Data independent acquisition-mass spectrometry (DIA-MS)-based comprehensive profiling of bone metastatic cancers revealed molecular fingerprints to assist clinical classifications for bone metastasis of unknown primary (BMUP)
Introduction
Cancer of unknown primary (CUP) is a heterogeneous metastatic carcinoma in the absence of an identifiable primary neoplasm. It is estimated that about 15% of all cancer patients were diagnosed for the first time due to the clinical metastatic syndromes. The primary tumor for the majority of these patients could be determined after standardized diagnostic work-ups including a thorough clinical history, physical examination, biopsies, and imaging studies (CT, MRI, PET) (1,2). However, still one third of all metastatic cancers with tumor origins remain elusive, which account for 3–10% of total cancer diagnoses worldwide (3-7). The peak incidence was at around 70 years of age and was slightly higher in males than in females (8-10). Although incidence has been declining since 1990s attributed to the diagnostic advances, CUP still remains the fifth leading cause of cancer-related death worldwide (3,11,12). Due to lack of indication of tumor origins, the treatment was largely empirical by utilizing taxane or platinum-based agents, which is usually associated with bad responses and resulted in poor prognosis (13-15). For this group, the median overall survival is only 6–9 months and less than 30% of 1-year overall survival (7,16).
Bone is the third most frequent site where metastases from primary tumor could develop after lung and liver (17-19). Over the past decades, average survival of patients with bone metastasis from lung cancer was dishearteningly 4–8 months (20,21). Treatment decisions are currently based on histology classifications of cancer primary. However, among patients with bone metastasis, 20–30% still have no indication of the primary tumor after comprehensive examinations (22,23). The etiology of this disease remains unknown. For these patients in routine clinical practice, the main strategy for identifying the primary tumor was tissue-of-origin (TOO) studies using histochemistry and immunohistochemistry (IHC), which were considered as standard tests to suggest the origin of the lesion. Tissue-specific protein markers such as cytokeratins (CKs) including CK7 (KRT7) and CK20 (KRT20), homeobox protein Nkx-2.1 (TTF1), mammaglobin-A, and homeobox protein CDX-2 have been widely applied to predict primary sites (2,24-29). These markers were mainly used as a panel, the choice of which greatly affected subsequent diagnosis (27,30-33). Meanwhile, the IHC evaluations of cases were performed mostly on formalin-fixed paraffin-embedded (FFPE) tissue sections, the specificity, sensitivity, and multiplexing capability of which are debated.
As molecular profiling was introduced as a new tool to identify the primary TOO for CUP patients, the expression profiles of larger set of genes in different tissue types were easily interpreted in a quantitative manner (34-38). Several studies to identify the primary sites for CUP patients have been carried our using RT-PCR or microarray techniques (39-41). The genetic mutational landscape of CUP patients was deconvoluted, which revealed a series of driver mutations and their roles for targeted therapy (42-44). Owing to technology advances, mass spectrometers with high resolution and sensitivity has become the method of choice for multiplexed and quantitative analysis of proteins and proteomes. Recently, mass spectrometry-based proteomics had showed pivotal roles for elucidation of disease mechanism as well as the discovery and validation of protein biomarkers from clinical samples (45-48). Data independent acquisition mass spectrometry (DIA-MS) using sequential windows for acquisition of all theoretical fragment ion spectra, has shown great value for the analysis of limited and nonrenewable clinical specimen (49-51). By generating a permanent “digital map” of the sample proteome with high reproducibility, the data can be analyzed, re-analyzed and mined in silico to identify and quantify thousands of proteins across multiple samples. In the present study, we generated the proteome signature by DIA-MS for individual patient who was firstly diagnosed with bone metastatic cancer, aiming for deepening our insight into the molecular fingerprints of bone metastatic cancers which will be of significance for guiding TOO elucidation and prognosis management.
Methods
Patient cohort
Informed consent forms were received from all patients included in this study, and all experimental work in this study was approved by the Review Board and Ethical Committee of Shanghai Changzheng Hospital, affiliated to The Second Military Medical University.
A total number of eighteen freshly frozen tissue samples were obtained from Shanghai Changzheng Hospital Tissue BioBank, encompassing bone metastatic carcinoma tissues with primary sites from lung cancer, prostate cancer, and liver cancer. The disease tissues were from patients that were firstly diagnosed with bone metastatic cancers and underwent surgery without any pre radio- or chemotherapy. The primary tumor site was identified after comprehensive examination including advanced imaging techniques and IHC analyses.
Tissue homogenization and protein extraction
After carefully review of all the sample information, the obtained bone metastatic tissue blocks were homogenized and lysis buffer was added into each sample. The lysis buffer contains 0.2% acid labile surfactant (ALS) in 20 mM HEPES buffer with 1× protease inhibitor (Roche, Basel, Switzerland). All the sample were homogenized in liquid nitrogen. The homogenates were then incubated for 30 minutes on ice. After that, the lysates were spin down at 20,000 g for 30 min at 4 °C. All the protein concentrations were determined by standard BCA assay.
Protein digestion and peptide purification
Urea (6M) was used to denature the proteins at room temperature for 1h. Then the proteins were reduced with 5 mM tris (2-carboxyethyl)phosphine (TCEP) and incubated at room temperature for 0.5 h. Iodoacetamide (IAA, 6.25 mM) was added to alkylate the protein for 0.5 h at room temperature at dark. The mixture was then diluted with 6 volumes of 50 mM ammonium bicarbonate buffer and digested using sequence modified trypsin (Promega, Madison, WI, USA) at an enzyme-to-substrate ratio of 1:100 (w/w), for 12 hours at 37 °C. Each digested protein solution was quenched with 1 uL phosphoric acid and the pH was adjusted to 2. Then the acidified mixture was loaded onto a 96-well C-18 cartridge (ThermoFisher, USA) and washed 3 times with 0.1% formic acid. After that, the desalted peptides were eluted with 50% ACN and dried under vacuum.
Liquid chromatography tandem mass spectrometry (LC-MS/MS) analysis
The dried peptides were dissolved in 0.1% formic acid (0.5 µg/µL). iRT kit (Biognosys, Switzerland) was added according to manufacturer’s instruction prior to LC-MS/MS analysis. LC-ESI-MS/MS was performed by coupling a nanoLC (Dionex Ultimate 3000, ThermoFisher Scientific) to an Orbitrap Fusion mass spectrometer (ThermoFisher Scientific). For each analysis, 2 µL of dissolved peptides was delivered to an analytical column (Dikma, inspire C18, 3 µm, Canada, 150 mm × 75 µm, self-packed) and separated using a 62 min gradient from 7% to 35% of solvent B (0.1% formic acid in acetonitrile) at 300 nL/minute flow rate.
For spectral library generation, an Orbitrap Fusion mass spectrometer was operated in data dependent acquisition (DDA) mode, automatically switching between MS and MS/MS. Full scan MS spectra (350–1,550 m/z) were acquired in the Orbitrap at 60,000 resolution (at m/z 400) after accumulation precursor ions to a target value of 200,000 for a maximum time of 100 ms. Internal lock mass calibration was enabled using the ion signal (Si(CH3)2O)6 H+ at m/z 445.120025 present in ambient laboratory air. Tandem mass spectra were recorded for maximum 3 seconds by higher energy collision induced dissociation (HCD, target value of 10,000, max 35 ms accumulation time) at a normalized collision energy of 30% in the orbitrap. To maximize the number of precursors targeted for analysis, dynamic exclusion was enabled with one repeat count in 60 s exclusion time.
DIA analysis was performed with the same mass spectrometer and LC system as DDA. The same analytical column, same gradient, same flow rate and buffers were used. The mass spectrometer was operated in t-MS2 mode. The duty cycle started from a high-resolution MS1 scan event acquired at a resolution of 60,000 and followed by 30 DIA scans with 21 m/z isolation windows (1 m/z overlapped between each window) at 30,000 resolution covering the precursor mass range from m/z 400 to 1,000 over the entire chromatographic elution profile. The rest of the acquisition parameters remained identical to the DDA analyses.
Spectral library construction and data analysis
The spectral library was built up by combination of 10 DDA files (acquired from different tissue subtypes), the peaks lists from these files were picked from raw MS data files and were then searched against Uniprot Human Protein Database (2016.09.16) using Proteome Discoverer version 1.4 (Thermo Fisher Scientific). The search was performed considering carbamidomethylation of cysteine residues as fixed modification and methionine oxidation as variable modifications. Trypsin was specified as the proteolytic enzyme and up to two missed cleavages were allowed. The mass tolerances were set to 10 ppm for the precursor ions and 0.02 Da for the fragments. All peptides were filtered at high confidence level. The protein false discovery rate, which was determined by a target-decoy search strategy, was set to 1%. The spectral library was generated from DDA data using Spectronaut (Biognosys, Switzerland) with a FDR cutoff set to 0.01. The assay fragments were restricted from 3 to 6, and fragments were selected from m/z 300–1800. All DIA raw data files were imported in Spectronaut after being converted in HTRMS Converter (Biognosys, Switzerland). Peptide fragment ion peak areas were extracted by searching against above-mentioned library. Retention time prediction type was set to dynamic iRT and correction factor of 1. Cross run normalization was enabled for systematic variance correction of the LC-MS performance and local normalization strategy was used based on the assumption that although a small fraction of peptides will be up or down regulated, the total quantity of all the peptides within the sample will remain equal across runs and along retention time due to the equal amount of protein sampling guided by BCA assay. All results were filtered by a Q value cutoff of 0.01 (corresponds to a FDR of 1%). Peptide intensity was calculated by summing the peak areas of their respective fragment ions for MS2 and protein intensity was calculated by summing the intensities of their respective peptides. Significance analysis of protein abundance changes was calculated using two-side t-test. Further data interpretation and functional annotation were performed using DAVID, v6.8, Ingenuity Pathway Analysis (IPA), R and online tool powered by R language (http://www.omicsolution.org/wu-kong-beta-linux/main/).
Results
Overview of patient proteomic profiles
As described in Figure 1, we collected three most common bone metastatic cancer tissues from each patient in the population (n=18), with primary tumors located in lung (n=7), prostate (n=3), and liver (n=8).
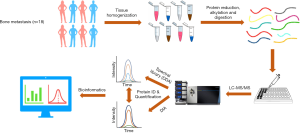
Our proteomic workflow followed the general sample preparation procedure, via tissue homogenization in liquid nitrogen, protein alkylation and digestion. Each fresh-frozen specimen was homogenized and lysed using a simplified and highly efficient “precipitation-free” protein extraction protocol as described before. Detergent-free, ALS were utilized in the lysis buffer, since ALS can degrade easily under acidic conditions which is perfect to be coupled to proteomics pipelines. Resulted peptides were purified via 96-well C18 plates and delivered to a nanoLC coupled with high resolution mass spectrometer (Orbitrap Fusion). Three samples from the cohort (from each primary location category) were used for data independent acquisition (DDA) to establish a comprehensive spectral library. All samples were subjected to LC-MS/MS individually for generation of a quantitative “proteome map” for each single patient via data independent acquisition mass spectrometry (DIA-MS). The raw data were processed and quantified proteins were further analyzed with bioinformatics tools.
The samples were divided into 3 subgroups according to their diagnostic tumor primary: 7 with primary lung cancer (BM_Lung), 3 with primary prostate cancer (BM_Prostate) and 8 with primary prostate cancer (BM_Liver). After protein quantification and data normalization (see methods), more than 6000 proteins were quantified (Figure 2A) from 18 DIA-MS runs at q-value less than 0.01, each in a 62-minute elution gradient. The majority of identified proteins were shared among different cancer tissues. With 527 proteins exclusively identified in patients with bone metastases from liver, this group showed the highest number of proteins identified in their proteomic profiles. There are 741 proteins that were found in patients with bone metastases from liver and lung, but not prostate; 675 proteins were shared by patients with bone metastases from liver and prostate, but not lung. Principle component analysis (PCA) on all identified proteins from all samples reveals no obvious batch effects between samples (Figure 2B). Gene ontology (GO) analysis on shared proteins suggested that these proteins mainly located in cytoplasm and extracellular exosomes, and participated in molecular functions such as cell adhesion and oxidation-reduction processes, many of which are vital activities taken place in tumorigenesis and metastasis (Figure 2C).
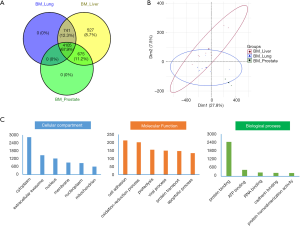
Tissue-specific proteome profiles
Current cancer treatment strategies were mainly based on histological classifications of cancer tissues. Therefore, treatment options for CUP patients are very much dependent on TOOs studies. Some reported molecular classifiers from gene/mRNA expression studies are able to predict primary of cancer tissues, but to the best of our knowledge, there are no systematic investigation on proteomic signatures of bone metastatic cancers. To uncover potential tissue-specific proteome profiles, we further applied statistical analysis (student’s t-test, double sided, P<0.01) on their expression profiles of all proteins, shortlisted protein candidates for each origin category (BM_Lung/Prostate/Liver) and annotated their roles in terms of cellular location, biological processes, and molecular functions (Figure 3). Most quantified proteins were from cytosol or cytoplasm, for BM_Lung/Prostate groups, considerable proteins were also identified from nucleus/nucleoplasm, indicating potential active transcriptional regulations in the cancer cells of these tissues. As expected, the top enriched biological process for BM_liver group is oxidation-reduction process. While for BM_liver/prostate groups, transcription regulation and cell division are the top two enriched processes. Most of the tissue-specific proteins were found to be binding proteins in terms of molecular function, to interact with metal ions (Zinc), kinases, ATPs, RNAs, chromatins and so on.
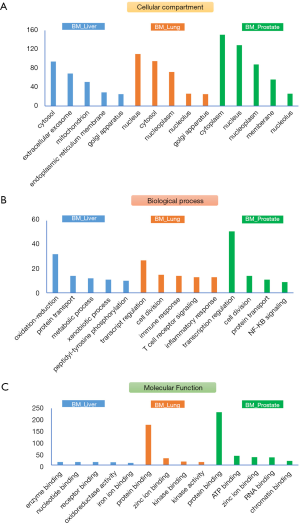
Protein marker panel to differentiate bone metastasis from lung, prostate and liver cancers
Systematic analyses of known primaries provide tumor-specific gene signatures for classifying cancers which were reported in many studies with high accuracy (52-54). Many of these gene classifiers have been applied successfully to assist TOO prediction and diagnosis. Based on the aforementioned tissue-specific proteome signature proteins, we further probed the possibility to generate a protein marker panel to differentiate the three metastatic cancer groups (BM_Liver/Prostate/Lung). Analysis of variance (ANOVA) and orthogonal partial least square-discriminant analysis (OPLS-DA) was applied which led to 30 proteins with significance (P<0.01, Figure 4A). PCA clearly showed that each group represented a distinct cluster from the rest groups on PC1 or PC2 dimension, indicating the obvious variations of marker protein expressions among three groups (Figure 4B). Heat map using 30 significant proteins revealed details on protein abundance among three groups (normalized abundance, Figure 4C). Unsupervised clustering also gave acceptable grouping results, showing four subgroups representing BM_Liver (purple), BM_Liver/Prostate (cyan), BM_Prostate/Lung (green), and BM_Lung (red) (Figure 4D). There is a high likelihood that interference from prostate samples gave rise to the internal split of both BM_Lung and BM_Liver groups, which resulted in 4 individual subgroups. Network analysis showed alcohol dehydrogenase (ADH) and aldehyde dehydrogenase (ALDH) family members are significant molecules in aberrant metabolic pathways across many cancers as reported (Figure 4E) (55,56).
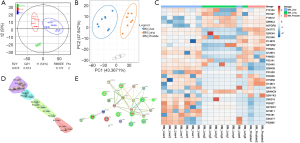
To further narrow down the candidate list to obtain a more practical panel for clinical applications, we applied regression analysis and assess the performance of these classifiers by plotting their receiver operating characteristics (ROC) curves (Figure 5A). Four protein classifiers, RFIP1, KRT15, ESYT2 and MAL2, were finalized to distinguish bone metastasis from liver and lung cancer (prostate samples were excluded due to small sample size). These markers displayed very good sensitivity and specificity to differentiate two tumor primaries, with areas under the curves (AUC) higher than 0.8 (Figure 5B). Their expression patterns across different primaries were plotted in Figure 5C. CK family members (KRT7 and KRT20) were existing tumor markers for IHC analysis in clinically practice. In this study, another CK family member KRT15 (CK15) has achieved the highest AUC of 0.93, indicating the strong capability of this molecule to distinguish tumor origins from lung and liver.
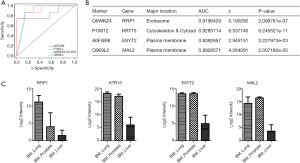
Discussion
Bone metastatic cancers showed highly heterogeneous morphology and clinical outcomes. The rarity of this tumor around the globe has resulted in poor understanding of the tumor biology and etiology. This study carried out proteomic profiling of individual bone metastatic cancer patients, using 18 fresh frozen samples across different cancer primaries, including lung, prostate and liver. To the best of our knowledge, this is the first comprehensive proteomic study with identification of over 6,000 proteins, which were associated with a vast area of cellular activities such as inflammation and cell adhesion. It is exciting that two plasma membrane proteins, ESYT2 and MAL2, were found with good differentiating performance, which could act as cell surface markers to facilitate further research on bone metastatic cancer primary studies and the many further uses that can be envisaged.
The more comprehensive picture of the proteomic profile of bone metastases will complement available genomic and transcriptomic data, in the meantime enable navigation of patient proteomes, which will deepen our understanding concerning cancer metastasis development and progression. The generated digital, re-minable “proteome map” by next generation data independent acquisition mass spectrometry for each individual sample provided fundamental information to characterize the landscape of the proteome variations for bone metastatic cancers, which will provide biological insight and hold considerable promise to accelerate the development of novel markers for precise diagnosis of bone metastasis of unknown primary (BMUP).
Further experiments concerning extending the scope of bone metastatic cancer primaries including breast cancers, gastrointestinal cancers, kidney cancers as well as thyroid cancers are currently ongoing. With more upcoming proteomics data acquired from bone metastatic patient samples in the future, we could conduct big data analysis eventually, which will deepen our insight into the molecular fingerprints of BMUPs and guide the therapeutic and prognostic management.
Conclusions
We characterized proteome landscape for individual bone metastasis patient tissues. Compared to previous proteomic studies, it is the first time that proteome signatures of fresh-frozen samples with more than 6,000 newly identified proteins which covered various cellular locations and biological processes. Systematic analysis of patient proteome revealed four novel and significant protein classifiers, two of which were cell surface proteins. Furthermore, the permanent proteome map generated in this study by DIA-MS allows future re-analysis and re-mining of data in silico as our knowledge advances, which is extremely practical for precious and nonrenewable clinical specimen, to assist investigation of bone metastasis and progression of tumors as well as the development of diagnostic tools and prognosis management for BMUPs.
Acknowledgments
Funding: This work is supported by
Footnote
Conflicts of Interest: All authors have completed the ICMJE uniform disclosure form (available at http://dx.doi.org/10.21037/tcr.2020.03.41). The authors have no conflicts of interest to declare.
Ethical Statement: The authors are accountable for all aspects of the work in ensuring that questions related to the accuracy or integrity of any part of the work are appropriately investigated and resolved. Informed consent forms were received from all patients included in this study, and all experimental work in this study was approved by the Review Board and Ethical Committee of Shanghai Changzheng Hospital, affiliated to The Second Military Medical University. The study was conducted in accordance with the Declaration of Helsinki (as revised in 2013).
Open Access Statement: This is an Open Access article distributed in accordance with the Creative Commons Attribution-NonCommercial-NoDerivs 4.0 International License (CC BY-NC-ND 4.0), which permits the non-commercial replication and distribution of the article with the strict proviso that no changes or edits are made and the original work is properly cited (including links to both the formal publication through the relevant DOI and the license). See: https://creativecommons.org/licenses/by-nc-nd/4.0/.
References
- Oien KA, Dennis JL. Diagnostic work-up of carcinoma of unknown primary: from immunohistochemistry to molecular profiling. Ann Oncol 2012;23:x271-7. [Crossref] [PubMed]
- Greco FA, Oien K, Erlander M, et al. Cancer of unknown primary: progress in the search for improved and rapid diagnosis leading toward superior patient outcomes. Ann Oncol 2012;23:298-304. [Crossref] [PubMed]
- Varadhachary GR, Raber MN. Cancer of unknown primary site. N Engl J Med 2014;371:757-65. [Crossref] [PubMed]
- Stewart JF, Tattersall MH, Woods RL, et al. Unknown primary adenocarcinoma: incidence of over investigation and natural history. Br Med J 1979;1:1530-3. [Crossref] [PubMed]
- van de Wouw AJ, Janssen-Heijnen ML, Coebergh JW, et al. Epidemiology of unknown primary tumours; incidence and population-based survival of 1285 patients in Southeast Netherlands, 1984-1992. Eur J Cancer 2002;38:409-13. [Crossref] [PubMed]
- Moran S, Martinez-Cardus A, Sayols S, et al. Epigenetic profiling to classify cancer of unknown primary: a multicentre, retrospective analysis. Lancet Oncol 2016;17:1386-95. [Crossref] [PubMed]
- Moran S, Martinez-Cardus A, Boussios S, et al. Precision medicine based on epigenomics: the paradigm of carcinoma of unknown primary. Nat Rev Clin Oncol 2017;14:682-94. [Crossref] [PubMed]
- van de Wouw AJ, Jansen RL, Speel EJ, et al. The unknown biology of the unknown primary tumour: a literature review. Ann Oncol 2003;14:191-6. [Crossref] [PubMed]
- Pavlidis N, Briasoulis E, Hainsworth J, et al. Diagnostic and therapeutic management of cancer of an unknown primary. Eur J Cancer 2003;39:1990-2005. [Crossref] [PubMed]
- Fehri R, Rifi H, Alboueiri A, et al. Carcinoma of unknown primary: retrospective study of 437 patients treated at Salah Azaiez Institute. Tunis Med 2013;91:205-8. [PubMed]
- Pavlidis N, Fizazi K. Cancer of unknown primary (CUP). Crit Rev Oncol Hematol 2005;54:243-50. [Crossref] [PubMed]
- Pavlidis N, Pentheroudakis G. Cancer of unknown primary site. Lancet 2012;379:1428-35. [Crossref] [PubMed]
- Hainsworth JD, Daugaard G, Lesimple T, et al. Paclitaxel/carboplatin with or without belinostat as empiric first-line treatment for patients with carcinoma of unknown primary site: A randomized, phase 2 trial. Cancer 2015;121:1654-61. [Crossref] [PubMed]
- Greco FA. Cancer of unknown primary site: evolving understanding and management of patients. Clin Adv Hematol Oncol 2012;10:518-24. [PubMed]
- Hainsworth JD, Rubin MS, Spigel DR, et al. Molecular gene expression profiling to predict the tissue of origin and direct site-specific therapy in patients with carcinoma of unknown primary site: a prospective trial of the Sarah Cannon research institute. J Clin Oncol 2013;31:217-23. [Crossref] [PubMed]
- Hainsworth JD, Fizazi K. Treatment for patients with unknown primary cancer and favorable prognostic factors. Semin Oncol 2009;36:44-51. [Crossref] [PubMed]
- Vandecandelaere M, Flipo RM, Cortet B, et al. Bone metastases revealing primary tumors. Comparison of two series separated by 30 years. Joint Bone Spine 2004;71:224-9. [Crossref] [PubMed]
- Nottebaert M, Exner GU, von Hochstetter AR, et al. Metastatic bone disease from occult carcinoma: a profile. Int Orthop 1989;13:119-23. [Crossref] [PubMed]
- Cooke KS, Kirpekar M, Abiri MM, et al. US case of the day. Skeletal metastasis from poorly differentiated carcinoma of unknown origin. Radiographics 1997;17:542-4. [Crossref] [PubMed]
- Katagiri H, Takahashi M, Inagaki J, et al. Determining the site of the primary cancer in patients with skeletal metastasis of unknown origin: a retrospective study. Cancer 1999;86:533-7. [Crossref] [PubMed]
- Xu DL, Zhang XT, Wang GH, et al. Clinical features of pathologically confirmed metastatic bone tumors--a report of 390 cases. Ai Zheng 2005;24:1404-7. [PubMed]
- Shih LY, Chen TH, Lo WH. Skeletal metastasis from occult carcinoma. J Surg Oncol 1992;51:109-13. [Crossref] [PubMed]
- Piccioli A, Maccauro G, Spinelli MS, et al. Bone metastases of unknown origin: epidemiology and principles of management. J Orthop Traumatol 2015;16:81-6. [Crossref] [PubMed]
- Briasoulis E, Pavlidis N. Cancer of Unknown Primary Origin. Oncologist 1997;2:142-52. [Crossref] [PubMed]
- Green AC. Cancer of unknown primary: does the key lie in molecular diagnostics? Cytopathology 2015;26:61-3. [Crossref] [PubMed]
- Ariza A, Balana C, Concha A, et al. Update on the diagnosis of cancer of unknown primary (CUP) origin. Clin Transl Oncol 2011;13:434-41. [Crossref] [PubMed]
- Kandalaft PL, Gown AM. Practical Applications in Immunohistochemistry: Carcinomas of Unknown Primary Site. Arch Pathol Lab Med 2016;140:508-23. [Crossref] [PubMed]
- Rubin BP, Skarin AT, Pisick E, et al. Use of cytokeratins 7 and 20 in determining the origin of metastatic carcinoma of unknown primary, with special emphasis on lung cancer. Eur J Cancer Prev 2001;10:77-82. [Crossref] [PubMed]
- Gunia S, Koch S, May M. Is CDX2 immunostaining useful for delineating anorectal from penile/vulvar squamous cancer in the setting of squamous cell carcinoma with clinically unknown primary site presenting with histologically confirmed inguinal lymph node metastasis? J Clin Pathol 2013;66:109-12. [Crossref] [PubMed]
- Rossi G, Nannini N, Costantini M. Morphology and more specific immunohistochemical stains are fundamental prerequisites in detection of unknown primary cancer. J Clin Oncol 2009;27:649-50; author reply 650-2. [Crossref] [PubMed]
- Onofre AS, Pomjanski N, Buckstegge B, et al. Immunocytochemical diagnosis of hepatocellular carcinoma and identification of carcinomas of unknown primary metastatic to the liver on fine-needle aspiration cytologies. Cancer 2007;111:259-68. [Crossref] [PubMed]
- Hashimoto K, Sasajima Y, Ando M, et al. Immunohistochemical profile for unknown primary adenocarcinoma. PLoS One 2012;7:e31181. [Crossref] [PubMed]
- Conner JR, Hornick JL. Metastatic carcinoma of unknown primary: diagnostic approach using immunohistochemistry. Adv Anat Pathol 2015;22:149-67. [Crossref] [PubMed]
- Tothill RW, Kowalczyk A, Rischin D, et al. An expression-based site of origin diagnostic method designed for clinical application to cancer of unknown origin. Cancer Res 2005;65:4031-40. [Crossref] [PubMed]
- Horlings HM, van Laar RK, Kerst JM, et al. Gene expression profiling to identify the histogenetic origin of metastatic adenocarcinomas of unknown primary. J Clin Oncol 2008;26:4435-41. [Crossref] [PubMed]
- Varadhachary GR, Talantov D, Raber MN, et al. Molecular profiling of carcinoma of unknown primary and correlation with clinical evaluation. J Clin Oncol 2008;26:4442-8. [Crossref] [PubMed]
- van Laar RK, Ma XJ, de Jong D, et al. Implementation of a novel microarray-based diagnostic test for cancer of unknown primary. Int J Cancer 2009;125:1390-7. [Crossref] [PubMed]
- Yoon HH, Foster NR, Meyers JP, et al. Gene expression profiling identifies responsive patients with cancer of unknown primary treated with carboplatin, paclitaxel, and everolimus: NCCTG N0871 (alliance). Ann Oncol 2016;27:339-44. [Crossref] [PubMed]
- Talantov D, Baden J, Jatkoe T, et al. A quantitative reverse transcriptase-polymerase chain reaction assay to identify metastatic carcinoma tissue of origin. J Mol Diagn 2006;8:320-9. [Crossref] [PubMed]
- Ma XJ, Patel R, Wang X, et al. Molecular classification of human cancers using a 92-gene real-time quantitative polymerase chain reaction assay. Arch Pathol Lab Med 2006;130:465-73. [PubMed]
- Rosenfeld N, Aharonov R, Meiri E, et al. MicroRNAs accurately identify cancer tissue origin. Nat Biotechnol 2008;26:462-9. [Crossref] [PubMed]
- Gatalica Z, Millis SZ, Vranic S, et al. Comprehensive tumor profiling identifies numerous biomarkers of drug response in cancers of unknown primary site: analysis of 1806 cases. Oncotarget 2014;5:12440-7. [Crossref] [PubMed]
- Subbiah IM, Tsimberidou A, Subbiah V, et al. Next generation sequencing of carcinoma of unknown primary reveals novel combinatorial strategies in a heterogeneous mutational landscape. Oncoscience 2017;4:47-56. [PubMed]
- Ross JS, Wang K, Gay L, et al. Comprehensive Genomic Profiling of Carcinoma of Unknown Primary Site: New Routes to Targeted Therapies. JAMA Oncol 2015;1:40-9. [Crossref] [PubMed]
- Schiess R, Wollscheid B, Aebersold R. Targeted proteomic strategy for clinical biomarker discovery. Mol Oncol 2009;3:33-44. [Crossref] [PubMed]
- Guo T, Li L, Zhong Q, et al. Multi-region proteome analysis quantifies spatial heterogeneity of prostate tissue biomarkers. Life Sci Alliance 2018; [Crossref] [PubMed]
- Guo T, Kouvonen P, Koh CC, et al. Rapid mass spectrometric conversion of tissue biopsy samples into permanent quantitative digital proteome maps. Nat Med 2015;21:407-13. [Crossref] [PubMed]
- Zhang H, Liu AY, Loriaux P, et al. Mass spectrometric detection of tissue proteins in plasma. Mol Cell Proteomics 2007;6:64-71. [Crossref] [PubMed]
- Zhao M, Liu X, Sun H, et al. Evaluation of Urinary Proteome Library Generation Methods on Data-Independent Acquisition MS Analysis and its Application in Normal Urinary Proteome Analysis. Proteomics Clin Appl 2019;e1800152. [Crossref] [PubMed]
- Schlotterbeck J, Chatterjee M, Gawaz M, et al. Comprehensive MS/MS profiling by UHPLC-ESI-QTOF-MS/MS using SWATH data-independent acquisition for the study of platelet lipidomes in coronary artery disease. Anal Chim Acta 2019;1046:1-15. [Crossref] [PubMed]
- Ortea I, Rodriguez-Ariza A, Chicano-Galvez E, et al. Discovery of potential protein biomarkers of lung adenocarcinoma in bronchoalveolar lavage fluid by SWATH MS data-independent acquisition and targeted data extraction. J Proteomics 2016;138:106-14. [Crossref] [PubMed]
- Meiri E, Mueller WC, Rosenwald S, et al. A second-generation microRNA-based assay for diagnosing tumor tissue origin. Oncologist 2012;17:801-12. [Crossref] [PubMed]
- Kerr SE, Schnabel CA, Sullivan PS, et al. Multisite validation study to determine performance characteristics of a 92-gene molecular cancer classifier. Clin Cancer Res 2012;18:3952-60. [Crossref] [PubMed]
- Pillai R, Deeter R, Rigl CT, et al. Validation and reproducibility of a microarray-based gene expression test for tumor identification in formalin-fixed, paraffin-embedded specimens. J Mol Diagn 2011;13:48-56. [Crossref] [PubMed]
- Orywal K, Szmitkowski M. Alcohol dehydrogenase and aldehyde dehydrogenase in malignant neoplasms. Clin Exp Med 2017;17:131-9. [Crossref] [PubMed]
- Matejcic M, Gunter MJ, Ferrari P. Alcohol metabolism and oesophageal cancer: a systematic review of the evidence. Carcinogenesis 2017;38:859-72. [Crossref] [PubMed]