Predicting miRNA targets for hepatocellular carcinoma with an integrated method
Introduction
MicroRNAs (miRNAs), as a kind of small non-coding RNA molecules (~22 nucleotides in length), were estimated to regulate as much as 60% of the human protein-coding genes (1,2). miRNAs modulated the levels of post-transcriptionally targeted genes, according to their complementary sequences in the 3’/5’-untranslated regions or the open reading frames of the messenger RNAs (mRNAs) (3,4). Meanwhile, the previous study has demonstrated that miRNAs might be promising biomarkers for cancer classification and outcome prediction (5). The possible inferences were that miRNAs participated in multiple complex processes related to cancer development and progression, such as proliferation, metabolism, differentiation, and apoptosis (6,7). Therefore, the investigation of miRNA functions could offer an excellent approach to elucidate the complex pathological mechanisms underlying malignant tumors, such as hepatocellular carcinoma (HCC).
Currently, several methods have been proposed to identify miRNA targets with sequence data or to study miRNA-mRNA interaction by incorporating expression data into their regulatory network (8,9). Nonetheless, results from different predicted methods were generally inconsistent, even with a high rate of false positives and false negatives (10). The binding sites of miRNA were too small to support statistically significant prediction; besides, a small difference in the algorithm could lead to a great diversity in the results (10,11). Besides, there were generally a series of hypotheses on data when the model was established in each method, and these assumptions may be suitable for some datasets but not for others. Thus, these methods may not perform well if the assumptions violated the underlying relationships. What is more, for individual methods, the predicting process was static, and the predicted targets of a certain miRNA might not be expressed at all under a specific condition (12).
Fortunately, an integrated method has been proposed by combining different prediction methods, and it has been validated to perform better than all the individual component methods (13). Here, the integrated method combining a correlation method (Pearson’s correlation coefficient, PCC), a causal inference method (IDA), and a regression method (Lasso) was generated with the Borda count election method. It could not only solve the inconsistent problems by considering complementary results, (14) but also find confirmed interactions in the incomplete ground truth that not discovered by existing individual methods (13). Above all, with the integrated method, more reliable results could be obtained than that of existing individual methods.
Therefore, in the present work, an integrated method was employed to predict miRNA targets for HCC patients because only a few studies focused on this aspect. The flow diagram was displayed (Figure 1). First, miRNA targets were predicted with the PCC, IDA, and Lasso methods, respectively. Subsequently, the top 100 predicted targets of each miRNA generated by individual methods were integrated based on the
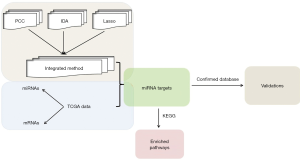
Borda count election method. Then, the miRNA targets were confirmed by comparing them with confirmed databases. Finally, the Kyoto Encyclopedia of Genes and Genomes (KEGG) pathway enrichment analysis was conducted for target genes enriched in top 1,000 interactions to capture significant pathways. These targets might be critical for HCC treatment and supply great insights for revealing the underlying pathological mechanism.
Methods
Dataset
The Cancer Genome Atlas (TCGA) was a comprehensive and coordinated effort to accelerate our understanding of the molecular basis of cancer through the application of genome analysis technologies, including large-scale genome sequencing (15). Thus, miRNA and mRNA expression data for HCC were recruited from the TCGA database (http://cancergenome.nih.gov/). Only samples simultaneously existed in the two expression sets were regarded as study objects. For improving the quality of the datasets, miRNAs, and mRNAs with the expression values equaled to zero were deleted; the reserved expression values were normalized and converted into log2 forms. Consequently, a total of 854 miRNAs and 20,140 mRNAs were included in the expression data for further exploitation.
miRNA target prediction
The integrated method was designed to take advantage of the individual methods (PCC, IDA, and Lasso) and to compensate for their drawbacks. Specifically, the miRNA-mRNA interactions were ranked in individual methods based on certain criteria, such as the strength of the correlation coefficients. However, the new rankings in the integrated method were formed by merging the ranking rules from different methods. In detail, firstly, the miRNA targets were predicted the PCC, IDA, and Lasso method respectively, and the top k (k=100) performers in identifying miRNA targets were chosen (13). Secondly, the Borda rank election method was employed for ranking each miRNA and providing a single ranking list of elected mRNAs concerning the miRNA. Finally, the top-ranked genes from the list were extracted as the final output, i.e., the potential target genes for a certain miRNA.
Particularly, the Borda rank election method was an efficient algorithm for integrating orderly appraising results from several separated methods (16). Its specific process was described as following: setting an election consist of a set V of voters, and each identified candidate was assigned with a preference order, a strict, complete, and transitive order on the set C of candidates. Subsequently, each candidate was given ||C|| − N points for each voter who ranked him or her in Nth place (so, ||C|| − 1 points for first, ||C|| − 2 for second, and so forth until the candidate which the voter ranked last received no points). Finally, the average point of the candidate across all voters was calculated, which was defined as the z-score. In brief, for all voters, u and all candidates v, a score (u, v) were defined as the number of points which u gained from v’s vote (in other words, the difference between the total number of candidates and the ordinal at which v ranked u). In the case of a weighted selection, score (u, v) was multiplied by v’s weight, which was termed as z-score. The higher the z-score was, the more significant the prediction results were. The top k ranked target genes for HCC could be obtained, ranking the predicted miRNA targets according to their z-scores.
Ground truth for validation
Since the number of experimentally confirmed miRNAs targets has been still limited, it was difficult to evaluate and compare different computational methods with complete ground-truth. (17) In previous literature, several methods have been proposed (18,19), especially a semi-supervised method. (18) It was mainly dependent on the support vector machine (SVM), which involved experimentally confirmed database miRTarBase (20) as a train set and TarBase (21) as a test set. Because of the good classification performances of miRTarBase and Tarbase, both of them were employed, and another two commonly applied databases [miRecords (22) and miRWalk (23)] were also combined for validating miRNA targets, due to the limited number of confirmed interactions. In particular, miRTarbase provided the most current and comprehensive information of experimentally validated miRNA target interactions (24). While TarBase was the first resource to provide experimentally verified miRNA target interactions by surveying pertinent literature (25). Concerning miRecords, both experimentally validated miRNA targets and computationally predicted miRNA targets were accumulated (22). And miRWalk was a publicly available comprehensive resource, hosting the predicted as well as the experimentally validated miRNA target interaction pairs (23).
Pathway analysis for miRNA targets
To investigate functional biological processes associated with miRNA targets in top k’ (k’ =1,000) miRNA-mRNA interactions (13), pathways enriched in the KEGG pathway database were screened by Database for Annotation, Visualization, and Integrated Discovery (DAVID, http://david.abcc.ncifcrf.gov/tools.jsp) (26). Pathways with P<0.05 were selected based on the Expression Analysis Systematic Explorer (EASE) test applied in DAVID. EASE analysis of the regulated genes showed molecular functions and biological processes unique to each category (27). The threshold of the minimum number of genes ≥2 of the corresponding term was considered significant for a category.
Results
Predicted miRNA targets
In the current study, after performing the standard pretreatment on expression data of HCC in the TCGA database, a total of 854 miRNAs and 20,140 mRNAs were obtained and included for the later analysis. These miRNA and mRNA data were analyzed with the integrated method to predict miRNA targets for HCC patients. The integrated method combined a correlation method (PCC), a causal IDA, and a regression method (Lasso), according to the Borda count election. During this process, miRNA targets were referred to as target mRNAs or genes defined according to miRNA-mRNA interactions. Importantly, a z-score was assigned to each miRNA-mRNA interaction. The higher the z-score was, the more significant the prediction results were. As a result, a total of 4783 target interactions were obtained from the integrated method. Since the large scale of miRNA targets, only the top 1,000 ranked interactions were selected, which might be more important than the others for HCC as study objects. Furthermore, the top 50 were considered to be highly-confident miRNA-mRNA interactions for HCC (Table 1) (13). The interaction between AATK and hsa-mir-338 was observed to be the most significant one with the highest z-score of 4,468. The following four important interactions were hsa-mir-203-ASPG (z-score =4,434), hsa-mir-505-ATP11C (z-score =4,391), hsa-mir-1180-B9D1 (z-score =4,308),and hsa-mir-185-C22orf25 (z-score =4,297). Interestingly, among the 50 interactions, AATK was simultaneously controlled by both hsa-mir-338 and hsa-mir-766. Consequently, the expression of one gene might be co-adjusted by several miRNAs. Moreover, if a gene was regulated by many miRNAs or predicted for several times, perhaps it may be inferred as more significant than those only were predicted for once. Hence, the predicted times for genes among 1,000 miRNA-mRNA interactions were calculated by summing up their total predicted times from different miRNAs, and the targets with predicted times ≥10 were listed (Table 2). A total of 6 miRNA targets were obtained, MEG3, OLFML3, DSCAML1, CCDC8, SSC5D, and MFAP2. Specifically, the MEG3 possessed the highest predicted times of 29. Both OLFML3 and DSCAML1 were predicted for 12 times, but most of the miRNAs were different. This condition was also applied to targets SSC5D and MFAP2.
Table 1
ID | mRNA | miRNA | z-score |
---|---|---|---|
1 | AATK | hsa-mir-338 | 4,468 |
2 | ASPG | hsa-mir-203 | 4,434 |
3 | ATP11C | hsa-mir-505 | 4,391 |
4 | B9D1 | hsa-mir-1180 | 4,308 |
5 | C22orf25 | hsa-mir-185 | 4,297 |
6 | C6orf155 | hsa-mir-30a | 4,272 |
7 | C7orf50 | hsa-mir-339 | 4,241 |
8 | C9orf5 | hsa-mir-32 | 4,236 |
9 | CALCR | hsa-mir-653 | 4,219 |
10 | COPZ2 | hsa-mir-152 | 4,200 |
11 | FGF13 | hsa-mir-504 | 4,187 |
12 | GIPR | hsa-mir-642a | 4,168 |
13 | GPC1 | hsa-mir-149 | 4,105 |
14 | HOXA10 | hsa-mir-196b | 4,063 |
15 | HOXD8 | hsa-mir-10b | 4,001 |
16 | HTR2C | hsa-mir-1911 | 3,974 |
17 | IGF2 | hsa-mir-483 | 3,881 |
18 | KLF7 | hsa-mir-2355 | 3,863 |
19 | MEST | hsa-mir-335 | 3,847 |
20 | OSBP2 | hsa-mir-3200 | 3,809 |
21 | PDE2A | hsa-mir-139 | 3,794 |
22 | PDE4D | hsa-mir-582 | 3,766 |
23 | PTK2 | hsa-mir-151 | 3,735 |
24 | PTPRN2 | hsa-mir-153- | 3,682 |
25 | RASGRF1 | hsa-mir-184 | 3,657 |
26 | RCL1 | hsa-mir-101-2 | 3,584 |
27 | RPS6KA1 | hsa-mir-1976 | 3,560 |
28 | RSAD2 | hsa-mir-3614 | 3,548 |
29 | AATK | hsa-mir-766 | 3,504 |
30 | SH3TC2 | hsa-mir-584 | 3,499 |
31 | SREBF1 | hsa-mir-33b | 3,485 |
32 | TMEM164 | hsa-mir-652 | 3,468 |
33 | TRPM1 | hsa-mir-211 | 3,461 |
34 | TYW3 | hsa-mir-186 | 3,457 |
35 | UGT8 | hsa-mir-577 | 3,442 |
36 | ZNF826 | hsa-mir-1270-2 | 3,423 |
37 | C21orf34 | hsa-mir-125b-2 | 3,419 |
38 | MIA3 | hsa-mir-664 | 3,406 |
39 | ODZ4 | hsa-mir-708 | 3,376 |
40 | RMST | hsa-mir-1251 | 3,364 |
41 | TTF2 | hsa-mir-942 | 3,351 |
42 | CHRM2 | hsa-mir-490 | 3,307 |
43 | LRRC29 | hsa-mir-328 | 3,240 |
44 | RXRB | hsa-mir-219-1 | 3,197 |
45 | SKA2 | hsa-mir-454 | 3,007 |
46 | XKR6 | hsa-mir-598 | 2,972 |
47 | CXorf66 | hsa-mir-505 | 2,748 |
48 | HOXA9 | hsa-mir-196b | 2,680 |
49 | HOXD9 | hsa-mir-10b | 2,549 |
50 | hsa-mir-155 | 2,308 |
Table 2
Targets | Times | miRNAs |
---|---|---|
MEG3 | 29 | hsa-mir-127, hsa-mir-134, hsa-mir-154, hsa-mir-299, hsa-mir-323, hsa-mir-323b, hsa-mir-337, hsa-mir-369, hsa-mir-370, hsa-mir-376a-2, hsa-mir-376b, hsa-mir-377, hsa-mir-379, hsa-mir-381, hsa-mir-382, hsa-mir-409, hsa-mir-410, hsa-mir-411, hsa-mir-412, hsa-mir-431, hsa-mir-432, hsa-mir-433, hsa-mir-485, hsa-mir-487a, hsa-mir-493, hsa-mir-494, hsa-mir-539, hsa-mir-541, hsa-mir-889 |
OLFML3 | 12 | hsa-mir-127, hsa-mir-299, hsa-mir-337, hsa-mir-370, hsa-mir-377, hsa-mir-379, hsa-mir-381, hsa-mir-382, hsa-mir-409, hsa-mir-411, hsa-mir-433, hsa-mir-494 |
DSCAML1 | 12 | hsa-mir-299, hsa-mir-323, hsa-mir-323b, hsa-mir-369, hsa-mir-370, hsa-mir-377, hsa-mir-409, hsa-mir-432, hsa-mir-433, hsa-mir-485, hsa-mir-493, hsa-mir-889 |
CCDC8 | 11 | hsa-mir-125a, hsa-mir-136, hsa-mir-181c, hsa-mir-199b, hsa-mir-299, hsa-mir-377, hsa-mir-381, hsa-mir-409, hsa-mir-411, hsa-mir-598, hsa-mir-758 |
SSC5D | 10 | hsa-mir-10a, hsa-mir-1247, hsa-mir-125a, hsa-mir-136, hsa-mir-153-2, hsa-mir-199b, hsa-mir-214, hsa-mir-376b, hsa-mir-376c, hsa-mir-758 |
MFAP2 | 10 | hsa-mir-1287, hsa-mir-299, hsa-mir-376b, hsa-mir-409, hsa-mir-485, hsa-mir-487a, hsa-mir-493, hsa-mir-496, hsa-mir-887, hsa-mir-99b |
Validation of miRNA targets
The results were compared with confirmed miRTarBase, Tarbase, miRecords and miRWalk database was used to validate the miRNA targets predicted by the integrated method. There were 37,372 interactions with 576 miRNAs, 20,095 interactions with 228 miRNAs, 21,590 interactions with 195 miRNAs, and 1,710 interactions with 226 miRNAs in miRTarBase, Tarbase, miRecords and miRWalk database, respectively. After removing the duplicated or invalid miRNA-mRNA interactions, 62,858 interactions were kept for the validations, termed as background interactions. If one miRNA target interaction participated in background interactions, the predicted miRNA target was confirmed. A total of 40 miRNA-mRNA interactions were confirmed, which further showed that our method was an available and valuable measure for predicting miRNA targets.
Enriched pathways for miRNA targets
As described above, the KEGG pathway enrichment analysis was conducted for 860 genes in the top 1,000 miRNA-mRNA interactions. When setting the thresholds with P <0.05 and count ≥2, 26 pathways were named (Table 3). In detail, complement and coagulation cascades (P=2.05E-08), bile secretion (P=4.36E-05), primary immunodeficiency (P=7.13E-04), Arginine and proline metabolism (P =2.25E-03) and Alanine aspartate and glutamate metabolism (P=5.02E-03) were the most significant five pathways. Of note 10 of 26 pathways were attributed to metabolism pathways, including arginine and proline metabolism, alanine aspartate and glutamate metabolism, drug metabolism—cytochrome P450, phenylalanine metabolism, metabolism of xenobiotics by cytochrome P450, histidine metabolism, drug metabolism—other enzymes, fructose, and mannose metabolism, ether lipid metabolism, and ascorbate and aldarate metabolism.
Table 3
ID | Pathway | P value |
---|---|---|
1 | Complement and coagulation cascades | 2.05E-08 |
2 | Bile secretion | 4.36E-05 |
3 | Primary immunodeficiency | 7.13E-04 |
4 | Arginine and proline metabolism | 2.25E-03 |
5 | Alanine aspartate and glutamate metabolism | 5.02E-03 |
6 | Cytokine-cytokine receptor interaction | 9.22E-03 |
7 | Drug metabolism—cytochrome P450 | 1.41E-02 |
8 | Phenylalanine metabolism | 1.84E-02 |
9 | Metabolism of xenobiotics by cytochrome P450 | 2.83E-02 |
10 | Pancreatic secretion | 3.07E-02 |
11 | Histidine metabolism | 3.16E-02 |
12 | Drug metabolism—other enzymes | 3.46E-02 |
13 | Cell adhesion molecules (CAMs) | 3.53E-02 |
14 | Peroxisome | 3.73E-02 |
15 | Pentose and glucuronate interconversions | 3.83E-02 |
16 | Phenylalanine tyrosine and tryptophan biosynthesis | 3.84E-02 |
17 | Proximal tubule bicarbonate reclamation | 4.15E-02 |
18 | Fat digestion and absorption | 4.16E-02 |
19 | Vascular smooth muscle contraction | 4.19E-02 |
20 | Fructose and mannose metabolism | 4.23E-02 |
21 | Ether lipid metabolism | 4.29E-02 |
22 | Hematopoietic cell lineage | 4.38E-02 |
23 | Ascorbate and aldarate metabolism | 4.59E-02 |
24 | Primary bile acid biosynthesis | 4.69E-02 |
25 | Glycolysis/gluconeogenesis | 4.71E-02 |
26 | T cell receptor signaling pathway | 4.94E-02 |
KEGG, Kyoto Encyclopedia of Genes and Genomes.
Discussion
HCC has been the third leading cause of cancer-related mortality, and there were 700,000 new cases diagnosed every year (28). The heterogeneity of HCC brought about great unique challenges for treatments (29); thus, it was so important to find early diagnostic markers and therapeutic targets (30). Among various cancer-associated markers, miRNAs have attracted particular attention for its regulation of genes. Since many miRNAs were located on chromosomal regions that were frequently varied in cancer (31) indicating their roles as a novel class of oncogenes and tumor suppressors (32). This finding clarified a new aspect for us to investigate miRNA targets as HCC biomarkers.
Up to date, several computational approaches were developed to predict miRNA targets utilizing the expression data, such as PCC (33), IDA (34,35), and Lasso (36). Specifically, PCC, a correlation method, was the commonly used measure for evaluating the strength of the association between a pair of variables (33). The data was ranked in the descending order based on absolute PCC values. Thus negative miRNA-mRNA correlations may be ranked at the top since the general down-regulation effects of miRNAs (13). Meanwhile, the availability of PCC would be greatly reduced if the correlations were non-linear (37). IDA, a causal IDA, was aimed to evaluate the causal effect between two variables (34,35). A large portion of miRNA-mRNA causal regulatory relationships revealed by IDA was overlapped with the results of the follow-up gene knockdown experiments (38). Lasso, a regression method, minimized the usual sum of squared errors, with a bound on the sum of the absolute values of the coefficients (36). Similar to the PCC method, the downregulation was favored, and the miRNA-mRNA pairs with negative effects were ranked at the top of the ranking list.
In our study, the Borda count election method was employed to combine the above three methods and obtain the integrated method. Later, the prediction results from the integrated method were compared with the background interactions from the confirmed database for confirming the feasibility of the integrated method. Based on the integrated method, miRNA targets from the top 1,000 miRNA-mRNA interactions for HCC samples were predicted. Because experimentally validated databases were still spare, a set of highly confident interactions were reported for further experiment validations. Among the 50 interactions, hsa-mir-338-AATK was the most important one with the highest z-score. AATK (Apoptosis-associated tyrosine kinase), a tyrosine kinase domain at the N-terminus and a proline-rich domain at the C-terminus, has been shown to play a role in cell differentiation, growth, and apoptosis (39). It demonstrated a coordinated reduction of miR-338-3p and AATK under insulin resistance conditions, providing evidence for a cooperative action of the miRNA and its hosting gene in compensatory β-cell mass expansion (40). Apart from the hosting role of AATK for miR-338-3p, this gene also played active roles in the enzymatic activity and the chromosomal generation of intronic miR-338. Huang et al. suggested that the level of miR-338 expression was associated with clinical aggressiveness of HCC in patients, such as tumor size, tumor-node-metastasis stage, vascular invasion, and intrahepatic metastasis (41). In all, AATK played significant roles in the progression of HCC.
It was well known that one gene might be targeted by several miRNAs [8], and dysregulation of these relationships would make effects on the biological functions associated with a specific tumor (42). Thus, the repeated predicted times for one target gene were computed, and the result uncovered that MEG3 possessed the highest predicted times of 29. MEG3 (maternally expressed gene 3) was an imprinted gene belonging to the imprinted DLK1 (delta-like non-canonical Notch ligand 1)-MEG3 locus, which was located at chromosome 14q32.3 in humans (43). It was expressed in normal tissues, but its expression was lost in multiple cancer cell lines from various tissue. The previous study revealed that MEG3 was one of the most significantly down-regulated long non-coding RNAs (lncRNAs) in malignant hepatocytes of HCC patients (44). Besides, deregulation of MEG3 accompanied by extensive aberrations in DNA methylation could be confirmed experimentally in an independent series of
HCC (45). Zhuo et al. indicated that MEG3, acting as a potential biomarker in predicting the prognosis of HCC, was regulated by UHRF1 (ubiquitin-like with Ph.D. and ring finger domains 1) via recruiting DNMT1 (DNA methyltransferase 1) and regulating p53 expression (46). Hence MEG3 was closely correlated to HCC.
For investigating functional gene sets involved in the miRNA targets, KEGG pathway enrichment analysis was conducted. A total of 26 pathways were obtained, of which complement and coagulation cascades were most significant. The complement system was a proteolytic cascade in blood plasma and a mediator of innate immunity, as well as a nonspecific defense mechanism against pathogens (47). While blood coagulation was another series of proenzyme-to-serine protease conversions. The formation of thrombin culminated, and the enzyme was responsible for the conversion of soluble fibrinogen to the insoluble fibrin clot (48). It reported that the differentially expressed genes might be involved in hepatocarcinogenesis through downregulating the pathways of complement and coagulation cascades (49). Hence this pathway was important for HCC.
In summary, miRNA targets (such as AATK and MEG3) for HCC were predicted based on the integrated method and confirmed according to confirmed databases. The results showed that this method was valuable and possible for miRNA prediction. Meanwhile, the findings would supply potential biomarkers for the diagnosis and treatment of HCC, as well as revealing the pathological mechanisms underlying this tumor. However, experimental validations should be performed to confirm the target genes and highly confident miRNA-mRNA interactions in the future.
Acknowledgments
Funding: None.
Footnote
Conflicts of Interest: All authors have completed the ICMJE uniform disclosure form (available at http://dx.doi.org/10.21037/tcr.2020.02.46). The authors have no conflicts of interest to declare.
Ethical Statement: The authors are accountable for all aspects of the work in ensuring that questions related to the accuracy or integrity of any part of the work are appropriately investigated and resolved. The study was conducted in accordance with the Declaration of Helsinki (as revised in 2013). Institutional ethical approval and informed consent were waived.
Open Access Statement: This is an Open Access article distributed in accordance with the Creative Commons Attribution-NonCommercial-NoDerivs 4.0 International License (CC BY-NC-ND 4.0), which permits the non-commercial replication and distribution of the article with the strict proviso that no changes or edits are made and the original work is properly cited (including links to both the formal publication through the relevant DOI and the license). See: https://creativecommons.org/licenses/by-nc-nd/4.0/.
References
- Friedman RC, Farh KK, Burge CB, et al. Most mammalian mRNAs are conserved targets of microRNAs. Genome Res 2009;19:92-105. [Crossref] [PubMed]
- Bartel DP. MicroRNAs: genomics, biogenesis, mechanism, and function. Cell 2004;116:281-97. [Crossref] [PubMed]
- Berezikov E, Cuppen E, Plasterk RH. Approaches to microRNA discovery. Nat Genet 2006;38:S2-7. [Crossref] [PubMed]
- Bartel DP. MicroRNAs: target recognition and regulatory functions. Cell 2009;136:215-33. [Crossref] [PubMed]
- Jay C, Nemunaitis J, Chen P, et al. miRNA profiling for diagnosis and prognosis of human cancer. DNA Cell Biol 2007;26:293-300. [Crossref] [PubMed]
- Ha M, Kim VN. Regulation of microRNA biogenesis. Nature Reviews Molecular Cell Biol 2014;15:509-524. [Crossref] [PubMed]
- Xu C, Lu Y, Pan Z, et al. The muscle-specific microRNAs miR-1 and miR-133 produce opposing effects on apoptosis by targeting HSP60, HSP70 and caspase-9 in cardiomyocytes. J Cell Sci 2007;120:3045-52. [Crossref] [PubMed]
- Krek A, Grün D, Poy MN, et al. Combinatorial microRNA target predictions. Nat Genet 2005;37:495-500. [Crossref] [PubMed]
- Liu B, Li J, Tsykin A, et al. Exploring complex miRNA-mRNA interactions with Bayesian networks by splitting-averaging strategy. BMC Bioinformatics 2009;10:408. [Crossref] [PubMed]
- Rajewsky N. microRNA target predictions in animals. Nat Genet 2006;38:S8-13. [Crossref] [PubMed]
- Sethupathy P, Megraw M, Hatzigeorgiou AG. A guide through present computational approaches for the identification of mammalian microRNA targets. Nat Methods 2006;3:881-6. [Crossref] [PubMed]
- Farazi TA, Spitzer JI, Morozov P, Tuschl T. miRNAs in human cancer. J Pathol 2011;223:102-15. [Crossref] [PubMed]
- Le TD, Zhang J, Liu L, et al. Ensemble Methods for MiRNA Target Prediction from Expression Data. PLoS One 2015;10:e0131627. [Crossref] [PubMed]
- Marbach D, Costello JC, Küffner R, et al. Wisdom of crowds for robust gene network inference. Nat Methods 2012;9:796-804. [Crossref] [PubMed]
- Zhu Y, Qiu P, Ji Y. TCGA-assembler: open-source software for retrieving and processing TCGA data. Nat Methods 2014;11:599-600. [Crossref] [PubMed]
- Russell N. Complexity of control of Borda count elections. 2007.
- Le TD, Liu L, Zhang J, et al. From miRNA regulation to miRNA-TF co-regulation: computational approaches and challenges. Brief Bioinform 2015;16:475-96. [Crossref] [PubMed]
- Pio G, Malerba D, D'Elia D, et al. Integrating microRNA target predictions for the discovery of gene regulatory networks: a semi-supervised ensemble learning approach. BMC Bioinformatics 2014;15:S4. [Crossref] [PubMed]
- Zhang Y, Verbeek FJ. Comparison and integration of target prediction algorithms for microRNA studies. J Integr Bioinform 2010;7:127. [Crossref] [PubMed]
- Chou CH, Chang NW, Shrestha S, et al. miRTarBase 2016: updates to the experimentally validated miRNA-target interactions database. Nucleic Acids Res 2016;44:D239-47. [Crossref] [PubMed]
- Vergoulis T, Vlachos IS, Alexiou P, et al. TarBase 6.0: capturing the exponential growth of miRNA targets with experimental support. Nucleic Acids Res 2012;40:D222-9. [Crossref] [PubMed]
- Xiao F, Zuo Z, Cai G, et al. miRecords: an integrated resource for microRNA-target interactions. Nucleic Acids Res 2009;37:D105-10. [Crossref] [PubMed]
- Dweep H, Gretz N, Sticht C. miRWalk database for miRNA-target interactions. Methods Mol Biol 2014;1182:289-305. [Crossref] [PubMed]
- Hsu SD, Tseng YT, Shrestha S, et al. miRTarBase update 2014: an information resource for experimentally validated miRNA-target interactions. Nucleic Acids Res 2014;42:D78-85. [Crossref] [PubMed]
- Papadopoulos GL, Reczko M, Simossis VA, et al. The database of experimentally supported targets: a functional update of TarBase. Nucleic Acids Res 2009;37:D155-8. [Crossref] [PubMed]
- Huang W, Sherman BT, Lempicki RA. Systematic and integrative analysis of large gene lists using DAVID bioinformatics resources. Nat Protoc 2009;4:44-57. [Crossref] [PubMed]
- Ford G, Xu Z, Gates A, et al. Expression Analysis Systematic Explorer (EASE) analysis reveals differential gene expression in permanent and transient focal stroke rat models. Brain Res 2006;1071:226-36. [Crossref] [PubMed]
- Mu X, Español-Suñer R, Mederacke I, et al. Hepatocellular carcinoma originates from hepatocytes and not from the progenitor/biliary compartment. J Clin Invest 2015;125:3891. [Crossref] [PubMed]
- Llovet JM, Peña CE, Lathia CD, et al. Plasma biomarkers as predictors of outcome in patients with advanced hepatocellular carcinoma. Clin Cancer Res 2012;18:2290-300. [Crossref] [PubMed]
- Arzumanyan A, Reis HM, Feitelson MA. Pathogenic mechanisms inHBV-and HCV-associated hepatocellular carcinoma. Nat Rev Cancer 2013.13:123-35.
- Croce CM. Causes and consequences of microRNA dysregulation in cancer. Nat Rev Genet 2009;10:704-14. [Crossref] [PubMed]
- Lujambio A, Lowe SW. The microcosmos of cancer. Nature 2012;482:347-55. [Crossref] [PubMed]
- Nahler G. Pearson correlation coefficient. Dictionary of Pharmaceutical Medicine. Springer, Vienna, 2009;132-32.
- Maathuis MH, Kalisch M, Bühlmann P. Estimating high-dimensional intervention effects from observational data. Ann Statist 2009;37:3133-64. [Crossref]
- Maathuis MH, Colombo D, Kalisch M, et al. Predicting causal effects in large-scale systems from observational data. Nat Methods. 2010;7:247-248. [Crossref] [PubMed]
- Friedman J, Hastie T, Tibshirani R. glmnet: Lasso and elastic-net regularized generalized linear models. R package version 1.9–5. 2013, R Foundation for Statistical Computing Vienna.
- Speed T. A correlation for the 21st century. Science 2011;334:1502-3. [Crossref] [PubMed]
- Le TD, Liu L, Tsykin A, et al. Inferring microRNA-mRNA causal regulatory relationships from expression data. Bioinformatics 2013;29:765-71. [Crossref] [PubMed]
- Ma S, Rubin BP. Apoptosis-associated tyrosine kinase 1 inhibits growth and migration and promotes apoptosis in melanoma. Lab Invest 2014;94:430-8. [Crossref] [PubMed]
- Jacovetti C, Jimenez V, Ayuso E, et al. Contribution of Intronic miR-338-3p and Its Hosting Gene AATK to Compensatory β-Cell Mass Expansion. Mol Endocrinol 2015;29:693-702. [Crossref] [PubMed]
- Huang XH, Wang Q, Chen JS, et al. Bead-based microarray analysis of microRNA expression in hepatocellular carcinoma: miR-338 is downregulated. Hepatol Res 2009;39:786-94. [Crossref] [PubMed]
- Liu D, Liu J, Lin B, et al. Lewis y Regulate Cell Cycle Related Factors in Ovarian Carcinoma Cell RMG-I in Vitro via ERK and Akt Signaling Pathways. Int J Mol Sci 2012;13:828-39. [Crossref] [PubMed]
- Zhou Y, Zhang X, Klibanski A. MEG3 noncoding RNA: a tumor suppressor. J Mol Endocrinol 2012;48:R45-53. [Crossref] [PubMed]
- Braconi C, Kogure T, Valeri N, et al. microRNA-29 can regulate expression of the long non-coding RNA gene MEG3 in hepatocellular cancer. Oncogene 2011;30:4750-6. [Crossref] [PubMed]
- Anwar SL, Krech T, Hasemeier B, et al. Loss of imprinting and allelic switching at the DLK1-MEG3 locus in human hepatocellular carcinoma. PLoS One 2012;7:e49462. [Crossref] [PubMed]
- Zhuo H, Tang J, Lin Z, et al. The aberrant expression of MEG3 regulated by UHRF1 predicts the prognosis of hepatocellular carcinoma. Mol Carcinog 2016;55:209-19. [Crossref] [PubMed]
- Amara U, Flierl MA, Rittirsch D, et al. Molecular intercommunication between the complement and coagulation systems. J Immunol 2010;185:5628-36. [Crossref] [PubMed]
- Ekdahl KN, Lambris JD, Markiewski MM, et al. Complement and coagulation: strangers or partners in crime? Trends Immunol 2007;28:184-92. [Crossref] [PubMed]
- Cheng P, Cheng Y, Su MX, et al. Bicluster and Pathway Enrichment Analysis of HCV-induced Cirrhosis and Hepatocellular Carcinoma. Asian Pac J Cancer Prev 2012;13:3741-5. [Crossref] [PubMed]