Exploring metabolomics biomarkers for evaluating the effectiveness of concurrent radiochemotherapy for cervical cancers
Introduction
Cervical cancer is the second most common gynecological malignancy worldwide, with an estimated 570,000 new cases and 311,000 deaths in 2018, thus making it a major public health problem (1). Approximately two-thirds of patients in developing countries are already in a locally advanced tumor stage when diagnosed (2). The main treatment for patients with locally advanced stage is concurrent chemoradiotherapy (CCRT), followed by evaluation of the effectiveness of therapy by imaging and tumor biomarkers (3,4). However, the assessment function of imaging for the primary and tiny lymph node metastases lesions after treatment, as well as the sensitivity and specificity of tumor biomarkers such as squamous cell carcinoma antigen are not sufficient to meet the clinical needs (5,6).
With the development of metabolomics, the previously dismissed notion of cancer as a metabolic disease has regained prominence (7,8). In recent studies, metabolomics was used to discuss the diagnosis of cervical cancer and the effectiveness of neoadjuvant chemotherapy, which provided scientific evidence for the further study (9). However, the application of this approach in the effectiveness of CCRT has not been reported. Ultra-performance liquid chromatography with quadrupole time-of-flight mass spectrometry (UPLC-Q-TOF/MS) was performed to describe the metabolic profiles of primary, post-CCRT, and recurrent cervical cancer patients and to identify potential biomarkers for evaluating the effectiveness of CCRT and disease progression of cervical cancer patients to provide scientific evidence for providing timely treatment.
Methods
Patients
This study was approved by the Ethics Committee at Harbin Medical University in Harbin, China. From Harbin Medical University Cancer Hospital, the patients were enrolled who met the following inclusion criteria: they had been diagnosed with stage IB–IIIB cervical squamous cell carcinoma (CSCC) via physical examination, histopathology, and imaging tests; were primary patients who had no prior cervical cancer-related treatment and the following treatment was CCRT, or were patients diagnosed with local recurrence or distant metastasis; had no other malignant tumors; and had no other metabolic diseases, like diabetes. The exclusion criteria included: the inability to undergo imaging tests; missing clinical data; drop-out or changed treatment during the study period. Written informed consent was obtained from each enrolled patient before participation in the study.
Treatment regimen
Eligible primary patients received 5–6 cycles of CCRT. External beam radiotherapy was performed with a dose of 1.8 Gy per fraction five times per week with a total dose of 45–51 Gy, and the dose was increased to 63.5 Gy in areas which were lymph-node-positive. Intra-cavity brachytherapy was performed to point A (a reference location 2 cm lateral and 2 cm superior to the cervical os) one time per week during external beam radiotherapy with a total dose was 30 Gy. Meanwhile, platinum-based drugs were administered at a dose of 40 mg once per week. The treatment regimens of recurrent patients were developed by clinicians according to the patients’ personal situation.
Response evaluation
The response evaluation of primary patients was based on the response evaluation criteria in solid tumors (version 1.1) and was classified as follows: complete response signified that all target lesions had disappeared; partial response was defined as at least 30% decrease in the sum of the largest diameter of the target lesions; stable disease signified that there had been no significant decrease in the size of target lesions, based on the smallest sum of the largest diameter; progressive disease was defined as at least 20% increase in the sum of the largest diameter of the target lesions.
Plasma samples
The plasma samples were collected from all patients on admission and from primary patients after CCRT. After fasting and avoiding medication and alcohol for 12 h, 5 mL of whole blood from each patient was collected into a heparin sodium anticoagulation tube. Fresh blood was centrifuged at 3,000 rpm for 10 min at room temperature, and the supernatant was collected and frozen at −80 °C until analysis.
Quality control samples
To ensure the stability and repeatability of the UPLC-Q-TOF/MS system, quality control samples that were prepared by mixing equal volumes of different individual plasma samples were used in both electrospray ionization positive and negative modes. One quality control sample was run after every eight test samples.
Sample pretreatment
After thawing at room temperature, the plasma sample (80 µL) was transferred into a 1.5-mL centrifuge tube, mixed with 10 µL internal standard (L-2-chlorophenylalanine, 0.3 mg/mL), and vortexed for 10 s. Next, 240 µL of a mixture of methanol and acetonitrile (1:1) was prepared and vortexed for 1 min. The mixture was then ultrasound extracted in an ice water bath for 10 min and allowed to stand at −20 °C for 30 min. After centrifugation for 15 min at 4 °C, the supernatant (200 µL) was transferred into an LC vial and stored at −80 °C until injection analysis.
Chromatography
A pretreated sample (5 µL) was injected into an ACQUITY UPLC BEH C18 column (100× 2.1 mm, id. 1.7 µm; Waters, Milford, USA) using the ACQUITY UPLC system (Waters, Milford, USA). The mobile phase was a mixture of water containing 0.1% formic acid (A) and acetonitrile containing 0.1% formic acid (B). The elution gradient was as follows: 5–20% B for 0–2 min; 20–60% B for 2–4 min; 60–100% B for 4–11 min; 11–13 min, held at 100% B; 13–13.5 min, decreased to 5% B; and 13.5–14.5 min, held at 5% B. The mobile phase flow rate was 0.40 mL·min−1 at 45 °C.
Mass spectrometry
Mass spectrometry was performed using an AB Triple TOF 5600 (AB Sciex, USA) equipped in both electrospray ionization modes. The ion spray voltage was set at 5.5 kV for positive mode and 4.5 kV for negative mode. The ion source temperature was 550 °C, and the centroid data were collected in full scan mode from 70 to 1,000 m/z.
Data preprocessing
The raw data files were imported to Progenesis QI software (Waters, Milford, USA) for preprocessing, which produced a matrix containing retention time, m/z values, and peak area, after baseline filtering, peak identification, integration, retention time correction, peak alignment, and normalization. In the matrix, the unique variable identification (ID) was generated with the combination of retention time and m/z values in order to distinguish metabolites with significant differences in both retention time modes.
Statistical analysis
After preprocessing, the matrix was imported into SIMCA software (Umetrics, Umeå, Sweden). Unsupervised principle component analysis (PCA) was used to visualize the overall distribution of the samples and the stability of the analysis process. Then, the supervised partial least squares-discriminant analysis (PLS-DA) and orthogonal projection to latent structures discriminant analysis (OPLS-DA) were performed to determine the global metabolic differences between the two groups. Corresponding variable importance in the projection values were calculated in the OPLS-DA model. To prevent overfitting, the 200-response permutation test of the OPLS-DA model was conducted to evaluate the quality of the model, and the main parameter analyzed was Q2. If the intercept of Q2 was found to negative, it was considered that the model lacked overfitting.
Biomarker identification and selection
The differential metabolites that were selected using the criteria of variable importance in the projection >1 and P<0.05 were identified by comparison of exact m/z values and MS/MS spectra with the structural information of the metabolites obtained from Human Metabolome Database (http://www.hmdb.ca/) and LIPID MAPS (http://www.lipidmaps.org/). A Venn diagram was drawn based on ID to screen for the differential metabolites among the three groups. The metabolites with high variable importance in the projection values were retained if repeated in both modes. Receiver operating characteristic analysis was performed using MetaboAnalyst 4.0 to screen for the differential metabolites. The metabolites which had an area under curve value above 0.75 were considered as potential markers, and the multivariate receiver operating characteristic analysis of these potential markers was conducted based on PLS-DA.
Pathway analysis
Pathway analysis was conducted with MetaboAnalyst 4.0 and KEGG (https://www.genome.jp/kegg/pathway.html). Pathways with P<0.05 were considered significantly enriched pathways.
Results
Demographic and clinical characteristics
Between November 2016 and November 2017, 24 primary patients and 30 recurrent patients were enrolled in this prospective study. According to the response evaluation criteria in solid tumors, 11 of the 24 primary patients were diagnosed as complete responses and 13 as partial responses after CCRT. The baseline characteristics were comparable in each group except for the squamous cell carcinoma antigen levels (shown in Table 1).
Table 1
Characteristics | Primary | Post-CCRT | Recurrent | P value | |
---|---|---|---|---|---|
CR | PR | ||||
Number of subjects | 24 | 11 | 13 | 30 | |
Age () | 53.5±8.3 | 56.6±10.0 | 50.9±5.7 | 53.3±8.6 | 0.425 |
BMI () | 25.2±4.3 | 26.7±5.8 | 23.9±2.1 | 24.0±2.6 | 0.145 |
Marry age () | 22.6±3.1 | 21.6±3.1 | 23.6±3.0 | 22.5±2.8 | 0.475 |
Pregnancy times () | 2.8±1.6 | 2.8±1.6 | 2.8±1.6 | 3.2±1.6 | 0.685 |
Births times () | 1.5±1.0 | 1.8±1.3 | 1.2±0.6 | 1.6±0.7 | 0.447 |
Abortion times () | 1.3±1.4 | 1.0±1.0 | 1.5±1.7 | 1.7±1.5 | 0.524 |
Primiparous age () | 23.7±2.9 | 22.9±3.1 | 24.3±2.8 | 23.5±2.9 | 0.690 |
Amenorrhea (yes/no) | 13/11 | 8/3 | 5/8 | 13/17 | 0.300 |
FIGO stage | 0.121 | ||||
I | 0 | 0 | 0 | 5 | |
II | 17 | 9 | 8 | 16 | |
III | 7 | 2 | 5 | 9 | |
Lymphatic metastasis (yes/no) | 11/13 | 4/7 | 7/6 | 14/16 | 0.864 |
Squamous carcinoma | 24 | 11 | 13 | 30 | |
SCC-Ag () | 12.7±16.2 | 0.9±0.2 | 1.0±0.5 | 5.8±10.9 | 0.008 |
Hemoglobin () | 116.9±24.6 | 109.7±10.4 | 109.7±15.2 | 119.9±11.3 | 0.194 |
BMI, body mass index; FIGO, the International Federation of Gynecology and Obstetrics; SCC-Ag, squamous cell carcinoma antigen; CCRT, concurrent chemoradiotherapy; CR, complete response; PR, partial response.
Plasma metabolic profiles
Metabolic profiles of all samples
The PCA score plot (Figure S1) to visualize the metabolic profiles of all samples revealed that the QC samples were clustered densely. Because no clear separation was found between CR and PR, these two groups were combined into one group (post-CCRT) for the following analysis.
Metabolic profiles of primary vs. post-CCRT patients
Although the PCA score plot (Figure 1A) showed some overlap, the PLS-DA and OPLS-DA score plots revealed excellent classifications between primary and post-CCRT patients (Figure 1B,C). A validation plot obtained from 200 permutation tests (Figure 1D) showed that all permuted R2 and Q2 values on the left were lower than the original values on the right, and the Q2 regression line in blue had a negative intercept, indicating that the model prevented overfitting and it was stable and credible. According to the VIP >1 and P<0.05 criteria, 395 differential metabolites between primary and post-CCRT patients were identified, including 231 metabolites in the ESI+ mode and 164 in the ESI- mode, of which 30 metabolites were duplicates.
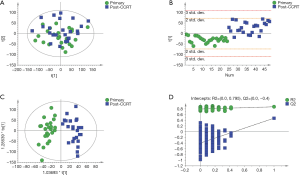
Metabolic profiles of primary vs. recurrent patients
There was no clear separation between primary and recurrent patients in the PCA score plot (Figure 2A), but the PLS-DA and OPLS-DA score plots indicated that these two groups were markedly divided into two categories (Figure 2B,C). The validation plot strongly supported the validity of the established OPLS-DA model (Figure 2D). A total of 529 metabolites were significantly different between primary and recurrent patients, including 321 ions in ESI+ mode and 208 in ESI- mode, of which 43 metabolites were duplicates.
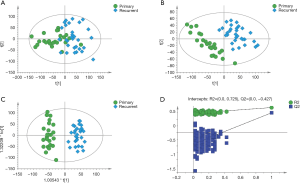
Metabolic profiles of post-CCRT vs. recurrent patients
As shown in the PCA, PLS-DA and OPLS-DA score plots, there were clear differences between post-CCRT and recurrent patients (Figure 3A,B,C), and the validity of the OPLS-DA model was supported by the result of validation plots (Figure 3D). Based on the same criteria, there were 557 differential metabolites (325 metabolites in ESI+ mode and 232 in ESI− mode, of which 35 were duplicates) between post-CCRT and recurrent patients.
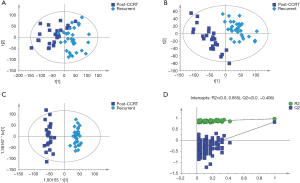
Selection and dynamic changes of potential biomarkers
A Venn diagram (Figure 4) showed that a total of 39 metabolites were significantly different among the three groups. After removing the duplicates, the clustering results indicated that these 37 metabolites could clearly distinguish between two groups (Figure S2). The detailed information of these metabolites is listed in Table 2, and the dynamic changes in their levels are shown in Figure 5. Twenty-one metabolites in primary patients showed a significant increase after CCRT, which were lowest in recurrent patients (Figure 5A), whereas one metabolite, N-acetylornithine, showed the opposite trend (Figure 5B). Among these 22 metabolites, the areas under curve of the following 8 metabolites: ceramide [Cer (d18:1/16:0)], phosphatidylcholine [PC (15:0/16:0)], PC (16:0/16:0), phosphatidylethanolamine [PE (16:0/20:0)], PC (14:0/20:0), phosphatidylserine [PS (17:0/22:2(13Z,16Z))], phosphatidylglycerol [PG (21:0/22:4(7Z,10Z,13Z,16Z))] and sphingomyelin [SM (d18:1/20:0)], were above 0.75 (shown in Table 2). As shown in Figure S3, the combined areas under curve were 0.854, 0.936, and 0.983 in primary versus post-CCRT patients, primary versus recurrent patients, and post-CCRT versus recurrent patients, respectively.
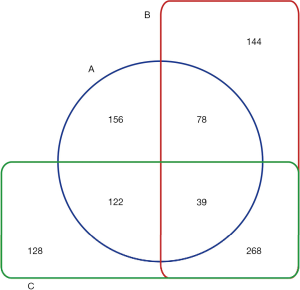
Table 2
Metabolites | m/z | Retention time (min) | Mass error (ppm) | Primary vs. post-CCRT | Primary vs. recurrent | Post-CCRT vs. recurrent | ||||||||
---|---|---|---|---|---|---|---|---|---|---|---|---|---|---|
VIP | P value | AUC | VIP | P value | AUC | VIP | P value | AUC | ||||||
ESI+ | ||||||||||||||
N-Acetylornithine | 175.108 | 0.711 | 0.623 | 1.083 | 0.034 | 0.667 | 1.237 | 0.001 | 0.779 | 1.937 | <0.001 | 0.892 | ||
Neuraminic acid* | 268.103 | 1.547 | 2.419 | 2.003 | 0.003 | 0.788 | 1.981 | <0.001 | 0.994 | 1.547 | <0.001 | 0.949 | ||
N-(6-aminohexanoyl)-6-aminohexanoic acid | 245.185 | 1.939 | −2.277 | 1.108 | 0.022 | 0.648 | 1.582 | <0.001 | 0.979 | 1.469 | <0.001 | 0.924 | ||
Ceramide (d18:1/16:0)* | 560.502 | 10.120 | 0.550 | 2.365 | <0.001 | 0.906 | 1.258 | 0.001 | 0.781 | 2.217 | <0.001 | 0.968 | ||
DG [14:1(9Z)/22:2(13Z,16Z)/0:0] | 641.512 | 10.599 | 0.335 | 1.856 | 0.010 | 0.705 | 1.030 | 0.006 | 0.753 | 1.768 | <0.001 | 0.846 | ||
PC (O-14:0/18:0) | 742.572 | 10.840 | -0.521 | 1.389 | 0.010 | 0.693 | 1.501 | <0.001 | 0.775 | 2.054 | <0.001 | 0.897 | ||
Methyl 8-[2-(2-formyl-vinyl)-3-hydroxy-5-oxo-cyclopentyl]-octanoate | 333.166 | 3.844 | −2.719 | 1.120 | 0.041 | 0.667 | 1.364 | <0.001 | 0.779 | 1.959 | <0.001 | 0.861 | ||
dodecanamide | 200.200 | 5.193 | −2.754 | 1.276 | 0.035 | 0.653 | 1.997 | <0.001 | 0.893 | 1.072 | 0.003 | 0.726 | ||
octadec-11Z-enol | 286.310 | 5.420 | −1.761 | 1.322 | 0.031 | 0.675 | 1.050 | 0.011 | 0.729 | 1.699 | <0.001 | 0.857 | ||
1-Heneicosene | 312.362 | 6.041 | −1.697 | 1.236 | 0.022 | 0.691 | 2.074 | <0.001 | 0.947 | 1.924 | <0.001 | 0.896 | ||
PE (16:0/0:0) | 454.293 | 6.769 | −0.246 | 1.700 | 0.010 | 0.741 | 1.633 | <0.001 | 0.817 | 1.010 | 0.012 | 0.676 | ||
Loperamide | 459.219 | 7.483 | −2.482 | 1.204 | 0.048 | 0.691 | 1.725 | <0.001 | 0.832 | 1.108 | 0.005 | 0.735 | ||
Cer (d18:0/18:0) | 568.566 | 7.990 | −0.274 | 1.196 | 0.021 | 0.722 | 1.912 | <0.001 | 0.942 | 1.311 | 0.001 | 0.876 | ||
1alpha,25-dihydroxy-3-deoxy-3-thiavitamin D3 3-oxide | 457.272 | 8.401 | 2.219 | 1.342 | 0.035 | 0.681 | 1.980 | <0.001 | 0.926 | 1.854 | <0.001 | 0.868 | ||
3beta-hydroxy-4beta-methyl-5alpha-cholest-7-ene-4alpha-carboxylic acid | 445.367 | 9.085 | −1.505 | 1.326 | 0.047 | 0.667 | 1.859 | <0.001 | 0.863 | 1.404 | <0.001 | 0.796 | ||
3-oxoglycyrrhetinic acid | 486.358 | 9.085 | 0.124 | 1.772 | 0.008 | 0.688 | 1.984 | <0.001 | 0.897 | 1.304 | 0.001 | 0.750 | ||
1-(6-[3]-ladderane-hexanoyl)-2-(8-[3]-ladderane-octanyl)-sn-glycero-3-phospho-(1'-sn-glycerol) | 759.496 | 9.133 | −0.111 | 1.694 | 0.041 | 0.649 | 1.862 | <0.001 | 0.861 | 1.178 | 0.003 | 0.744 | ||
G (17:2(9Z,12Z)/20:1(11Z)/20:4(5Z,8Z,11Z,14Z))[iso6] | 919.777 | 9.435 | 2.753 | 1.671 | 0.031 | 0.672 | 1.946 | <0.001 | 0.885 | 1.168 | 0.002 | 0.714 | ||
TG (17:2(9Z,12Z)/22:3(10Z,13Z,16Z)/22:5(7Z,10Z,13Z,16Z,19Z))[iso6] | 986.764 | 9.435 | 0.512 | 2.073 | 0.012 | 0.745 | 2.069 | <0.001 | 0.900 | 1.143 | 0.004 | 0.693 | ||
LacCer (d18:1/16:0) | 884.607 | 9.490 | 0.507 | 1.066 | 0.035 | 0.686 | 2.455 | <0.001 | 0.967 | 1.900 | <0.001 | 0.878 | ||
PC (24:0/24:1(15Z)) | 478.906 | 9.819 | 1.382 | 1.570 | 0.027 | 0.677 | 1.903 | <0.001 | 0.876 | 1.098 | 0.004 | 0.707 | ||
ESI- | ||||||||||||||
PC (15:0/16:0)* | 718.540 | 10.289 | 0.506 | 2.456 | <0.001 | 0.858 | 1.457 | <0.001 | 0.807 | 2.285 | <0.001 | 0.956 | ||
PC (16:0/16:0)* | 778.561 | 10.289 | 1.511 | 2.416 | <0.001 | 0.859 | 1.566 | <0.001 | 0.838 | 2.349 | <0.001 | 0.963 | ||
Cer (d18:0/16:0) | 584.526 | 10.406 | −0.560 | 1.046 | 0.050 | 0.649 | 1.578 | <0.001 | 0.872 | 1.528 | <0.001 | 0.904 | ||
PI (20:2(11Z,14Z)/22:6(4Z,7Z,10Z,13Z,16Z,19Z)) | 979.553 | 10.468 | −2.947 | 2.914 | <0.001 | 0.847 | 1.175 | 0.006 | 0.724 | 2.153 | <0.001 | 0.918 | ||
PC (15:0/20:5(5Z,8Z,11Z,14Z,17Z)) | 810.527 | 10.562 | −2.481 | 1.925 | 0.001 | 0.792 | 1.023 | 0.010 | 0.746 | 1.802 | <0.001 | 0.914 | ||
DG (14:1(9Z)/22:2(13Z,16Z)/0:0) | 663.521 | 10.596 | 0.241 | 1.942 | 0.006 | 0.712 | 1.142 | 0.003 | 0.760 | 1.839 | <0.001 | 0.881 | ||
PE (O-18:0/16:0) | 704.561 | 10.844 | 0.892 | 1.381 | 0.009 | 0.698 | 1.813 | <0.001 | 0.861 | 2.192 | <0.001 | 0.929 | ||
PE (O-18:0/17:0) | 764.582 | 10.844 | 0.579 | 1.434 | 0.009 | 0.700 | 1.885 | <0.001 | 0.883 | 2.245 | <0.001 | 0.946 | ||
PE (16:0/20:0)* | 746.571 | 11.199 | 0.986 | 2.126 | <0.001 | 0.859 | 1.312 | 0.001 | 0.763 | 2.111 | <0.001 | 0.938 | ||
PC (14:0/20:0)* | 806.592 | 11.199 | 0.980 | 2.152 | <0.001 | 0.872 | 1.414 | <0.001 | 0.792 | 2.168 | <0.001 | 0.963 | ||
PS(17:0/22:2(13Z,16Z))* | 874.580 | 11.199 | −1.811 | 2.155 | <0.001 | 0.861 | 1.361 | <0.001 | 0.785 | 2.165 | <0.001 | 0.958 | ||
PG (21:0/22:4(7Z,10Z,13Z,16Z))* | 913.617 | 11.303 | −0.760 | 1.952 | 0.002 | 0.760 | 1.665 | <0.001 | 0.883 | 2.084 | <0.001 | 0.904 | ||
SM (d18:2/24:1) | 855.661 | 11.309 | 1.477 | 1.833 | 0.009 | 0.705 | 1.610 | <0.001 | 0.824 | 1.979 | <0.001 | 0.892 | ||
SM (d18:1/19:0) | 743.608 | 11.481 | 1.234 | 1.927 | 0.009 | 0.708 | 2.080 | <0.001 | 0.921 | 2.366 | <0.001 | 0.967 | ||
SM (d18:1/20:0)* | 803.629 | 11.481 | 1.016 | 2.361 | <0.001 | 0.764 | 2.183 | <0.001 | 0.946 | 2.458 | <0.001 | 0.981 | ||
PC (P-20:0/22:4(7Z,10Z,13Z,16Z)) | 894.661 | 11.534 | 2.006 | 1.197 | 0.041 | 0.660 | 1.259 | 0.001 | 0.765 | 1.745 | <0.001 | 0.849 | ||
LacCer (d18:1/16:0) | 906.617 | 9.481 | 1.005 | 1.561 | 0.004 | 0.724 | 2.506 | <0.001 | 0.981 | 2.170 | <0.001 | 0.925 | ||
PI (22:0/20:0) | 949.672 | 9.637 | −2.963 | 1.408 | 0.019 | 0.682 | 1.653 | <0.001 | 0.836 | 2.245 | <0.001 | 0.951 |
*, the metabolites with AUCs results above 0.75 between each pair of groups. CCRT, concurrent chemoradiotherapy; VIP, variable importance in the projection; AUC, area under curve.
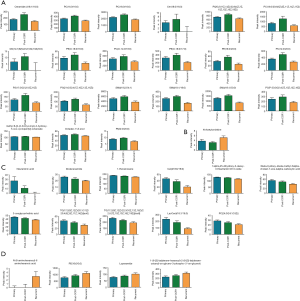
Pathway analysis
The 37 differential metabolites described above were used for pathway analysis, and 7 pathways were enriched, of which sphingolipid metabolism and glycerophospholipid metabolism were enriched significantly based on Impact >0.1 (Figure 6 and Table S1).
Discussion
In this study, we collected the plasma samples from primary patients both upon admission and after CCRT. To reduce the heterogeneity of plasma samples between groups, patients who were absent for the follow-up were excluded. Because the use of metabolomics approaches limits the collection time of the biological samples, we were unable to follow-up the patients to observe the long-term prognostic outcome. Thus, the plasma samples of patients with locally recurrent or distant metastases were collected to eliminate the effects of treatment on metabolites and to screen tumor biomarkers more clearly.
Although no clear separation was found between complete response and partial response, the plasma metabolic profiles were obviously different among primary, post-CCRT (complete response combined with partial response) and recurrent cervical cancer patients with locally advanced stages. After multivariate analyses, there were 37 metabolites which were different between the two groups. Among them, the levels of 22 metabolites, such as Cer (d18:1/16:0) and N-acetylornithine, were changed significantly, and they recovered or even exceeded the levels in primary patients when the tumor reappeared. Therefore, the dynamic changes of these 22 metabolites might be caused by tumor.
Among the 22 metabolites mentioned above, 8 metabolites with AUCs above 0.75 between each pair of groups, namely Cer (d18:1/16:0), PC (15:0/16:0), PC (16:0/16:0), PE (16:0/20:0), PC (14:0/20:0), PS [17:0/22:2(13Z,16Z)], PG [21:0/22:4(7Z,10Z,13Z,16Z)] and SM (d18:1/20:0), demonstrated great potential for evaluating the effectiveness of CCRT and disease progression. All of these 8 metabolites were phospholipids, which have many important biological functions, such as material transportation, energy metabolism, and signal transduction (10-12). Recent studies have shown that abnormal phospholipid metabolism was closely related to tumor occurrence, development, invasion, and metastasis (13,14).
These 8 metabolites included 6 glycerophospholipids and 2 sphingolipids, and they primarily enriched the metabolic pathways of glycerophospholipid metabolism and sphingolipid metabolism, respectively. Glycerophospholipid, the most abundant phospholipid in biological organisms, is a key component of the cell membrane and an important bioactive substance in the body (15,16). The different kinds of glycerophospholipids form a metabolic network under the regulation of various enzymes, in which the phosphatidylcholine and phosphatidylethanolamine are mainly synthesized under the action of phosphocholine cytidylyltransferase and cholinephosphotransferase, and phosphatidylethanolamine can produce phosphatidylcholine under the action of phosphatidylethanolamine N-methyltransferase (17,18). Therefore, the content of these types of glycerophospholipids is significantly correlated. Phosphatidylcholine can produce arachidonic acid through deacylation under the action of cytosolic phospholipase A2 (19). Previous studies showed that the expression cytosolic phospholipase A2 was significantly increased in tumor cells, resulting in a decrease in phosphatidylcholine and an increase in arachidonic acid (20), whereas arachidonic acid and its downstream products had a role in promoting tumor proliferation, invasion and metastasis (21,22).
Sphingolipids are commonly found in cell membranes and apolipoproteins, and are classified into sphingomyelin, glycosphingolipid, and ceramide. Ceramide could be generated by sphingomyelin under the action of sphingomyelinase, which is involved in many cell functions as a secondary messenger, including inhibition of cell proliferation and promotion of apoptosis (23,24). The metabolites of sphingomyelin include ceramide, sphingosine, and sphingosine-1-phosphate, which are transformed into each other in cells. Cer can be hydrolyzed by ceramidase to form sphingosine, whereas sphingosine can produce sphingosine-1-phosphate under the action of sphingosine kinases, and sphingosine-1-phosphate can be reversely converted into ceramide. The synthesis and decomposition of these three products constitute a dynamic equilibrium relationship, called ‘sphingolipid rheostat’, in which ceramide and sphingosine have the effect of inhibiting cell growth and promoting apoptosis, whereas sphingosine-1-phosphate has the opposite effect (25). When this equilibrium relationship is hampered, the normal apoptosis mechanism of cells is disturbed owing to the reduction of ceramide content, which promotes the development of tumors (26). The dynamic changes observed in the corresponding products in the present study were consistent with the results of previous studies. Hence, the formation and recurrence of tumors might be promoted by the inhibition of sphingolipid and glycerophospholipid metabolisms in cervical cancer.
Of the 22 metabolites which were significant between the three groups, only N-acetylornithine showed an opposite trend to others, with a significant decrease after CCRT and an increase in recurrence. N-acetylornithine, which mainly exists in human plasma, could be converted into ornithine under the action of deacetylase; the level of ornithine is negatively correlated with tumor occurrence, that is, its level is reduced in cancer patients (27). The metabolic pathway-arginine and proline metabolism, which was enriched by N-acetylornithine in this study—is involved in the tumor hypoxic microenvironment and is significantly changed in cancer patients (28). In the process of proliferation and metastasis of tumor cells, a large amount of proline—an important component of cell membrane is produced, and proline oxidase, which is the first enzyme in the proteolytic pathway of proline, can inhibit cell proliferation and promote apoptosis (29). Thus, attenuating the synthesis of arginine and proline could inhibit the growth and proliferation of tumor cells. The dynamic changes of N-acetylornithine in this study were consistent with the results of previous studies.
Strengths and limitations
The study was a combination of prospective and cross-sectional design, and this was the first study to examine plasma metabolic changes in response to cervical cancer with post-CCRT and recurrence. There were several limitations in this study. One problem is that the metabolomics data of primary and recurrent patients were generated from different populations. But we have tried our best to improve the comparability of samples during collection. In addition, a targeted metabolomics investigation is needed to verify the potential biomarkers in order to advance clinical translation in the future.
Conclusions
In summary, we found that plasma metabolic profiles significantly differed among the three groups of patients with primary, post-CCRT, and recurrent cervical cancers. The 8 metabolites, namely Cer (d18:1/16:0), PC (15:0/16:0), PC (16:0/16:0), PE (16:0/20:0), PC (14:0/20:0), PS [17:0/22:2(13Z,16Z)], PG [21:0/22:4(7Z,10Z,13Z,16Z)] and SM (d18:1/20:0), were screened as potential biomarkers to evaluate the effectiveness of CCRT and disease progression of cervical cancer patients. The key metabolic pathways involved were of sphingolipid and glycerophospholipid metabolism. These findings may be invaluable in the clinic for accurately evaluating the prognosis of patients and providing timely treatment.
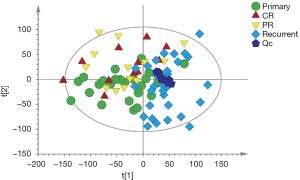
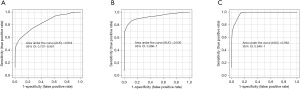
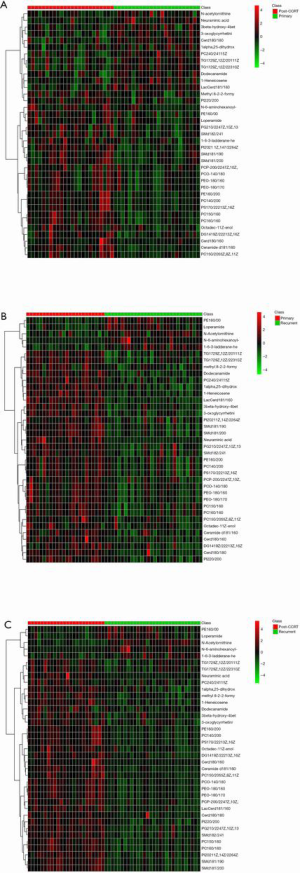
Table S1
Pathway | Total | Hits | Raw P | FDR | Impact |
---|---|---|---|---|---|
Sphingolipid metabolism | 25 | 3 | <0.001 | 0.004 | 0.304 |
Glycerophospholipid metabolism | 39 | 2 | 0.007 | 0.269 | 0.228 |
Glycosylphosphatidylinositol (GPI)-anchor biosynthesis | 14 | 1 | 0.046 | 0.977 | 0.044 |
Linoleic acid metabolism | 15 | 1 | 0.049 | 0.977 | 0 |
alpha-Linolenic acid metabolism | 29 | 1 | 0.093 | 1 | 0 |
Arachidonic acid metabolism | 62 | 1 | 0.189 | 1 | 0 |
Arginine and proline metabolism | 77 | 1 | 0.229 | 1 | 0.004 |
FDR, false discovery rate.
Acknowledgments
The authors would like to show their sincere gratitude to all the faculty members in their departments and all the patients and their families involved in this study.
Funding: This study was supported by
Footnote
Data Availability: The metadata of metabolomics reported in this study is available via https://doi.org/10.6084/m9.figshare.7262195.v3
Conflicts of Interest: All authors have completed the ICMJE uniform disclosure form (available at http://dx.doi.org/10.21037/tcr.2020.02.49). The authors have no conflicts of interest to declare.
Ethical Statement: The authors are accountable for all aspects of the work in ensuring that questions related to the accuracy or integrity of any part of the work are appropriately investigated and resolved. All procedures performed in studies involving human participants were in accordance with the ethical standards of the ethics committee at Harbin Medical University in Harbin, China and with the 1964 Helsinki declaration and its later amendments or comparable ethical standards. This article does not contain any studies with animals performed by any of the authors. Informed consent was obtained from all individual participants included in the study.
Open Access Statement: This is an Open Access article distributed in accordance with the Creative Commons Attribution-NonCommercial-NoDerivs 4.0 International License (CC BY-NC-ND 4.0), which permits the non-commercial replication and distribution of the article with the strict proviso that no changes or edits are made and the original work is properly cited (including links to both the formal publication through the relevant DOI and the license). See: https://creativecommons.org/licenses/by-nc-nd/4.0/.
References
- Bray F, Ferlay J, Soerjomataram I, et al. Global cancer statistics 2018: GLOBOCAN estimates of incidence and mortality worldwide for 36 cancers in 185 countries. CA Cancer J Clin 2018;68:394-424. [Crossref] [PubMed]
- Bazaz M, Shahry P, Latifi SM, et al. Cervical Cancer Literacy in Women of Reproductive Age and Its Related Factors. J Cancer Educ 2019;34:82-9. [Crossref] [PubMed]
- Chen P, Jiao L, Wang DB. Squamous cell carcinoma antigen expression in tumor cells is associated with the chemosensitivity and survival of patients with cervical cancer receiving docetaxel-carboplatin-based neoadjuvant chemotherapy. Oncol Lett 2017;13:1235-41. [Crossref] [PubMed]
- Dappa E, Elger T, Hasenburg A, et al. The value of advanced MRI techniques in the assessment of cervical cancer: a review. Insights Imaging 2017;8:471-81. [Crossref] [PubMed]
- Dag Z, Yilmaz B, Dogan AK, et al. Comparison of the prognostic value of F-18 FDG PET/CT metabolic parameters of primary tumors and MRI findings in patients with locally advanced cervical cancer treated with concurrent chemoradiotherapy. Brachytherapy 2019;18:154-62. [Crossref] [PubMed]
- Oh J, Bae JY. Optimal cutoff level of serum squamous cell carcinoma antigen to detect recurrent cervical squamous cell carcinoma during post-treatment surveillance. Obstet Gynecol Sci 2018;61:337-43. [Crossref] [PubMed]
- Poljsak B, Kovac V, Dahmane R, et al. Cancer Etiology: A Metabolic Disease Originating from Life's Major Evolutionary Transition? Oxid Med Cell Longev 2019;2019:7831952. [Crossref] [PubMed]
- Wishart DS. Is Cancer a Genetic Disease or a Metabolic Disease? EBioMedicine 2015;2:478-79. [Crossref] [PubMed]
- Khan I, Nam M, Kwon M, et al. LC/MS-Based Polar Metabolite Profiling Identified Unique Biomarker Signatures for Cervical Cancer and Cervical Intraepithelial Neoplasia Using Global and Targeted Metabolomics. Cancers (Basel) 2019;11:511. [Crossref] [PubMed]
- Chmielewski M, Carrero JJ, Stenvinkel P, et al. Metabolic abnormalities in chronic kidney disease that contribute to cardiovascular disease, and nutritional initiatives that may diminish the risk. Curr Opin Lipidol 2009;20:3-9. [Crossref] [PubMed]
- Mascitelli L, Seneff S, Goldstein MR. Association of Alzheimer disease pathology with abnormal lipid metabolism: the Hisayama study. Neurology 2012;78:151-52. [Crossref] [PubMed]
- Negro F. Abnormalities of lipid metabolism in hepatitis C virus infection. Gut 2010;59:1279-87. [Crossref] [PubMed]
- Long J, Zhang CJ, Zhu N, et al. Lipid metabolism and carcinogenesis, cancer development. Am J Cancer Res 2018;8:778-91. [PubMed]
- Maan M, Peters JM, Dutta M, et al. Lipid metabolism and lipophagy in cancer. Biochem Biophys Res Commun 2018;504:582-89. [Crossref] [PubMed]
- Bogani G, Ditto A, Martinelli F, et al. Impact of Blood Transfusions on Survival of Locally Advanced Cervical Cancer Patients Undergoing Neoadjuvant Chemotherapy Plus Radical Surgery. Int J Gynecol Cancer 2017;27:514-22. [Crossref] [PubMed]
- Gargiulo P, Arenare L, Pisano C, et al. Long-Term Toxicity and Quality of Life in Patients Treated for Locally Advanced Cervical Cancer. Oncology 2016;90:29-35. [Crossref] [PubMed]
- Wu L, Yu HG. The role of phosphatidylethanolamine N-methyltransferase pathway in liver diseases. Chinese Journal of Difficult and Complicated Cases 2017;16:1288-91.
- Sa RN, Wu HJ. Pathways of lipid metabolism and their associated disorders in cancer. Cancer Research on Prevention and Treatment 2016;43:907-12.
- You JC, Yang J, Fang RP, et al. Analysis of Phosphatidylcholines (PCs) and Lysophosphatidylcholines (Lyso PCs) in Metastasis of Breast Cancer Cells. Prog Biochem Biophys 2015;42:563-73.
- Cai H, Chiorean EG, Chiorean MV, et al. Elevated phospholipase A2 activities in plasma samples from multiple cancers. PLoS One 2013;8:e57081. [Crossref] [PubMed]
- Benesch MG, Ko YM, McMullen TP, et al. Autotaxin in the crosshairs: taking aim at cancer and other inflammatory conditions. FEBS Lett 2014;588:2712-27. [Crossref] [PubMed]
- Nimptsch A, Pyttel S, Paasch U, et al. A MALDI MS investigation of the lysophosphatidylcholine/phosphatidylcholine ratio in human spermatozoa and erythrocytes as a useful fertility marker. Lipids 2014;49:287-93. [Crossref] [PubMed]
- Nganga R, Oleinik N, Ogretmen B. Mechanisms of Ceramide-Dependent Cancer Cell Death. Adv Cancer Res 2018;140:1-25. [Crossref] [PubMed]
- Ogretmen B. Sphingolipid metabolism in cancer signalling and therapy. Nat Rev Cancer 2018;18:33-50. [Crossref] [PubMed]
- Newton J, Lima S, Maceyka M, et al. Revisiting the sphingolipid rheostat: Evolving concepts in cancer therapy. Exp Cell Res 2015;333:195-200. [Crossref] [PubMed]
- Zalewska A, Maciejczyk M, Szulimowska J, et al. High-Fat Diet Affects Ceramide Content, Disturbs Mitochondrial Redox Balance, and Induces Apoptosis in the Submandibular Glands of Mice. Biomolecules 2019;9:877. [Crossref] [PubMed]
- Hu L, Gao Y, Cao Y, et al. Identification of arginine and its "Downstream" molecules as potential markers of breast cancer. IUBMB Life 2016;68:817-22. [Crossref] [PubMed]
- Tsai IL, Kuo TC, Ho TJ, et al. Metabolomic Dynamic Analysis of Hypoxia in MDA-MB-231 and the Comparison with Inferred Metabolites from Transcriptomics Data. Cancers 2013;5:491-510. [Crossref] [PubMed]
- Liang Q, Wang C, Li B. Metabolomic analysis using liquid chromatography/mass spectrometry for gastric cancer. Appl Biochem Biotechnol 2015;176:2170-84. [Crossref] [PubMed]