Exome sequencing identified six copy number variations as a prediction model for recurrence of primary prostate cancers with distinctive prognosis
Introduction
Second-generation sequencing is an efficient technique to identify gross genomic changes in cancer, including gene fusion events and copy number variations (CNVs). In this context, whole genome sequencing (WGS) would be the ideal application but, due to the cost and the complexity of data analysis, its applicability in many clinical settings has reduced. Instead, exome sequencing is a more cost effective approach which has been largely used to identify single nucleotide polymorphisms (SNPs) and small insertions and deletions (indels). Moreover, several studies have successfully utilized whole exome sequencing (WES) as a platform for the identification of CNVs (1), and a number of algorithms have been developed for this purpose, including ExomeCNV, VarScan2, exomeCopy, CoNIFER, ExomeDepth and XHMM (2). Lastly, exome sequencing can also be used to identify subsets of genomic rearrangements whose breakpoints are located in or near exon regions (3).
CNVs can play important roles in prostate carcinogenesis. Using an array comparative genomic hybridization (aCGH) method, Saramäki and colleagues have confirmed common gains and losses along the genome of prostate cancer (PCa) samples, including gains at 1q, 7, 8q, 16p and 17q, and losses at 2q, 4p/q, 6q, 8p, 13q, 16q, 17p and 18q, in addition to a novel recurrent gain at 9p13-q21 (4). CNVs have been also related to the prognosis of PCa. According to the current literature, a CNV-based model can correctly predict 73% of relapsed cases and 75% of the cases with short PSA doubling time (PSADT, <4 months) (5). In another WGS study, Camacho and colleagues detected 64 recurrent regions of genomic loss and gain, and confirmed that the burden of SCNAs (somatic copy number alteration) was predictive for biochemical recurrence (BCR). At the same time, nine individual regions were also associated with relapse, including two deletions and seven gains (6).
Gene fusion events also play important roles in PCas. Indeed, the first gene fusion event occurring in solid tumors was discovered in PCa, which involved the identification of the fused TMPRSS2-ETS gene (7). Another example relates to TMPRSS2:ERG gene fusions, which are present in ~50% of PCa cases. TMPRSS2:ERG is capable of inducing TGF-β signaling and epithelial to mesenchymal transition (EMT) in human PCa cells (8). Moreover, the incidence of TMPRSS2:ERG gene fusions have been also related to PCa prognosis. As reported, Kulda and colleagues showed that a combination between high level of prostate specific antigen (PSA) in preoperative serum and increased expression of TMPRSS2-ERG in tumor tissues (P=0.0001) was the most promising marker of recurrence risk after radical prostatectomy (9). Consistently, Nam and colleagues showed that, in a 5-year period, patients with TMPRSS2:ERG fusion had a significantly higher risk of recurrence than patients without this gene fusion (58.4% versus 8.1%, respectively; P<0.0001) (10). Still, TMPRSS2-ERG fusion was not a frequent genomic alteration among PCa patients from Asian countries such as China and Turkey and, in this case, it appears to have a limited significance in the clinical practice (11,12).
In order to predict PCa recurrence after radical prostatectomy (RP), a variety of prediction models and biomarkers have been developed. For instance, Lalonde and colleagues developed a 100-locus genomic classifier (later refined to 31 functional loci) which could identify patients with elevated BCR rates (hazard ratio =2.73, P<0.001) (13). Oh and colleagues selected 16 significant predictive SNPs of BCR, from a large-scale exome wide association study, and indicated that the built GRS (genetic risk score) had additional predictive gain for BCR after RP (14). Furthermore, Campbell and colleagues performed a systematic review of prediction tools and suggested different tools for different purpose (15). For example, they recommended Stephenson nomograms for BCR, the CAPRA nomogram for aggressive BCR and metastasis for preoperative prognosis. For postoperative prognosis, different prediction models were suggested (15). For example, they suggested the CAPRA-Surgery (CAPRA-S), Stephenson, Kattan, Duke prostate cancer (DPC), and the Suardi nomograms for the prediction of BCR, the DPC nomogram for aggressive BCR (15).
In the present study, we have conducted the WES of primary PCa samples with known follow-up outcomes (defined as recurrent or non-recurrent) after 5 or more years of clinical follow-up. Specifically, we sequenced the exome of 30 recurrent and 44 non-recurrent PCa patients and then analyzed the presence of CNVs and fusion genes specific to recurrent/non-recurrent PCa or common to both outcomes. These data were then used to develop a prediction model for PCa recurrence. Clinical assays using genes associated with this model might be further utilized for prediction of recurrence of PCa.
Methods
Patients and study design
This study has been conducted in accordance with the ethical standards, stated by the Declaration of Helsinki as well as by National and International guidelines. This work was approved by the authors’ institutional review board. Several thousands of PCa patients who underwent radical prostatectomy, between 2003 and 2010, at the First Affiliated Hospital of Zhejiang University were screened and classified as recurrent or non-recurrent PCa patients. Non-recurrent PCa patients were defined as those with Gleason score lower than 7 at diagnosis, and with no BCR within 5 years of follow-up. Recurrent PCa patients were those with Gleason score lower than 7 at diagnosis but the presence of BCR within 5 years of follow-up. Paraffin-fixed tissues sections were retrieved from the pathological archives. Tumor contents were sectioned and then analyzed by IHC (immunohistochemistry) staining. Tissue blocks with high tumor content were eventually used for DNA extraction. A total of 44 non-recurrent PCa samples and 30 recurrent PCa samples were involved in this study.
DNA library preparation and sequencing
QIAamp DNA FFPE Tissue Kit (Qiagen, USA) was used to extract DNA from FFPE (formalin-fixed, paraffin-embedded) tissue sections. The quality and concentration of the extracted DNA were evaluated using Nanodrop 2000 spectrophotometer and Invitrogen Qubit 2.0 fluorometer. Thereafter, a sequencing system covering about 180,000 exons of the human genome (SureSelect Human All Exon V5 & V5 + UTRs, Agilent) was used to capture the respective exomes, using 2 µg DNA per sample. A 2×76 bp paired-end sequencing was conducted using the Illumina HiSeq2500 machine.
CNV calling and annotation
Figure 1 presents a flowchart for the analysis of CNVs and gene fusion events originated from WES data. The XHMM (eXome-Hidden Markov Model) software suite (16) was used to annotate CNVs in the exome sequencing data. At first, the “best practice” pipeline (i.e., BWA + Picard + GATK) was used to generate processed alignment files. After running the cnv calling pipeline, the result file (xcnv) was analyzed using the bedtools (17), which computed both the depth and breadth of the coverage of CNV events along the whole genome. After that, duplicated (copy number gain) or deleted (copy number loss) regions (>10 kb), specific to the recurrent group, were filtered accordingly. For this, respective duplicated and deleted regions should be solely present in at least 10% samples of the recurrent group (i.e., absent in the non-recurrent group). In addition, these CNVs should be consistently present in all samples from the recurrent group. The Annovar software tool was further used to annotate these CNV events with the refgene and cytoband databases.
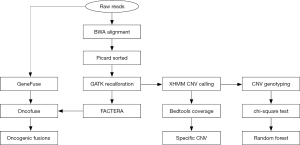
Building of random forest model with CNV events
Using the output file (vcf) of XHMM, the genotypes (DIP, DEL, DUP) related to the CNV events in every sample were picked. Thereafter, the frequency of three genotypes per CNV event in recurrent group and/or non-recurrent group were counted. The chi-square test was used to evaluate the correlation between CNV events and PCa recurrence (CNV events with P value <0.1 were selected). After that, CNV events which could not be genotyped in more than 10% of PCa samples were removed. The remaining CNV events were further used to build a random forest model, with missing genotypes replaced by the most common genotype (DIP, Diploidy). Meanwhile, the importance of predictors (CNV events) was assessed. Lastly, the function tool rfcv of the randomForest package was used to show the cross-validated prediction performance of models with sequentially reduced number of predictors (ranked by variable importance). Based on this modelling performance, a final panel of CNV events was established to build the prediction model (random forest) for PCa recurrence.
Gene fusion detection and annotation
To detect gene fusions in respective PCa samples, we used the software FACTERA (Fusion And Chromosomal Enumeration and Recovery Algorithm) and the GeneFuse program by adopting different algorithms. FACTERA directly processed alignment files (BAM) in three phases: identification of discordant read clusters, detection of breakpoints at nucleotide resolution and in silico validation of candidate fusions (18). By contrast, GeneFuse searched for reads that could be mapped into two different genes (left and right sides), but could not be entirely mapped to any position of the whole reference genome (19). These reads were called “supporting reads for potential gene fusions”. GeneFuse started to run with raw sequences (FASTQ) rather than alignment files (BAM). So, compared with FACTERA, GeneFuse could detect more potential gene fusions but required longer computing time. Accordingly, FACTERA aimed at whole genome to search for fusion events involved with all genes, while GeneFuse aimed at all COSMIC (Catalogue of Somatic Mutations in Cancer) curated fusion genes and druggable fusion genes to search for fusion events involved with specific genes. Afterwards, Oncofuse was used to predict the oncogenic potential of all gene fusions with a naive Bayes Network Classifier, trained and tested using the Weka machine learning package (20).
Results
WES of primary PCa, with different prognosis status, after a 5-year clinical follow-up
We sequenced a total of 44 non-recurrent and 30 recurrent samples by WES. The raw data was submitted to the SRA (Sequence Read Archive) database accordingly [BioProject ID PRJNA496568 (SUB4629515) and SRA submission ID SUB4637601]. After alignment of raw sequences to the human genome (hg19), both coverage rate and average depth were calculated. In most of PCa samples, coverage rate exceeded 99% and the mean coverage rate was 99.4%. The average sequence depth ranged from 20× to 100×, and the average depth of the targeted regions for all samples was 44.8. The detailed description of this dataset has been recently reported (21).
Copy number gains in the chromosome 8q2 and centromere protein F (CENPF) locus is detected in the recurrent PCa samples
Using XHMM, a total of 3,484 CNV events have been identified in the PCa samples. Specifically, 764 duplications and 603 deletions were detected in 76.7% (23/30) of recurrent PCa samples, with a median length of 33.29 and 55.94 kb, respectively. A total of 1,302 duplications and 815 deletions were detected in 68.2% (30/44) of the non-recurrent PCa samples, with a median length of 37.44 and 48.41 kb, respectively.
To identify the CNVs specifically related to the recurrent group, we applied a filter (see Methods) and identified a total of 66 specific CNVs (2 deleted and 64 duplicated regions) specific to the recurrent group. Strikingly, most of the specific duplicated regions in the recurrent group were located on chromosome 8q2, including 8q21, 8q22, 8q23 and 8q24, which were previously shown to be associated with PCa progression and recurrence (22). Except for the well-known gene MYC (MYC Proto-Oncogene, BHLH Transcription Factor), some PCa-related genes were present in duplicated regions of 8q2, such as PTK2 (Protein Tyrosine Kinase 2), RAD21 (RAD21 Cohesin Complex Component), KIAA0196 (WASH Complex Subunit 5) and EIF3H (Eukaryotic Translation Initiation Factor 3 Subunit H). According to the COSMIC database (release v89, 15th May 2019), RAD21 was duplicated in 158 and deleted in 18 PCa samples. KIAA0196 was duplicated in 166 and deleted in 6 PCa samples, and EIF3H was duplicated in 158 and deleted in 26 PCa samples. No copy number variants involving the PTK2 gene were reported. In addition, we have found copy number gains of CENPF (centromere protein F) on chromosome 1q41 and RFPL4A (Ret Finger Protein Like 4A) on 19q13.42, which was reported to be duplicated in 31 and 16 PCa samples according to the COSMIC database, respectively.
Although some of these specific CNVs had been reported in PCa, our findings suggest that these specific duplication or deletion events in recurrent PCa might affect the expression of genes in these chromosomal regions and play roles in the recurrence of PCa. For instance, duplication of 8q24 has been associated with MYC overexpression and characterized as an independent predictor of recurrence after RP (22).
Identification of common CNV events in both recurrent and non-recurrent PCa
In addition to CNV events specific to recurrent and non-recurrent PCa groups, we have also identified 7 duplications and 3 deletions, commonly present in both groups, using the criterion that the specified regions (over 10 kb) should be either duplicated or deleted in, at least, 2 recurrent and 2 non-recurrent PCa samples, with no deletion or duplication in any PCa samples, respectively (Table 1). We noted that one particular CNV resulted in CHD1 (Chromodomain Helicase DNA Binding Protein 1) gene deletion at 5q21.1. According to the COSMIC database, CHD1 deletion was detected in 135 recurrent PCa samples. As previously reported, CHD1 deletion might increase cell invasiveness in PCa, indicating a possible novel role of altered chromatin remodeling during prostate tumorigenesis (23).
Table 1
Region | Gene | Chr | Start | End | Band | Type |
---|---|---|---|---|---|---|
Exonic | RPTN, TCHH | chr1 | 152086333 | 152127241 | 1q21.3 | Gain |
Exonic | OR4C11, OR4C16 | chr11 | 55339551 | 55370953 | 11q11 | Gain |
Exonic | NOMO2 | chr16 | 18570167 | 18604037 | 16p12.3 | Gain |
Exonic | TMPRSS11E, UGT2B17 | chr4 | 69344564 | 69416377 | 4q13.2 | Gain |
Exonic | ZFHX4 | chr8 | 77763117 | 77776784 | 8q21.11 | Gain |
Exonic | GLI4, ZNF696 | chr8 | 144358035 | 144378371 | 8q24.3 | Gain |
Exonic | SPANXB1 | chrX | 140084812 | 140097840 | Xq27.1 | Gain |
Exonic | RHD | chr1 | 25599065 | 25634302 | 1p36.11 | Loss |
Exonic | KIR2DL1, KIR2DL3, KIR3DL3 | chr19 | 55246682 | 55286924 | 19q13.42 | Loss |
Exonic | CHD1 | chr5 | 98204186 | 98240822 | 5q21.1 | Loss |
Note: gain and loss represent copy number gain (duplication) and copy number loss (deletion) respectively. CNV, copy number variation.
Random forest modelling to predict PCa recurrence
To build a prediction model for PCa recurrence, the output file (vcf) of XHMM software which contained a total of 2,866 CNV events, derived from the genome of all PCa samples, were utilized. After statistical analysis of respective CNV genotypes in every PCa sample, a chi-square test indicated that 51 CNV events were correlated with PCa recurrence. Among these, 34 CNVs could not be genotyped in more than 10% of the PCa samples, and 2 CNV events were too small (DNA length less than 1 kb). As a result, the remaining 15 CNVs were used to build a random forest model. Based on a 10-fold cross validation, a prediction model was built with the six most important CNV events (Table 2) and the cross-validated error rate was 11.3% (Figure 2A). In addition, we conducted a receiver operating characteristic (ROC) analysis of the prediction model, based on these same events, resulting in an AUC (area under curve) of 0.857 for predicting PCa recurrence (Figure 2B).
Table 2
ID | Band | Recurrent | Non-recurrent | |||||
---|---|---|---|---|---|---|---|---|
DIP | DEL | DUP | DIP | DEL | DUP | |||
chr1:12907321-12921169 | p36.21 | 15 | 1 | 6 | 22 | 5 | 1 | |
chr5:150632769-150647161 | q33.1 | 18 | 4 | 1 | 24 | 0 | 1 | |
chr10:18112033-18122813 | p12.33 | 19 | 1 | 3 | 26 | 0 | 0 | |
chr7:102208555-102235842 | q22.1 | 16 | 1 | 4 | 24 | 3 | 0 | |
chr11:60978529-60998542 | q12.2 | 16 | 4 | 1 | 29 | 0 | 1 | |
chr8:141799474-141931119 | q24.3 | 19 | 1 | 3 | 29 | 0 | 0 |
Note: DIP, DEL, DUP indicate the genotype of CNVs and the numbers below indicate the frequency of every genotype in recurrent prostate cancers and non-recurrent prostate cancers. CNV, copy number variation; DIP, diploid; DEL, deletion; DUP, duplication.
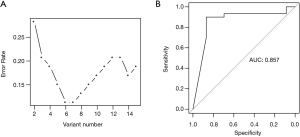
Identification of gene fusion events in PCa
We identified a total of 324 gene fusion events by FACTERA. Furthermore, 67 gene fusion events involving COSMIC curated fusion genes and 150 gene fusion events involving druggable fusion genes were identified by GeneFuse (Table 3). According to the COSMIC database, many of these gene fusions had been previously identified in different types of cancers, including (I) BCR-ABL1, CLTC-ALK, ETV6-ABL1 and RANBP2-ALK in haematopoietic and lymphoid-related cancers, (II) EML4-ALK in lung and thyroid cancers, (III) STRN-ALK in lung, peritoneum and thyroid cancers, (IV) RANBP2-ALK, TPM3-ALK and ATIC-ALK in soft, haematopoietic and lymphoid-related cancers, and (V) NTRK1-TPM3 in thyroid and colorectal cancers. Among these nine fusion genes, seven involved the ALK (ALK receptor tyrosine kinase) gene.
Table 3
Type | Total | Putative oncogenic | New | New + putative oncogenic |
---|---|---|---|---|
FACTERA | 324 | 0 | 324 | 0 |
GeneFuse_COSMIC | 67 | 18 | 67 | 18 |
GeneFuse_druggable | 150 | 59 | 129 | 40 |
Note: The “New” category covers those that were not included in COSMIC database, and oncogenic gene fusions were predicted by Oncofuse. The statistical figures did not eliminate duplicates, which meant that the same fusion events detected in many samples would be re-counted. So the unique gene fusion events would be less than the statistics shown in the figure.
After Oncofuse analysis, we discovered that 18 gene fusions (i.e., 16 unique events) involving COSMIC curated fusion genes and 59 gene fusions (i.e., 33 unique events) related to druggable fusion genes were potentially oncogenic (corrected P value <0.05) (Figure 3). Among these potentially oncogenic gene fusions, 16 events were detected in at least 2 PCa samples which were called as “recurrent gene fusions” (Table 4). From these, 5 gene fusions (BCR-ABL1, EML4-ALK, STRN-ALK, CLTC-ALK and ETV6-ABL1) were previously reported in the COSMIC database, while ETV6-ALK was identified in epithelioid fibrous histiocytoma (24). Ten recurrent oncogenic gene fusions were novel, among which three new fusion partners of oncogenic ALK were discovered (i.e., FRYL-ALK, ABL1-ALK, and BCR-ALK).
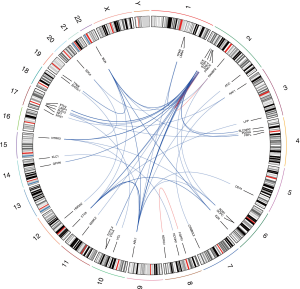
Table 4
Type | ID | bcr | no_bcr | Total (≥2) | P value | COSMIC |
---|---|---|---|---|---|---|
GeneFuse_COSMIC | FRYL:ALK | 1 | 1 | 2 | 0.001947 | N |
GeneFuse_druggable | BCR:ABL1 | 0 | 2 | 2 | 1.3E-05 | Y |
GeneFuse_druggable | ETV6:ALK | 1 | 1 | 2 | 5.57E-05 | N |
GeneFuse_druggable | EML4:ALK | 0 | 2 | 2 | 0.000138 | Y |
GeneFuse_druggable | BCR:ALK | 0 | 2 | 2 | 0.000161 | N |
GeneFuse_druggable | STRN:ALK | 0 | 2 | 2 | 0.000747 | Y |
GeneFuse_druggable | RANBP2:BCR | 0 | 2 | 2 | 0.00309 | N |
GeneFuse_druggable | TPM4:BCR | 0 | 2 | 2 | 0.009371 | N |
GeneFuse_druggable | BCR:EZR | 1 | 1 | 2 | 0.037034 | N |
GeneFuse_druggable | ETV6:EZR | 0 | 2 | 2 | 0.037034 | N |
GeneFuse_druggable | CLTC:ALK | 1 | 2 | 3 | 0.000247 | Y |
GeneFuse_druggable | EML4:NTRK3 | 0 | 3 | 3 | 8.99E-05 | N |
GeneFuse_druggable | GOPC:NTRK3 | 0 | 3 | 3 | 0.00241 | N |
GeneFuse_druggable | ABL1:ALK | 1 | 3 | 4 | 0.000138 | N |
GeneFuse_druggable | ABL1:STRN | 2 | 4 | 6 | 6.07E-05 | N |
GeneFuse_druggable | ETV6:ABL1 | 3 | 4 | 7 | 1.3E-05 | Y |
Note: “bcr” and “no_bcr” stand for recurrent prostate cancer group and non-recurrent prostate cancer group. The number below stands for the frequency of every gene fusion event in recurrent group and non-recurrent group. “P value” stems from OncoFuse and indicates whether this gene fusion is predicted to be oncogenic. “Y” and “N” in the last column indicate whether this gene fusion had been included in COSMIC database.
Discussion
We presently conducted an analysis of CNVs and gene fusion events in PCa, using WES data from primary tumors of 74 PCa patients. This genomic data was originated from 30 recurrent and 44 non-recurrent PCa cases, after 5 years of clinical follow-up. Finally, we identified 66 specific CNVs (2 deletions and 64 duplications) that were specific to primary tumor tissues from the recurrent PCa group. Most of the 64 duplication regions were located at 8q2, including 8q21, 8q22, 8q23, 8q24, indicating that this chromosomal region is an important duplication site for PCa. As previously reported, 8q24 duplication has been associated with MYC overexpression and PCa progression (22). In addition, this duplication event may serve an independent predictor for PCa recurrence after RP (22). The chromosomal 8q24 region has been also associated with certain aggressive forms of PCa (25). Our findings suggest that duplication regions may have occurred specifically in primary PCa tissues that were recurrent after 5-year follow-up. This observation indicates that the 8q2 duplication might be an early event that predisposes PCa to recurrence, and not solely an outcome of PCa recurrence. These duplication regions could be further used to develop assays to predict PCa recurrence, using primary cancer tissues.
Specifically, a high number of cancer-risk SNPs have been detected in the 8q24 region, which also harbors c-MYC (26,27). Another important gene located in the region is the prostate-specific noncoding RNA gene, PCaT-1, whose overexpression promotes proliferation, migration, invasion and inhibits apoptosis in PCa cells (28). In the recurrent PCa group, our data analyses have revealed that copy number gain of 8q2 includes other important genes, such as PTK2 (Protein Tyrosine Kinase 2), RAD21 (RAD21 Cohesin Complex Component) and KIAA0196 (WASHC5, WASH Complex Subunit 5). Based on a large validation cohort, PTK2 was duplicated in 1% of localized PCa and 35% of CRPC (castration resistant PCa). Interestingly, inhibition of PTK2 expression significantly affects cell proliferation and migration of PC3 PCa cells (29), suggesting that duplication of PTK2 might be associated with more aggressive PCa. Two additional genes from chromosomal 8q24—RAD21 and KIAA0196—have shown increased expression in PCa and were also duplicated in 30–40% of PCa xenografts and hormone-refractory tumors (30). Moreover, KIAA0196 expression appears to be significantly higher in tumors with gene duplication than those with no CNV. These data suggest that KIAA0196 and RAD21 are putative effector genes for the common duplication of 8q23-24 in PCa (30). The duplication of 8q2 region in recurrent PCa were consistent with our current understanding of the important roles of 8q2 in PCa. In addition, our findings also suggest a potential mechanism of action (MOA) of specific duplicated regions in PCa recurrence and prognosis, by enhancing the expression of genes in these particular chromosomal sites.
We have also identified a duplicated 1q41 region in the recurrent PCa group. This region harbors CENPF (Centromere Protein F). It has been reported that CENPF acts synergistically with FOXM1 to promote PCa growth, and co-expression of FOXM1 and CENPF is a robust prognostic indicator of poor survival and metastasis (31). Overexpression of CENPF has been related to higher Gleason grade, advanced pathological tumor stage, accelerated cell proliferation, and lymph node metastasis, which corroborate its application as a potential biomarker for PCa aggressiveness (32). In addition, up-regulation of CENPF is an independent predictor of poor BCR-free survival (33). Therefore, the copy number gain of CENPF in 1q41 might promote its expression and contribute to prostate recurrence.
Upon CNV analysis, we have also detected several common copy number gain/loss in both the recurrent and the non-recurrent PCa, including CHD1 deletion. As previously reported, CHD1 loss sensitizes PCa to DNA damaging therapy by promoting error-prone double-strand break repair (34). Loss of CHD1 causes defects on DNA repair and enhances the responsiveness to PCa therapy. In fact, CHD1 gene loss may serve as a marker for PCa patient stratification for targeted therapies, such as PARP inhibitors, which specifically affect tumors with HR (homologous recombination) defects (35). In summary, these common CNVs potentially shed light on the mechanism(s) of prostate carcinogenesis.
Remarkably, we have also discovered a total of 324 gene fusion events by FACTERA. Furthermore, we identified 67 gene fusions related to COSMIC curated fusion genes, and 150 gene fusions related to druggable fusion genes, respectively, by GeneFuse (Table 3). We have additionally found fusion events involving the ALK gene, which was the most common type of gene fusion in our data. In this case, a total of 7 recurrent oncogenic ALK fusion events were annotated, where four of these (EML4-ALK, STRN-ALK, CLTC-ALK, ETV6-ALK) have been previously identified in other types of cancers, and the remaining three fusions (FRYL-ALK, ABL1-ALK, and BCR-ALK) are novel events. These seven ALK fusion events were identified, for the first time, in PCa.
ALK is a receptor tyrosine kinase (RTK) that was first identified in anaplastic large-cell lymphoma (ALCL), due to a characteristic NPM-ALK fusion event (36,37). Since then, oncogenic ALK fusions have been identified in several types of cancer. Therefore, treatment with ALK inhibitors has been further considered in cancer therapy. ALK gene fusions have also been implicated in the pathogenesis of many kinds of tumors, including anaplastic large cell lymphomas, non-small cell lung cancer (38), melanomas (39), thyroid cancer (40), renal cell carcinoma (41), colorectal adenocarcinoma (42) and others (43). For instance, EML4-ALK translocation can lead to constitutive ALK kinase activity and represents an oncogenic addiction pathway in lung cancer (38). Intriguingly, ALK fusions have not been previously reported in PCa.
So far, multiple fusion partners of ALK have been identified in anaplastic large cell lymphomas (ALCLs) and inflammatory myofibroblastic tumors (IMTs), including nucleophosmin (NPM), 5-aminoimidazole-4-carboxamide ribonucleotide formyltransferase/IMP cyclohydrolase (ATIC), tropomyosin 3 (TPM3), tropomyosin 4 (TPM4), clathrin heavy chain (CLTC), myosin heavy chain 9 (MYH), and the TRK-fused gene (TFG) (44). Renal cell carcinoma (RCC) also contains multiple ALK fusion partners, such as vinculin (VCL), tropomyosin 3 (TPM3), echinoderm microtubule associated protein-like 4 (EML4), hook microtubule tethering protein 1 (HOOK1) and striatin (STRN) genes (41). In particular, we have previously reported that the ALK gene is truncated (mostly by 5' deletion) in 18 of 281 (6.4%) Chinese PCa cases (45), however, no ALK fusion partners were identified.
In our current study, we have detected three novel ALK fusion events, including FRYL:ALK. FRY-like transcription coactivator (FRYL) is a putative target of miR-1205, which is encoded by the long non-coding PVT1 gene located at 8q24, a PCa susceptibility chromosomal region (46). Indeed, transfection of oligonucleotide mimics of miR-1205 into androgen-independent PC3 cells can lead to a significant decrease in the expression of FRYL, suggesting that FRYL may act as a direct target of miR-1205. Loss of miR-1205 promotes a tumorigenic phenotype in PCa (46). Interestingly, we have observed that the FRYL:ALK fusion event negatively affects the binding site of miR-1205 in 3’UTR of the FRYL gene. Therefore, we concluded that FRYL:ALK gene fusion might protect FRYL expression from miR-1205-dependent inhibition, contributing to PCa tumorigenesis due to elevated FRYL expression.
Conclusions
Here we demonstrated that WES is a cost effective approach to identify CNVs and gene fusion events in PCa. Our findings that 8q2 duplication specifically occurs in primary PCa samples that were recurrent, after 5-year follow-up, suggests that this particular duplication is an early genetic event that predispose PCa to recurrence. Meanwhile, a random forest model with most informative CNV events (n=6) were developed. This model could effectively predict PCa recurrence, with an accuracy close to 90%. Furthermore, we found a high frequency of ALK gene fusion events in our dataset. Interestingly, all seven recurrent oncogenic ALK fusion events were here identified, for the first time, in PCa. Altogether, these findings expand our understanding of prostate carcinogenesis and may lead to the development of more specific assays focused on the prediction of PCa recurrence.
Acknowledgments
Funding: The work was funded by
Footnote
Conflicts of Interest: All authors have completed the ICMJE uniform disclosure form (available at http://dx.doi.org/10.21037/tcr.2020.03.31). BL reports other from Hangzhou Proprium Biotech Co. Ltd, outside the submitted work. The other authors have no conflicts of interest to declare.
Ethical Statement: The authors are accountable for all aspects of the work in ensuring that questions related to the accuracy or integrity of any part of the work are appropriately investigated and resolved. This study has been conducted in accordance with the ethical standards according to the Declaration of Helsinki and the national and international guidelines, and it was also approved by ethics review board of the First Affiliated Hospital of Zhejiang University (No. 2012-42) and Shaoxing People’s Hospital (No. 2016-48). Written informed consent was obtained from all of the participants when enrolled in the study.
Open Access Statement: This is an Open Access article distributed in accordance with the Creative Commons Attribution-NonCommercial-NoDerivs 4.0 International License (CC BY-NC-ND 4.0), which permits the non-commercial replication and distribution of the article with the strict proviso that no changes or edits are made and the original work is properly cited (including links to both the formal publication through the relevant DOI and the license). See: https://creativecommons.org/licenses/by-nc-nd/4.0/.
References
- Zare F, Dow M, Monteleone N, et al. An evaluation of copy number variation detection tools for cancer using whole exome sequencing data. BMC Bioinformatics 2017;18:286. [Crossref] [PubMed]
- Kadalayil L, Rafiq S, Rose-Zerilli MJ, et al. Exome sequence read depth methods for identifying copy number changes. Brief Bioinform 2015;16:380-92. [Crossref] [PubMed]
- Yang L, Lee MS, Lu H, et al. Analyzing Somatic Genome Rearrangements in Human Cancers by Using Whole-Exome Sequencing. Am J Hum Genet 2016;98:843-56. [Crossref] [PubMed]
- Saramäki OR, Porkka KP, Vessella RL, et al. Genetic aberrations in prostate cancer by microarray analysis. Int J Cancer 2006;119:1322-9. [Crossref] [PubMed]
- Yu YP, Song C, Tseng G, et al. Genome abnormalities precede prostate cancer and predict clinical relapse. Am J Pathol 2012;180:2240-8. [Crossref] [PubMed]
- Camacho N, Van Loo P, Edwards S, et al. Appraising the relevance of DNA copy number loss and gain in prostate cancer using whole genome DNA sequence data. PLoS Genet 2017;13:e1007001. [Crossref] [PubMed]
- Tomlins SA, Rhodes DR, Perner S, et al. Recurrent fusion of TMPRSS2 and ETS transcription factor genes in prostate cancer. Science 2005;310:644-8. [Crossref] [PubMed]
- Ratz L, Laible M, Kacprzyk LA, et al. TMPRSS2:ERG gene fusion variants induce TGF-beta signaling and epithelial to mesenchymal transition in human prostate cancer cells. Oncotarget 2017;8:25115-30. [Crossref] [PubMed]
- Kulda V, Topolcan O, Kucera R, et al. Prognostic Significance of TMPRSS2-ERG Fusion Gene in Prostate Cancer. Anticancer Res 2016;36:4787-93. [Crossref] [PubMed]
- Nam RK, Sugar L, Yang W, et al. Expression of the TMPRSS2:ERG fusion gene predicts cancer recurrence after surgery for localised prostate cancer. Br J Cancer 2007;97:1690-5. [Crossref] [PubMed]
- Kong DP, Chen R, Zhang CL, et al. Prevalence and clinical application of TMPRSS2-ERG fusion in Asian prostate cancer patients: a large-sample study in Chinese people and a systematic review. Asian J Androl 2020;22:200-7. [Crossref] [PubMed]
- Gümrükcü G, Celik BO, Caliskan S, et al. The positive immunostaining of TMPRSS2-ERG is not associated with unfavourable outcomes and biochemical recurrence after radical prostatectomy in Turkish patients. Cent European J Urol 2018;71:276-79. [PubMed]
- Lalonde E, Alkallas R, Chua MLK, et al. Translating a Prognostic DNA Genomic Classifier into the Clinic: Retrospective Validation in 563 Localized Prostate Tumors. Eur Urol 2017;72:22-31. [Crossref] [PubMed]
- Oh JJ, Park S, Lee SE, et al. Genetic risk score to predict biochemical recurrence after radical prostatectomy in prostate cancer: prospective cohort study. Oncotarget 2017;8:75979-88. [Crossref] [PubMed]
- Campbell JM, Raymond E, O'Callaghan ME, et al. Optimum Tools for Predicting Clinical Outcomes in Prostate Cancer Patients Undergoing Radical Prostatectomy: A Systematic Review of Prognostic Accuracy and Validity. Clin Genitourin Cancer 2017;15:e827-e834. [Crossref] [PubMed]
- Fromer M, Purcell SM. Using XHMM Software to Detect Copy Number Variation in Whole-Exome Sequencing Data. Curr Protoc Hum Genet 2014;81:7.23.1-21.
- Quinlan AR, Hall IM. BEDTools: a flexible suite of utilities for comparing genomic features. Bioinformatics 2010;26:841-2. [Crossref] [PubMed]
- Newman AM, Bratman SV, Stehr H, et al. FACTERA: a practical method for the discovery of genomic rearrangements at breakpoint resolution. Bioinformatics 2014;30:3390-3. [Crossref] [PubMed]
- Chen S, Liu M, Huang T, et al. GeneFuse: detection and visualization of target gene fusions from DNA sequencing data. Int J Biol Sci 2018;14:843-8. [Crossref] [PubMed]
- Shugay M, Ortiz de Mendibil I, Vizmanos JL, et al. Oncofuse: a computational framework for the prediction of the oncogenic potential of gene fusions. Bioinformatics 2013;29:2539-46. [Crossref] [PubMed]
- Liu J, Mao R, Ren G, et al. Whole Exome Sequencing Identifies Putative Predictors of Recurrent Prostate Cancer with High Accuracy. OMICS 2019;23:380-8. [Crossref] [PubMed]
- Fromont G, Godet J, Peyret A, et al. 8q24 amplification is associated with Myc expression and prostate cancer progression and is an independent predictor of recurrence after radical prostatectomy. Hum Pathol 2013;44:1617-23. [Crossref] [PubMed]
- Huang S, Gulzar ZG, Salari K, et al. Recurrent deletion of CHD1 in prostate cancer with relevance to cell invasiveness. Oncogene 2012;31:4164-70. [Crossref] [PubMed]
- Dickson BC, Swanson D, Charames GS, et al. Epithelioid fibrous histiocytoma: molecular characterization of ALK fusion partners in 23 cases. Mod Pathol 2018;31:753-62. [Crossref] [PubMed]
- Pal P, Xi H, Guha S, et al. Common variants in 8q24 are associated with risk for prostate cancer and tumor aggressiveness in men of European ancestry. Prostate 2009;69:1548-56. [Crossref] [PubMed]
- Hudson BD, Kulp KS, Loots GG. Prostate cancer invasion and metastasis: insights from mining genomic data. Brief Funct Genomics 2013;12:397-410. [Crossref] [PubMed]
- Tong Y, Yu T, Li S, et al. Cumulative Evidence for Relationships Between 8q24 Variants and Prostate Cancer. Front Physiol 2018;9:915. [Crossref] [PubMed]
- Xu W, Chang J, Du X, et al. Long non-coding RNA PCAT-1 contributes to tumorigenesis by regulating FSCN1 via miR-145-5p in prostate cancer. Biomed Pharmacother 2017;95:1112-8. [Crossref] [PubMed]
- Menon R, Deng M, Ruenauver K, et al. Somatic copy number alterations by whole-exome sequencing implicates YWHAZ and PTK2 in castration-resistant prostate cancer. J Pathol 2013;231:505-16. [Crossref] [PubMed]
- Porkka KP, Tammela TL, Vessella RL, et al. RAD21 and KIAA0196 at 8q24 are amplified and overexpressed in prostate cancer. Genes Chromosomes Cancer 2004;39:1-10. [Crossref] [PubMed]
- Aytes A, Mitrofanova A, Lefebvre C, et al. Cross-species regulatory network analysis identifies a synergistic interaction between FOXM1 and CENPF that drives prostate cancer malignancy. Cancer Cell 2014;25:638-51. [Crossref] [PubMed]
- Göbel C, Ozden C, Schroeder C, et al. Upregulation of centromere protein F is linked to aggressive prostate cancers. Cancer Manag Res 2018;10:5491-504. [Crossref] [PubMed]
- Zhuo YJ, Xi M, Wan YP, et al. Enhanced expression of centromere protein F predicts clinical progression and prognosis in patients with prostate cancer. Int J Mol Med 2015;35:966-72. [Crossref] [PubMed]
- Shenoy TR, Boysen G, Wang MY, et al. CHD1 loss sensitizes prostate cancer to DNA damaging therapy by promoting error-prone double-strand break repair. Ann Oncol 2017;28:1495-507. [Crossref] [PubMed]
- Kari V, Mansour WY, Raul SK, et al. Loss of CHD1 causes DNA repair defects and enhances prostate cancer therapeutic responsiveness. EMBO Rep 2018; [Crossref] [PubMed]
- Elmberger PG, Lozano MD, Weisenburger DD, et al. Transcripts of the npm-alk fusion gene in anaplastic large cell lymphoma, Hodgkin's disease, and reactive lymphoid lesions. Blood 1995;86:3517-21. [Crossref] [PubMed]
- Morris SW, Kirstein MN, Valentine MB, et al. Fusion of a kinase gene, ALK, to a nucleolar protein gene, NPM, in non-Hodgkin's lymphoma. Science 1994;263:1281-4. [Crossref] [PubMed]
- Du X, Shao Y, Qin HF, et al. ALK-rearrangement in non-small-cell lung cancer (NSCLC). Thorac Cancer 2018;9:423-30. [Crossref] [PubMed]
- Couts KL, Bemis J, Turner JA, et al. ALK Inhibitor Response in Melanomas Expressing EML4-ALK Fusions and Alternate ALK Isoforms. Mol Cancer Ther 2018;17:222-31. [Crossref] [PubMed]
- Bastos AU, de Jesus AC, Cerutti JM. ETV6-NTRK3 and STRN-ALK kinase fusions are recurrent events in papillary thyroid cancer of adult population. Eur J Endocrinol 2018;178:83-91. [Crossref] [PubMed]
- Kuroda N, Sugawara E, Kusano H, et al. A review of ALK-rearranged renal cell carcinomas with a focus on clinical and pathobiological aspects. Pol J Pathol 2018;69:109-13. [Crossref] [PubMed]
- Yakirevich E, Resnick MB, Mangray S, et al. Oncogenic ALK Fusion in Rare and Aggressive Subtype of Colorectal Adenocarcinoma as a Potential Therapeutic Target. Clin Cancer Res 2016;22:3831-40. [Crossref] [PubMed]
- Holla VR, Elamin YY, Bailey AM, et al. ALK: a tyrosine kinase target for cancer therapy. Cold Spring Harb Mol Case Stud 2017;3:a001115. [Crossref] [PubMed]
- Wong DW, Leung EL, Wong SK, et al. A novel KIF5B-ALK variant in nonsmall cell lung cancer. Cancer 2011;117:2709-18. [Crossref] [PubMed]
- Song R, Ren G, Liu X, et al. Alterations of ALK gene and protein expression in prostatic cancer and its clinical significance. Zhonghua Bing Li Xue Za Zhi 2015;44:382-5. [PubMed]
- Durojaiye V, Ilboudo A, Levine F, et al. Abstract 187: miR-1205/FRYL as a novel regulatory mechanism in androgen-independent prostate cancer. Cancer Res 2015;75:187.