A prognostic model guides surgical resection in cervical squamous cell carcinoma
Introduction
Cervical squamous cell carcinoma is one of the most common malignancy of the female reproductive system in the world, the third most common female cancer in the world, and the fourth most common cause of cancer-related death. It is reported that there were 569,847 new cases (3.2%) and 569,847 deaths (3.3%) in 2018 alone (1). Women without insurance or regular health care providers have a higher risk of developing the disease. Worldwide, the incidence rate is higher in developing countries with inadequate medical services and lower in developed countries such as North America and West Asia. The squamous cell carcinoma, adenocarcinoma, and squamous cell carcinoma are common in cervical cancer, in which squamous cell carcinoma accounts for more than 80% (2). The vast majority of cervical cancer patients are middle-aged women aged around 40 (3).
Surgery, radiotherapy and chemotherapy are all important methods for the treatment of cervical cancer. Due to limited data, we only studied the effect of surgery on the prognosis of cervical cancer patients. However, the clinical role of hysterectomy in locally advanced cervical cancer (LACC) remains unclear (4,5). Another study showed that single-mode surgery or radiation therapy was the preferred treatment for cervical cancer, but the combination of the two treatments had a higher incidence (6). Therefore, in this study, we need to explore the prognosis of cervical cancer patients with surgery and explore which surgery is more effective.
Methods
Data sources
Data in this study were obtained from the Surveillance, Epidemiology, and End Results (SEER) database. Database including the patient’s age, race, sex, year of diagnosis, the primary lesion, grade, TNM stage, the primary site, tumor size, tumor surgery information coding, tumor-infiltrating degree, treatment plan, the cause of death and marital status, etc., for clinical oncology research, provides a good data to support. Established in 1973 by the National Cancer Institute (NCI), the SEER database includes data from patients who have been treated at the Cancer Accreditation Center Committee, covering approximately 70% of newly diagnosed cancer cases in more than 1,500 hospitals in the United States and 28% of the population in the US. The database has a large sample size and high accuracy, and records the pathogenesis, treatment, pathology, prognosis and other information of millions of patients.
Study population
In this study, the clinical pathology and follow-up data of 94,179 patients with cervical cancer from 1973 to 2015 were obtained by SEER*Stat. The data included patient age, sex, race, age of diagnosis, grade, primary site, derived AJCC stage group, CS tumor size, lymph nodes, age of diagnosis, marital status at diagnosis and so on. We first excluded the first tumor is not a cervical cancer patient data, and then clear the errors, blank, no record, unavailability of the pathological data, and then excluded the subtypes of cervical cancer except squamous cell carcinoma. The 5,620 patients with cervical squamous cell carcinoma screened from 2010 to 2015 were included in this study. We randomly divided 2,248 cases into the training cohort and the remaining 3,372 cases into the verification cohort. The data cleaning process was shown in Figure 1.
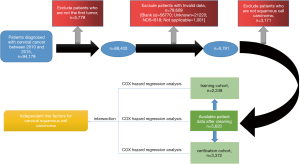
Statistical analysis
We used Excel 2016 version to collate the data, and then used createDataPartition function in the Caret package in R software 3.5.3 version to conduct simple random sampling of the data, and randomly divided the patients into training cohort and verification cohort.
In the first step, we used the Cox proportional hazard regression model to perform univariate and multivariate analysis of the training cohort. If the variable had P>0.05 and no NA in both analyses, the variable was statistically significant with cervical cancer. Then we screen out the statistically significant variables, calculate the hazard ratio (HR) and its confidence interval (95% CI), and use the coxph function of the Survival package to calculate the C-index. At the same time, we obtain the degree of differentiation between the predicted values of the Cox proportional risk regression model and the real values, and then constructed the nomogram. The independent risk factors that can be derived from the nomograms predict the survival rate of cervical cancer patients at 1 year, 3 years, and 5 years. At this point, we use the rcorr.cens function of the Hmisc package to calculate the C-index, and obtain the degree of discrimination between the results of the nomogram prediction and the real results. At the same time, the Bootstrap method is used to carry out 1,600 times of simulated operation training cohort data (b=1,600). Then we draw the calibration, we get the calibration degree between the survival rate of the nomogram prediction and the real result. Next, we calculated the risk score of each patient, and used the risk scoring system to evaluate the accuracy of the model through the ROC curve. AUC indicates the area under the ROC curve (7).
In the second step, we use the same method as the training cohort to analyze the verification cohort. Univariate and multivariate analyses were conducted on the data of the verification cohort with the Cox proportional risk regression model. We screened out the meaningful variables in the verification cohort, and obtained the corresponding HR, 95% CI and Nomogram. Then we use C-index to obtain the degree of discrimination between the Cox proportional hazard regression model of the verification cohort and the real value, and use the Bootstrap method to obtain the calibration degree. Finally, we use the ROC curve to evaluate the predictive model, but the risk scoring system uses the data from the verification cohort.
Third, the Cox proportional risk regression model was again used to conduct univariate and multivariate analyses of the total data before grouping. The significant variables obtained from the multivariate variables were intersected with the significant variables obtained from the training cohort and the verification cohort, and the real variables with statistical significance for cervical cancer were finally determined. Next, according to the final obtained variables, we obtained the overall nomogram, the corresponding discrimination degree and calibration degree between Cox proportional risk regression model and the real value and the AUC used to evaluate the accuracy of the model by ROC curve.
Finally, according to the intersection variables of statistically significant variables from the training cohort, the verification cohort and the overall group, we obtained the total risk score of the total data. Then, we used the Kaplan-Meier method to predict the overall data, calculate the high-risk and low-risk survival rate of cervical cancer, and map the high-risk survival curves of cervical cancer for 1, 3, and 5 years, and the 1-, 3-, and 5-year survival curves of the three independent risk factors.
The C-index is similar to the AUC in the ROC curve and is used to measure the predictive value of the Nomogram. The minimum value is 0.5 and the maximum value is 1.0. The higher the C-index is, the higher the predictive value is. The Bootstrap method is a simulated sampling statistical inference method based on the original data and re-sampling. The sampling concept is the same, and the number of times can be denoted as B, which can be used to analyze the distribution characteristics of a certain statistic. The AUC value can be used as the evaluation standard of the ROC curve. The value range is generally between 0.5 and 1, where the AUC is less than or equal to 0.5 without any prediction ability, 0.71< AUC <0.9 has moderate accuracy prediction ability, AUC >0.9 has high accuracy prediction ability.
Results
Clinical and pathological features
The data included in this study included 5 years of follow-up from 2010 to 2015. During the recording period, 154 patients died from other diseases besides the tumor, 1,023 died from the tumor, and 4,443 survived at the end of the recording period.
In this study, data of 5,620 patients were divided into the training cohort and verification cohort, and their clinical and pathological characteristics were shown in Table 1. Among all patients, cervical cancer was most likely to occur in middle-aged and elderly women aged 30 to 55 (constituent ratio >10%), with a median age of 48.6 (45–49 years old), and the survivors were generally normally distributed. Among the vulnerable races, there were 4,177 cases (74.3%) in Caucasians, which may be due to the fact that most of the races recorded in the SEER database were Caucasians. In the tumor grade, grade II and grade III periods account for most of the tumor, there were 2,689 cases (47.8%) and 2,394 cases (42.6%). Surgery is one of the most effective methods to treat cervical cancer. In the display of the RX Summ-Surg Prim Site, most patients have received different degrees of surgical treatment. The majority of patients (n=1,326, 23.6%) underwent a radical hysterectomy, extended radical hysterectomy, modified radical hysterectomy or extended hysterectomy. There were 279 patients (5.0%) who underwent total hysterectomy without removal of tubes and ovaries, 827 patients (14.7%) who underwent total hysterectomy with removal of tubes and/or ovary, and 16 patients (0.3%) who underwent pelvic clearance. However, there were still 2,375 patients (42.2%) who had no primary site surgery. In RX Summ-Surg Oth Reg/Dis, an investigation or post-mortem autopsy found that virtually none of the patients underwent metastatic surgery, which may be related to the infrequent involvement of cervical cancer in the lymph nodes. From 2010 to 2015, 2,254 cases (40.1%) were diagnosed with tumor size less than or equal to 30 mm. The degree of tumor infiltration was uneven, and the degree of infiltration in the ≥200 and <300 interval accounted for 2,071 cases (36.8%). Lymph nodes of 3,938 patients (70.1%) were not invaded by a tumor, and most tumors did not metastasize at the time of diagnosis (90.1%). Only 1,023 patients died of cervical cancer (18.2%); by the end of the investigation, 4,443 patients (79.0%) survived. Most patients had only one primary tumor (95.4%). Almost all were malignant (99.8%), but most patients had only one malignant tumor (96.2%); the tumor was diagnosed in all adult female age groups, with more women in their 40s diagnosed with cervical cancer. The majority of patients with cervical cancer were married or cohabiting (42.4%).
Table 1
Variable | Before cleaning (n=94,179) | After cleaning (total cohort) (n=5,620) | Grouping | ||||||
---|---|---|---|---|---|---|---|---|---|
All subjects (n=94,179) | All subjects (n=5,620) | Alive (n=4,443) | Dead of this cancer (n=1,023) | Dead of other diseases (n=154) | Training cohort (n=2,248) | Verification cohort (n=3,372) | |||
Age | |||||||||
1–19 | 172 | 0 | 0 | 0 | 0 | 0 | 0 | ||
20–29 | 6,147 | 336 (6.0) | 283 (6.4) | 51 (5.0) | 2 (1.3) | 144 (5.1) | 192 (5.7) | ||
30–34 | 8,809 | 563 (10.0) | 468 (10.5) | 92 (9.0) | 3 (1.9) | 214 (9.5) | 349 (10.3) | ||
35–39 | 10,958 | 652 (11.6) | 578 (13.0) | 73 (7.1) | 1 (0.6) | 260 (11.6) | 392 (11.6) | ||
40–44 | 11,866 | 750 (13.3) | 625 (14.1) | 117 (11.4) | 8 (5.2) | 291 (12.9) | 459 (13.6) | ||
45–49 | 10,914 | 716 (12.7) | 561 (12.6) | 141 (13.8) | 14 (9.1) | 287 (12.8) | 429 (12.7) | ||
50–54 | 9,598 | 687 (12.2) | 530 (11.9) | 139 (13.6) | 18 (11.7) | 280 (12.4) | 407 (12.0) | ||
55–59 | 8,343 | 553 (9.8) | 430 (9.7) | 106 (10.4) | 17 (11.0) | 237 (10.5) | 316 (9.4) | ||
60–64 | 7,316 | 481 (8.6) | 367 (8.3) | 95 (9.3) | 19 (12.3) | 194 (8.6) | 287 (8.5) | ||
65–74 | 11,069 | 559 (9.9) | 407 (9.2) | 117 (11.4) | 35 (22.7) | 223 (9.9) | 336 (10.0) | ||
≥75 | 8,987 | 323 (5.7) | 194 (4.4) | 92 (9.0) | 37 (24.0) | 118 (5.2) | 205 (6.0) | ||
Race | |||||||||
Black | 13,556 | 818 (14.6) | 591 (13.3) | 193 (18.9) | 34 (22.1) | 328 (14.6) | 490 (14.5) | ||
White | 70,994 | 4,177 (74.3) | 3,342 (75.2) | 725 (70.9) | 110 (71.4) | 1,670 (74.3) | 2,507 (74.3) | ||
Other | 8,811 | 625 (11.1) | 510 (11.5) | 105 (10.3) | 10 (6.5) | 250 (11.1) | 375 (11.1) | ||
Unknown | 818 | 0 | 0 | 0 | 0 | 0 | 0 | ||
Grade | |||||||||
Grade I | 7,816 | 462 (8.2) | 418 (9.4) | 35 (3.4) | 9 (5.8) | 199 (8.8) | 263 (7.8) | ||
Grade II | 23,526 | 2,689 (47.8) | 2,194 (49.4) | 422 (41.2) | 73 (47.4) | 1059 (47.1) | 1630 (48.3) | ||
Grade III | 24,415 | 2,394 (42.6) | 1,774 (39.9) | 551 (53.9) | 69 (44.8) | 956 (42.5) | 1438 (42.6) | ||
Grade IV | 2,361 | 75 (1.3) | 57 (1.3) | 15 (1.5) | 3 (1.9) | 34 (1.5) | 41 (1.2) | ||
Unknown | 36,061 | 0 | 0 | ||||||
Stage | |||||||||
IA | 3,147 | 737 (13.1) | 719 (16.2) | 8 (0.8) | 10 (6.5) | 288 (12.8) | 449 (13.3) | ||
IANOS | 425 | 0 | 0 | 0 | 0 | 0 | 0 | ||
INOS | 410 | 0 | 0 | 0 | 0 | 0 | 0 | ||
IB | 4,556 | 1,736 (30.9) | 1,600 (36.0) | 106 (10.4) | 30 (19.5) | 663 (29.5) | 1073 (31.8) | ||
IBNOS | 308 | 0 | 0 | 0 | 0 | 0 | 0 | ||
IIA | 652 | 262 (4.7) | 206 (4.6) | 45 (4.4) | 11 (7.1) | 111 (4.9) | 151 (4.5) | ||
IIANOS | 149 | 0 | 0 | 0 | 0 | 0 | 0 | ||
IIB | 1,716 | 598 (10.6) | 485 (10.9) | 87 (8.5) | 26 (16.9) | 253 (11.2) | 345 (10.2) | ||
IINOS | 16 | 0 | 0 | 0 | 0 | 0 | 0 | ||
III | 4,194 | 1,610 (28.6) | 1,131 (25.4) | 424 (41.4) | 55 (35.7) | 661 (29.4) | 949 (28.1) | ||
IIINOS | 93 | 0 | 0 | 0 | 0 | 0 | 0 | ||
IV | 3,138 | 677 (12.0) | 302 (6.8) | 353 (34.5) | 22 (14.3) | 272 (12.1) | 405 (12.0) | ||
NA | 158 | 0 | 0 | 0 | 0 | 0 | 0 | ||
UNK stage | 1,371 | 0 | 0 | 0 | 0 | 0 | 0 | ||
Blank (s) | 73,836 | 0 | 0 | 0 | 0 | 0 | 0 | ||
Stag_T | |||||||||
T0 | 14 | 0 | 0 | 0 | 0 | 0 | 0 | ||
T1a | 3,267 | 762 (13.6) | 741 (16.7) | 11 (1.1) | 10 (6.5) | 297 (13.2) | 465 (13.8) | ||
T1aNOS | 492 | 0 | 0 | 0 | 0 | 0 | 0 | ||
T1b | 5,760 | 2,254 (40.1) | 2,011 (45.3) | 204 (19.9) | 39 (25.3) | 890 (39.6) | 1,364 (40.4) | ||
T1bNOS | 401 | 0 | 0 | 0 | 0 | 0 | 0 | ||
T1NOS | 609 | 0 | 0 | 0 | 0 | 0 | 0 | ||
T2a | 1,104 | 465 (8.3) | 355 (8.0) | 88 (8.6) | 22 (14.3) | 192 (8.5) | 273 (8.1) | ||
T2aNOS | 309 | 0 | 0 | 0 | 0 | 0 | 0 | ||
T2b | 2,846 | 1,014 (18.0) | 758 (17.1) | 219 (21.4) | 37 (24.0) | 420 (18.7) | 594 (17.6) | ||
T2NOS | 29 | 0 | 0 | 0 | 0 | 0 | 0 | ||
T3a | 689 | 191 (3.4) | 94 (2.1) | 90 (8.8) | 7 (4.5) | 78 (3.5) | 113 (3.4) | ||
T3b | 2,187 | 730 (13.0) | 390 (8.8) | 309 (30.2) | 31 (20.1) | 281 (12.5) | 449 (13.3) | ||
T3NOS | 225 | 0 | 0 | 0 | 0 | 0 | 0 | ||
T4 | 752 | 204 (3.6) | 94 (2.1) | 102 (10.0) | 8 (5.2) | 90 (4.0) | 114 (3.4) | ||
T4b | 1 | 0 | 0 | 0 | 0 | 0 | 0 | ||
TX | 1,500 | 0 | 0 | 0 | 0 | 0 | 0 | ||
NA | 158 | 0 | 0 | 0 | 0 | 0 | 0 | ||
Blank (s) | 73,836 | 0 | 0 | 0 | 0 | 0 | 0 | ||
Stag_N | |||||||||
N0 | 13,700 | 3,938 (70.1) | 3,338 (75.1) | 489 (47.8) | 111 (72.1) | 1,564 (69.6) | 2,374 (70.4) | ||
N1 | 4,894 | 1,682 (29.9) | 1,105 (24.9) | 534 (52.2) | 43 (27.9) | 684 (30.4) | 998 (29.6) | ||
NA | 158 | 0 | 0 | 0 | 0 | 0 | 0 | ||
NX | 1,591 | 0 | 0 | 0 | 0 | 0 | 0 | ||
Blank (s) | 73,836 | 0 | 0 | 0 | 0 | 0 | 0 | ||
Stag_M | |||||||||
M0 | 17,452 | 5,072 (90.2) | 4,208 (94.7) | 727 (71.1) | 137 (89.0) | 2,034 (90.5) | 3,038 (90.1) | ||
M1 | 2,733 | 548 (9.8) | 235 (5.3) | 296 (28.9) | 17 (11.0) | 214 (9.5) | 334 (9.1) | ||
NA | 158 | 0 | 0 | 0 | 0 | 0 | 0 | ||
Blank (s) | 73,836 | 0 | 0 | 0 | 0 | 0 | 0 | ||
rx_site | |||||||||
0 | 25,064 | 2,375 (42.2) | 1,532 (34.5) | 743 (72.6) | 100 (64.9) | 985 (43.8) | 1,390 (41.2) | ||
10 | 306 | 24 (0.4) | 21 (0.5) | 2 (0.2) | 1 (0.6) | 7 (0.3) | 17 (0.5) | ||
20 | 6,848 | 557 (9.9) | 481 (10.8) | 61 (6.0) | 15 (9.7) | 217 (9.6) | 340 (10.1) | ||
30 | 3,305 | 279 (5.0) | 263 (5.9) | 14 (1.4) | 2 (1.3) | 115 (5.1) | 164 (4.9) | ||
40 | 8,884 | 827 (14.7) | 757 (17.0) | 59 (5.8) | 11 (7.1) | 329 (14.6) | 498 (14.8) | ||
50 | 11,782 | 1,326 (23.6) | 1,196 (26.9) | 107 (10.4) | 23 (14.9) | 500 (22.2) | 826 (24.5) | ||
60 | 2,213 | 216 (3.8) | 185 (4.2) | 30 (2.9) | 1 (0.6) | 89 (4.0) | 127 (3.8) | ||
70 | 204 | 16 (0.3) | 8 (0.2) | 7 (0.7) | 1 (0.6) | 6 (0.3) | 10 (0.3) | ||
90 | 390 | 0 | 0 | 0 | 0 | 0 | 0 | ||
99 | 694 | 0 | 0 | 0 | 0 | 0 | 0 | ||
Blank (s) | 34,489 | 0 | 0 | 0 | 0 | 0 | 0 | ||
rx_reg | |||||||||
None | 42,000 | 5,398 (96.0) | 4,267 (96.0) | 981 (95.9) | 150 (97.4) | 2,160 (96.1) | 3,238 (96.0) | ||
Other | 2,218 | 222 (4.0) | 176 (4.0) | 42 (4.1) | 4 (2.6) | 88 (3.9) | 134 (4.0) | ||
Blank (s) | 49,426 | 0 | 0 | 0 | 0 | 0 | 0 | ||
Unknown | 535 | 0 | 0 | 0 | 0 | 0 | 0 | ||
Size | |||||||||
0 | 23 | 0 | 0 | 0 | 0 | 0 | 0 | ||
≤30 | 11,173 | 2,254 (40.1) | 2,110 (47.5) | 102 (10.0) | 42 (27.3) | 871 (38.7) | 1,383 (41.0) | ||
>30, ≤50 | 6,314 | 1,450 (25.8) | 1,147 (25.8) | 258 (15.4) | 45 (29.2) | 594 (26.4) | 856 (25.4) | ||
>50, ≤100 | 7,396 | 1,813 (32.2) | 1,145 (25.8) | 605 (59.1) | 63 (40.9) | 751 (33.4) | 1,062 (31.5) | ||
>100 | 622 | 103 (1.8) | 41 (0.9) | 58 (5.7) | 4 (2.6) | 32 (1.4) | 71 (2.1) | ||
888 | 1 | 0 | 0 | 0 | 0 | 0 | 0 | ||
990 | 1,025 | 0 | 0 | 0 | 0 | 0 | 0 | ||
999 | 14,676 | 0 | 0 | 0 | 0 | 0 | 0 | ||
Blank (s) | 52,949 | 0 | 0 | 0 | 0 | 0 | 0 | ||
Exten | |||||||||
<200 | 7,684 | 752 (13.4) | 732 (16.5) | 10 (1.0) | 10 (6.5) | 293 (13.0) | 459 (13.6) | ||
≥200, <300 | 11,438 | 2,071 (36.8) | 1,868 (42.0) | 172 (16.8) | 31 (20.1) | 822 (36.6) | 1249 (37.0) | ||
≥300, <500 | 5,584 | 643 (11.4) | 497 (11.2) | 117 (11.4) | 29 (18.8) | 256 (11.4) | 387 (11.5) | ||
≥500, <600 | 6,011 | 1,029 (18.3) | 768 (17.3) | 223 (21.8) | 38 (24.7) | 428 (19.0) | 601 (17.8) | ||
≥600, <700 | 6,140 | 921 (16.4) | 484 (10.9) | 399 (39.0) | 38 (24.7) | 359 (16.0) | 562 (16.7) | ||
≥700, <999 | 1,438 | 204 (3.6) | 94 (2.1) | 102 (10.0) | 8 (5.2) | 90 (4.0) | 114 (3.4) | ||
Blank (s) | 52,949 | 0 | 0 | 0 | 0 | 0 | 0 | ||
LN | |||||||||
No involvement of lymph nodes | 28,801 | 3,938 (70.1) | 3,338 (75.1) | 489 (47.8) | 111 (72.1) | 1,564 (69.6) | 2,374 (70.4) | ||
Lymphoid involvement | 12,429 | 1,682 (29.9) | 1105 (24.9) | 534 (52.2) | 43 (27.9) | 684 (30.4) | 998 (29.6) | ||
Blank (s) | 52,949 | 0 | 0 | 0 | 0 | 0 | 0 | ||
Mets_dx | |||||||||
0 | 33,973 | 5,062 (90.1) | 4,204 (94.6) | 721 (70.5) | 137 (89.0) | 2,030 (90.3) | 3,032 (89.9) | ||
1–99 | 7,257 | 558 (9.9) | 239 (5.4) | 302 (29.5) | 17 (11.0) | 218 (9.7) | 340 (10.1) | ||
Blank (s) | 52,949 | 0 | 0 | 0 | 0 | 0 | 0 | ||
Canc_dth | |||||||||
Alive or dead of other cause | 20,714 | 4,597 (81.8) | 1,834 (81.6) | 2,763 (81.9) | |||||
Dead | 25,981 | 1,023 (18.2) | 414 (18.4) | 609 (18.1) | |||||
Dead (missing/unknown COD) | 1,254 | 0 | 0 | 0 | |||||
N/A not first tumor | 6,230 | 0 | 0 | 0 | |||||
Oth_dth | |||||||||
Alive or dead due to cancer | 73,388 | 5,466 (97.2) | 2,185 (97.2) | 3,281 (97.3) | |||||
Dead of others | 13,307 | 154 (2.7) | 63 (2.8) | 91 (2.7) | |||||
Dead (missing/unknown COD) | 1,254 | 0 | 0 | 0 | |||||
N/A not first tumor | 6,230 | 0 | 0 | 0 | |||||
Status | |||||||||
Alive | 49,830 | 4,443 (79.0) | 4,443 (100.0) | 0 (0) | 0 (0) | 1,771 (78.8) | 2,672 (79.2) | ||
Dead | 44,349 | 1,177 (20.9) | 0 (0) | 1,023 (100.0) | 154 (100.0) | 477 (21.2) | 700 (20.8) | ||
Seq_num | |||||||||
One primary only | 78,299 | 5,359 (95.4) | 4,251 (95.7) | 982 (96.0) | 126 (81.8) | 2,128 (94.7) | 3,231 (95.8) | ||
1st of 2 or more primaries | 15,875 | 261 (4.6) | 192 (4.3) | 41 (4.0) | 28 (18.2) | 120 (5.3) | 141 (4.2) | ||
Unknown seq num | 5 | 0 | 0 | 0 | 0 | 0 | 0 | ||
Total_malig | |||||||||
1 | 79,161 | 5,407 (96.2) | 4,292 (96.6) | 987 (96.5) | 128 (83.1) | 2,156 (95.9) | 3,251 (96.4) | ||
2 | 12,497 | 199 (3.5) | 140 (3.2) | 35 (3.4) | 24 (15.6) | 87 (3.9) | 112 (3.3) | ||
3 | 2,061 | 13 (0.2) | 10 (0.2) | 1 (<0.1) | 2 (1.3) | 5 (0.2) | 8 (0.2) | ||
4 | 378 | 1 (<0.1) | 1 (<0.1) | 0 (0) | 0 (0) | 0 | 1 (<0.1) | ||
5–10 | 77 | 0 | 0 | 0 | 0 | 0 | 0 | ||
Unknown | 5 | 0 | 0 | 0 | 0 | 0 | 0 | ||
Total_begn | |||||||||
0 | 93,988 | 5,608 (99.8) | 4,434 (99.8) | 1,020 (99.7) | 154 (100.0) | 2,243 (99.8) | 3,365 (99.8) | ||
1 | 185 | 12 (0.2) | 9 (0.2) | 3 (0.3) | 0 (0) | 5 (0.2) | 7 (0.2) | ||
2 | 6 | 0 | 0 | 0 | 0 | 0 | 0 | ||
Age_diag | |||||||||
3–19 | 172 | 0 | 0 | 0 | 0 | 0 | 0 | ||
20–34 | 14,956 | 899 (16.0) | 751 (16.9) | 143 (14.0) | 5 (3.2) | 358 (15.9) | 541 (16.0) | ||
35–39 | 10,958 | 652 (11.6) | 578 (13.0) | 73 (7.1) | 1 (0.6) | 260 (11.6) | 392 (11.6) | ||
40–44 | 11,866 | 750 (13.3) | 625 (14.1) | 117 (11.4) | 8 (5.2) | 291 (12.9) | 459 (13.6) | ||
45–49 | 10,914 | 716 (12.7) | 561 (12.6) | 141 (13.8) | 14 (9.1) | 287 (12.8) | 429 (12.7) | ||
50–54 | 9,598 | 687 (12.2) | 530 (11.9) | 139 (13.6) | 18 (11.7) | 280 (12.4) | 407 (12.0) | ||
55–59 | 8,343 | 553 (9.8) | 430 (9.7) | 106 (10.4) | 17 (11.0) | 237 (10.5) | 316 (9.4) | ||
60–69 | 13,573 | 829 (14.8) | 623 (14.0) | 164 (16.0) | 42 (27.3) | 332 (14.8) | 497 (14.7) | ||
70–99 | 13,773 | 514 (9.1) | 345 (7.8) | 120 (11.7) | 49 (31.8) | 203 (9.0) | 311 (9.2) | ||
100–104 | 26 | 0 | 0 | 0 | 0 | 0 | 0 | ||
Mrit | |||||||||
Single | 20,585 | 1,887 (33.6) | 1,466 (33.0) | 392 (38.3) | 29 (18.8) | 760 (33.8) | 1,127 (33.4) | ||
Married or partner | 41,892 | 2,385 (42.4) | 1,982 (44.6) | 350 (34.2) | 53 (34.4) | 929 (41.3) | 1,456 (43.2) | ||
Separated divorced or widowed | 26,227 | 1,348 (24.0) | 995 (22.4) | 281 (27.5) | 72 (46.8) | 559 (24.9) | 789 (23.4) | ||
Unknown | 5,475 | 0 | 0 | 0 | 0 | 0 | 0 |
Determination of independent risk factors affecting the prognosis of patients
The Cox proportional risk regression model was used to conduct a univariate analysis of all variables in the training cohort, and the results showed that age, race, grade, Derived AJCC Stage Group, Derived AJCC T, Derived AJCC N, Derived AJCC M, RX Summ-Surg Prim Site, tumor size, CS extension, CS lymph nodes, CS Mets at dx, SEER cause-specific death classification, SEER other cause of death classification, age at diagnosis, marital status at diagnosis are all correlated with the prognosis of cervical cancer patients (P<0.05), which has statistical significance (Table S1). The meaningful variables obtained by univariate Cox proportional risk regression analysis were carried out for multivariate Cox proportional risk regression analysis. Age, grade, Derived AJCC M, RX Summ-Surg Prim Site, tumor size, CS Mets at dx were independent risk factors affecting the prognosis of cervical cancer patients (Table S1).
Then, the COX proportional risk regression model was used for univariate analysis of all variables in the verification cohort, and the results showed that age, race, grade, Derived AJCC Stage Group, Derived AJCC T, Derived AJCC N, Derived AJCC M, RX Summ-Surg Prim Site, tumor size, CS extension, CS lymph nodes, CS Mets at dx, SEER cause-specific death classification, SEER other cause of death classification, total number of in situ/malignant tumors for a patient, age at diagnosis, marital status at diagnosis are all related to the prognosis of cervical cancer patients (P<0.05), which have statistical significance (Table S2). The meaningful variables obtained by univariate Cox proportional risk regression analysis were carried out for multivariate Cox proportional risk regression analysis. Age, RX Summ-Surg Prim Site, tumor size, Total number of in situ/malignant tumors for patients are independent risk factors affecting the prognosis of cervical cancer (Table S2).
Finally, COX proportional risk regression model was used for univariate analysis of the overall data after cleaning, and the results showed that age, race, grade, Derived AJCC Stage Group, Derived AJCC T, Derived AJCC N, Derived AJCC M, RX Summ-Surg Prim Site, tumor size, CS extension, CS lymph nodes, CS Mets at dx, SEER cause-specific death Classification, SEER other cause of death classification, age at diagnosis, and marital status at diagnosis are all correlated with the prognosis of cervical cancer patients (P<0.05), which has statistical significance (Table 2). The meaningful variables obtained by univariate Cox proportional risk regression analysis were carried out for multivariate Cox proportional risk regression analysis. It was concluded that age, grade, RX Summ-Surg Prim Site and tumor size were independent risk factors affecting the prognosis of cervical cancer patients (Table 2).
Table 2
Variable | Univariate analysis | Multivariate analysis | |||||||
---|---|---|---|---|---|---|---|---|---|
HR | 95% CI | P | C-Index | SE | HR | 95% CI | P | ||
Age | 0.599 | 0.009 | |||||||
20–29 | 1 | Reference | 1 | Reference | |||||
30–34 | 0.999 | 0.714–1.398 | 0.995 | 0.606 | 0.428–0.858 | 0.005 | |||
35–39 | 0.685 | 0.482–0.975 | 0.036 | 0.654 | 0.455–0.940 | 0.022 | |||
40–44 | 0.998 | 0.724–1.376 | 0.99 | 0.664 | 0.478–0.922 | 0.015 | |||
45–49 | 1.332 | 0.975–1.820 | 0.071 | 0.63 | 0.456–0.872 | 0.005 | |||
50–54 | 1.455 | 1.066–1.987 | 0.018 | 0.602 | 0.434–0.835 | 0.002 | |||
55–59 | 1.428 | 1.035–1.970 | 0.03 | 0.595 | 0.422–0.837 | 0.003 | |||
60–64 | 1.502 | 1.084–2.081 | 0.014 | 0.606 | 0.373–0.986 | 0.044 | |||
65–74 | 1.742 | 1.275–2.382 | <0.001 | 0.708 | 0.477–1.051 | 0.086 | |||
≥75 | 3.156 | 2.292–4.345 | <0.001 | 0.902 | 0.639–1.274 | 0.558 | |||
Race | 0.527 | 0.007 | |||||||
Black | 1 | Reference | 1 | Reference | |||||
White | 0.712 | 0.614–0.824 | <0.001 | 0.994 | 0.851–1.160 | 0.935 | |||
Other | 0.676 | 0.540–0.846 | 0.001 | 1.118 | 0.885–1.412 | 0.349 | |||
Grade | 0.569 | 0.008 | |||||||
Grade I | 1 | Reference | 1 | Reference | |||||
Grade II | 2.008 | 1.475–2.733 | <0.001 | 0.771 | 0.561–1.060 | 0.109 | |||
Grade III | 2.961 | 2.181–4.020 | <0.001 | 0.795 | 0.578–1.093 | 0.158 | |||
Grade IV | 2.69 | 1.555–4.656 | <0.001 | 0.55 | 0.310–0.979 | 0.042 | |||
Stage | 0.766 | 0.006 | |||||||
IA | 1 | Reference | 1 | Reference | |||||
IB | 2.986 | 1.826–4.881 | <0.001 | 1.134 | 0.245–5.239 | 0.872 | |||
IIA | 10.058 | 5.914–17.108 | <0.001 | 1.345 | 0.284–6.365 | 0.708 | |||
IIB | 7.634 | 4.642–12.554 | <0.001 | 1.206 | 0.264–5.516 | 0.81 | |||
III | 13.91 | 8.688–22.269 | <0.001 | 1.099 | 0.242–4.981 | 0.903 | |||
IV | 39.82 | 24.805–63.924 | <0.001 | 1.406 | 0.294–6.727 | 0.67 | |||
Stag_T | 0.748 | 0.007 | |||||||
T1a | 1 | Reference | 1 | Reference | |||||
T1b | 3.722 | 2.383–5.813 | <0.001 | 0.77 | 0.102–5.789 | 0.8 | |||
T2a | 9.859 | 6.182–15.723 | <0.001 | 0.57 | 0.076–4.238 | 0.582 | |||
T2b | 9.939 | 6.369–15.508 | <0.001 | 0.353 | 0.039–3.185 | 0.353 | |||
T3a | 26.141 | 16.306–41.907 | <0.001 | 1.53 | 0.362–6.460 | 0.563 | |||
T3b | 24.277 | 15.621–37.729 | <0.001 | 1.709 | 0.407–7.169 | 0.464 | |||
T4 | 32.652 | 20.466–52.093 | <0.001 | 1.665 | 0.385–7.192 | 0.495 | |||
Stag_N | 0.621 | 0.008 | |||||||
N0 | 1 | Reference | 1 | Reference | |||||
N1 | 2.712 | 2.419–3.041 | <0.001 | 1.145 | 0.975–1.346 | 0.099 | |||
Stag_M | 0.623 | 0.007 | |||||||
M0 | 1 | Reference | 1 | Reference | |||||
M1 | 5.986 | 5.251–6.824 | <0.001 | 0.951 | 0.389–2.322 | 0.912 | |||
rx_site | 0.704 | 0.007 | |||||||
0 | 1 | Reference | 1 | Reference | |||||
10–19 | 0.248 | 0.080–0.770 | 0.016 | 0.586 | 0.183–1.874 | 0.368 | |||
20–29 | 0.327 | 0.258–0.413 | <0.001 | 0.986 | 0.767–1.268 | 0.915 | |||
30–39 | 0.104 | 0.063–0.170 | <0.001 | 0.868 | 0.517–1.457 | 0.591 | |||
40–49 | 0.195 | 0.153–0.249 | <0.001 | 0.782 | 0.602–1.016 | 0.066 | |||
50–59 | 0.201 | 0.167–0.242 | <0.001 | 0.735 | 0.597–0.904 | 0.004 | |||
60–64 | 0.311 | 0.217–0.445 | <0.001 | 0.839 | 0.576–1.222 | 0.36 | |||
65–75 | 1.251 | 0.623–2.510 | 0.529 | 0.451 | 0.209–0.971 | 0.042 | |||
rx_reg | 0.504 | 0.003 | |||||||
None | 1 | Reference | |||||||
Other* | 0.938 | 0.699–1.260 | 0.672 | ||||||
Size | 0.729 | 0.007 | |||||||
≤30 | 1 | Reference | 1 | ||||||
>30, ≤50 | 3.593 | 2.946–4.382 | <0.001 | 1.073 | 0.866–1.330 | 0.517 | |||
>50, ≤100 | 7.846 | 6.551–9.397 | <0.001 | 1.509 | 1.226–1.859 | <0.001 | |||
>100 | 17.017 | 12.629–22.931 | <0.001 | 1.874 | 1.344–2.613 | <0.001 | |||
Extension | 0.753 | 0.007 | |||||||
<200 | 1 | Reference | 1 | ||||||
≥200, <300 | 3.471 | 2.192–5.494 | <0.001 | 1.891 | 0.160–22.322 | 0.613 | |||
≥300, <500 | 9.403 | 5.893–15.005 | <0.001 | 2.647 | 0.230–30.522 | 0.435 | |||
≥500, <600 | 10.352 | 6.569–16.312 | <0.001 | 3.67 | 0.267–50.373 | 0.33 | |||
≥600, <700 | 25.492 | 16.280–39.915 | <0.001 | NA | NA | NA | |||
≥700, <999 | 33.745 | 20.947–54.362 | <0.001 | NA | NA | NA | |||
LN | 0.621 | 0.008 | |||||||
No involvement of lymph nodes | 1 | Reference | 1 | ||||||
Lymphoid involvement | 2.712 | 2.419–3.041 | <0.001 | NA | NA | NA | |||
Mets_dx | 0.625 | 0.007 | |||||||
0 | 1 | Reference | 1 | ||||||
1–99 | 5.992 | 5.261–6.826 | <0.001 | 1.476 | 0.646–3.371 | 0.356 | |||
Canc_dth | 0.871 | 0.005 | |||||||
Alive or dead of other cause | 1 | Reference | 1 | ||||||
Dead | 61.379 | 51.440–73.250 | <0.001 | 3.98E+09 | 0.000–Inf | 0.974 | |||
Oth_dth | 0.548 | 0.004 | |||||||
Alive or dead due to cancer | 1 | Reference | 1 | ||||||
Dead of others | 6.405 | 5.404–7.591 | <0.001 | 3.30E+09 | 0.000–Inf | 0.975 | |||
seq_num | 0.495 | 0.003 | |||||||
One primary only | 1 | Reference | |||||||
1st of 2 or more primaries | 1.048 | 0.822–1.336 | 0.707 | ||||||
Total_malig | 0.497 | 0.003 | |||||||
1 | 1 | Reference | |||||||
2 | 1.128 | 0.868–1.465 | 0.369 | ||||||
3 | 0.892 | 0.287–2.771 | 0.844 | ||||||
4 | <0.001 | 0.000–Inf | 0.981 | ||||||
Total_begn | 0.501 | 0.001 | |||||||
0 | 1 | Reference | |||||||
1 | 1.018 | 0.328–3.161 | 0.975 | ||||||
Age_diag | 0.598 | 0.009 | |||||||
20–34 | 1 | Reference | 1 | ||||||
35–39 | 0.686 | 0.519–0.906 | 0.008 | NA | NA | NA | |||
40–44 | 0.999 | 0.787–1.267 | 0.991 | NA | NA | NA | |||
45–49 | 1.333 | 1.064–1.670 | 0.012 | NA | NA | NA | |||
50–54 | 1.456 | 1.163–1.823 | 0.001 | NA | NA | NA | |||
55–59 | 1.429 | 1.125–1.815 | 0.003 | NA | NA | NA | |||
60–69 | 1.564 | 1.267–1.932 | <0.001 | 1.035 | 0.736–1.456 | 0.844 | |||
70–99 | 2.617 | 2.110–3.246 | <0.001 | NA | NA | NA | |||
Mrit | 0.567 | 0.008 | |||||||
Single | 1 | Reference | 1 | ||||||
Married or partner | 0.709 | 0.618–0.813 | <0.001 | 0.913 | 0.789–1.055 | 0.218 | |||
Separated divorced or widowed | 1.204 | 1.045–1.387 | 0.01 | 1.161 | 0.990–1.360 | 0.066 |
*, it is include non-primary surgical procedure performed, non-primary surgical procedure to other regional sites, non-primary surgical procedure to distant lymph node(s), non-primary surgical procedure to distant site and any combination of surgical procedure to other regional, distant lymph node, and/or distant site (combination of codes 2, 3, or 4). inf, infinite; NA, not application.
In summary, through the intersection of the three groups of data results, we found that the variables with statistical significance of cervical cancer are age, RX Summ-Surg Prim Site, tumor size.
Nomogram model and validation
According to the meaningful risk factors obtained by the training group and the validation group, we prepared the corresponding Nomogram respectively, and obtained the final independent risk factors (age, RX Summ-Surg Prim Site, tumor size) and their nomogram through the intersection of the three data cohorts (Figure 2). For each variable, the corresponding score of each item was obtained according to the small points in the first line corresponding to the tumor situation, and then the total value was added corresponding to the overall scale at the bottom, and corresponding downward, the overall survival rate of patients at 1, 3 and 5 years could be obtained.
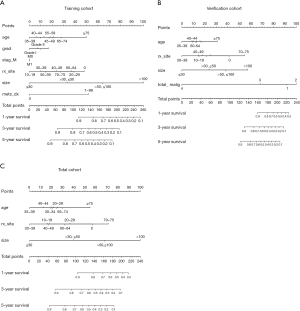
The C-index of the training cohort was 0.792, the C-index of the verification cohort was 0.778, and the C-index of the overall group was 0.771, with little difference in values and high accuracy in prediction. The nomogram was internally verified by the Bootstrap method, and the fitting coefficient b=1,600. The calibration of 1-, 3-, and 5-year survival rates in the training cohort (Figure S1A,B,C), verification cohort (Figure S1D,E,F), and total cohort (Figure 3A,B,C) were shown in the figure respectively. It can be seen that the slope of the consistency curve of the calibration graphs of the training cohort and the verification cohort is close to 1, indicating that there is good consistency between the predicted value and the actual observed value.
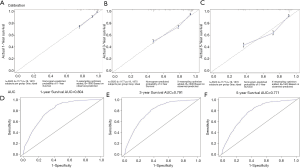
Finally, the prediction ability of the nomogram was evaluated by ROC curve. The AUC of 1, 3 and 5 years in the training cohort (0.841, 0.8 and 0.795; Figure S2A,B,C), the AUC of 1, 3 and 5 years in the verification cohort (0.801, 0.798 and 0.768; Figure S2D,E,F) and the AUC of 1, 3 and 5 years in the overall group (0.804, 0.791 and 0.771; Figure 3D,E,F) were all located at (0.71, 0.9), and all had a high predictive value of accuracy.
Prognosis and survival analysis of cervical cancer patients
The overall model has good recognition ability. According to the respective nomograms, we obtained the survival curves of the training cohort, the verification cohort and the overall cohort, respectively. According to the nomogram established in this study, the survival curve of our high-risk patients will decline faster (Figure 4A). In the overall group, the 1-, 3- and 5-year high-risk survival rates were 79.2%, 56.0% and 47.5%, respectively, and the low-risk survival rates were 98.0%, 90.9% and 85.5%, respectively (Figure S3A,B; Table 3). The median survival time in the age group greater than 75 years was 37 months. The 5-year survival rates were higher than 80% in patients who had both local tumor resection and hysterectomy, thus not draw the median survival time. On the contrary, for those who had not had primary site surgery or had only pelvic exenteration, the 5-year survival rate was particularly low, and intermediate survival time was 46.2 and 22.5 months, respectively. In the grouping of tumor size, the survival rate was lower as the tumor size increased, and only the median survival time (>50, ≤100) and (>100) were shown 41.7 and 13.2 months respectively (Table 3; Figure 4B,C,D). The 1-, 3-, and 5-year survival rates of age, RX Summ-Surg Prim Site, tumor size are shown in Table 3.
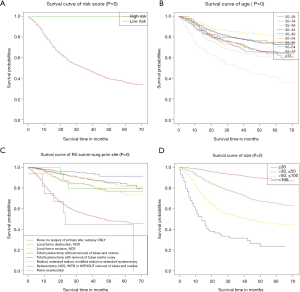
Table 3
Variable | Median survival time | 1-year survival rate | 3-year survival rate | 5-year survival rate |
---|---|---|---|---|
Risk_level | ||||
Training cohort | ||||
High | 48 | 0.79 | 0.566 | 0.455 |
Low | NA | 0.98 | 0.905 | 0.839 |
Verification cohort | ||||
High | 49.8 | 0.795 | 0.559 | 0.488 |
Low | NA | 0.978 | 0.914 | 0.854 |
Total cohort | ||||
High | 47.9 | 0.792 | 0.56 | 0.475 |
Low | NA | 0.98 | 0.909 | 0.855 |
Age | ||||
20–29 | NA | 0.914 | 0.789 | NA |
30–34 | NA | 0.918 | 0.784 | NA |
35–39 | NA | 0.946 | 0.849 | NA |
40–44 | NA | 0.918 | 0.783 | NA |
45–49 | NA | 0.903 | 0.74 | 0.65 |
50–54 | NA | 0.875 | 0.712 | 0.612 |
55–59 | NA | 0.867 | 0.733 | 0.904 |
60–64 | NA | 0.869 | 0.698 | NA |
65–74 | NA | 0.864 | 0.66 | 0.57 |
≥75 | 37 | 0.704 | 0.508 | 0.363 |
rx_site | ||||
0 | 46.2 | 0.788 | 0.539 | 0.467 |
10–19 | NA | 0.938 | NA | NA |
20–29 | NA | 0.93 | 0.83 | 0.733 |
30–39 | NA | 0.977 | 0.941 | NA |
40–49 | NA | 0.959 | 0.891 | 0.828 |
50–59 | NA | 0.969 | 0.892 | NA |
60–64 | NA | 0.946 | 0.822 | NA |
65–75 | 22.5 | 0.67 | NA | NA |
Size | ||||
≤30 | NA | 0.982 | 0.922 | 0.881 |
>30, ≤50 | NA | 0.91 | 0.748 | 0.644 |
>50, ≤100 | 41.7 | 0.771 | 0.52 | 0.448 |
>100 | 13.2 | 0.523 | 0.289 | NA |
NA, not application.
Discussion
Cervical squamous cell carcinoma is one of the most common subtypes of cervical cancer. We conducted a practical analysis of patients in the SEER database and established a prognostic Nomogram and risk score system. Nomogram has been used to predict the survival of various cancers. The C-index, calibration, and ROC curves show that Nomogram performs well both internally and externally. Because Nomogram quantifies risk by combining and illustrating the relative importance of various prognostic factors, it has been used in clinical tumor evaluation (8). In the study, six variables were identified as independent prognostic variables for overall survival, including age, RX Summ-Surg Prim Site, and size.
Cervical cancer is one kind of cancer peculiar to women, and it is also a disease closely related to middle age. Meanwhile, there are a large number of elderly patients over the age of 55. Studies by Landoni and Quinn et al. have shown that the increase in age is an independent hazard ratio for the increased mortality of cervical cancer patients (6,9). In this study, the risk ratio of cervical cancer began to increase significantly in patients aged >45, and the 1-, 3-, and 5-year survival rates began to decline. It is well known that menopause in women between the ages of 45 and 55 results in dramatic changes in physical and psychological functioning, including a lack of sex hormones such as estrogen, as well as physical conditions (10). It is currently known that long-term exposure to sex hormones is one of the risk factors for cervical cancer (11,12). Studies have found that estrogen receptor (ER) and HPV genomes are highly displayed sequences. ER alpha receptor activated by estrogen can be combined with the control elements in the HPV gene to increase the level of HPVE6/E7 mRNA. It promotes the production of viral oncoprotein, while the progression of cervical cancer is related to the increased expression of a viral oncogene (13-15). For example, increasing estrogen levels through the long-term use of oral contraceptives significantly increases the risk of developing cervical cancer (16,17). Estrogen has been identified as one of the major drivers of cervical cancer (18), but the controlled ovarian hyperstimulation (COH) through in vitro fertilization (IVF) does not increase the risk of cervical cancer (19). Among survivors of cervical cancer, estrogen replacement therapy is also used to improve prognosis and increase survival (20). This may be mainly due to decreased expression of sex steroid hormone receptors in irradiated cervical cancer survivors (21). In this way, estrogen replacement therapy can reduce other chronic diseases after estrogen inactivation without inducing the recurrence of cervical cancer. It can be concluded from previous studies that the use of estrogen concentration, frequency, mode, period and other factors will influence the occurrence, treatment and prognosis of cervical cancer. There is an interaction between HPV and estrogen (13). We speculate that there may be two induction mechanisms of HPV. One is the viral oncoprotein, mostly premenopausal. Another is that when estrogen is not released enough after menopause, the virus directly stimulates the upregulation of ER receptors in order to obtain estrogen, so that epithelial cells excessive proliferation, thereby inducing cervical cancer. Because the second type of direct stimulation is more rapid, it may be employed as an explanation for the fact that the postmenopausal survival rate is relatively low. But further experiments are needed on exactly what kind of mechanism it is.
Surgery is one of the most effective treatments for cervical cancer. Radical hysterectomy or chemoradiotherapy is the standard treatment for patients with early cervical cancer (22,23). In this study, the recurrent mortality rate of patients with different degrees of surgery was only 8.04%, and the survival rate was higher than that without surgery. The prognosis of total hysterectomy with tubal and ovary preserved was good, and the 1- and 3-year survival rates were 97.7% and 94.1%, respectively, higher than other surgical procedures. Many studies have also shown that ovarian preservation is an important factor in determining cervical cancer surgery in young women (24,25). At the same time, the study by Zhou et al. also reported that the metastasis rate of non-squamous cell carcinoma was higher than that of squamous cell carcinoma in the case of ovarian reservation, and the metastasis rate was also increased in young patients due to the abundant vascular network. Both conditions reduce the survival rate for tubal sparing and total ovarian hysterectomy. That’s why some clinical cases show the lowest survival rates for cervical cancer with surgical sterilization (24). The most common type of recurrence after hysterectomy is the pelvic region, especially in advanced cancer (stage III–IV) (4,26,27). Therefore, the postoperative residual tumor should be carefully assessed. In addition, studies have shown that for patients undergoing preoperative radiotherapy, the overall pathological remission rate of squamous cell carcinoma patients is higher than that of adenocarcinoma patients, which may be related to residual tumor and increased risk of local diseases. However, the data that we have don’t have all the data on radiation therapy, so we can’t compare the effects of surgery after radiation therapy. At present, hysterectomy is divided into minimally invasive and open. Studies have shown that in the early uterine tumor (stage I), minimally invasive surgery (MIS) and open surgery survival rates are similar (22), and short-term safety of MIS is higher than open surgery, with fewer complications, less pain, faster recovery, and significantly shorter hospital stays (28). However, other studies have also proved that considering the difference in histological type and tumor size, the risk of MIS is significantly higher than that of open surgery, and the tumor size is greater than 2 cm (29,30), here we hypothesize that this may be due to differences in surgical operator ability that correlate risk with histologic type and tumor size. As opposed to early cervical cancer, multi-mode treatment, including hysterectomy, can also improve the survival rate of LACC patients, but its clinical role is still unclear (4,6). Urinary toxicity is the most common postoperative complication. One study found that patients who underwent hysterectomy had a twofold increased risk of urinary fistula compared to those who received specific radiation (31). Pelvic exenteration refers to the radical or sweeping resection of the entire pelvic tumor, but this surgery is very harmful to patients. In this study, only 16 patients were performed, but nearly half of the death rate was also found. In the latest Clinical Practice Guidelines for cervical cancer, patients who are locally treated with stage I–II are typically treated with a cervical or hysterectomy followed by radiotherapy. However, similar studies have shown that for cervical adenocarcinoma, chemoradiotherapy plus hysterectomy has a better survival outcome (32). Other studies have also shown that only surgery can accurately evaluate the pathological response to chemoradiotherapy, and in fact, tumors often remain after radiotherapy, which further reflects the advantages of surgical assistance in local treatment (33).
Although hysterectomy has a good prognosis and it is very difficult to maintain the fertility of young women in the future, this type of female prefers uterine-preserving surgery (UPS), but according to NCCN Clinical Practice Guidelines in Cervical Cancer, UPS is only selected for patients before stage IB1. In related studies, in patients undergoing UPS, only 58.8% of the people of the true success of retain fertility (90.8% of the patients with tumor is equal to or less than 20 mm), but this study, comparing the patients have no UPS so not to do detailed analysis (34), but at the same time to preserve fertility and good prognosis of young female patients still need to be careful choice.
Tumor size has long been considered as an independent prognostic factor affecting the survival of cervical cancer. As with the previous studies (35-37), the larger the tumor size, the lower the survival rate. Compared with other statistically significant HR, the risk ratio of tumor size was relatively high (3.071), which was also the most influential factor in Nomogram. Some studies have shown that grade in early cervical cancer has the most significant effect on prognosis, while tumor size is the most significant in advanced cervical cancer, so the treatment is a little different (35). In our study, only total hysterectomy data were shown, but all patients with diameters of 100 mm or less had undergone a total hysterectomy to varying degrees, and the survival rate reached 80.0%. This more directly reflected in the surgery, the tumor diameter small (less than or equal to 20 mm) can use the uterus to keep operation can achieve good prognosis as well as retain complete fertility, but a hysterectomy in tumor diameter greater than 20 mm more significant effect on the survival rate, survival rate was high, should be a priority. This is more directly reflected in surgical treatment. Small-diameter tumors (less than or equal to 20 mm) can use uterine retention surgery to obtain a good prognosis while retaining intact fertility, and hysterectomy has a greater impact on survival in tumors larger than 20 mm in diameter. But overall, for tumors larger than 20 mm in diameter, the risk of surgical patients also increased with the increase in diameter (29,34,38).
We study one advantage is that it is a population-based study surveyed, the largest U.S. cancer registry. But there are limitations. First, radiotherapy and chemotherapy are the most important strategies for the treatment of cervical cancer, but there is no information on radiotherapy and chemotherapy in the SEER database, so a better treatment plan cannot be analyzed. Second, SEER lacks clinical information, especially the preoperative features and postoperative complications of hysterectomy. Also, our study found that there is a higher survival rate in total hysterectomy with the retention of ovaries and fallopian tubes than with the removal of both; Third, the study was limited to the U.S. population, and the results may not be adaptive to the global population.
In summary, our study determined that age, RX Summ-Surg Prim Site and tumor size at the distance were independent risk factors for cervical cancer. In addition, for early (stage I) or tumor diameter of less than 20 mm, minimally invasive hysterectomy had better surgical success rate and higher survival rate of the patients with uterine surgery can be preserved to keep women’s fertility; Advanced cases of stage IIB and above are usually not treated with surgery. For most patients with stage III–IV or tumor diameter greater than 20 mm, chemoradiotherapy is still used.
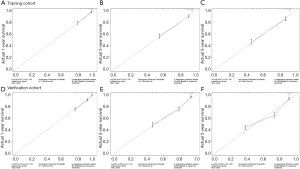
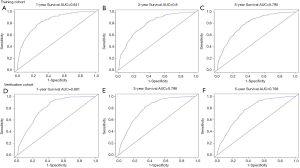
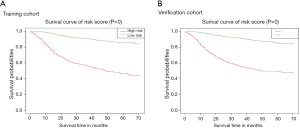
Table S1
Variable | Univariate analysis | Multivariate analysis | |||||||
---|---|---|---|---|---|---|---|---|---|
HR | 95% CI | P | C-Index | SE | HR | 95% CI | P | ||
Age | 0.613 | 0.013 | |||||||
20–29 | 1 | Reference | 1 | Reference | |||||
30–34 | 1 | 0.589–1.701 | 0.997 | 0.417 | 0.233–0.746 | 0.003 | |||
35–39 | 0.72 | 0.414–1.253 | 0.245 | 0.669 | 0.371–1.206 | 0.182 | |||
40–44 | 0.926 | 0.554–1.549 | 0.771 | 0.744 | 0.431–1.284 | 0.288 | |||
45–49 | 1.407 | 0.866–2.286 | 0.168 | 0.93 | 0.551–1.571 | 0.787 | |||
50–54 | 1.55 | 0.955–2.517 | 0.076 | 0.621 | 0.364–1.060 | 0.08 | |||
55–59 | 1.634 | 1.002–2.667 | 0.049 | 0.643 | 0.374–1.106 | 0.11 | |||
60–64 | 1.628 | 0.980–2.707 | 0.06 | 0.665 | 0.300–1.474 | 0.315 | |||
65–74 | 2.012 | 1.241–3.263 | 0.004 | 0.894 | 0.470–1.701 | 0.733 | |||
≥75 | 3.496 | 2.117–5.772 | <0.001 | 1.133 | 0.647–1.983 | 0.662 | |||
Race | 0.539 | 0.011 | |||||||
Black | 1 | Reference | 1 | Reference | |||||
White | 0.622 | 0.497–0.779 | <0.001 | 1.016 | 0.789–1.307 | 0.904 | |||
Other | 0.553 | 0.385–0.794 | 0.001 | 1.28 | 0.854–1.921 | 0.232 | |||
Grade | 0.578 | 0.012 | |||||||
Grade I | 1 | Reference | 1 | Reference | |||||
Grade II | 1.821 | 1.161–2.858 | 0.009 | 0.648 | 0.403–1.040 | 0.072 | |||
Grade III | 2.883 | 1.848–4.498 | <0.001 | 0.665 | 0.414–1.066 | 0.09 | |||
Grade IV | 2.167 | 0.921–5.097 | 0.076 | 0.313 | 0.122–0.803 | 0.016 | |||
Stage | 0.756 | 0.011 | |||||||
IA | 1 | Reference | 1 | Reference | |||||
IB | 2.305 | 1.086–4.889 | 0.03 | 2.016 | 0.205–19.823 | 0.548 | |||
IIA | 8.831 | 3.967–19.660 | <0.001 | 1.86 | 0.179–19.281 | 0.603 | |||
IIB | 7.491 | 3.565–15.740 | <0.001 | 1.59 | 0.164–15.449 | 0.689 | |||
III | 12.448 | 6.140–25.239 | <0.001 | 1.536 | 0.164–14.343 | 0.706 | |||
IV | 32.29 | 15.842–65.817 | <0.001 | 1.726 | 0.168–17.770 | 0.646 | |||
Stag_T | 0.753 | 0.011 | |||||||
T1a | 1 | Reference | 1 | Reference | |||||
T1b | 2.895 | 1.455–5.763 | 0.002 | 1.152 | 0.090–14.770 | 0.913 | |||
T2a | 9.258 | 4.537–18.893 | <0.001 | 0.786 | 0.066–9.431 | 0.849 | |||
T2b | 9.617 | 4.880 –18.953 | <0.001 | 0.285 | 0.035–2.314 | 0.24 | |||
T3a | 25.02 | 12.136–51.582 | <0.001 | 0.98 | 0.120–8.015 | 0.985 | |||
T3b | 24.571 | 12.518–48.230 | <0.001 | 1.52 | 0.188–12.281 | 0.695 | |||
T4 | 25.276 | 12.332–51.810 | <0.001 | 1.094 | 0.131–9.117 | 0.934 | |||
Stag_N | 0.61 | 0.012 | |||||||
N0 | 1 | Reference | 1 | Reference | |||||
N1 | 2.499 | 2.088–2.992 | <0.001 | 1.13 | 0.874–1.461 | 0.352 | |||
Stag_M | 0.615 | 0.011 | |||||||
M0 | 1 | Reference | 1 | Reference | |||||
M1 | 5.506 | 4.474–6.777 | <0.001 | 0.049 | 0.010–0.232 | <0.001 | |||
rx_site | 0.693 | 0.01 | |||||||
0 | 1 | Reference | 1 | Reference | |||||
10–19 | 0.299 | 0.042–2.129 | 0.228 | 0.518 | 0.067–4.031 | 0.53 | |||
20–29 | 0.422 | 0.298–0.598 | <0.001 | 0.982 | 0.657–1.467 | 0.928 | |||
30–39 | 0.141 | 0.073–0.273 | <0.001 | 1.327 | 0.635–2.774 | 0.452 | |||
40–49 | 0.223 | 0.153–0.324 | <0.001 | 0.781 | 0.506–1.206 | 0.265 | |||
50–59 | 0.187 | 0.136–0.257 | <0.001 | 0.858 | 0.595–1.236 | 0.411 | |||
60–64 | 0.366 | 0.214–0.625 | <0.001 | 0.92 | 0.515–1.644 | 0.778 | |||
65–75 | 0.675 | 0.168–2.710 | 0.579 | 0.127 | 0.026–0.630 | 0.011 | |||
rx_reg | 0.501 | 0.005 | |||||||
None | 1 | Reference | |||||||
Other* | 1.239 | 0.808–1.902 | 0.325 | ||||||
Size | 0.726 | 0.01 | |||||||
≤30 | 1 | Reference | 1 | Reference | |||||
>30, ≤50 | 3.36 | 2.451–4.606 | <0.001 | 1.151 | 0.815–1.626 | 0.424 | |||
>50, ≤100 | 7.87 | 5.918–10.468 | <0.001 | 1.522 | 1.096–2.112 | 0.012 | |||
>100 | 17.793 | 10.334–30.637 | <0.001 | 3.071 | 1.661–5.674 | <0.001 | |||
Extension | 0.759 | 0.011 | |||||||
<200 | 1 | Reference | 1 | Reference | |||||
≥200, <300 | 2.486 | 1.240–4.986 | 0.01 | 0.864 | 0.206–3.629 | 0.841 | |||
≥300, <500 | 8.377 | 4.154–16.894 | <0.001 | 1.2 | 0.347–4.229 | 0.776 | |||
≥500, <600 | 9.563 | 4.856–18.836 | <0.001 | NA | NA | NA | |||
≥600, <700 | 24.238 | 12.405–47.362 | <0.001 | NA | NA | NA | |||
≥700, <999 | 24.838 | 12.118–50.911 | <0.001 | NA | NA | NA | |||
LN | 0.61 | 0.012 | |||||||
No involvement of lymph nodes | 1 | Reference | 1 | Reference | |||||
Lymphoid involvement | 2.499 | 2.088–2.992 | <0.001 | NA | NA | NA | |||
Mets_dx | 0.618 | 0.011 | |||||||
0 | 1 | Reference | 1 | Reference | |||||
1–99 | 5.544 | 4.509–6.815 | <0.001 | 35.55 | 7.917–159.604 | <0.001 | |||
Canc_dth | 0.871 | 0.008 | |||||||
Alive or dead of other cause | 1 | Reference | 1 | Reference | |||||
Dead | 56.66 | 43.150–74.390 | <0.001 | 3.76E+09 | 0–inf | 0.982 | |||
Oth_dth | 0.547 | 0.007 | |||||||
Alive or dead due to cancer | 1 | Reference | 1 | Reference | |||||
Dead of others | 6.236 | 4.779–8.138 | <0.001 | 2.88E+09 | 0–inf | 0.982 | |||
Seq_num | 0.511 | 0.005 | |||||||
One primary only | 1 | Reference | |||||||
1st of 2 or more primaries | 0.814 | 0.548–1.210 | 0.309 | ||||||
Total_malig | 0.51 | 0.004 | |||||||
1 | 1 | Reference | |||||||
2 | 0.776 | 0.490–1.227 | 0.278 | ||||||
3 | 0.914 | 0.128–6.506 | 0.929 | ||||||
Total_begn | 0.502 | 0.001 | |||||||
0 | 1 | Reference | |||||||
1 | <0.001 | 0.986 | |||||||
Age_diag | 0.613 | 0.013 | |||||||
20–34 | 1 | Reference | 1 | Reference | |||||
35–39 | 0.719 | 0.461–1.123 | 0.148 | NA | NA | NA | |||
40–44 | 0.926 | 0.624–1.374 | 0.702 | NA | NA | NA | |||
45–49 | 1.406 | 0.984–2.009 | 0.061 | NA | NA | NA | |||
50–54 | 1.55 | 1.086–2.211 | 0.016 | NA | NA | NA | |||
55–59 | 1.634 | 1.137–2.347 | 0.008 | NA | NA | NA | |||
60–69 | 1.694 | 1.211–2.369 | 0.002 | 0.97 | 0.566–1.661 | 0.91 | |||
70–99 | 3.011 | 2.143–4.230 | <0.001 | NA | NA | NA | |||
Mrit | 0.584 | 0.013 | |||||||
Single | 1 | Reference | 1 | Reference | |||||
Married or partner | 0.717 | 0.575–0.894 | 0.003 | 0.978 | 0.768–1.245 | 0.857 | |||
Separated divorced or widowed | 1.387 | 1.115–1.725 | 0.003 | 1.271 | 0.981–1.648 | 0.07 |
*, it is include non-primary surgical procedure performed, non-primary surgical procedure to other regional sites, non-primary surgical procedure to distant lymph node(s), non-primary surgical procedure to distant site and any combination of surgical procedure to other regional, distant lymph node, and/or distant site (combination of codes 2, 3, or 4). inf, infinite; NA, not application.
Table S2
Variable | Training cohort | Verification cohort | |||||||
---|---|---|---|---|---|---|---|---|---|
HR | 95% CI | P | C-Index | SE | HR | 95% CI | P | ||
Age | 0.59 | 0.012 | |||||||
20–29 | 1 | Reference | 1 | Reference | |||||
30–34 | 0.99 | 0.641–1.530 | 0.966 | 0.817 | 0.516–1.294 | 0.389 | |||
35–39 | 0.66 | 0.418–1.043 | 0.075 | 0.659 | 0.407–1.067 | 0.09 | |||
40–44 | 1.034 | 0.684–1.564 | 0.873 | 0.647 | 0.419–1.000 | 0.05 | |||
45–49 | 1.274 | 0.848–1.914 | 0.244 | 0.521 | 0.335–0.808 | 0.004 | |||
50–54 | 1.388 | 0.925–2.085 | 0.114 | 0.682 | 0.439–1.057 | 0.087 | |||
55–59 | 1.276 | 0.831–1.959 | 0.266 | 0.617 | 0.388–0.982 | 0.042 | |||
60–64 | 1.419 | 0.928–2.170 | 0.106 | 0.66 | 0.342–1.272 | 0.214 | |||
65–74 | 1.571 | 1.043–2.367 | 0.031 | 0.752 | 0.438–1.289 | 0.3 | |||
≥75 | 2.946 | 1.945–4.464 | <0.001 | 0.803 | 0.507–1.274 | 0.352 | |||
Race | 0.519 | 0.009 | |||||||
Black | 1 | Reference | 1 | Reference | |||||
White | 0.778 | 0.641–0.944 | 0.011 | 0.946 | 0.768–1.165 | 0.602 | |||
Other | 0.772 | 0.579–1.028 | 0.077 | 1.094 | 0.804–1.488 | 0.569 | |||
Grade | 0.564 | 0.01 | |||||||
Grade I | 1 | Reference | 1 | Reference | |||||
Grade II | 2.175 | 1.424–3.323 | <0.001 | 0.916 | 0.582–1.443 | 0.706 | |||
Grade III | 3.055 | 2.004–4.656 | <0.001 | 0.939 | 0.598–1.476 | 0.786 | |||
Grade IV | 3.191 | 1.555–6.546 | 0.001 | 1.795 | 0.830–3.881 | 0.137 | |||
Stage | 0.773 | 0.008 | |||||||
IA | 1 | Reference | 1 | Reference | |||||
IB | 3.517 | 1.830–6.756 | <0.001 | 1.244 | 0.138–11.167 | 0.846 | |||
IIA | 11.004 | 5.410–22.384 | <0.001 | 1.488 | 0.159–13.959 | 0.728 | |||
IIB | 7.63 | 3.903–14.915 | <0.001 | 1.565 | 0.173–14.135 | 0.69 | |||
III | 15.042 | 8.005–28.265 | <0.001 | 1.401 | 0.157–12.466 | 0.763 | |||
IV | 46.171 | 24.497–87.024 | <0.001 | 1.871 | 0.196–17.893 | 0.587 | |||
Stag_T | 0.745 | 0.009 | |||||||
T1a | 1 | Reference | 1 | Reference | |||||
T1b | 4.348 | 2.418–7.816 | <0.001 | 0.9 | 0.115–7.039 | 0.92 | |||
T2a | 10.273 | 5.541–19.046 | <0.001 | 0.84 | 0.108–6.517 | 0.868 | |||
T2b | 10.103 | 5.603–18.214 | <0.001 | 0.845 | 0.070–10.214 | 0.894 | |||
T3a | 26.967 | 14.466–50.269 | <0.001 | 1.314 | 0.162–10.672 | 0.798 | |||
T3b | 24.197 | 13.511–43.337 | <0.001 | 1.31 | 0.161–10.622 | 0.801 | |||
T4 | 39.286 | 21.227–72.709 | <0.001 | 1.407 | 0.166–11.944 | 0.754 | |||
Stag_N | 0.629 | 0.01 | |||||||
N0 | 1 | Reference | 1 | Reference | |||||
N1 | 2.866 | 2.470–3.325 | <0.001 | 1.16 | 0.934–1.441 | 0.178 | |||
stag_M | 0.628 | 0.009 | |||||||
M0 | 1 | Reference | 1 | Reference | |||||
M1 | 6.349 | 5.362–7.518 | <0.001 | 1.332 | 0.434–4.092 | 0.617 | |||
rx_site | 0.712 | 0.008 | |||||||
0 | 1 | Reference | 1 | Reference | |||||
10–19 | 0.226 | 0.056–0.907 | 0.036 | 0.764 | 0.176–3.320 | 0.719 | |||
20–29 | 0.273 | 0.198–0.375 | <0.001 | 1.037 | 0.735–1.462 | 0.838 | |||
30–39 | 0.078 | 0.037–0.164 | <0.001 | 0.809 | 0.366–1.791 | 0.602 | |||
40–49 | 0.178 | 0.129–0.246 | <0.001 | 0.801 | 0.563–1.140 | 0.218 | |||
50–59 | 0.208 | 0.166–0.262 | <0.001 | 0.69 | 0.531–0.898 | 0.006 | |||
60–64 | 0.276 | 0.170–0.447 | <0.001 | 0.861 | 0.512–1.448 | 0.573 | |||
65–75 | 1.753 | 0.784–3.922 | 0.172 | 1.226 | 0.505–2.977 | 0.652 | |||
rx_reg | 0.507 | 0.003 | |||||||
None | 1 | Reference | |||||||
Other* | 0.77 | 0.512–1.156 | 0.207 | ||||||
Size | 0.731 | 0.009 | |||||||
≤30 | 1 | Reference | 1 | Reference | |||||
>30, ≤50 | 3.754 | 2.908–4.846 | <0.001 | 1.081 | 0.814–1.436 | 0.59 | |||
>50, ≤100 | 7.811 | 6.187–9.862 | <0.001 | 1.535 | 1.162–2.027 | 0.002 | |||
>100 | 16.9 | 11.786–24.233 | <0.001 | 1.678 | 1.102–2.556 | 0.016 | |||
Extension | 0.749 | 0.009 | |||||||
<200 | 1 | Reference | 1 | Reference | |||||
≥200, <300 | 4.292 | 2.322–7.934 | <0.001 | 1.364 | 0.074–25.198 | 0.835 | |||
≥300, <500 | 10.231 | 5.464–19.157 | <0.001 | 1.936 | 0.108–34.725 | 0.654 | |||
≥500, <600 | 10.907 | 5.907–20.140 | <0.001 | 1.375 | 0.053–35.469 | 0.848 | |||
≥600, <700 | 26.619 | 14.554–48.683 | <0.001 | NA | NA | NA | |||
≥700, <999 | 42.235 | 22.297–80.002 | <0.001 | NA | NA | NA | |||
LN | 0.629 | 0.01 | |||||||
No involvement of lymph nodes | 1 | Reference | 1 | Reference | |||||
Lymphoid involvement | 2.866 | 2.470–3.325 | <0.001 | NA | NA | NA | |||
Mets_dx | 0.63 | 0.009 | |||||||
0 | 1 | Reference | 1 | Reference | |||||
1–99 | 6.329 | 5.351–7.486 | <0.001 | 1.077 | 0.388–2.986 | 0.887 | |||
Canc_dth | 0.871 | 0.006 | |||||||
Alive or dead of other cause | 1 | Reference | 1 | Reference | |||||
Dead | 64.745 | 51.300–81.720 | <0.001 | 3.39E+09 | 0.000–Inf | 0.979 | |||
Oth_dth | 0.549 | 0.006 | |||||||
Alive or dead due to cancer | 1 | Reference | 1 | Reference | |||||
Dead of others | 6.509 | 5.220–8.117 | <0.001 | 3.38E+09 | 0.000–Inf | 0.979 | |||
Seq_num | 0.498 | 0.004 | |||||||
One primary only | 1 | Reference | |||||||
1st of 2 or more primaries | 1.255 | 0.922–1.709 | 0.149 | ||||||
Total_malig | 0.503 | 0.004 | |||||||
1 | 1 | Reference | 1 | Reference | |||||
2 | 1.428 | 1.038–1.965 | 0.029 | 0.583 | 0.408–0.832 | 0.003 | |||
3 | 0.881 | 0.220–3.531 | 0.858 | 0.189 | 0.042–0.856 | 0.031 | |||
4 | <0.001 | 0.000–Inf | 0.988 | 0.777 | 0.000–Inf | 1 | |||
Total_begn | 0.502 | 0.002 | |||||||
0 | 1 | Reference | |||||||
1 | 2.684 | 0.863–8.344 | 0.088 | ||||||
Age_diag | 0.588 | 0.012 | |||||||
20–34 | 1 | Reference | 1 | Reference | |||||
35–39 | 0.664 | 0.464–0.950 | 0.025 | NA | NA | NA | |||
40–44 | 1.041 | 0.772–1.404 | 0.793 | NA | NA | NA | |||
45–49 | 1.282 | 0.959–1.714 | 0.094 | NA | NA | NA | |||
50–54 | 1.397 | 1.046–1.867 | 0.024 | NA | NA | NA | |||
55–59 | 1.284 | 0.932–1.769 | 0.126 | NA | NA | NA | |||
60–69 | 1.486 | 1.132–1.950 | 0.004 | 1.001 | 0.629–1.593 | 0.997 | |||
70–99 | 2.387 | 1.808–3.153 | <0.001 | NA | NA | NA | |||
Mrit | 0.556 | 0.011 | |||||||
Single | 1 | Reference | 1 | Reference | |||||
Married or partner | 0.702 | 0.590–0.835 | <0.001 | 0.878 | 0.727–1.060 | 0.176 | |||
Separated divorced or widowed | 1.084 | 0.899–1.306 | 0.399 | 1.057 | 0.858–1.304 | 0.601 |
*, it is include non-primary surgical procedure performed, non-primary surgical procedure to other regional sites, non-primary surgical procedure to distant lymph node(s), non-primary surgical procedure to distant site and any combination of surgical procedure to other regional, distant lymph node, and/or distant site (combination of codes 2, 3, or 4). inf, infinite; NA, not application.
Acknowledgments
Funding: This work was supported partly by
Footnote
Conflicts of Interest: All authors have completed the ICMJE uniform disclosure form (available at http://dx.doi.org/10.21037/tcr.2020.02.71). The authors have no conflicts of interest to declare.
Ethical Statement: The authors are accountable for all aspects of the work in ensuring that questions related to the accuracy or integrity of any part of the work are appropriately investigated and resolved. The study was conducted in accordance with the Declaration of Helsinki (as revised in 2013). Institutional ethical approval and informed consent were waived.
Open Access Statement: This is an Open Access article distributed in accordance with the Creative Commons Attribution-NonCommercial-NoDerivs 4.0 International License (CC BY-NC-ND 4.0), which permits the non-commercial replication and distribution of the article with the strict proviso that no changes or edits are made and the original work is properly cited (including links to both the formal publication through the relevant DOI and the license). See: https://creativecommons.org/licenses/by-nc-nd/4.0/.
References
- Yang H, Ye S, Goswami S, et al. Highly immunosuppressive HLADR(hi) regulatory T cells are associated with unfavorable outcomes in cervical squamous cell carcinoma. Int J Cancer 2020;146:1993-2006. [Crossref] [PubMed]
- Bray F, Ferlay J, Soerjomataram I, et al. Global cancer statistics 2018: GLOBOCAN estimates of incidence and mortality worldwide for 36 cancers in 185 countries. CA Cancer J Clin 2018;68:394-424. [Crossref] [PubMed]
- Mpunga T, Chantal Umulisa M, et al. Human papillomavirus genotypes in cervical and other HPV-related anogenital cancer in Rwanda, according to HIV status. Int J Cancer 2020;146:1514-22. [Crossref] [PubMed]
- Wu SG, Zhang WW, Sun JY, et al. Multimodal treatment including hysterectomy improves survival in patients with locally advanced cervical cancer: A population-based, propensity score-matched analysis. Int J Surg 2017;48:122-7. [Crossref] [PubMed]
- Song L, Liu S, Yao H, et al. MiR-362-3p is downregulated by promoter methylation and independently predicts shorter OS of cervical squamous cell carcinoma. Biomed Pharmacother 2019;115:108944. [Crossref] [PubMed]
- Landoni F, Maneo A, Colombo A, et al. Randomised study of radical surgery versus radiotherapy for stage Ib-IIa cervical cancer. Lancet 1997;350:535-40. [Crossref] [PubMed]
- Zhu X, Luo H, Xu Y. Transcriptome analysis reveals an important candidate gene involved in both nodal metastasis and prognosis in lung adenocarcinoma. Cell Biosci 2019;9:92. [Crossref] [PubMed]
- Kawai K, Sunami E, Yamaguchi H, et al. Nomograms for colorectal cancer: A systematic review. World J Gastroenterol 2015;21:11877-86. [Crossref] [PubMed]
- Quinn BA, Deng X, Colton A, et al. Increasing age predicts poor cervical cancer prognosis with subsequent effect on treatment and overall survival. Brachytherapy 2019;18:29-37. [Crossref] [PubMed]
- Lizcano F, Guzman G. Estrogen Deficiency and the Origin of Obesity during Menopause. Biomed Res Int 2014;2014:757461.
- Moodley M, Moodley J, Chetty R, et al. The role of steroid contraceptive hormones in the pathogenesis of invasive cervical cancer: a review. Int J Gynecol Cancer 2003;13:103-10. [Crossref] [PubMed]
- Potish RA, Twiggs LB, Adcock LL, et al. Prognostic importance of progesterone and estrogen receptors in cancer of the uterine cervix. Cancer 1986;58:1709-13. [Crossref] [PubMed]
- Riera-Leal A, Ramirez De Arellano A, Ramirez-Lopez IG, et al. Effects of 60 kDa prolactin and estradiol on metabolism and cell survival in cervical cancer: Coexpression of their hormonal receptors during cancer progression. Oncol Rep 2018;40:3781-93. [PubMed]
- Chung SH, Shin MK, Korach KS, et al. Requirement for stromal estrogen receptor alpha in cervical neoplasia. Horm Cancer 2013;4:50-9. [Crossref] [PubMed]
- Fontecha N, Basaras M, Hernaez S, et al. Assessment of human papillomavirus E6/E7 oncogene expression as cervical disease biomarker. BMC Cancer 2016;16:852. [Crossref] [PubMed]
- International Collaboration of Epidemiological Studies of Cervical Cancer. Cervical cancer and hormonal contraceptives: collaborative reanalysis of individual data for 16,573 women with cervical cancer and 35,509 women without cervical cancer from 24 epidemiological studies. Lancet 2007;370:1609-21. [Crossref] [PubMed]
- Lyu J, Sun T, Tan X. Ovarian preservation in young patients with stage I cervical adenocarcinoma: a surveillance, epidemiology, and end results study. Int J Gynecol Cancer 2014;24:1513-20. [Crossref] [PubMed]
- Munguía-Moreno JA, Diaz-Chavez J, Garcia-Villa E, et al. Early synergistic interactions between the HPV16E7 oncoprotein and 17beta-oestradiol for repressing the expression of Granzyme B in a cervical cancer model. Int J Oncol 2018;53:579-91. [PubMed]
- Siristatidis C, Sergentanis TN, Kanavidis P, et al. Controlled ovarian hyperstimulation for IVF: impact on ovarian, endometrial and cervical cancer--a systematic review and meta-analysis. Hum Reprod Update 2013;19:105-23. [Crossref] [PubMed]
- Everhov ÅH, Nyberg T, Bergmark K, et al. Hormone therapy after uterine cervical cancer treatment: a Swedish population-based study. Menopause 2015;22:633-9. [Crossref] [PubMed]
- Hofsjö A, Bohm-Starke N, Bergmark K, et al. Sex steroid hormone receptor expression in the vaginal wall in cervical cancer survivors after radiotherapy. Acta Oncol 2019;58:1107-15. [Crossref] [PubMed]
- Walker JL, Piedmonte MR, Spirtos NM, et al. Recurrence and survival after random assignment to laparoscopy versus laparotomy for comprehensive surgical staging of uterine cancer: Gynecol Oncol Group LAP2 Study. J Clin Oncol 2012;30:695-700. [Crossref] [PubMed]
- Fanfani F, Vizza E, Landoni F, et al. Radical hysterectomy after chemoradiation in FIGO stage III cervical cancer patients versus chemoradiation and brachytherapy: Complications and 3-years survival. Eur J Surg Oncol 2016;42:1519-25. [Crossref] [PubMed]
- Zhou L, Sun CT, Lin L, et al. Independent risk factors for ovarian metastases in stage IA-IIB cervical carcinoma. Acta Obstet Gynecol Scand 2019;98:18-23. [Crossref] [PubMed]
- Matsuo K, Machida H, Shoupe D, et al. Ovarian Conservation and Overall Survival in Young Women With Early-Stage Cervical Cancer. Obstet Gynecol 2017;129:139-51. [Crossref] [PubMed]
- Zhu X, Lin MCM, Fan W, et al. An intronic polymorphism in GRP78 improves chemotherapeutic prediction in non-small cell lung cancer. Chest 2012;141:1466-72. [Crossref] [PubMed]
- Tan S, Li D, Zhu X. Cancer immunotherapy: Pros, cons and beyond. Biomed Pharmacother 2020;124:109821. [Crossref] [PubMed]
- Aarts JW, Nieboer TE, Johnson N, et al. Surgical approach to hysterectomy for benign gynaecological disease. Cochrane Database Syst Rev 2015;CD003677. [PubMed]
- Melamed A, Margul DJ, Chen L, et al. Survival after Minimally Invasive Radical Hysterectomy for Early-Stage Cervical Cancer. N Engl J Med 2018;379:1905-14. [Crossref] [PubMed]
- Tang Z, Li D, Hou S, et al. The cancer exosomes: Clinical implications, applications and challenges. Int J Cancer 2019; [Epub ahead of print]. [Crossref] [PubMed]
- Eifel PJ, Levenback C, Wharton JT, et al. Time course and incidence of late complications in patients treated with radiation therapy for FIGO stage IB carcinoma of the uterine cervix. Int J Radiat Oncol Biol Phys 1995;32:1289-300. [Crossref] [PubMed]
- Huang XB, Zhang WW, Wu SG, et al. Survival benefits with the addition of adjuvant hysterectomy to radiochemotherapy for treatment of stage I-II adenocarcinoma of the uterine cervix. J Surg Oncol 2018;118:574-80. [PubMed]
- Carcopino X, Houvenaeghel G, Buttarelli M, et al. Equivalent survival in patients with advanced stage IB-II and III-IVA cervical cancer treated by adjuvant surgery following chemoradiotherapy. Eur J Surg Oncol 2008;34:569-575. [Crossref] [PubMed]
- Tseng JH, Aloisi A, Sonoda Y, et al. Long-Term Oncologic Outcomes of Uterine-Preserving Surgery in Young Women With Stage Ib1 Cervical Cancer. Int J Gynecol Cancer 2018;28:1350-9. [Crossref] [PubMed]
- Wagner AE, Pappas L, Ghia AJ, et al. Impact of tumor size on survival in cancer of the cervix and validation of stage IIA1 and IIA2 subdivisions. Gynecol Oncol 2013;129:517-21. [Crossref] [PubMed]
- Trattner M, Graf AH, Lax S, et al. Prognostic factors in surgically treated stage ib-iib cervical carcinomas with special emphasis on the importance of tumor volume. Gynecol Oncol 2001;82:11-16. [Crossref] [PubMed]
- Liu J, Li D, Luo H, et al. Circular RNAs: The star molecules in cancer. Mol Aspects Med 2019;70:141-52. [Crossref] [PubMed]
- Zhou J, Wu SG, Sun JY, et al. The effect of local treatment modalities in patients with early-stage adenocarcinoma of the uterine cervix: A population-based analysis. Int J Surg 2017;41:16-22. [Crossref] [PubMed]