Screening key miRNAs and genes in prostate cancer by microarray analysis
Introduction
Prostate cancer (PCa) is the second most frequent cancer and the fifth leading cause of cancer-related death in men (1). It is estimated that there will be 1.7 million new cases annually worldwide by 2030 (2). However, the mechanisms underlying the development and progression of PCa remain to be fully elucidated. Hence, there is an urgent needed for exploring the molecular pathogenesis and developing novel biomarkers and therapeutic strategies for PCa.
PCa carcinogenesis have been reported to be associated with multiple alterations in oncogenes and tumor suppressor genes (3-5). MicroRNAs (miRNAs) are short non-coding RNAs (~22 nucleotides) that regulate up to 30% of human genes post-transcriptionally which represses translation or degrades messenger RNA (mRNA) and play a crucial role in multiple cellular processes such as proliferation, differentiation, apoptosis, migration, and invasion (6,7). Furthermore, accumulated evidence indicated that miRNA play important roles in the processes of PCa (8-10).
In this present study, differentially expressed mRNAs (DEmRNAs) and miRNAs (DEmiRNAs) were identified between PCa and non-tumor tissues by microarray analysis. Functional annotation of DEmRNAs, construction of protein-protein interaction (PPI) network and prediction of upstream transcription factors and downstream target DEmRNAs of DEmiRNAs were conducted to further research functions of key DEmRNAs and DEmiRNAs. Our study may provide new clues for exploring molecular mechanism of PCa and developing PCa-associated diagnostic and therapeutic approaches.
Methods
Patients
Seven patients with PCa and two patients with benign prostatic hyperplasia (BPH) were recruited in this study from The 2nd Hospital of Tianjin medical university between June 2010 and June 2011.The inclusion criteria were as follows: (I) patients with PCa (T2N0M0) were diagnosed based on pathological examination and MRI results; (II) age and sex matched patients with BPH were diagnosed via biopsy. Table 1 displayed the detailed information of all these participants.
Table 1
Index | PCa1 | PCa2 | PCa3 | PCa4 | PCa5 | PCa6 | PCa7 | BPH_1 | BPH_2 |
---|---|---|---|---|---|---|---|---|---|
Gender | Male | Male | Male | Male | Male | Male | Male | Male | Male |
Age (years) | 70 | 62 | 66 | 68 | 72 | 68 | 61 | 60 | 64 |
Pathological type | PRAD | PRAD and DAP | PRAD | PRAD | PRAD | PRAD | PRAD | BPH | BPH |
Gleason score | 6 | 6 | 7 | 5 | 7 | 7 | 8 | NA | NA |
TNM stage | T2N0M0 | T2N0M0 | T2N0M0 | T2N0M0 | T2N0M0 | T3aN0M0 | T3bN0M0 | NA | NA |
PCa, prostate cancer; PRAD, prostate adenocarcinoma; DAP, ductal adenocarcinoma of the prostate.
This study has been approved by the ethics institute of The 2nd Hospital of Tianjin medical university (KY2014K016). All these participants signed the informed consent. This research complied with the principles of the Declaration of Helsinki.
Preparation of RNA
Pca tumor tissue samples (PCa_1-PCa_7) were obtained from the seven patients with PCa (PCa1-PCa7). Five adjacent non-tumor tissue samples (Con_1-Con_5) obtained from five patients with PCa (PCa1-PCa5) and two prostate tissue samples (Con_6-Con_7) obtained from two patients with BPH (BPH1-BPH2) were serve as non-tumor controls. According to the manufacturer’s instructions, total RNA from each sample was isolated and purified by using Trizol (Invitrogen, Gaithersburg, MD, USA) and NucleoSpin® RNA clean-up (MACHEREY-NAGEL, Germany), respectively. The concentration and purity of the total RNA were assayed with a Nanodrop spectrophotometer (Thermo Fisher Scientifc Inc., Waltham, MA, USA). The integrity of the total RNA was confirmed using electrophoresis in agarose gel containing formaldehyde. By using MirVana® miRNA isolation Kit (Ambion, AM1560), miRNA was isolated from each sample.
Gene array profiling
By using Jingxin cRNA linear amplification and labeling kit (CapitalBio) (11), RNA from each sample was labeled with Cy3-dCTP or Cy5-Dctp. Then, labeled products were purified with the PCR NucleoSpin Extract II kit (Machery-Nagel Inc.) and hybridized with the 35k human Genome Array (CapitalBio Inc., Beijing, China). After hybridization, the gene arrays were washed and scanned with CapitalBio LuxScan™10K-A scanners. LuxScan 3.0, BoaoAnalyzer6_step1.pl and BoaoAnalyzer6_step2.pl software products (CapitalBio Inc.) were used to extract and analyze the data. The fluorescence intensity was normalized prior to analysis.
MiRNA array profiling
By using the FlashTagTM Biotin RNA Labeling Kit (Genisphere, LLC, Hatfeld, PA, USA), miRNA was biotin labeled according to the manufacturer’s instructions. Then, biotin labeled miRNAs was hybridized to Affymetrix® GeneChip® miRNA Arrays (Affymetrix, Santa Clara, CA, USA) with the Eukaryotic Hybridization Control Kit (Affymetrix, P/N 900454) in the Hybridization Oven 640 (Affymetrix). Hybridized miRNA arrays were washed and stained using the Hybridization, Wash and Stain Kit (Affymetrix) on the Fluidics Station 450 (Affymetrix) and scanned on a GeneChip® Scanner 3000 (Affymetrix) using Command Console™ 1. Data summarization and quality control were performed by using Affymetrix miRNA QC tool. With the Robust Multichip Average (RMA), background correction and normalization were conducted.
Identification of DEmRNAs and DEmiRNAs
The gene array data and the miRNA array data obtained were normalized by locally weighted scatterplot smoothing (LOWESS) method (12). Then, the t-test method in the LIMMA package of R software (13) was used to identify the DEmRNAs and DEmiRNAs between PCa and non-tumor controls. |log2Foldchange| >1 and P value <0.05 were the thresholds for the DEmRNAs and P value <0.05 was the threshold for DEmiRNAs. Hierarchical clustering analysis of DEmiRNAs and DEmRNAs were conducted by using R package “pheatmap”.
PPI network
To further research the biological functions of DEmRNAs between PCa and non-tumor controls, the PPI network was constructed by using STRING (http://string-db.org/cgi/about.pl?sessionId=DfAjFN3R1SaY&footer_active_subpage=content) and the Cytoscape software. Nodes and edges were used to represent the proteins and interactions between two proteins, respectively. Proteins with a degree of 35 were defined as hub proteins of this PPI network.
Functional annotation
Gene ontology (GO) and Kyoto Encyclopedia of Genes and Genomes (KEGG) pathway enrichment analysis of DEmRNAs between PCa and non-tumor controls were conducted by using online-based software DAVID (https://david.ncifcrf.gov/). Statistical significance was defined as P value <0.05.
Prediction of potential transcription factors of DE-miRNAs
FunRich software, is a tool mainly used for functional enrichment and interaction network analysis of genes and proteins (14). By using FunRich software, the upstream transcription factors of DEmiRNAs were predicted.
DEmiRNA-DEmRNA interaction analysis
Four bioinformatic algorithms (miRDB, TarPmiR, miRTarBase and Targetscan) with miRwalk 3.0 (http://miRwalk.umm.uni-heidelberg.de/) were used to predict the downstream target genes. Then, DEmiRNA-DEmRNA pairs predicted by these four bioinformatic algorithms in which DEmRNA was negatively correlated with DEmiRNAs in this study were retained for our following research. Based on these above DEmiRNA-DEmRNA pairs, the DEmiRNA-DEmRNA interaction network was constructed by the Cytoscape software.
Validation for the expression of DEmRNAs
The Cancer Genome Atlas (TCGA; https://tcga-data.nci.nih.gov/tcga/) is a public-funded project which consists of multi-dimensional data of for multiple cancers at DNA, RNA, and protein levels. The mRNA profile of 492 prostate adenocarcinoma (PRAD) cases and 52 adjacent non-tumor controls was stored in TCGA. Gene Expression Profiling Interactive Analysis (GEPIA, http://gepia.cancer-pku.cn/) is a web-based tool to deliver fast and customizable functionalities based on TCGA and GTEx data (15). By using GEPIA, expression of selected DEmRNAs obtained in this study were validated by TCGA.
Survival analysis
To evaluate the prognostic value of all these obtained DEmRNAs in PCa, survival analysis was performed for all these DEmRNAs by using GEPIA.
Results
DEmRNAs and DEmiRNAs between PCa and non-tumor controls
Total of 91 DEmRNAs (51 up-regulated and 40 down-regulated DEmRNAs) and 62 DEmiRNAs (39 up-regulated and 23 down-regulated DEmiRNAs) between PCa and non-tumor controls were obtained. Top 20 significantly DEmRNAs and DEmiRNAs were displayed in Tables 2 and 3, respectively. Hierarchical cluster analysis of DEmRNAs and DEmiRNAs were shown in Figure 1A and B, respectively.
Table 2
Gene ID | Gene symbol | Log2FC | P value |
---|---|---|---|
ENSG00000113296 | THBS4 | 3.420962 | 0.030016 |
ENSG00000198542 | ITGBL1 | 3.116457 | 0.036118 |
ENSG00000169507 | NP_775783.1 | 3.067172 | 0.049361 |
ENSG00000144355 | DLX1 | 2.998112 | 0.044163 |
ENSG00000105664 | COMP | 2.532081 | 0.047278 |
ENSG00000106819 | ASPN | 2.481949 | 0.025225 |
ENSG00000132821 | C20orf102 | 2.271759 | 0.006654 |
ENSG00000153982 | GDPD1 | 2.24658 | 0.025275 |
ENSG00000109625 | CPZ | 2.028859 | 0.031082 |
ENSG00000147676 | MAL2 | 1.979245 | 0.047306 |
ENSG00000170369 | CST2 | 1.893333 | 0.027599 |
ENSG00000164687 | FABP5 | 1.891178 | 0.044616 |
ENSG00000163110 | PDLIM5 | 1.807621 | 0.02019 |
ENSG00000154917 | RAB6B | 1.771981 | 0.029096 |
ENSG00000187210 | GCNT1 | 1.756058 | 0.009711 |
ENSG00000177707 | PVRL3 | 1.654416 | 0.020207 |
ENSG00000105707 | HPN | 1.653384 | 0.00697 |
ENSG00000183010 | PYCR1 | 1.647304 | 0.011148 |
ENSG00000140479 | PCSK6 | 1.612633 | 0.02487 |
ENSG00000122641 | INHBA | 1.59196 | 0.025026 |
ENSG00000074590 | NUAK1 | −3.677414 | 0.033604 |
ENSG00000112175 | BMP5 | −2.569483 | 0.031053 |
ENSG00000143196 | DPT | −2.213348 | 0.018534 |
ENSG00000172005 | MAL | −2.184924 | 0.034631 |
ENSG00000101938 | CHRDL1 | −2.024137 | 0.044497 |
ENSG00000186847 | KRT14 | −1.955376 | 0.048046 |
ENSG00000049319 | SRD5A2 | −1.933853 | 0.041699 |
ENSG00000096141 | LY6G6D | −1.920051 | 0.048276 |
ENSG00000110244 | APOA4 | −1.89642 | 0.046703 |
ENSG00000204789 | ZNF204 | −1.877634 | 0.016781 |
ENSG00000154188 | ANGPT1 | −1.7652 | 0.036458 |
ENSG00000126258 | FXYD1 | −1.747271 | 0.03719 |
ENSG00000162641 | C1orf62 | −1.721416 | 0.008241 |
ENSG00000151778 | C13orf21 | −1.703242 | 0.041983 |
ENSG00000165646 | SLC18A2 | −1.686371 | 0.047647 |
ENSG00000176049 | JAKMIP2 | −1.471425 | 0.025972 |
ENSG00000137273 | FOXF2 | −1.463853 | 0.035233 |
ENSG00000161055 | SCGB3A1 | −1.463547 | 0.00583 |
ENSG00000173376 | C4orf31 | −1.446799 | 0.046379 |
ENSG00000172201 | ID4 | −1.420409 | 0.020618 |
PCa, prostate cancer; DEmRNAs, differentially expressed mRNAs; Log2FC, log2Fold change.
Table 3
miRNA | log2FC | P value |
---|---|---|
hsa-miR-183 | 1.572899 | 0.047641 |
hsa-miR-182 | 1.553273 | 0.03888 |
hsa-miR-4284 | 1.318899 | 0.04523 |
hsa-miR-663b | 1.283888 | 0.016842 |
hsa-miR-375 | 1.229287 | 0.021521 |
hsa-miR-941 | 1.083061 | 0.018677 |
hsa-miR-3180-3p | 0.926267 | 0.043474 |
hsa-miR-93 | 0.893455 | 0.015524 |
hsa-miR-106b | 0.885544 | 0.047635 |
hsa-miR-1231 | 0.866421 | 0.034641 |
hsa-miR-217 | 0.823899 | 0.04281 |
hsa-miR-1292 | 0.742308 | 0.020546 |
hsa-miR-25 | 0.733483 | 0.015988 |
hsa-miR-484 | 0.692888 | 0.027458 |
hsa-miR-1307 | 0.687092 | 0.023083 |
hsa-miR-339 | 0.653019 | 0.03867 |
hsa-miR-200c | 0.620878 | 0.013658 |
hsa-miR-3175 | 0.610865 | 0.023216 |
hsa-miR-23a | 0.601889 | 0.022816 |
hsa-miR-1972 | 0.550624 | 0.016358 |
hsa-miR-376c | −1.10784 | 0.031947 |
hsa-miR-1 | −1.04235 | 0.046145 |
hsa-miR-30e | −0.82655 | 0.014027 |
hsa-miR-221 | −0.81939 | 0.006079 |
hsa-miR-503 | −0.77411 | 0.025611 |
hsa-miR-99a | −0.75539 | 0.006023 |
hsa-miR-338 | −0.74427 | 0.042402 |
hsa-miR-15a | −0.73052 | 0.041203 |
hsa-miR-29a | −0.7237 | 0.007211 |
hsa-miR-455 | −0.7106 | 0.01735 |
hsa-miR-455 | −0.70809 | 0.033923 |
hsa-miR-221 | −0.70405 | 0.044713 |
hsa-miR-27b | −0.63631 | 0.005391 |
hsa-miR-422a | −0.63095 | 0.021855 |
hsa-miR-379 | −0.60175 | 0.04474 |
hsa-miR-376a | −0.59835 | 0.033791 |
hsa-miR-494 | −0.59444 | 0.011013 |
hsa-let-7g | −0.5942 | 0.043972 |
hsa-miR-337 | −0.58311 | 0.039703 |
hsa-miR-224 | −0.52878 | 0.034879 |
PCa, prostate cancer; DEmiRNAs, differentially expressed miRNAs; Log2FC, log2 fold change.
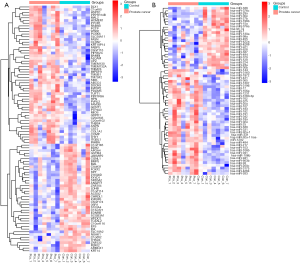
Functional annotation
The most significantly enriched GO terms in PCa were extracellular space (P=5.71E-05), extracellular exosome (P=3.44E-04), cell adhesion (P=4.65E-04), extracellular matrix (P=1.11E-03) and membrane raft (P=1.33E-03). The significantly enriched GO terms including “biological process”, “molecular function”, and “cellular component” were displayed in Figure 2A. TGF-beta signaling pathway (P=5.62E-03) was the only pathway that enriched for DEmRNAs between PCa and non-tumor controls. Four DEmRNAs including inhibin subunit beta A (INHBA) and BMP and activin membrane bound inhibitor (BAMBI), inhibitor of DNA binding 4, HLH protein (ID4) and bone morphogenetic protein 5 (BMP5) were enriched in this pathway (Figure 2B).
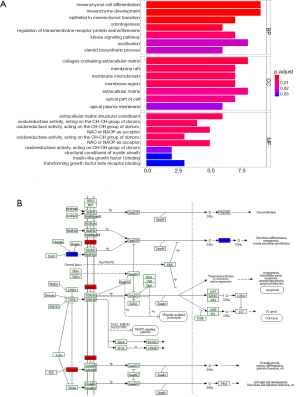
Prediction of upstream transcription factors of DEmiRNAs
After analysis by using FunRich software, upstream transcription factors of DEmiRNAs were predicted. The top 10 transcription factors for DEmiRNAs (Figure 3A) were early growth response 1 (EGR1), POU class 2 homeobox 1 (POU2F1), NK6 homeobox 1 (NKX6-1), Sp1 transcription factor (SP1), NOBOX oogenesis homeobox (NOBOX), AT-rich interaction domain 3A (ARID3A), YY1 transcription factor (YY1), homeobox A9 (HOXA9), myogenic factor 5 (MYF5), POU class 4 homeobox 3 (POU4F3).
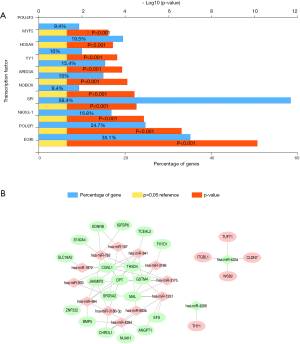
DEmiRNAs-DEmRNAs interaction network
A total of 54 DEmiRNA-DEmRNA pairs consisted of 24 DEmiRNAs (12 up-regulated miRNAs and 2 down-regulated mRNAs) and 14 DEmRNAs (5 up-regulated mRNAs and 19 down-regulated mRNAs) were obtained. The DEmiRNA-DEmRNA interaction network was displayed in Figure 3B, hsa-miR-484, hsa-miR-1231 and hsa-miR-941 were three hub miRNAs.
PPI network
The PPI network of DEmRNAs were consisted of 36 nodes and 30 edges (Figure 4). Two hub proteins including collagen type I alpha 1 chain (COL1A1, degree =7) and Thy-1 cell surface antigen (THY1, degree =5) were identified based on this PPI network (Figure 4).
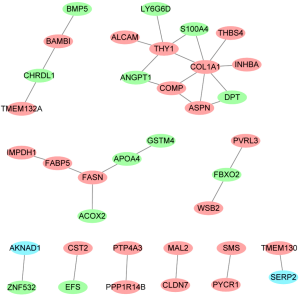
Validation of selected DEmRNAs in TCGA
Based on our microarray analysis, three up-regulated DEmRNAs including thrombospondin-4 precursor (THBS4), PDZ and LIM domain protein 5 (PDLIM5) and ATP binding cassette subfamily C member 4 (ABCC4) and five down-regulated DEmRNAs including bone morphogenetic protein 5 precursor (BMP5), FXYD domain-containing ion transport regulator 1 (FXYD1), inhibitor of DNA binding 4 (ID4), thrombospondin type 1 domain containing 4 (THSD4) and endothelin receptor type B (EDNRB) were selected to perform the expression validation by TCGA. The different expression levels of these eight DEmRNAs between PRAD and adjacent non-tumor controls in TCGA were analyzed and depicted through box-plots (Figure 5) which were consistent with our microarray analysis, generally.
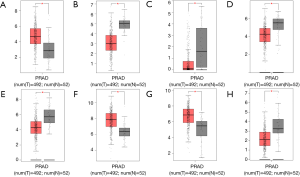
Survival analysis
Up-regulation of ABCC4 is significantly associated with poor survival of patients with PCa (P=0.045, Figure 6A). Decreased EDNRB predicts poor survival in PCa (P=0.027, Figure 6B). Both these two genes have great prognostic value for PCa.
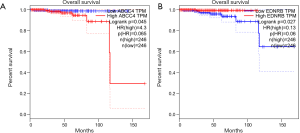
Discussion
PCa is the most lethal urogenital system malignancy in men. Therefore, investigating the molecular mechanisms involved in the progression of PCa is crucial and may reveal novel biomarkers and therapeutic strategies. In this study, DEmRNAs and DEmiRNAs between PCa and non-tumor controls were identified by microarray analysis and their potential roles were further explored by bioinformatics analysis.
THBS4 was the most significantly up-regulated gene between PCa and non-tumor controls in this study. As a member of the extracellular calcium-binding protein family (16), THBS4 was reported to involve with various cancers including colorectal, gastric and prostate cancers (17-19). Furthermore, increased THBS4 has been demonstrated to play a significant role in the proliferation and migration of PCa by regulating the matrix metalloproteinases-9 (MMP-9) and p38 Mitogen-activated protein kinase (MAPK) signaling pathway (4). In this present study, interaction between THBS4 and a potential regulator in metastasis of PCa, COL1A1 (5,8) was found which provided clues for exploring the precise role of THBS4 in PCa.
Two DEmRNAs including ABCC4 and EDNRB with great prognostic value for PCa were identified in this study which might serve as potential biomarkers. Highly methylated EDNRB (20,21) and dysregulated ABCC4 (22,23) have been reported to be regulators of PCa. In addition, EDNRB could be regulated by both hsa-miR-107 and hsa-miR-762. Hsa-miR-107 was a potential regulator of multiple cancers (24-26). Aberrant expressed hsa-miR-107 has been found in meningioma (25) which promoted the proliferation and inhibited apoptosis of colon cancer cells (26). Therefore, hsa-miR-107/hsa-miR-762-EDNRB interactions might play a key role in the processes of PCa.
According to the KEGG enrichment analysis, TGF-beta signaling pathway is the sole pathway that enriched for DEmRNAs of PCa. Previous studies have confirmed that TGF-beta signaling pathway is involved in cancer invasion, metastasis and angiogenesis (27). Furthermore, TGF-beta might involve with the regulation of collagen (28), fbronectin (29), laminin (30) and MMP-9 (31) to affect migratory and invasive capacity. BMP5 and BAMBI were two DEmRNAs that enriched in TGF-beta signaling pathway. Aberrant transcriptional expression levels of several bone morphogenetic proteins (BMPs) such as BMP 2–7 have been observed in PCa compared to normal prostate tissues which highlighted the importance of the BMP family in PCa (32). Based on our PPI network, BMP5, a member of BMP family was found to interact with BAMBI, and BAMBI could make an inhibition on BMP as well as TGF-beta signaling pathway (33). Moreover, BMP5 was a shared downstream target of two cancer-related miRNAs, hsa-miR-4284 (34) and hsa-miR-484 (35,36). We speculated that these two miRNAs play potential roles in PCa by regulating BMP5-BAMBI interaction and TGF-beta signaling pathway.
Based on DEmiRNA-DEmRNA interaction network, THSD4 is a down-regulated DEmRNA regulated by a maximum of DEmiRNAs including hsa-miR-107, hsa-miR-1972, hsa-miR-3175, hsa-miR-3196, hsa-miR-484, hsa-miR-663b, hsa-miR-762 and hsa-miR-941. Although no previous study reports the association between THSD4 and PCa, THSD4 has been indicated to involve in tumorigenesis and development of various cancers including breast cancer, glioblastoma and esophageal carcinoma (37-39). Moreover, has-miR-107, hsa-miR-3175 and hsa-miR-484 have been proved to be potential mediators of PCa (26,40,41) which suggested that THSD4 might be potential regulators of PCa with regulation by these PCa-related miRNAs.
Conclusions
In conclusion, key DEmRNAs and DEmiRNAs between PCa and non-tumor controls were identified in this study. Both ABCC4 and EDNRB have great prognostic value for PCa which were potential prognostic biomarkers. THSD4 serves as a potential regulator of PCa regulated by several cancer-related miRNAs including has-miR-107, hsa-miR-3175 and hsa-miR-484. Hsa-miR-428 and hsa-miR-4284 might play potential roles in PCa by regulating BMP5-BAMBI interaction and TGF-beta signaling pathway. All these findings provided clues for exploring the underlying mechanism of PCa and further experiment was needed to confirm our conclusion.
Acknowledgments
Funding: This study was supported by
Footnote
Conflicts of Interest: All authors have completed the ICMJE uniform disclosure form (available at http://dx.doi.org/10.21037/tcr.2019.12.30). The authors have no conflicts of interest to declare.
Ethical Statement: The authors are accountable for all aspects of the work in ensuring that questions related to the accuracy or integrity of any part of the work are appropriately investigated and resolved. This study has been approved by the ethics institute of The Second Hospital of Tianjin medical university (KY2014K016). All these participants signed the informed consent. This research complied with the principles of the Declaration of Helsinki (as revised in 2013).
Open Access Statement: This is an Open Access article distributed in accordance with the Creative Commons Attribution-NonCommercial-NoDerivs 4.0 International License (CC BY-NC-ND 4.0), which permits the non-commercial replication and distribution of the article with the strict proviso that no changes or edits are made and the original work is properly cited (including links to both the formal publication through the relevant DOI and the license). See: https://creativecommons.org/licenses/by-nc-nd/4.0/.
References
- Bray F, Ferlay J, Soerjomataram I, et al. Global cancer statistics 2018: GLOBOCAN estimates of incidence and mortality worldwide for 36 cancers in 185 countries. CA 2018;68:394-424. [PubMed]
- Center MM, Jemal A, Lortet-Tieulent J, et al. International variation in prostate cancer incidence and mortality rates. Eur Urol 2012;61:1079-92. [Crossref] [PubMed]
- Kido T, Li Y, Tanaka Y, et al. The X-linked tumor suppressor TSPX downregulates cancer-drivers/oncogenes in prostate cancer in a C-terminal acidic domain dependent manner. Oncotarget 2019;10:1491-506. [Crossref] [PubMed]
- Liu J, Cheng G, Yang H, et al. Reciprocal regulation of long noncoding RNAs THBS4003 and THBS4 control migration and invasion in prostate cancer cell lines. Mol Med Rep 2016;14:1451-8. [Crossref] [PubMed]
- Zemskova MY, Song JH, Cen B, et al. Regulation of prostate stromal fibroblasts by the PIM1 protein kinase. Cell Signal 2015;27:135-46. [Crossref] [PubMed]
- Lujambio A, Lowe SW. The microcosmos of cancer. Nature 2012;482:347-55. [Crossref] [PubMed]
- Bartel DP. MicroRNAs: target recognition and regulatory functions. Cell 2009;136:215-33. [Crossref] [PubMed]
- Ivanovic RF, Viana NI, Morais DR, et al. miR-29b enhances prostate cancer cell invasion independently of MMP-2 expression. Cancer Cell Int 2018;18:18. [Crossref] [PubMed]
- Lin HM, Nikolic I, Yang J, et al. MicroRNAs as potential therapeutics to enhance chemosensitivity in advanced prostate cancer. Sci Rep 2018;8:7820. [Crossref] [PubMed]
- Lekchnov EA, Amelina EV, Bryzgunova OE, et al. Searching for the Novel Specific Predictors of Prostate Cancer in Urine: The Analysis of 84 miRNA Expression. Int J Mol Sci 2018; [Crossref] [PubMed]
- Liu M, Pan H, Zhang F, et al. Identification of TNM stage-specific genes in lung adenocarcinoma by genome-wide expression profiling. Oncol Lett 2013;6:763-8. [Crossref] [PubMed]
- Yang YH, Dudoit S, Luu P, et al. Normalization for cDNA microarray data: a robust composite method addressing single and multiple slide systematic variation. Nucleic Acids Res 2002;30:e15. [Crossref] [PubMed]
- Diboun I, Wernisch L, Orengo CA, et al. Microarray analysis after RNA amplification can detect pronounced differences in gene expression using limma. BMC Genomics 2006;7:252. [Crossref] [PubMed]
- Pathan M, Keerthikumar S, Chisanga D, et al. A novel community driven software for functional enrichment analysis of extracellular vesicles data. J Extracell Vesicles 2017;6:1321455. [Crossref] [PubMed]
- Tang Z, Li C, Kang B, et al. GEPIA: a web server for cancer and normal gene expression profiling and interactive analyses. Nucleic Acids Res 2017;45:W98-W102. [Crossref] [PubMed]
- McCart Reed AE, Song S, Kutasovic JR, et al. Thrombospondin-4 expression is activated during the stromal response to invasive breast cancer. Virchows Arch 2013;463:535-45. [Crossref] [PubMed]
- Greco SA, Chia J, Inglis KJ, et al. Thrombospondin-4 is a putative tumour-suppressor gene in colorectal cancer that exhibits age-related methylation. BMC Cancer 2010;10:494. [Crossref] [PubMed]
- Förster S, Gretschel S, Jons T, et al. THBS4, a novel stromal molecule of diffuse-type gastric adenocarcinomas, identified by transcriptome-wide expression profiling. Mod Pathol 2011;24:1390-403. [Crossref] [PubMed]
- Dakhova O, Ozen M, Creighton CJ, et al. Global gene expression analysis of reactive stroma in prostate cancer. Clin Cancer Res 2009;15:3979-89. [Crossref] [PubMed]
- Vasiljević N, Wu K, Brentnall AR, et al. Absolute quantitation of DNA methylation of 28 candidate genes in prostate cancer using pyrosequencing. Dis Markers 2011;30:151-61. [Crossref] [PubMed]
- Phé V, Cussenot O, Roupret M. Methylated genes as potential biomarkers in prostate cancer. BJU Int 2010;105:1364-70. [Crossref] [PubMed]
- Bova GS, Kallio HM, Annala M, et al. Integrated clinical, whole-genome, and transcriptome analysis of multisampled lethal metastatic prostate cancer. Cold Spring Harb Mol Case Stud 2016;2:a000752. [Crossref] [PubMed]
- Demidenko R, Razanauskas D, Daniunaite K, et al. Frequent down-regulation of ABC transporter genes in prostate cancer. BMC Cancer 2015;15:683. [Crossref] [PubMed]
- Wang WX, Kyprianou N, Wang X, et al. Dysregulation of the mitogen granulin in human cancer through the miR-15/107 microRNA gene group. Cancer Res 2010;70:9137-42. [Crossref] [PubMed]
- Katar S, Baran O, Evran S, et al. Expression of miRNA-21, miRNA-107, miRNA-137 and miRNA-29b in meningioma. Clin Neurol Neurosurg 2017;156:66-70. [Crossref] [PubMed]
- Liu F, Liu S, Ai F, et al. miR-107 Promotes Proliferation and Inhibits Apoptosis of Colon Cancer Cells by Targeting Prostate Apoptosis Response-4 (Par4). Oncol Res 2017;25:967-74. [Crossref] [PubMed]
- Zhou Q, Zheng X, Chen L, et al. Smad2/3/4 Pathway Contributes to TGF-beta-Induced MiRNA-181b Expression to Promote Gastric Cancer Metastasis by Targeting Timp3. Cell Physiol Biochem 2016;39:453-66. [Crossref] [PubMed]
- Zimmerman KA, Xing D, Pallero MA, et al. Calreticulin Regulates Neointima Formation and Collagen Deposition following Carotid Artery Ligation. J Vasc Res 2015;52:306-20. [Crossref] [PubMed]
- Ren X, Bo Y, Fan J, et al. Dalbergioidin Ameliorates Doxorubicin-Induced Renal Fibrosis by Suppressing the TGF-beta Signal Pathway. Mediators Inflamm 2016;2016:5147571. [Crossref] [PubMed]
- Tennant BR, Chen J, Shih AZ, et al. Myt3 Mediates Laminin-V/Integrin-beta1-Induced Islet-Cell Migration via Tgfbi. Mol Endocrinol 2015;29:1254-68. [Crossref] [PubMed]
- Zhao J, Cheng Q, Ye P, et al. Atorvastatin improves pathological changes in the aged kidney by upregulating peroxisome proliferator-activated receptor expression and reducing matrix metalloproteinase-9 and transforming growth factor-beta1 levels. Exp Gerontol 2016;74:37-42. [Crossref] [PubMed]
- Bentley H, Hamdy FC, Hart KA, et al. Expression of bone morphogenetic proteins in human prostatic adenocarcinoma and benign prostatic hyperplasia. Br J Cancer 1992;66:1159-63. [Crossref] [PubMed]
- Wordinger RJ, Agarwal R, Talati M, et al. Expression of bone morphogenetic proteins (BMP), BMP receptors, and BMP associated proteins in human trabecular meshwork and optic nerve head cells and tissues. Mol Vis 2002;8:241-50. [PubMed]
- Li Y, Shen Z, Jiang H, et al. MicroRNA4284 promotes gastric cancer tumorigenicity by targeting ten-eleven translocation 1. Mol Med Rep 2018;17:6569-75. [PubMed]
- Zare A, Ahadi A, Larki P, et al. The clinical significance of miR-335, miR-124, miR-218 and miR-484 downregulation in gastric cancer. Mol Biol Rep 2018;45:1587-95. [Crossref] [PubMed]
- Lu X, Lu J. The significance of detection of serum miR-423-5p and miR-484 for diagnosis of colorectal cancer. Clin Lab 2015;61:187-90. [Crossref] [PubMed]
- Su P, Wen S, Zhang Y, et al. Identification of the Key Genes and Pathways in Esophageal Carcinoma. Gastroenterol Res Pract 2016;2016:2968106. [Crossref] [PubMed]
- Cohen H, Ben-Hamo R, Gidoni M, et al. Shift in GATA3 functions, and GATA3 mutations, control progression and clinical presentation in breast cancer. Breast Cancer Res 2014;16:464. [Crossref] [PubMed]
- Ma J, Hou X, Li M, et al. Genome-wide methylation profiling reveals new biomarkers for prognosis prediction of glioblastoma. J Cancer Res Ther 2015;11:C212-5. [Crossref] [PubMed]
- Fomicheva KA, Osip'yants AI, Knyazev EN, et al. Detection of Potential Metastatic Prostate Cancer Circulating Biomarkers by Comparison of miRNA Profiles in DU145 Cells and Culture Medium. Bull Exp Biol Med 2017;162:792-6. [Crossref] [PubMed]
- Guo X, Han T, Hu P, et al. Five microRNAs in serum as potential biomarkers for prostate cancer risk assessment and therapeutic intervention. Int Urol Nephrol 2018;50:2193-200. [Crossref] [PubMed]