 25°, respectively. The dynamic scanning (repetition time/echo time, 4.2 ms/2 ms; flip angle 25°; slice thickness 5 mm; field of view with 320 mm; matrix 320×288) was then acquired. After the first three dynamic scans were used as the baseline images, the gadodiamide contrast agent (Omniscan, GE healthcare, Milwaukee, WI) at a dose of 0.1 mmol/kg of body weight was administered at a rate of 3 mL/s intravenously by a power injector, followed by a 20 mL saline flush at the equivalent injection rate to improve bolus coherence. In total, 20 consecutive phases with a time resolution of 22.5 s were acquired for 7.5 min.
MR image post-processing
All imaging results were double-checked by two experienced radiologists and the images were input to the Omni-Kinetics workstation (GE Healthcare, China) for quantitative analysis. To overcome the low time resolution problem, reference region model (RRM) was adopted as the pharmacokinetic model (25). In this model, the generalized kinetic model of DCE-MRI was used to describe the pharmacokinetic behavior of contrast agent (CA) voxel. The equation is as below:
Where CP(t) and CTOI(t) are the concentrations of the CA at time t in plasma and tissue of interest (TOI), respectively. Ktrans,TOI is the transfer constant (min-1) between plasma and the extravascular extracellular space (EES) of the TOI. Kep,TOI is the rate constant (min−1) between EES of the TOI and plasma, equal to Ktrans,TOI divided by Ve,TOI (the extravascular-extracellular fractional volume of TOI).
To eliminate the dependence of CP(t), a second tissue (reference region) as a surrogate for the arterial input function (AIF) in the TOI was introduced. It is described by:
Where CRR(t) is the concentration of the CA in the RR at time t, Ktrans,RR is the transfer constant for the RR, and Kep,RR is the rate constant for the RR. By assuming that CP(t) is the same for both tissues, it’s possible to solve Eq. for CP(t) and thus obtain the permeability parameters of , Kep,TOI and Kep,RR.
In our study, the reference region with an area of 16–21 mm2 in the pectoralis major muscle was selected by an irregularly-shaped ROI to avoid the ribs. For the analysis of tumor, a series of ROIs were manually delineated at the successive slices of each lesion in the phase of the enhanced DCE-MRI. By reference to the T2-weighted image, visible necrosis, vascular, calcification and cystic regions were avoided when delineated. The ROIs were then mapped to the functional MRI images for histogram analysis. The histogram metrics included mean values, the 10th/25th/50th/75th/90th percentiles, energy, entropy, skewness, kurtosis and variance. In the study, the overlapping areas that all three inputs considered tumor were taken to ensure that overestimation of tumor burden did not occur when quantifying values. Furthermore, three radiologists blinded to the clinical information independently delineated the ROIs, and the average of the histogram features was utilized as the final result to reduce individual error.
Energy: it expresses the uniformity of the distribution of image pixel value. The larger the energy value is, the more uniform the distribution of image pixel value is. Entropy: it refers to the uniformity of the distribution of the pixel values in the image histogram. The larger the entropy value is, the more uniform in the distribution of the pixel values in the image is. Mean: it expresses the average pixel mean of an image. Kurtosis: it refers to the approximate state of the image when the pixel value is close to the mean. The smaller the kurtosis is, the more concentrated it is. The equations are as follows:
Prognostic factors
Immunohistochemical staining of the tumor parenchyma without necrosis was performed. The expression levels of ER, PR, Ki-67 and HER-2 were quantitatively observed by microscope (400×). HER-2 was located on the cell membrane, and the remaining markers ER, PR, Ki-67 were distributed in the nucleus. ER or PR positivity was defined as immunostaining in 1% or more of invasive tumor cells based on treatment-oriented classification of breast cancer subgroups. HER2 positivity was defined as +3 score when circumferential membrane staining was completed, and intense staining was observed in >10% of the invasive tumor cells. HER2 positivity with +2 score was subject to fluorescence in situ hybridization analysis. HER2 gene amplification was defined as the ratio of HER2 gene signals to chromosome 17 signals >2.0. Positive expression of Ki-67 was defined as being above a cutoff percentage of 14% of positive staining tumor cells among the total number of assessed malignant cells.
Statistical analysis
All quantitative variables were expressed as mean ± standard deviation (SD). The normal analysis was performed using the Kolmogorov-Smirnov test. Student’s t-test was used for normal distribution data, and the remaining data were analyzed using the Mann-Whitney U test to assess the differences in DCE-MRI parameters and histograms of ER/PR/HER2/Ki-67 expression status. Receiver operating characteristic (ROC) curves were generated to evaluate the diagnostic performance of the histogram metrics in assessing the prognostic factors. The optimal threshold was chosen according to the Youden index. A P value of less than 0.05 was considered as statistically significant. All statistical analyses were performed using Statistical Product and Service Solutions version 19.0 (SPSS, SPSS Inc., Chicago, IL, USA) and MedCalc 15.8 (MedCalc, Mariakerke, Belgium).
Results
Clinical and histopathological findings
Core biopsy specimens were available from all 100 patients. All these lesions were proven as malignant breast cancers by biopsy, and included 75 cases of invasive ductal carcinomas, 19 cases of ductal carcinomas in situ, 4 cases of invasive lobular carcinomas, and 2 cases of invasive micropapillary carcinoma. Among 100 patients, 69 cases were ER+, 57 PR+, 74 HER2+, and 56 Ki-67+ on biopsy specimens (Table 1).
Table 1
Histogram variables | ER | PR | HER2 | Ki-67 | |||||||||||
---|---|---|---|---|---|---|---|---|---|---|---|---|---|---|---|
Positive (n=69) | Negative (n=31) | P | Positive (n=57) | Negative (n=43) | P | Positive (n=74) | Negative (n=26) | P | Positive (n=56) | Negative (n=44) | P | ||||
RKtrans | |||||||||||||||
Mean | 2.453±1.074 | 3.389±2.020 | 0.812 | 3.301±1.342 | 3.092±1.621 | 0.382 | 3.493±1.754 | 2.093±1.274 | 0.100 | 3.493±1.061 | 2.998±1.546 | 0.588 | |||
0.100 | 0.749±0.440 | 0.651±0.539 | 0.726 | 0.806±1.964 | 0.608±1.356 | 0.282 | 0.806±1.058 | 0.499±0.931 | 0.015 | 0.679±1.412 | 0.727±1.731 | 0.912 | |||
0.250 | 1.831±1.143 | 1.637±1.060 | 0.754 | 1.905±1.868 | 1.576±1.653 | 0.345 | 1.606±1.011 | 1.854±1.480 | 0.067 | 1.781±1.885 | 1.606±1.936 | 0.771 | |||
0.500 | 2.492±1.400 | 3.383±1.200 | 0.947 | 3.236±1.831 | 3.236±1.861 | 0.386 | 3.383±1.554 | 2.179±1.135 | 0.072 | 3.139±1.165 | 2.867±1.364 | 0.569 | |||
0.750 | 3.383±1.396 | 4.364±1.484 | 0.935 | 4.141±1.889 | 3.909±1.863 | 0.378 | 3.781±1.033 | 2.969±1.989 | 0.084 | 4.563±1.985 | 3.907±1.104 | 0.598 | |||
0.900 | 3.945±1.571 | 4.667±1.364 | 0.823 | 4.975±1.584 | 4.583±1.571 | 0.349 | 4.908±1.095 | 3.977±1.010 | 0.146 | 5.787±1.892 | 4.564±1.059 | 0.588 | |||
Energy | 0.007±0.008 | 0.001±0.007 | 0.096 | 0.006±0.008 | 0.005±0.007 | 0.679 | 0.001±0.005 | 0.009±0.007 | 0.000 | 0.001±0.006 | 0.006±0.008 | 0.049 | |||
Entropy | 7.320±2.882 | 9.290±2.994 | 0.059 | 7.433±3.025 | 9.216±3.013 | 0.454 | 9.430±2.718 | 7.058±0.751 | 0.000 | 9.253±3.274 | 7.442±2.867 | 0.050 | |||
Kurtosis | 1.492±3.274 | 2.333±1.559 | 0.067 | 1.994±2.609 | 2.286±2.630 | 0.328 | 2.434±1.301 | 0.565±2.187 | 0.002 | 2.315±1.199 | 2.068±3.178 | 0.505 | |||
Skewness | 0.265±0.933 | 0.185±0.999 | 0.293 | 0.184±0.720 | 0.201±1.069 | 0.975 | 0.184±0.877 | 0.450±0.944 | 0.026 | 0.201±0.838 | 0.203±0.998 | 0.331 | |||
Variance | 1.495±8.865 | 2.751±5.883 | 0.644 | 2.550±13.60 | 2.730±5.111 | 0.255 | 2.866±7.736 | 1.820±6.801 | 0.366 | 2.747±6.804 | 1.985±8.684 | 0.607 | |||
Kep,TOI | |||||||||||||||
Mean | 0.238±0.562 | 1.770±0.583 | <0.001 | 0.515±0.607 | 1.825±2.793 | <0.001 | 1.277±2.497 | 0.422±1.265 | 0.009 | 1.544±2.926 | 0.631±1.924 | 0.420 | |||
0.100 | 0.003±0.006 | 0.195±0.625 | <0.001 | 0.006±0.010 | 0.208±0.622 | <0.001 | 0.116±0.618 | 0.008±0.121 | 0.011 | 0.174±0.608 | 0.019±0.186 | 0.071 | |||
0.250 | 0.008±0.017 | 0.560±0.817 | <0.001 | 0.017±0.025 | 0.592±1.754 | <0.001 | 0.245±1.563 | 0.026±0.313 | 0.010 | 0.447±1.423 | 0.067±0.537 | 0.075 | |||
0.500 | 0.017±0.034 | 1.270±0.539 | <0.001 | 0.040±0.571 | 1.406±2.627 | <0.001 | 0.706±2.487 | 0.053±0.631 | 0.003 | 1.104±2.756 | 0.326±1.331 | 0.100 | |||
0.750 | 0.061±0.070 | 2.337±0.057 | <0.001 | 0.265±0.848 | 2.337±4.246 | <0.001 | 1.652±3.327 | 0.299±1.985 | 0.004 | 2.089±4.474 | 0.675±2.621 | 0.196 | |||
0.900 | 0.864±0.711 | 3.629±0.760 | <0.001 | 1.265±1.633 | 3.629±6.092 | <0.001 | 3.210±5.065 | 0.923±2.709 | 0.023 | 3.282±5.658 | 1.724±4.254 | 0.749 | |||
Energy | 0.587±0.546 | 0.003±0.005 | <0.001 | 0.337±0.728 | 0.002±0.005 | <0.001 | 0.005±0.139 | 0.286±0.752 | 0.029 | 0.003±0.037 | 0.034±0.335 | 0.093 | |||
Entropy | 2.238±2.898 | 8.701±2.374 | <0.001 | 3.528±5.051 | 8.776±2.242 | <0.001 | 7.825±4.120 | 3.954±7.544 | 0.036 | 8.259±4.514 | 5.799±5.241 | 0.094 | |||
Kurtosis | 21.23±43.35 | 3.687±3.186 | <0.001 | 10.32±20.88 | 3.874±3.287 | <0.001 | 4.218±6.189 | 9.021±17.37 | 0.003 | 4.218±8.857 | 5.743±7.222 | 0.728 | |||
Skewness | 3.960±3.947 | 1.013±0.984 | <0.001 | 2.815±2.915 | 1.014±0.816 | <0.001 | 1.252±1.895 | 2.736±3.166 | 0.002 | 1.272±1.902 | 1.779±1.998 | 0.389 | |||
Variance | 0.244±3.020 | 1.662±6.858 | 0.002 | 0.397±2.906 | 1.946±7.095 | 0.009 | 1.331±4.416 | 0.321±2.938 | 0.225 | 1.532±4.081 | 1.289±2.918 | 0.652 | |||
Kep,RR | |||||||||||||||
Mean | 0.395±0.170 | 0.266±0.112 | 0.127 | 0.359±0.129 | 0.268±0.126 | 0.319 | 0.279±0.107 | 0.336±0.111 | 0.718 | 0.258±0.188 | 0.347±0.164 | 0.397 | |||
0.100 | 0.059±0.129 | 0.032±0.105 | 0.377 | 0.055±0.129 | 0.032±0.096 | 0.444 | 0.035±0.133 | 0.040±0.086 | 0.869 | 0.014±0.074 | 0.057±0.131 | 0.128 | |||
0.250 | 0.158±0.171 | 0.091±0.125 | 0.265 | 0.145±0.156 | 0.091±0.132 | 0.319 | 0.107±0.156 | 0.107±0.172 | 1.000 | 0.041±0.142 | 0.145±0.154 | 0.137 | |||
0.500 | 0.327±0.166 | 0.218±0.193 | 0.110 | 0.319±0.111 | 0.224±0.183 | 0.293 | 0.243±0.182 | 0.246±0.179 | 0.944 | 0.211±0.119 | 0.289±0.170 | 0.241 | |||
0.750 | 0.542±0.131 | 0.386±0.152 | 0.058 | 0.511±0.190 | 0.386±0.141 | 0.248 | 0.419±0.198 | 0.497±0.103 | 0.753 | 0.351±0.167 | 0.480±0.138 | 0.378 | |||
0.900 | 0.705±0.185 | 0.525±0.124 | 0.060 | 0.696±0.148 | 0.558±0.155 | 0.205 | 0.627±0.191 | 0.700±0.127 | 0.441 | 0.653±0.151 | 0.654±0.191 | 0.797 | |||
Energy | 0.006±0.190 | 0.007±0.326 | 0.544 | 0.006±0.222 | 0.007±0.262 | 0.306 | 0.009±0.222 | 0.002±0.373 | 0.254 | 0.026±0.361 | 0.004±0.182 | 0.091 | |||
Entropy | 7.239±5.833 | 7.074±5.485 | 0.620 | 7.239±5.833 | 7.074±5.249 | 0.358 | 6.917±5.199 | 8.511±6.332 | 0.223 | 5.959±5.595 | 7.992±5.238 | 0.100 | |||
Kurtosis | 3.048±2.058 | 4.595±2.080 | 0.171 | 3.491±2.366 | 3.405±2.096 | 0.797 | 3.175±2.797 | 5.229±2.533 | 0.197 | 4.847±2.602 | 3.232±2.601 | 0.352 | |||
Skewness | 0.876±1.551 | 1.292±1.208 | 0.087 | 0.958±1.904 | 1.133±1.945 | 0.313 | 0.991±1.948 | 1.668±1.741 | 0.400 | 1.666±1.119 | 0.991±1.896 | 0.238 | |||
Variance | 0.093±0.112 | 0.058±0.092 | 0.096 | 0.076±0.112 | 0.058±0.102 | 0.200 | 0.059±0.095 | 0.091±0.095 | 0.258 | 0.083±0.113 | 0.059±0.084 | 0.744 |
Additionally, representative enhanced images and DCE-MRI derived parameters maps were illustrated in Figures 1-3. It was found that 13, 30, and 44 patients were diagnosed with luminal A-, luminal B-, and HER2-enriched phenotypes, respectively. As shown in Figure 1, a high-resolution contrast-enhanced axial T1w image (A) is shown with the corresponding axial parametric map (Figure 1B,C,D) for ER(+) and PR(+) breast cancer, and a high-resolution contrast-enhanced axial T1w image (Figure 1E) is shown with the corresponding axial parametric map Kep,TOI (Figure 1F), RKtrans (Figure 1G), Kep,RR (Figure 1H) for ER(−) and PR(−) breast cancer. A high-resolution contrast-enhanced axial T1w image (Figure 2A) is shown with the corresponding axial parametric map (Figure 2B,C,D) for HER2(+) breast cancer, and a high-resolution contrast-enhanced axial T1w image (Figure 2E) is shown with the corresponding axial parametric map (Figure 2F,G,H) for HER2(−) breast cancer. Accordingly, a high-resolution contrast-enhanced axial T1w image (Figure 3A) is shown with the corresponding axial parametric map (Figure 3B,C,D) for Ki-67(+) breast cancer, and a high-resolution contrast-enhanced axial T1w image (Figure 3E) is shown with the corresponding axial parametric map (Figure 3F,G,H) for Ki-67(−) breast cancer.
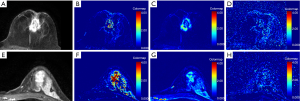
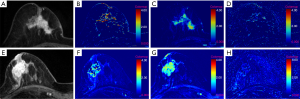
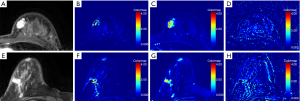
Correlation between DCE-MRI parameters and clinicopathological prognostic factors
The histogram metrics of RKep,TOI, Kep,TOI, and Kep,RR of different ER/PR/HER2/Ki-67 expression groups were summarized in Table 1. All the histogram parameters of Kep,TOI significantly differed between ER/PR/HER2 negative and positive groups (P<0.05), except for the relationship between the variance of Kep,TOI and HER2 (P>0.05). The histogram metrics of the mean value, all the percentiles, entropy and variance of Kep,TOI values were significantly increased in the ER/PR negative tumors (P<0.05), whereas the energy, kurtosis, and skewness of ER/PR negative tumors were significantly higher than those in the ER/PR positive groups (P<0.05). The mean, all the percentiles and entropy of Kep,TOI were significantly decreased in the HER2 negative tumors, whereas the energy, kurtosis and skewness were significantly increased in the HER2 negative tumors (P<0.05).
No significant differences in histogram metrics of RKtrans were found between the ER/PR negative and positive groups (P>0.05). Compared to the HER2 positive groups, the 10th percentile, entropy, and kurtosis were significantly decreased in the HER2 negative groups, whereas the energy and skewness were significantly increased in the HER2 negative group (P<0.05). Only the energy of RKtrans was suggested to be significantly promoted in the Ki-67 negative tumors in comparison with the Ki-67 positive tumors (P=0.049). All the histogram parameters of Kep,KOI showed no significant differences between the ER/PR/HER2/Ki-67 positive and negative groups (P>0.05).
Diagnostic performance of DCE-MRI histogram metrics
The ROC analysis revealed the AUC, specificity sensitivity, and threshold for DCE-MRI measures in the detection of patients with different ER/PR/HER2/Ki-67 expression status (Table 2). As illustrated in Figure 4, the skewness of Kep,KOI showed the highest AUC of 0.977 in the discrimination of ER positive tumors in all the histogram metrics, and the energy of the Kep,KOI exhibited the AUC of 0.879 in comparison with PR positive and negative tumors. Furthermore, as for HER2 and Ki-67, the energy of RKtrans yielded an AUC of 0.854 and 0.615, respectively (Figure 4).
Table 2
Histogram variables | ER | PR | HER-2 | Ki-67 | |||||||||||||||
---|---|---|---|---|---|---|---|---|---|---|---|---|---|---|---|---|---|---|---|
AUC | Sensitivity | Specificity | Threshold | AUC | Specificity | Sensitivity | Threshold | AUC | Specificity | Sensitivity | Threshold | AUC | Specificity (%) | Sensitivity (%) | Threshold | ||||
RKtrans | |||||||||||||||||||
Mean | 0.500 | 0.493 | 0.645 | >3.475 | 0.551 | 0.737 | 0.442 | ≤4.231 | 0.609 | 0.784 | 0.462 | >0.915 | 0.532 | 50.00 | 63.64 | ≤2.303 | |||
10% | 0.522 | 0.275 | 0.903 | >1.926 | 0.563 | 0.561 | 0.581 | ≤0.706 | 0.661 | 0.338 | 1.000 | >1.730 | 0.506 | 75.00 | 15.91 | >0.149 | |||
25% | 0.520 | 0.275 | 0.871 | >3.429 | 0.555 | 0.193 | 0.954 | ≤0.189 | 0.621 | 0.338 | 0.923 | >3.166 | 0.517 | 48.21 | 61.36 | ≤1.356 | |||
50% | 0.504 | 0.522 | 0.581 | >3.236 | 0.551 | 0.772 | 0.372 | ≤4.629 | 0.619 | 0.500 | 0.636 | ≤2.171 | 0.533 | 50.00 | 63.64 | ≤2.179 | |||
75% | 0.505 | 0.435 | 0.452 | ≤3.830 | 0.552 | 0.772 | 0.395 | ≤5.972 | 0.614 | 0.838 | 0.423 | >1.086 | 0.531 | 53.57 | 61.36 | ≤3.830 | |||
90% | 0.514 | 0.493 | 0.387 | ≤5.465 | 0.555 | 0.842 | 0.326 | ≤9.750 | 0.596 | 0.541 | 0.692 | >4.975 | 0.532 | 50.00 | 63.64 | ≤3.945 | |||
Energy | 0.604 | 0.638 | 0.710 | ≤0.003 | 0.524 | 0.597 | 0.581 | ≤0.003 | 0.854 | 0.824 | 0.923 | ≤0.007 | 0.615 | 87.50 | 36.36 | >0.001 | |||
Entropy | 0.618 | 0.667 | 0.710 | >7.776 | 0.544 | 0.632 | 0.581 | >8.136 | 0.795 | 0.811 | 0.846 | >7.320 | 0.614 | 50.00 | 72.73 | ≤7.433 | |||
Kurtosis | 0.615 | 0.681 | 0.645 | >1.994 | 0.557 | 0.790 | 0.372 | >0.789 | 0.702 | 0.811 | 0.692 | >1.306 | 0.539 | 41.07 | 77.27 | ≤1.492 | |||
Skewness | 0.566 | 0.290 | 0.903 | ≤−0.175 | 0.567 | 0.544 | 0.326 | ≤0.299 | 0.647 | 0.635 | 0.692 | ≤0.247 | 0.557 | 78.57 | 36.36 | >−0.132 | |||
Variance | 0.529 | 0.754 | 0.387 | ≤6.119 | 0.556 | 0.807 | 0.419 | ≤6.119 | 0.560 | 0.514 | 0.692 | >2.751 | 0.530 | 41.07 | 72.73 | ≤0.724 | |||
Kep,TOI | |||||||||||||||||||
Mean | 0.883 | 0.710 | 0.936 | >0.749 | 0.774 | 0.667 | 0.861 | >1.273 | 0.673 | 0.689 | 0.654 | >0.587 | 0.547 | 62.50 | 61.36 | ≤0.779 | |||
10% | 0.948 | 0.899 | 1.000 | >0.012 | 0.842 | 0.930 | 0.791 | >0.012 | 0.669 | 0.865 | 0.423 | >0.003 | 0.606 | 57.14 | 77.27 | ≤0.031 | |||
25% | 0.968 | 0.928 | 1.000 | >0.032 | 0.856 | 0.965 | 0.791 | >0.032 | 0.671 | 0.595 | 0.692 | >0.140 | 0.604 | 51.79 | 77.27 | ≤0.067 | |||
50% | 0.989 | 0.986 | 1.000 | >0.105 | 0.847 | 0.930 | 0.744 | >0.201 | 0.699 | 0.581 | 0.769 | >0.477 | 0.596 | 66.07 | 59.09 | ≤0.611 | |||
75% | 0.955 | 0.957 | 0.871 | >0.318 | 0.822 | 0.684 | 0.861 | >1.364 | 0.690 | 0.797 | 0.577 | >0.318 | 0.575 | 66.07 | 59.09 | ≤1.364 | |||
90% | 0.815 | 0.652 | 0.871 | >2.131 | 0.723 | 0.684 | 0.767 | >2.131 | 0.650 | 0.608 | 0.692 | >1.964 | 0.519 | 1.79 | 79.55 | ≤0.013 | |||
Energy | 0.979 | 0.986 | 0.936 | ≤0.143 | 0.879 | 0.842 | 0.861 | ≤0.012 | 0.644 | 0.811 | 0.539 | ≤0.244 | 0.598 | 69.64 | 61.36 | >0.005 | |||
Entropy | 0.972 | 0.971 | 0.936 | >3.954 | 0.855 | 0.825 | 0.837 | >7.133 | 0.639 | 0.770 | 0.539 | >3.954 | 0.598 | 66.07 | 65.91 | ≤7.624 | |||
Kurtosis | 0.954 | 0.884 | 0.936 | ≤7.995 | 0.794 | 0.772 | 0.767 | ≤5.626 | 0.699 | 0.622 | 0.769 | ≤5.324 | 0.520 | 62.50 | 54.55 | >4.386 | |||
Skewness | 0.977 | 0.928 | 1.000 | ≤2.327 | 0.811 | 0.912 | 0.721 | ≤2.327 | 0.704 | 0.622 | 0.731 | ≤1.390 | 0.520 | 44.64 | 75.00 | >2.327 | |||
Variance | 0.696 | 0.710 | 0.613 | >0.397 | 0.652 | 0.597 | 0.721 | >1.331 | 0.580 | 0.662 | 0.539 | >0.397 | 0.526 | 100.00 | 22.73 | >0.029 | |||
Kep,RR | |||||||||||||||||||
Mean | 0.596 | 0.652 | 0.613 | ≤0.359 | 0.558 | 0.614 | 0.558 | ≤0.343 | 0.524 | 0.500 | 0.615 | ≤0.268 | 0.550 | 73.21 | 43.18 | >0.185 | |||
10% | 0.555 | 0.638 | 0.548 | ≤0.055 | 0.545 | 0.772 | 0.395 | ≤0.097 | 0.511 | 0.365 | 0.769 | >0.077 | 0.589 | 53.57 | 70.45 | >0.054 | |||
25% | 0.570 | 0.652 | 0.581 | ≤0.143 | 0.558 | 0.649 | 0.535 | ≤0.134 | 0.500 | 0.784 | 0.308 | >0.002 | 0.587 | 53.57 | 70.45 | >0.134 | |||
50% | 0.600 | 0.667 | 0.581 | ≤0.298 | 0.562 | 0.667 | 0.535 | ≤0.284 | 0.505 | 0.176 | 0.731 | ≤0.005 | 0.569 | 73.21 | 45.45 | >0.128 | |||
75% | 0.619 | 0.652 | 0.645 | ≤0.487 | 0.568 | 0.632 | 0.535 | ≤0.487 | 0.521 | 0.622 | 0.500 | ≤0.519 | 0.552 | 69.64 | 50.00 | >0.341 | |||
90% | 0.618 | 0.580 | 0.742 | ≤0.627 | 0.574 | 0.491 | 0.674 | ≤0.525 | 0.551 | 0.595 | 0.577 | ≤0.668 | 0.515 | 82.14 | 31.82 | >0.422 | |||
Energy | 0.538 | 0.841 | 0.290 | >0.001 | 0.560 | 0.895 | 0.326 | >0.001 | 0.575 | 0.730 | 0.500 | >0.002 | 0.599 | 71.43 | 47.73 | ≤0.087 | |||
Entropy | 0.531 | 0.217 | 0.903 | ≤2.325 | 0.554 | 0.895 | 0.302 | ≤9.363 | 0.581 | 0.865 | 0.346 | ≤9.363 | 0.596 | 71.43 | 50.00 | >5.542 | |||
Kurtosis | 0.586 | 0.333 | 0.936 | >8.494 | 0.515 | 0.316 | 0.837 | >8.494 | 0.585 | 0.392 | 0.846 | ≤2.578 | 0.554 | 64.29 | 50.00 | ≤4.910 | |||
Skewness | 0.607 | 0.435 | 0.774 | >1.821 | 0.559 | 0.421 | 0.698 | >1.821 | 0.556 | 0.635 | 0.539 | ≤1.592 | 0.569 | 69.64 | 50.00 | ≤1.680 | |||
Variance | 0.604 | 0.464 | 0.774 | ≤0.049 | 0.575 | 0.421 | 0.744 | ≤0.045 | 0.575 | 0.487 | 0.731 | ≤0.054 | 0.519 | 64.29 | 50.00 | ≤0.082 |
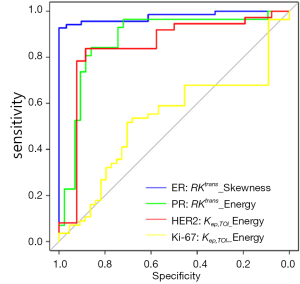
Discussion
Breast cancers consist of four different molecular types Luminal-A, Luminal-B, HER2-overexpressed and triple-negative breast cancers, and different molecular types are more conducive for clinicians to choose the best individualized treatment according to the characteristics of different molecular subtypes (27). To now, imaging techniques such as breast ultrasound, molybdenum target imaging, MRI, DCE-MRI and positron emission tomography (PET) have been widely used in clinical diagnosis (28). Previous study suggested that there was a statistically significant difference between benign and malignant breast lesions in enhancement rate and kinetic AUC for ultrafast MRI imaging and also in initial enhancement rate and signal enhancement ratio (SER) for standard imaging (29). In another study, it was suggest that molybdenum target combined with DCE-MRI in the diagnosis of different molecular types of breast cancer is better than the single imaging screening (30). In the present study, DCE-MRI quantitative analysis was successfully performed using the RRM. The histogram metrics of these parameters can predict the phenotypes of breast cancer with a relatively high accuracy, especially for the differentiation of ER/PR/HER2 positive and negative tumors.
In recent years, several mathematical models, such as Patlak and Tofts models based on the indicator dilution theory (31), have been developed for the analysis of DCE-MRI data to obtain quantitative pharmacokinetic parameters (32). Therefore, knowledge of the time course of the concentration of CA in the blood plasma, AIF, is required. Also, a temporal sampling less than 5 s/image is required to accurately measure the AIF (33). For soft tissues, such as the breast and prostate, the time required for one phase of DCE-MRI image is constantly longer than 10 s. Thus, Tofts and Patlak models may cause significant errors if they are directly applied in this situation. This difficulty has been resolved by using RRM which utilizes the concentration curve in a reference region as an alternative to AIF to eliminate the high time resolution requirement (26). In published studies, the application of RRM has been reported. For instance, to assess the temporal sampling requirements needed for quantitative analysis of DCE-MRI data with a RRM in human breast cancer, DCE-MRI data were acquired on 12 breast cancer patients and parameters were estimated using the native resolution data. The RRM resulted in an error less than 20% in the extracted parameters with temporal sampling as poor as 35.6 seconds (34). In the present study, it was also indicated that RRM is a promising alternative approach for analyzing DCE-MRI data with low temporal resolution.
In our study, a statistically significant increase in Kep,KOI was found with ER/PR negative (−). The breast cancer with ER/PR(−) is highly malignant and exhibits negative responses to hormone therapy with short survival time (35,36). Liu et al. (37) have found that the decrease in ER and PR was related to the rapid growth of tumor, poor differentiation and downregulation of cell synthesis hormone receptor. Compared with ER/PR(+) tumors, the ER/PR(−) tumors grow more rapidly, and the neovascularization is more abundant with more incomplete endothelial cells with increased permeability (37). Kep,KOI represents the rate of permeation of CA from the extravascular extracellular space to the plasma and is correlated with ER/PR status. The results of this study were consistent with previous studies of Koo et al., Chang et al., and Catalano et al. (38-40). However, these studies did not analyze the heterogeneity of the quantitative DCE-MRI parameters. We found that the energy, entropy, kurtosis, skewness, and variance of Kep,KOI significantly differed among various ER/PR subgroups of breast cancer, which might be explained by the extensive heterogeneity of ER/PR(−) tumors.
HER2, an orphan tyrosine kinase receptor and is overexpressed in 15–20% of breast cancers. Overexpression of HER2 can induce vascular endothelial growth factor (VEGF) to promote the tumor neovascularization (41). High HER2 expression indicates rich blood perfusion, high malignancy, and poor response to endocrine therapy (42). Previous finding demonstrated that HER2 amplification was associated with angiogenesis, which could be measured by DCE-MRI (43). The DCE-MRI texture analysis was ever used to predict HER2 2+ status with a high sensitivity, specificity and AUC (44). In the present study, almost all the Kep,KOI metrics and the 10th percentile, energy, entropy, kurtosis, and skewness of RKtrans were found to significantly differ in HER2 positive and negative tumors. The pathological explanation of these results was consistent with ER/PR. More recent studies have corroborated our findings of a correlation with increased Kep in HER2 positive tumors (9,40).
Ki-67 is a proliferation indicator which is mainly correlated with cellular proliferation (45). In our study, almost no histogram metrics of DCE-MRI parameters were found to be significantly different in Ki-67 positive and negative tumors, probably due to the fact that cellular proliferation mainly affects the diffusion behavior rather than impacts the tumor angiogenesis. Actually, inconsistent findings have been reported in the relationship between Ki-67 and DCE-MRI parameters in breast cancers. Li et al. (46) have found that Ktrans is positively correlated with Ki-67 with (r=0.329) in breast cancer without statistical significance (P=0.092). In contrast, Juan et al. [2018] investigated the association between Ki-67 expression and radiomics features of in invasive breast cancer and found that that the lesion area, SD, skewness, kurtosis, homogeneity and inverse differential moment (IDM) were significantly associated with the Ki-67 expression level (47). Given our results, DCE-MRI parameters cannot be used as the indicators of Ki-67 until the underlying mechanism has been unraveled.
There are several limitations in our study. First, as a retrospective study, there is an inevitable choice bias because many breast cancer patients have no pathological results. Second, the relatively small sample size and imbalanced population distribution within certain subtypes also limit the applicability of results. Thus, large prospective, multi-institutional cohort studies with greater statistical power are urgently required to validate the results of the present study. Third, the results between RRM and regular models, such as Tofts model, are not compared in our study.
Conclusions
In conclusion, the tumor heterogeneity is quantitatively analyzed by histogram analysis on quantitative pharmacokinetic breast DCE-MRI parameters. The findings demonstrate that DCE-MRI can improve the performance in the differentiation of histological phenotypes of breast cancer. Qualifying spatial heterogeneity of tumor region is suitable for reflecting the vasculature complexity of different molecular markers.
Acknowledgments
We would like to thank Jialiang Ren from the GE healthcare for his statistical analysis of the study and article revise.
Funding: None.
Footnote
Conflicts of Interest: All authors have completed the ICMJE uniform disclosure form (available at http://dx.doi.org/10.21037/tcr.2019.11.10). The authors have no conflicts of interest to declare.
Ethical Statement: The authors are accountable for all aspects of the work in ensuring that questions related to the accuracy or integrity of any part of the work are appropriately investigated and resolved. The study was conducted in accordance with the Declaration of Helsinki (as revised in 2013). This retrospective study was approved by the institutional review board and informed consent was waived.
Open Access Statement: This is an Open Access article distributed in accordance with the Creative Commons Attribution-NonCommercial-NoDerivs 4.0 International License (CC BY-NC-ND 4.0), which permits the non-commercial replication and distribution of the article with the strict proviso that no changes or edits are made and the original work is properly cited (including links to both the formal publication through the relevant DOI and the license). See: https://creativecommons.org/licenses/by-nc-nd/4.0/.
References
- Schneider AP, Zainer C, Kubat CK, et al. The breast cancer epidemic: 10 facts. Linacre Q 2014;81:244-77. [Crossref] [PubMed]
- Siegel RL, Miller KD, Jemal A. Cancer Statistics, 2017. CA Cancer J Clin 2017;67:7-30. [Crossref] [PubMed]
- Kabel AM. Tumor markers of breast cancer: New prospectives. J Oncol Sci 2017;3:5-11.
- Syed AK, Woodall R, Whisenant JG, et al. Characterizing Trastuzumab-Induced Alterations in Intratumoral Heterogeneity with Quantitative Imaging and Immunohistochemistry in HER2+ Breast Cancer. Neoplasia 2019;21:17-29. [Crossref] [PubMed]
- Riedl CC, Luft N, Bernhart C, et al. Triple-Modality Screening Trial for Familial Breast Cancer Underlines the Importance of Magnetic Resonance Imaging and Questions the Role of Mammography and Ultrasound Regardless of Patient Mutation Status, Age, and Breast Density. J Clin Oncol 2015;33:1128-35. [Crossref] [PubMed]
- Hylton NM. Vascularity assessment of breast lesions with gadolinium-enhanced MR imaging. Magn Reson Imaging Clin N Am 2001;9:321-32. [PubMed]
- Lavini C, Kramer G, Pieters-den Bos I, Hoekstra O, Marcus JT. MRI protocol optimization for quantitative DCE-MRI of the spine. Magn Reson Imaging 2017;44:96-103. [Crossref] [PubMed]
- Heller SL, Moy L, Lavianlivi S, et al. Differentiation of malignant and benign breast lesions using magnetization transfer imaging and dynamic contrast-enhanced MRI. J Magn Reson Imaging 2013;37:138-45. [Crossref] [PubMed]
- Whisenant JG, Sorace AG, Mcintyre JO, et al. Evaluating treatment response using DW-MRI and DCE-MRI in trastuzumab responsive and resistant HER2-overexpressing human breast cancer xenografts. Transl Oncol 2014;7:768-79. [Crossref] [PubMed]
- Elnasr SIS, Rahman RWA, Abdelrahman SF, et al. Role of diffusion weighted imaging and dynamic contrast enhanced MR mammography to detect recurrence in breast cancer patients after surgery. The Egyptian Journal of Radiology and Nuclear Medicine 2016;47:1151-7. [Crossref]
- Zetter BR. Angiogenesis and Tumor Metastasis. Annu Rev Med 1998;49:407-24. [Crossref] [PubMed]
- Abdalla AME, Xiao L, Ullah MW, et al. Current Challenges of Cancer Anti-angiogenic Therapy and the Promise of Nanotherapeutics. Theranostics 2018;8:533-48. [Crossref] [PubMed]
- Wang B, Gao Z, Yan X. Correlative study of angiogenesis and dynamic contrast-enhanced magnetic resonance imaging features of hepatocellular carcinoma. Acta Radiol 2005;46:353-8. [Crossref] [PubMed]
- Unetsubo T, Konouchi H, Yanagi Y, et al. Dynamic contrast-enhanced magnetic resonance imaging for estimating tumor proliferation and microvessel density of oral squamous cell carcinomas. Oral Oncol 2009;45:621-6. [Crossref] [PubMed]
- Trimboli RM, Codari M, Chalouhi KK, et al. Correlation between voxel-wise enhancement parameters on DCE-MRI and pathological prognostic factors in invasive breast cancers. Radiol Med 2018;123:91-7. [Crossref] [PubMed]
- Montemurro F, Martincich L, Sarotto I, et al. Relationship between DCE-MRI morphological and functional features and histopathological characteristics of breast cancer. Eur Radiol 2007;17:1490-7. [Crossref] [PubMed]
- Marusyk A, Polyak K. Tumor heterogeneity: causes and consequences. Biochim Biophys Acta 2010;1805:105-17. [PubMed]
- Vogelstein B, Papadopoulos N, Velculescu VE, et al. Cancer genome landscapes. Science 2013;339:1546-58. [Crossref] [PubMed]
- Allison KH, Sledge GW. Heterogeneity and cancer. Oncology 2014;28:772. [PubMed]
- Ng F, Ganeshan B, Kozarski R, et al. Assessment of Primary Colorectal Cancer Heterogeneity by Using Whole-Tumor Texture Analysis: Contrast-enhanced CT Texture as a Biomarker of 5-year Survival. Radiology 2013;266:177-84. [Crossref] [PubMed]
- Davnall F, Yip C, Ljungqvist G, et al. Assessment of tumor heterogeneity: an emerging imaging tool for clinical practice? Insights Imaging 2012;3:573-89. [Crossref] [PubMed]
- Miles KA. How to use CT texture analysis for prognostication of non-small cell lung cancer. Cancer Imaging 2016;16:10. [Crossref] [PubMed]
- Li Z, Ai T, Hu Y, et al. Application of whole-lesion histogram analysis of pharmacokinetic parameters in dynamic contrast-enhanced MRI of breast lesions with the CAIPIRINHA-Dixon-TWIST-VIBE technique. J Magn Reson Imaging 2018;47:91-6. [Crossref] [PubMed]
- Meyer HJ, Leifels L, Schob S, et al. Histogram analysis parameters identify multiple associations between DWI and DCE MRI in head and neck squamous cell carcinoma. Magn Reson Imaging 2018;45:72-7. [Crossref] [PubMed]
- Nave RD, Foresti S, Pratesi A, et al. Whole-Brain Histogram and Voxel-Based Analyses of Diffusion Tensor Imaging in Patients with Leukoaraiosis: Correlation with Motor and Cognitive Impairment. AJNR Am J Neuroradiol 2007;28:1313-9. [Crossref] [PubMed]
- Cardenasrodriguez J, Howison CM, Pagel MD. A linear algorithm of the reference region model for DCE-MRI is robust and relaxes requirements for temporal resolution. Magn Reson Imaging 2013;31:497-507. [Crossref] [PubMed]
- Rouzier R, Perou CM, Symmans WF, et al. Breast cancer molecular subtypes respond differently to preoperative chemotherapy. Clin Cancer Res 2005;11:5678-85. [Crossref] [PubMed]
- Wang CH, Yin FF, Horton J, et al. Review of treatment assessment using DCE-MRI in breast cancer radiation therapy. World J Methodol 2014;4:46-58. [Crossref] [PubMed]
- Abe H, Mori N, Tsuchiya K, et al. Kinetic Analysis of Benign and Malignant Breast Lesions With Ultrafast Dynamic Contrast-Enhanced MRI: Comparison With Standard Kinetic Assessment. AJR Am J Roentgenol 2016;207:1159-66. [Crossref] [PubMed]
- Hu Y, Zhang Y, Cheng J. Diagnostic value of molybdenum target combined with DCE-MRI in different types of breast cancer. Oncol Lett 2019;18:4056-63. [PubMed]
- Meier P, Zierler KL. On the Theory of the Indicator-Dilution Method for Measurement of Blood Flow and Volume. J Appl Physiol 1954;6:731-44. [Crossref] [PubMed]
- Sourbron SP, Buckley DL. Tracer kinetic modelling in MRI: estimating perfusion and capillary permeability. Phys Med Biol 2012;57:R1-33. [Crossref] [PubMed]
- Henderson E, Rutt BK, Lee T. Temporal sampling requirements for the tracer kinetics modeling of breast disease. Magn Reson Imaging 1998;16:1057-73. [Crossref] [PubMed]
- Planey CR, Welch EB, Xu L, et al. Temporal sampling requirements for reference region modeling of DCE-MRI data in human breast cancer. J Magn Reson Imaging 2009;30:121-34. [Crossref] [PubMed]
- Parise CA, Caggiano V. Breast Cancer Survival Defined by the ER/PR/HER2 Subtypes and a Surrogate Classification according to Tumor Grade and Immunohistochemical Biomarkers. J Cancer Epidemiol 2014;2014:469251. [Crossref] [PubMed]
- Bae MS, Seo M, Kim KG, et al. Quantitative MRI morphology of invasive breast cancer: correlation with immunohistochemical biomarkers and subtypes. Acta Radiol 2015;56:269-75. [Crossref] [PubMed]
- Liu C, Zhang H, Shuang C, et al. Alternations of ER, PR, HER-2/neu, and P53 protein expression in ductal breast carcinomas and clinical implications. Med Oncol 2010;27:747-52. [Crossref] [PubMed]
- Koo HR, Cho N, Song IC, et al. Correlation of perfusion parameters on dynamic contrast-enhanced MRI with prognostic factors and subtypes of breast cancers. J Magn Reson Imaging 2012;36:145-51. [Crossref] [PubMed]
- Catalano OA, Horn GL, Signore A, et al. PET/MR in invasive ductal breast cancer: correlation between imaging markers and histological phenotype. Br J Cancer 2017;116:893-902. [Crossref] [PubMed]
- Chang RF, Chen HH, Chang YC, et al. Quantification of breast tumor heterogeneity for ER status, HER2 status, and TN molecular subtype evaluation on DCE-MRI. Magn Reson Imaging 2016;34:809-19. [Crossref] [PubMed]
- Blackwell KL, Dewhirst MW, Liotcheva V, et al. HER-2 Gene Amplification Correlates with Higher Levels of Angiogenesis and Lower Levels of Hypoxia in Primary Breast Tumors. Clin Cancer Res 2004;10:4083-8. [Crossref] [PubMed]
- Shah D, Osipo C. Cancer stem cells and HER2 positive breast cancer: The story so far. Genes Dis 2016;3:114-23. [Crossref] [PubMed]
- Chou SS, Gombos EC, Chikarmane SA, et al. Computer-aided heterogeneity analysis in breast MR imaging assessment of ductal carcinoma in situ: Correlating histologic grade and receptor status. J Magn Reson Imaging 2017;46:1748-59. [Crossref] [PubMed]
- Jiang Z, Song L, Lu H, et al. The Potential Use of DCE-MRI Texture Analysis to Predict HER2 2+ Status. Front Oncol 2019;9:242. [Crossref] [PubMed]
- Gerdes J, Li L, Schlueter C, Duchrow M, et al. Immunobiochemical and molecular biologic characterization of the cell proliferation-associated nuclear antigen that is defined by monoclonal antibody Ki-67. Am J Pathol 1991;138:867-73. [PubMed]
- Li L, Wang K, Sun X, et al. Parameters of Dynamic Contrast-Enhanced MRI as Imaging Markers for Angiogenesis and Proliferation in Human Breast Cancer. Med Sci Monit 2015;21:376-82. [Crossref] [PubMed]
- Juan MW, Yu J, Peng GX, et al. Correlation between DCE-MRI radiomics features and Ki-67 expression in invasive breast cancer. Oncol Lett 2018;16:5084-90. [PubMed]