Optimization of internal reference genes for qPCR in human pancreatic cancer research
Introduction
Pancreatic cancer (PC) is the third leading cause of cancer mortality followed by lung cancer and colorectal cancer in the United States (1). The incidence and mortality have also been increasing in China over the years (2). PC shows no symptom in the early stage and surgery is the only way to cure it. However, majority of the patients were diagnosed at a distant stage and could not be surgically resected (3). The 5-year overall survival rate remains low at about 8% (4).
Quantitative real-time polymerase chain reaction (qPCR) is a relatively easy and common technique that is widely used to detect gene expression in molecular biology studies. The advantages of qPCR are its high accuracy, enhanced sensitivity, and rapid speed (5). The qPCR experiment is depended on the bond of fluorescent dyes into amplified products exponentially (6). The ideal internal reference gene should be expressed in all kinds of tissues and cells under various experimental conditions and its expression is similar without significant difference (7). There is also a requirement that there be no coamplification of pseudogenes, overlapping genes or other genes to avoid unexpected products of the genome which may result in inaccuracy (8,9). A reliable internal reference gene is of great importance for qPCR because the expression of one gene of interest (GOI) is normalized by selected reference gene (10). In general, some housekeeping genes, such as 18S ribosomal RNA (RS18), glyceraldehyde-3-phosphate dehydrogenase (GAPDH) and actin beta (ACTB) are often used as internal reference genes for relative quantification analysis currently. However, evidences show that the expression of these reference genes may vary in different tissues, cell lines and different conditions (11-13). Our previous microarray study also found that ACTB and GAPDH were up-regulated in PCs comparing with adjacent non-neoplastic tissues (Figure S1) (14). Meanwhile, the effect of qPCR may be hindered by some technical challenges such as sample preparation, storage and use of unspecific primers.
By reviewing the literature on the selection of internal reference genes in qPCR, and combining with the result of our previous microarray analysis, we included 11 most frequently used internal reference genes with non-difference or small difference between PCs and adjacent non-neoplastic tissues, which were RS18, ACTB, beta-2-microglobulin (B2M), GAPDH, ribosomal protein L13a (RPL13A), eukaryotic translation initiation factor 2B subunit alpha (EIF2B1), hydroxymethylbilane synthase (HMBS), importin 8 (IPO8), RNA polymerase II subunit A (POLR2A), ribosomal protein S17 (RPS17) and TATA-box binding protein (TBP). They were supposed to optional internal reference gene for relative quantification analysis. The aim of our study was to analyze the stabilities and look for the optimal internal reference gene of these 11 genes for relative quantification analysis by qPCR in 16 pairs of human PCs and non-neoplastic tissues.
Methods
Human tissue samples
At first 20 pairs of PCs and adjacent non-neoplastic tissues were collected from patients undergoing surgery in the Pancreas Center of the First Affiliated Hospital of Nanjing Medical University between November 2013 and January 2014. No chemotherapy or radiotherapy was performed before tumor removal. The pathology of all tissue specimens was diagnosed by pathologists. All resected specimens were taken immediately during operation and frozen quickly by liquid nitrogen until RNA extraction. Only histologically verified PCs and non-tumor tissues were used in this study. All patients signed informed consent for the use of clinical specimens for research and the Ethics Committees of the First Affiliated Hospital of Nanjing Medical University approved the study.
Internal reference gene selection and primer design
The primers of RS18 and ACTB were supplied by Sangon Biotech (Shanghai, China). The primers of GAPDH, B2M and RPL13A were referenced in the literature (15). The primers of remaining 6 pairs of internal reference genes were designed by using Primer Premier 5 and following principles: (I) specific primers were designed across intron as far as possible. In this way, the size of the fragments amplified from cDNA and genomic DNA was different, and the influence of genomic DNA was eliminated by limiting the amplification of large fragments in genome by appropriate extension time; (II) the amplification products were as short as possible in order not to affect the amplification efficiency; (III) the amplification region was designed at the 3'-end as far as possible for reducing the effect of sample degradation; (IV) other requirements: the length of primers was 18–25 bp; the Tm value was 58–62 °C; the Tm value of forward and reverse primers was no more than 2 °C; the content of GC% was 40–65%; there was no dimer and hairpin as far as possible; the length of products was limited to 50–350 bp. To meet above mentioned requirements, base modification could be performed at 5'-end. After the primers were qualified, they were synthesized by Nanjing Realgene Biotechnology.
RNA extraction and reverse transcription
Total RNA samples were prepared using TRIZOL reagent (Invitrogen, Carlsbad, CA, USA) according to the manufacturer’s instructions, and then stored at −80 °C. The concentration and purity of the isolated RNA were determined by a NanoDrop ND-1000 spectrophotometer (Thermo Fisher Scientific, Rockford, IL, USA). Samples under the A260/A280 ratio of 1.8 (n=4) and corresponding cancer tissues or non-neoplastic tissues were excluded from the 20 pairs of PCs and adjacent non-neoplastic tissues for further analysis. After spectrophotometric quantification, 2 µg of total RNA was used for reverse transcription in a final volume of 40 µL with PrimeScriptTM reverse transcription Master Mix (TAKARA, Tokyo, Japan) according to the manufacturer’s instructions in PTC-200 PCR instrument (Bio-Rad, Hercules, CA, USA). In order to make the input of RNA among different samples as close as possible for reducing experimental error, the concentration of aliquot approximate 3.5 µg RNA of each sample was diluted to 62.5–100 ng/µL (the least concentration of RNA was 62.5 ng/µL because there is a requirement to add 8 µL 5× reverse transcription reagent and the maximum volume of 2 µg RNA is 32 µL). Next, cDNA product from 2 ng RNA was diluted to 100 µL for 100 reactions.
Real-time qPCR and quantification
qPCR was performed using FastStart Universal SYBR Green Master (Roche, Basel, Switzerland) in a StepOne Plus Real-time qPCR System (Thermo Fisher Scientific). Reactions were performed in a volume of 10 µL containing 1 µL diluted cDNA (equivalent to 20 ng RNA), 2× SYBR Green Master. Thermal cycling conditions consisted of an initial denaturation step at 95 °C for 10 min, 40 cycles at 95 °C for 15 s, and 60 °C for 1 min, a melting curve analysis step at 95 °C for 15 s, 60 °C for 1 min, and 95 °C for 15 s. SYBR Green Master was used to detect 11 candidate internal reference genes. All samples for each reference gene were run on the same plate, and different reference genes were run on separate plates. Each qPCR was performed in triplicate. The genes are quantified on the same batch of cDNA to minimize experimental variation according to the manufacturer’s instructions.
Evaluation of stabilities of internal reference genes
Two software programs, including NormFinder (16) and geNorm (15) were used to analyze the stabilities of the 11 candidate internal reference genes. In both programs, ΔCt was calculated [ΔCt = sampleCt − minCt (the lowest average Ct from all tested samples of each internal reference gene)]. Then, 2^-ΔCt was calculated to input into the Microsoft Excel to perform programs according to requirement. NormFinder program ranks the candidate genes by calculating the intra-group variance and inter-group variance of each gene in two groups of PCs and adjacent non-neoplastic samples. GeNorm program also ranks the genes according to the gene expression stability measure M-value which is the average pairwise variation V of a particular gene with all other tested reference genes. The gene with the highest M-value was stepwise excluded. GeNorm expects to find two best genes with the same expression ratio in all samples. If the variation of expression ratios increases, the expression stability of the gene decreases.
Results
The specificity of qPCR amplification
A total of 11 candidate internal reference genes were evaluated in 16 pairs (4 of the 20 pairs were excluded) of PCs and adjacent non-neoplastic tissues in this study. The full gene name, ID number, primer sequences, location of probe indicating whether primers span an intron, amplicon length and melting temperature were shown in Table 1. As expected, the expression of RS18 was abundantly expressed in samples. RPL13A, ACTB, B2M and GAPDH were highly expressed with median Ct between 19 and 23, and the expression levels of the rest genes were low with Ct between 25 and 28 (Figure 1A). The melting curve analysis of each gene all revealed a single peak, which suggested the specificity of qPCR amplification (Figure 1B,C,D,E,F,G,H,I,J,K,L).
Table 1
Gene symbol | Gene name | ID | Forward primer | Reverse primer | Location of probe | Amplicon length (bp) | Tm (°C) |
---|---|---|---|---|---|---|---|
RS18 | 18S ribosomal RNA | No | CAGCCACCCGAGATTGAGCA | TAGTAGCGACGGGCGGTGTG | Not applicable | 253 | 85 |
RPL13A | Ribosomal protein L13a | 23521 | CCTGGAGGAGAAGAGGAAAGAGA | TTGAGGACCTCTGTGTATTTGTCAA | Exon 7–8 boundary | 126 | 77.38 |
ACTB | Actin beta | 60 | TGACGTGGACATCCGCAAAG | CTGGAAGGTGGACAGCGAGG | Exon 5–6 boundary | 205 | 84.25 |
B2M | Beta-2-microglobulin | 567 | TGCTGTCTCCATGTTTGATGTATCT | TCTCTGCTCCCCACCTCTAAGT | Exon 4 | 86 | 79.04 |
GAPDH | Glyceraldehyde-3-phosphate dehydrogenase | 2597 | TGCACCACCAACTGCTTAGC | GGCATGGACTGTGGTCATGAG | Exon 7–8 boundary | 87 | 79.17 |
RPS17 | Ribosomal protein S17 | 6218 | CCCCCGAGGTATCTCCATCA | CCCCCCAGCATTTCCTTAGT | Exon 3–4 boundary | 129 | 77.41 |
POLR2A | RNA polymerase II subunit A | 5430 | TTGACTTGCGTTTCCACCC | AGAATGCGGACCCGATGC | Exon 8–9 boundary | 151 | 81.14 |
EIF2B1 | Eukaryotic translation initiation factor 2B subunit alpha | 1967 | GGAAGGAGTTGTTGAAAACGGAG | GGAAGGGGCAGTGTAGTCG | Exon 7–9 boundary | 247 | 80.09 |
IPO8 | Importin 8 | 10526 | GCAGTAGATGCTGTGGTGGG | GGGTTGTCAAGGTCAAGTGGAG | Exon 23–24 boundary | 305 | 78.01 |
TBP | TATA-box binding protein | 6908 | CGTGCTCACCCACCAACAA | CGCAGGAAATAACTCTGGCTCATA | Exon 6–7 boundary | 52 | 72.63 |
HMBS | Hydroxymethylbilane synthase | 3145 | CCCTGGAGAAGAATGAAGTGG | ATTTTGGGTGAAAGACAACAGC | Exon 6–7 boundary | 135 | 80.38 |
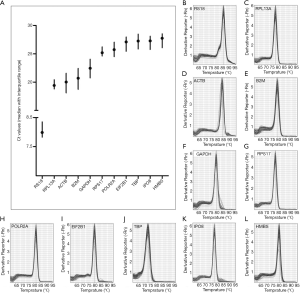
The results of NormFinder algorithm
NormFinder evaluated the stabilities of these 11 genes and calculated the intra-group variance and inter-group variance of each gene in two groups (Figure 2A). To investigate the expression of GOI in PCs and adjacent non-neoplastic tissues, we hoped that the expression of internal reference gene in two groups were consistent as far as possible. So the ideal internal reference gene should meet the conditions that the inter-group variance was as close to zero as possible, and at the same time the intra-group variation was as small as possible. Observed from Figure 2A, TBP, POLR2A, HMBS, IPO8 and EIF2B1 were the top ranked candidate genes.
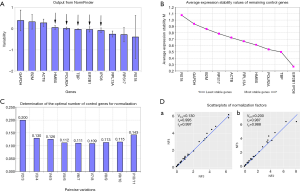
The results of geNorm algorithm
The stability M value of each internal reference gene is equal to the arithmetical mean of the standard deviation of the log2 transformed expression ratio between each gene and all other genes in the entire samples. The lower M value of the gene is, the higher stability is. The result of geNorm showed that the expression of EIF2B1 and IPO8 was the most stable (Figure 2B).
In addition, geNorm also had the function on how to select the optimal combination of internal reference genes. Because the V2/3 value was greater than 0.15 and the V3/4 value was less than 0.15, the normalization factor should contain at least the 3 best reference genes including EIF2B1, IPO8 and the TBP (Figure 2C). Moreover, according to manufacturer’s instructions, 0.15 is not a non-adjustable cut-off value. Through the calculation of correlation analysis, we found that the consistency between NF2 and NF3 was so high and the spearman correlation coefficient (rs) was up to 0.987 (Figure 2D). So we chose a NF2 by geNorm or calculated the geometric mean of two internal reference genes (i.e., EIF2B1 and IPO8) to test the expression of GOI.
We also considered whether the most stable genes—EIF2B1 and IPO8 expressed in two groups were stable in individual PC samples for GOI’s prognostic analysis (Figure 3). The result was that nothing changed.
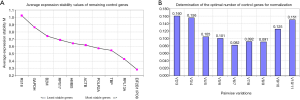
Re-quantification of internal reference genes
Hence, after calculation of a NF2 from the expression levels of EIF2B1 and IPO8 by geNorm, we computed the normalized levels of these 11 candidate internal reference genes again (Figure 4). The normalized GAPDH and ACTB expression levels were obviously up-regulated by 3.29- and 2.23-fold change, meanwhile RPS17 were down-regulated by 0.77-fold change in PCs comparing with corresponding adjacent tissues.
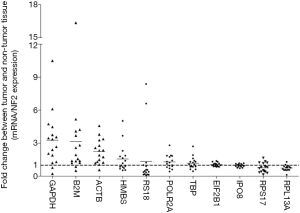
Discussion
The stability of internal reference gene is of great importance for relative quantitative analysis of gene expression. However, many researchers have found that the internal reference gene expression can vary considerably in distinct tissues, cell lines and conditions. Therefore, to get accurate results, it is necessary to evaluate whether the selected reference gene is expressed stably and find more stable internal reference gene for qPCR experiment.
In this study, 11 common internal reference genes on the histological verified 16 pairs of PCs and non-neoplastic tissues were used for qPCR in order to find reliable internal reference gene for relative quantification analysis. In consideration of many factors, such as sample storage method, temperature and laboratory reagent may affect the results, samples preparation, RNA extraction, reverse transcription and real time qPCR were all performed under the same standard in our study. Total RNA was extracted according to the manufacturer’s protocol strictly. The concentration and purity of the isolated RNA were determined by measuring the absorbance values. Only extracted RNA with satisfying concentration and high purity was used in next steps. After the design of primers, all amplification products of these primers were compared with the reference sequence of the human genome by using the Ensembl tool BLAST. Although both the Ensembl database and the NCBI website could do this blast comparison work, the annotation information of the genome was updated more comprehensively in the Ensembl database. So we used it and knew which transcripts were amplified in advance and whether the amplification products included other pseudogene, overlapping gene and so on. The single peak observed from melt curve analysis suggested the specificity of these primers.
NormFinder and geNorm were two of the most commonly used softwares for exploring the stabilities of the candidate internal reference genes. The NormFinder algorithm focus more on inter-group and intra-group variances, making it possible to confirm reference genes in different sample groups, but the program is less sensitive to co-regulation. The geNorm algorithm calculates pairwise variation of each selected gene with all other internal reference genes included in the study and then uses the average of the pairwise variation as the stability M-value for each internal reference gene. For geNorm analysis, 10 samples are the minimum recommended. When estimating expression stability in PCs and adjacent non-neoplastic tissues, we used 32 samples that exceeded minimum sample size, and made sure that the number of samples from each group was same in order to avoid bias. The stabilities of 11 common internal reference genes, including ACTB, B2M, EIF2B1, GAPDH, HMBS, IPO8, POLR2A, RPL13A, RPS17, RS18 and TBP were investigated in this study. According to the results of NormFinder, TBP, POLR2A, HMBS, IPO8 and EIF2B1 were suitable reference genes with high stability. But it didn’t offer the number of genes and quantitative method in the best combination. geNorm could cover the shortage, and it recommended EIF2B1 and IPO8 as the best internal reference genes with high stabilities. There are only limited studies to assess the stabilities of internal reference genes in PCs. Kosinová et al. [2016] found that unstable expression of commonly used reference genes in rat pancreatic islets early after isolation affected results of gene expression studies (17). RPL13A was the most suitable reference gene in mouse acute pancreatitis among 10 reference genes including ACTB and GAPDH (18). Mohelnikova-Duchonova et al. investigated stabilities of 24 reference genes in human pancreas tissue (19). They found both ACTB and GAPDH were considered the least stable genes, and EIF2B1 exhibited the most stable gene while IPO8 showed medium expression stability. Only 10 pairs of samples in their study were tested and fewer than ours. Our single center operated several hundred cases of pancreatic surgery every year, so the source of samples was sufficient. And the collection, preservation and extraction of samples were basically the same in order to reduce bias. EIF2B1 and IPO8 performed excellent stabilities in other cancers, such as non-small cell lung cancer (20) and meningiomas (21). We also considered whether most stable genes expressed in both PCs and adjacent non-neoplastic groups were stable in individual PC samples for GOI’s prognostic analysis. The result showed that the combination of EIF2B1 and IPO8 were also suitable. We also used a NF2 by geNorm as a composite variable to normalized the expression of 11 reference genes. The GAPDH and ACTB expression levels were actually up-regulated within experimental validation. It reflected that some common reference genes were not stable in PCs and should not be utilized without validation.
In conclusion, the use of the combination of EIF2B1 and IPO8 would provide more stable results in differential expression analysis and prognostic analysis of PCs.
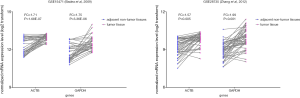
Acknowledgments
Funding: Our work was supported by
Footnote
Conflicts of Interest: All authors have completed the ICMJE uniform disclosure form (available at http://dx.doi.org/10.21037/tcr.2020.02.48). The authors have no conflicts of interest to declare.
Ethical Statement: The authors are accountable for all aspects of the work in ensuring that questions related to the accuracy or integrity of any part of the work are appropriately investigated and resolved. This study was approved by the Ethics Committee of the First Affiliated Hospital with Nanjing Medical University (2017-SR-171). All patients signed informed consent for the use of clinical specimens for research. The study was conducted in accordance with the Declaration of Helsinki (as revised in 2013).
Open Access Statement: This is an Open Access article distributed in accordance with the Creative Commons Attribution-NonCommercial-NoDerivs 4.0 International License (CC BY-NC-ND 4.0), which permits the non-commercial replication and distribution of the article with the strict proviso that no changes or edits are made and the original work is properly cited (including links to both the formal publication through the relevant DOI and the license). See: https://creativecommons.org/licenses/by-nc-nd/4.0/.
References
- Siegel RL, Miller KD, Jemal A. Cancer statistics, 2018. CA Cancer J Clin 2018;68:7-30. [Crossref] [PubMed]
- Chen W, Zheng R, Baade PD, et al. Cancer statistics in China, 2015. CA Cancer J Clin 2016;66:115-32. [Crossref] [PubMed]
- Chen Q, Zhang JJ, Ge WL, et al. YY1 inhibits the migration and invasion of pancreatic ductal adenocarcinoma by downregulating the FER/STAT3/MMP2 signaling pathway. Cancer Lett 2019;463:37-49. [Crossref] [PubMed]
- Goess R, Friess H. A look at the progress of treating pancreatic cancer over the past 20 years. Expert Rev Anticancer Ther 2018;18:295-304. [Crossref] [PubMed]
- Bevitori R, Oliveira MB, Grossi-de-Sa MF, et al. Selection of optimized candidate reference genes for qRT-PCR normalization in rice (Oryza sativa L.) during Magnaporthe oryzae infection and drought. Genet Mol Res 2014;13:9795-805. [Crossref] [PubMed]
- Zhang C, Wang YQ, Jin G, et al. Selection of reference genes for gene expression studies in human bladder cancer using SYBR-Green quantitative polymerase chain reaction. Oncol Lett 2017;14:6001-11. [PubMed]
- Freitag D, Koch A, Lawson McLean A, et al. Validation of Reference Genes for Expression Studies in Human Meningiomas under Different Experimental Settings. Mol Neurobiol 2018;55:5787-97. [Crossref] [PubMed]
- Valadan R, Hedayatizadeh-Omran A, Alhosseini-Abyazani MN, et al. Data supporting the design and evaluation of a universal primer pair for pseudogene-free amplification of HPRT1 in real-time PCR. Data Brief 2015;4:384-9. [Crossref] [PubMed]
- Valadan R, Amjadi O, Tehrani M, et al. Pseudogene-free amplification of HPRT1 in quantitative reverse transcriptase polymerase chain reaction. Anal Biochem 2015;485:46-8. [Crossref] [PubMed]
- Liu J, Wang Q, Sun MY, et al. Selection of Reference Genes for Quantitative Real-Time PCR Normalization in Panax ginseng at Different Stages of Growth and in Different Organs. Plos One 2014;9:e112177. [Crossref] [PubMed]
- Yu Y, Zhang G, Chen Y, et al. Selection of Reference Genes for qPCR Analyses of Gene Expression in Ramie Leaves and Roots across Eleven Abiotic/Biotic Treatments. Sci Rep 2019;9:20004. [Crossref] [PubMed]
- Ali H, Du ZW, Li XY, et al. Identification of suitable reference genes for gene expression studies using quantitative polymerase chain reaction in lung cancer in vitro. Molecular Medicine Reports 2015;11:3767-73. [Crossref] [PubMed]
- He YX, Zhang Y, Yang QW, et al. Selection of suitable reference genes for reverse transcription-quantitative polymerase chain reaction analysis of neuronal cells differentiated from bone mesenchymal stem cells. Molecular Medicine Reports 2015;12:2291-300. [Crossref] [PubMed]
- Shi G, Zhang J, Lu Z, et al. A novel messenger RNA signature as a prognostic biomarker for predicting relapse in pancreatic ductal adenocarcinoma. Oncotarget 2017;8:110849-60. [Crossref] [PubMed]
- Vandesompele J, De Preter K, Pattyn F, et al. Accurate normalization of real-time quantitative RT-PCR data by geometric averaging of multiple internal control genes. Genome Biol 2002;3:RESEARCH0034.
- Andersen CL, Jensen JL, Orntoft TF. Normalization of real-time quantitative reverse transcription-PCR data: A model-based variance estimation approach to identify genes suited for normalization, applied to bladder and colon cancer data sets. Cancer Res 2004;64:5245-50. [Crossref] [PubMed]
- Kosinová L, Cahova M, Fabryova E, et al. Unstable Expression of Commonly Used Reference Genes in Rat Pancreatic Islets Early after Isolation Affects Results of Gene Expression Studies. PLoS One 2016;11:e0152664. [Crossref] [PubMed]
- Yan Z, Gao J, Lv X, et al. Quantitative Evaluation and Selection of Reference Genes for Quantitative RT-PCR in Mouse Acute Pancreatitis. Biomed Res Int 2017;2017:3498537. [Crossref] [PubMed]
- Mohelnikova-Duchonova B, Oliverius M, Honsova E, et al. Evaluation of reference genes and normalization strategy for quantitative real-time PCR in human pancreatic carcinoma. Dis Markers 2012;32:203-10. [Crossref] [PubMed]
- Kristof J, Sakrison K, Jin X, et al. Real-Time Reverse-Transcription Quantitative Polymerase Chain Reaction Assay Is a Feasible Method for the Relative Quantification of Heregulin Expression in Non-Small Cell Lung Cancer Tissue. Biomark Insights 2017;12:1177271917699850. [Crossref] [PubMed]
- Pfister C, Tatabiga MS, Roser F. Selection of suitable reference genes for quantitative real-time polymerase chain reaction in human meningiomas and arachnoidea. BMC Res Notes 2011;4:275. [Crossref] [PubMed]