The association between genomic variations and histological grade in hepatocellular carcinoma
Introduction
Histological grade (HG) describes the aggressive potential of solid tumors. The classical and widely adopted grading system for hepatocellular carcinoma is Edmondson-Steiner (ES), which is based on microscopic evaluation of the tubule formation, mitotic count, and nuclear pleomorphism. According to the ES grading system, tumors can be classified into three or four grades. A tumor of a higher grade tends to grow and spread at a faster pace, which needs more urgent and aggressive treatment.
Needle biopsies and histopathological evaluation works as a gold standard for HG diagnosis. Collectively, clinical physicians have accumulated a large amount of experience in using this method. However, it has two major problems, diagnostic subjectivity and biopsy inaccessibility (1), which might hinder its full efficacy. A stricter tumor grading requires two or more pathologists with expertise in a specific cancer to reduce diagnostic subjectivity. Much effort has been made with non-invasive methods such as magnetic resonance and contrast computed tomography (CT) to avoid biopsy unavailability (2). In contrast to diverse imaging methods, molecular biomarkers could overcome the two problems mentioned above. For example, miR-1290 could work as a biomarker of high-grade serous ovarian carcinoma (3), and tumor tissue protein signatures could predict the HG of breast cancer (4). Apart from the expression of biomarkers as an indicator of HG, the genomic variation could also be used. Many gene mutations have been recognized to be associated with HG, such as TP53 (5), IDH1/2 (6) and ACVR2 (7). We are interested to know how far genomic variations are associated with HG in HCC because it can help us to understand HG as an important clinical measure.
With the development of precision medicine, DNA sequencing provides rich information for disease diagnosis and precision treatment. By using a liquid biopsy, genomic variations could prove to be more useful in predicting HG and could perfectly overcome the two major problems in the traditional ES grading system. Additionally, this method could provide necessary information for precise treatment in one-shot sequencing. However, ctDNA concentration is more easily affected by cancer development and clinical therapy, and the standard to detect ctDNA from liquid biopsy has not been well established.
This study sequenced 487 tissue samples from 459 Chinese HCC patients to build a solid connection between genomic variation and HG. Genomic variation, including nucleotide substitution/indel, truncation, gene homozygous deletion and fusion, were called. Association of HG with factors including genomic variation types, substitution types, mutational frequency related scores and biological processes was studied. Among the factors, those found to be significant were compared to those of the Western population.
Methods
Patients and samples
This study was approved by Shandong Provincial Hospital Affiliated to Shandong University and The Affiliated Hospital of Qingdao University. A total of 602 patients were enrolled. Each participant provided written informed consent. Samples were collected from surgery after diagnosis or relapse. HG was scored by a specialist in hepatobiliary pathology according to the Edmondson and Steiner method (8). Grade 1 was defined as well differentiated (WD), grade 2 and 3 as moderate differentiated (MD), grade 4 as poor differentiated (PD). The patients were staged according to the seventh edition of the tumor-node-metastasis (TNM) classification system for lung cancer from the American Joint Committee. We also collected another public dataset to validate our analysis. This dataset, MSKCC, containing 360 samples, was downloaded from cBioportal (https://www.cbioportal.org/, accessed on March 5, 2019).
Library preparation and next-generation sequencing
Tissue samples (40 µm section) were collected for each patient. KAPA Hyper Prep Kit (#07962363001, Roche, Basel, Switzerland) was used to extract DNA. PBS (phosphate-buffered saline) was added to those samples with volumes of less than 5 mL in order to make each sample volume equivalent to 5 mL. They were centrifuged (2 times at 1,600 g for 10 and 15 min, respectively) for extraction of DNA and the supernatant was separated. Invitrogen Qubit® DNA HS Assay Kit (#Q32854) was used to measure the DNA concentration. Single strand DNA and protein contamination were excluded. Library construction was only applied in samples with at least 50 ng of double-stranded DNA extracted. Molecular identifiers (MIDs) were added to the DNA segment ends for DNA libraries to reduce the false discovery rate (FDR). Barcodes were also added to the reads for multiplex sequencing. Sequencing was performed on an Illumina Novaseq 6000 (Illumina, San Diego, CA) for 151 bp read length from both ends. The average sequencing depth was about 3,000×.
Variants calling
A pan-cancer panel (Yuansuo®, Origimed, Shanghai, China) comprising 588 genes was captured with targeted amplification. Adaptors were trimmed from raw DNA reads by cutadapt (version 1.18) (9). MID-labeled reads were de-duplicated with an in-house pipeline. BWA MEM (version 0.7.9a) (10) mapped the high-quality reads to the UCSC hg19 reference sequences. Base quality was recalibrated by the BaseRecalibrator tool from GATK (version 3.8) (11). Mutect2 with a tumor-only mode (12) and Varscan (version 2.3.9) (13) with the default parameters were used to call variants.
For each sample, the germline variants having variant allele frequency (VAF) <0.1% were filtered according to the databases of ExAC (14), gnomAD (15), 1000 Genomes (16), and ESP6500 (17). Somatic variants that had not been filtered were further annotated by ANNOVAR (2017/07/17) (18) with RefSeq (version 2017/06/01).
Fermi-lite (19) was used to identify gene fusion and rearrangement. The breakpoints were further checked by BLAT (http://genome.ucsc.edu, version 3.50). Those reads uniquely mapping to the reference genome constituted rearrangement supported reads.
CNVKit (20) was used to estimate the logR scores. Copy number was assigned 1 for logR values below −0.25, 3 for logR values above 0.25, and 2 for logR values in between −0.25 and 0.25 (21).
Bioinformatics analysis
The mutant allele tumor heterogeneity (MATH) score for a tumor was calculated as the median absolute deviation divided by the median MAF of all somatic mutations detected in the tumor sample. As suggested by Jiang et al. (22), the calculation of MATH used somatic mutation calls with MAF of 0.075 or greater. Clonal mutation burden (CMB) (22) was defined as the number of mutations per clone, and divided into low (low TMB, high MATH), high (high TMB, low MATH), or intermediate (others).
Statistical analysis
The Mann-Whitney U test was used to compare TMB, MSAF, MATH, and CMB between different HGs. Fisher’s exact test was performed to compare the count number of nucleotide mutations for different HGs.
Survival analysis was conducted with R software. Samples were classified by a cutoff at the median mutational frequency. The survival time was plotted against overall survival probability by the Kaplan-Meier method. The log-rank test was applied to calculate the P value between the two groups.
Results
Patients and genomic variation detection
The analysis workflow of this study is illustrated in Figure S1. Initially, a total of 602 patients were enrolled in this study. Of these, only 459 patients had histological grading information available. The other patients’ samples were thus filtered out from the following study. Their clinicopathologic characteristics are summarized in Table S1. The median age of patients was 55 years old (range, 16 to 82 years old). Most of the patients were male (87.6%). According to the TNM classification system (23), the number of patients in the early stage (I/II/III) and late-stage (IV) were 418 and 41, respectively. Patients who consumed alcohol more than 200 days per year were classified as “drinking”, and those who had an immediate family member with any type of cancer were labeled as “family history”. HGs were divided into three categories: poorly differentiated (PD), moderately differentiated (MD) and well differentiated (WD).
Tissue samples were prepared by surgery and enriched with a pan-cancer panel of genes (Yuansuo®, Origimed Co., Ltd, Shanghai, China) (Figure S2). For the 459 patients, 487 samples were collected. Somatic genomic variations (SGVs) were called by Mutec2 (12) and Varscan (13) for each sample. Gene amplifications were called by CNVKit (20). Fermi-lite (19) was used to identify gene fusion and rearrangement. The genomic variations at top high frequency are depicted in Figure 1. Genomic variations from multiple samples of each patient were merged under the same patient.
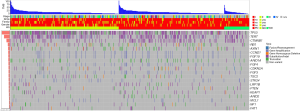
The bias of SGV types in different HGs
There are multiple types of SGVs deriving from different mechanisms. Those SGVs were classified into five types (fusion/rearrangement, gene amplification, gene homozygous deletion, substitution/indel, and truncation). The percentage of those groups was summarized according to the HG groups (Figure 2A). From poorly to moderately to well-differentiated HG, the percentage of the truncation and substitution/indel group increased but that of the gene amplification group dropped. The poorly differentiated group had the lowest percentage of fusion/rearrangement but the highest percentage of gene amplification variations.
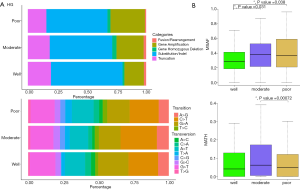
Single nucleotide variants (SNVs) can be classified into transversion substitution and transition substitution. Transition SNVs regularly had a higher frequency and caused no functional change because of codon “wobble”. To study the association between amino acid changes and HGs, the percentages of transversion and transition were compared (Figure 2A). In total, the percentage range of transversion and transition for different HGs was 65–72% and 28–34%, respectively. WD had higher transition than PD with percentages of 52% and 47%, respectively (P value =2.537e-11), but had lower transversion than PD with percentages of 48% and 53%, respectively (P value =2.2e-16). For specific substitutions, WD had less C>G transversion (P value =0.0058) and more G>A transition (P value =0.026) than non-WD. PD had higher C>T transition (P value =0.01) than non-PD.
Except for mutational occurrence, we also studied the association between HGs and mutational frequency related scores including maximum somatic allele frequency (MSAF) (24), MATH and CMB (22). MSAF was regularly used as a measure of cellular tumor prevalence. Higher MSAF denoted higher tumor content. The MATH score denoted allele heterogeneity among each sample, which reflected the diversity of mutational clones. CMB score combined the tumor mutation burden (TMB) and MATH score (22). High CMB was defined as high TMB and low MATH. These scores were compared among different HGs. WD had significantly lower MSAF than MD and PD with P values equal to 0.031 and 0.038, respectively (Figure 2B). As for the MATH score, MD was highest among HGs, but only MD transversion. PD had a P value of less than 0.05. We also tested the CMB score, but no significant difference was found among HGs (result not shown).
The functional bias of genomic variations for different HGs
Driver genes play a big part in cancer. Their specific effect on HG was also studied. The driver genes of HCC were collected from the literature (25,26). The top 10 variable genes are displayed in Figure 3A. Genes TP53, TERT, CTNNB1, RB1, AXIN1, and ARID1A were prone to substitution/indel/truncation variation, while CCND1, FGF19, FGF4, and FGF3 preferred gene amplification. Among those driver genes, there were three genes showing significantly different HGs after mutation (Figure 3B). Of these three genes, mutational TP53 (TP53+) had a higher average HG than non-mutational TP53 (TP53−). A non-parameter Wilcoxon’s rank-sum test showed significance at P value =3.8e-2. In contrast, mutational CTNNB1 (CTNNB1+) and FGF3 (FGF3+) showed significantly lower HG with P value =7.8e-4 and P value =0.04, respectively. We also tested the association between gene amplification variation and HG for those driver genes, but no significant difference was found (Figure 3C).
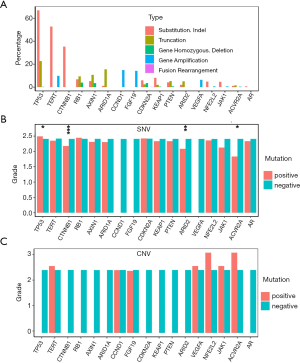
Mutations, such as substitution, indel, and truncation, can modify the targeted gene functions, and amplification can modify their expression. To study their functional bias, three HG groups were intersected with each other as displayed by a Venn plot in Figure 4A. WD, MD, and PD had 14, 57 and 62 unique mutated genes, respectively. WD had fewer unique mutated genes than other HGs. The unique genes for WD and PD were enriched with the biological processes of gene ontology. A hypergeometric test was performed for each biological process. The Bonferroni-Hochberg (BH) method was applied to correct for multiple testing errors. The top 10 enriched biological processes are listed in Figure 4B,C,D. WD was enriched in the regulation of the developmental process, cell differentiation, and membrane invagination. There were 1,168 biological processes enriched for PD specific mutations with multiple testing corrected P values less than 0.05, such as cell proliferation, protein phosphorylation, and cellular response to a stimulus.
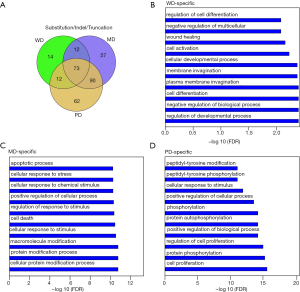
Apart from substitution/indel/truncation mutations, copy number variation can also disrupt cellular function by modifying gene regulation. The amplified genes were intersected with each other (Figure S3A) to obtain the HG-specific genes. The specific genes had similar distribution as substitution/indel/truncation for gene amplification. WD had less specific gene amplification than other HGs, while PD had the highest number of specific genes. WD was enriched in the regulation of fibroblast migration and the negative regulation of transport (Figure S3B); MD was enriched in the positive regulation of cellular processes and the regulation of cell proliferation (Figure S3C); and PD was enriched in the positive regulation of metabolic processes (Figure S3D).
Comparison to the Western population
The findings above were compared against the Western population. An MSKCC dataset from the Western population was downloaded from cBioportal (https://www.cbioportal.org/, accessed on March 5, 2019). With this dataset, nucleotide usage, TMB, driver genes, and biological processes were analyzed using the same procedures as in our dataset. Results showed that WD possessed a higher percentage of transition mutation than PD in the Western population. Meanwhile, PD held a higher percentage of transversion than WD. Such results were in line with those from our dataset. As for the nucleotide usage, only C>G transversion showed higher frequency in PD than in WD (P value =0.017), matching the result from our dataset. Specifically to the Western population, WD had higher A>G mutation than non-WD with P value =0.031. PD had higher A>C and lower A>G substitution than non-PD with P values =0.036 and 7.5e-4, respectively. Among the driver genes, only TP53 mutation was consistently associated with higher HG (P value =1.3e-3, Mann-Whitney U test). Additionally in the Western population, the RB1 mutation tended to be enriched in the high-grade samples.
Further investigation of the similarity between the functional biases for WD- and PD-specific genes revealed an extraordinary consistency. The top significantly enriched biological processes in our dataset showed similar significance in the MSKCC dataset (Figure 5A,B,C). For example, for both our dataset and the MSKCC dataset, PD-specific genes took part in cell proliferation, protein phosphorylation, and regulation of cell proliferation; MD-specific genes took part in the cellular protein modification process and cellular response to stimulus; and WD-specific genes taking part in the regulation of developmental processes and cell differentiation.
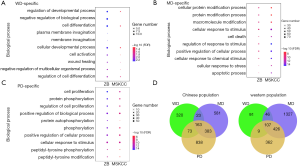
It was noteworthy that HG-specific genes may share common enriched biological processes (Figure 5D). To extract the consistent HG-specific biological processes between the two datasets, we first extracted the HG-specific biological processes taken by HG-specific genes for both datasets. Then an intersection was conducted between HG-specific biological processes for both datasets. Through these means, we identified the HG-specific biological processes commonly taken by both datasets. There were 3 WD-specific, 150 MD-specific and 64 PD-specific common biological processes (Table S2). These gene lists were applied for gene ontology enrichment analysis. The PD-specific common biological processes included angiogenesis, phosphatidylinositol-3-phosphate biosynthetic process, glycerophospholipid metabolic process and development of primary male sexual characteristics; the MD-specific common biological processes included response to hydrogen peroxide, response to peptide hormone and protein localization to the nucleus; and the three WD-specific common biological processes were regulation of epithelial to mesenchymal transition involved in endocardial cushion formations, epithelial to mesenchymal transition involved in endocardial cushion formations and regulation of homotypic cell-cell adhesion.
Discussion
Although there have been many studies on the association between gene expression and HG, information on the association between genomic variation and HG is still scarce. The intention of this study was to understand the association between genomic variation and HG and explore the potential of genomic variation as an indicator of HG.
A stable genomic variation pattern should be associated with a hidden molecular mechanism. For example, C>T and C>G substitution could come from DNA editing catalyzed by apolipoprotein B mRNA catalytic subunit-like (APOBEC) and activation-induced deaminase (AID) family, which can bind to both RNA and single-stranded (ss) DNA. DNA deamination by these proteins results in the C>U conversion in single-stranded DNA. Such mutations could result in C>T transition and C>G transversion by different DNA repair polymerases (27). In lung cancer, different cancer subtypes also showed a large difference in C>T transition and C>G transversion (28). Due to the existence of such molecular mechanisms, those stable genomic variation patterns could be stable predictors of HG. In this study, we have analyzed the association of HGs with genomic variation and mutational frequency in the Chinese population and the Western population, and have found a higher C>G transversion mutated in patients with PD HCC for both populations. This association was meaningful in the treatment of such a subset of HCC patients. As reported, APOBEC-related mutagenesis was found to be highly correlated with immunotherapy response (29). Thus, detected C>G transversion could be a good indicator of immunotherapy efficacy. In spite of high C>G transversion being found in HCC and believed as an etiology of HCC by Morishita et al. (30), they did not associate it with any biological significance. Our results revealed that patients with high C>G transversion were strongly associated with poorly differentiated HCC, involving in APOBEC-related mutagenesis.
Taking into account the important mutational scores in relation to survival, we also studied TMB, MSAF, MATH and CMB score, among which only MSAF is significantly associated with HG. As a measure of cellular tumor prevalence, MSAF has been used in many studies (24,31,32). Studies have shown that MSAF is also correlated with tumor burden (31) and several other research studies have revealed that tumor burden is strongly associated with HG (32). Therefore, it is reasonable that MSAF was significantly associated with HG.
Among the driver genes of HCC, TP53 mutation was a consistent biomarker of high HG in both populations, which agreed with the previous studies in ovarian cancer (5) and HCC (33). However, we also noticed difference between the Chinese and Western populations. In the Chinese population, mutations in CTNNB1, ARID2, and ACVR2A were associated with a lower HG, and in the western population, RB1 was associated with a high HG.
During the analysis of biological processes of substitution/indel/truncation and amplification for WD- and PD-specific genes, we found that the biological processes were highly matched for mutation and amplification. For example, WD tumors showed higher substitution/indel/truncation and amplification in the cell differentiation, and PD tumor showed higher substitution/indel/truncation and amplification in the protein phosphorylation. These results demonstrated that a tumor could become WD or PD either through mutations or by amplification, or both. Comparisons between the Chinese and the Western populations also proved that the WD was most enriched in cell differentiation, and the PD was most enriched in phosphorylation. Furthermore, there were also genes for WD or PD involved in phosphorylation or cell differentiation, respectively. A further intersection of their biological processes disclosed the unique biological processes for different HGs. Although these biological processes have been well recognized in basic cancer research, they have not been systematically associated with genomic variations and HG in HCC before.
It should be noted that, instead of ctDNA (circulating tumor DNA) from blood, DNA from the solid tumor was extracted to detect gene mutations. Considering the instability of ctDNA detection, this should be a very important step for applying genomic variations as a predictor of HG. For example, ctDNA is easier to be detected in late-stage cancer and its concentration can be changed by many factors including clinical therapy and tumor development. How the instability of ctDNA detection affects its prediction is another issue to be discussed. The other limitation of this study is that the comparison with the Western population did not include CNV due to the missing information in the MSKCC dataset.
In summary, this pilot study has revealed multiple factors associated with HG. These findings improved our understanding of the molecular mechanism in different HGs of HCC. Further research using ctDNA to detect the genomic variation should be performed to verify this study.
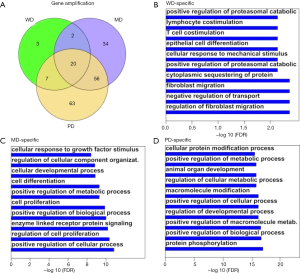
Table S1
Characteristics | Histological grade | |
---|---|---|
Poor/moderate | Well | |
Age (mean ± SD) | 54.9±15.0 | 60.7±12.5 |
Gender | ||
Male | 358 | 44 |
Female | 54 | 3 |
Stage | ||
I/II/III | 375 | 43 |
IV | 37 | 4 |
Drink | ||
Yes | 50 | 7 |
No | 362 | 40 |
Family | ||
Yes | 92 | 5 |
No | 320 | 42 |
Table S2
GOID | P values_adjusted | go_terms | Dataset | HG-specific |
---|---|---|---|---|
GO:0001525 | 1.264E-06 | Angiogenesis | ZB | PD |
GO:0036092 | 1.584E-05 | Phosphatidylinositol-3-phosphate biosynthetic process | MSKCC | PD |
GO:0006661 | 9.918E-05 | Phosphatidylinositol biosynthetic process | MSKCC | PD |
GO:0006650 | 0.0002442 | Glycerophospholipid metabolic process | ZB | PD |
GO:0036092 | 0.000248 | Phosphatidylinositol-3-phosphate biosynthetic process | ZB | PD |
GO:1902751 | 0.0002485 | Positive regulation of cell cycle G2/M phase transition | ZB | PD |
GO:0006650 | 0.0003463 | Glycerophospholipid metabolic process | MSKCC | PD |
GO:0006661 | 0.0007599 | Phosphatidylinositol biosynthetic process | ZB | PD |
GO:0006644 | 0.000767 | Phospholipid metabolic process | ZB | PD |
GO:0008584 | 0.0008151 | Male gonad development | MSKCC | PD |
GO:0046546 | 0.0008151 | Development of primary male sexual characteristics | MSKCC | PD |
GO:0006644 | 0.0010525 | Phospholipid metabolic process | MSKCC | PD |
GO:0046474 | 0.0010952 | Glycerophospholipid biosynthetic process | MSKCC | PD |
GO:0090218 | 0.0015026 | Positive regulation of lipid kinase activity | MSKCC | PD |
GO:0045017 | 0.0018219 | Glycerolipid biosynthetic process | MSKCC | PD |
GO:0019637 | 0.0019276 | Organophosphate metabolic process | ZB | PD |
GO:0007126 | 0.002289 | Meiotic nuclear division | ZB | PD |
GO:0008654 | 0.0023611 | Phospholipid biosynthetic process | MSKCC | PD |
GO:0043551 | 0.0026075 | Regulation of phosphatidylinositol 3-kinase activity | MSKCC | PD |
GO:1903046 | 0.0027839 | Meiotic cell cycle process | ZB | PD |
GO:1903727 | 0.0027864 | Positive regulation of phospholipid metabolic process | MSKCC | PD |
GO:0001525 | 0.0030352 | Angiogenesis | MSKCC | PD |
GO:0010518 | 0.0032304 | Positive regulation of phospholipase activity | ZB | PD |
GO:0030855 | 0.0040562 | Epithelial cell differentiation | MSKCC | PD |
GO:0035272 | 0.0042767 | Exocrine system development | MSKCC | PD |
GO:0043550 | 0.0042767 | Regulation of lipid kinase activity | MSKCC | PD |
GO:1902749 | 0.0046541 | Regulation of cell cycle G2/M phase transition | MSKCC | PD |
GO:0048146 | 0.0049414 | Positive regulation of fibroblast proliferation | MSKCC | PD |
GO:0033008 | 0.0050369 | Positive regulation of mast cell activation involved in immune response | ZB | PD |
GO:0043306 | 0.0050369 | Positive regulation of mast cell degranulation | ZB | PD |
GO:0046474 | 0.0050474 | Glycerophospholipid biosynthetic process | ZB | PD |
GO:0051321 | 0.0051928 | Meiotic cell cycle | ZB | PD |
GO:0043269 | 0.0052714 | Regulation of ion transport | MSKCC | PD |
GO:0014068 | 0.0059387 | Positive regulation of phosphatidylinositol 3-kinase signaling | ZB | PD |
GO:0033008 | 0.0062257 | Positive regulation of mast cell activation involved in immune response | MSKCC | PD |
GO:0043306 | 0.0062257 | Positive regulation of mast cell degranulation | MSKCC | PD |
GO:0033005 | 0.0066599 | Positive regulation of mast cell activation | ZB | PD |
GO:0045017 | 0.0071944 | Glycerolipid biosynthetic process | ZB | PD |
GO:0044839 | 0.0072853 | Cell cycle G2/M phase transition | MSKCC | PD |
GO:0014068 | 0.0073914 | Positive regulation of phosphatidylinositol 3-kinase signaling | MSKCC | PD |
GO:0002888 | 0.0080025 | Positive regulation of myeloid leukocyte mediated immunity | ZB | PD |
GO:0043302 | 0.0080025 | Positive regulation of leukocyte degranulation | ZB | PD |
GO:0033005 | 0.0082744 | Positive regulation of mast cell activation | MSKCC | PD |
GO:0008654 | 0.0087335 | Phospholipid biosynthetic process | ZB | PD |
GO:0034109 | 0.0088759 | Homotypic cell-cell adhesion | ZB | PD |
GO:0030855 | 0.0089828 | Epithelial cell differentiation | ZB | PD |
GO:1902751 | 0.0091779 | Positive regulation of cell cycle G2/M phase transition | MSKCC | PD |
GO:0006629 | 0.0093471 | Lipid metabolic process | MSKCC | PD |
GO:0008610 | 0.0100358 | Lipid biosynthetic process | MSKCC | PD |
GO:0002888 | 0.0100358 | Positive regulation of myeloid leukocyte mediated immunity | MSKCC | PD |
GO:0043302 | 0.0100358 | Positive regulation of leukocyte degranulation | MSKCC | PD |
GO:0034109 | 0.0114927 | Homotypic cell-cell adhesion | MSKCC | PD |
GO:0051656 | 0.0119185 | Establishment of organelle localization | ZB | PD |
GO:0043269 | 0.0125078 | Regulation of ion transport | ZB | PD |
GO:0060735 | 0.0129404 | Regulation of eif2 alpha phosphorylation by dsRNA | ZB | PD |
GO:0042102 | 0.0130323 | Positive regulation of T cell proliferation | ZB | PD |
GO:0030178 | 0.0141809 | Negative regulation of Wnt signaling pathway | ZB | PD |
GO:0006629 | 0.0150464 | Lipid metabolic process | ZB | PD |
GO:0051656 | 0.0165508 | Establishment of organelle localization | MSKCC | PD |
GO:0060735 | 0.0165508 | Regulation of eif2 alpha phosphorylation by dsRNA | MSKCC | PD |
GO:0007126 | 0.0170219 | Meiotic nuclear division | MSKCC | PD |
GO:0042102 | 0.0174305 | Positive regulation of T cell proliferation | MSKCC | PD |
GO:0090218 | 0.0181076 | Positive regulation of lipid kinase activity | ZB | PD |
GO:0032885 | 0.0187746 | Regulation of polysaccharide biosynthetic process | ZB | PD |
GO:0030178 | 0.0195602 | Negative regulation of Wnt signaling pathway | MSKCC | PD |
GO:1903046 | 0.0198476 | Meiotic cell cycle process | MSKCC | PD |
GO:0008610 | 0.020913 | Lipid biosynthetic process | ZB | PD |
GO:0032752 | 0.0213557 | Positive regulation of interleukin-3 production | ZB | PD |
GO:0042223 | 0.0213557 | Interleukin-3 biosynthetic process | ZB | PD |
GO:0043366 | 0.0213557 | Beta selection | ZB | PD |
GO:0045399 | 0.0213557 | Regulation of interleukin-3 biosynthetic process | ZB | PD |
GO:0045401 | 0.0213557 | Positive regulation of interleukin-3 biosynthetic process | ZB | PD |
GO:0007257 | 0.0216117 | Activation of JUN kinase activity | ZB | PD |
GO:0032881 | 0.0216117 | Regulation of polysaccharide metabolic process | ZB | PD |
GO:1902749 | 0.0218429 | Regulation of cell cycle G2/M phase transition | ZB | PD |
GO:0044839 | 0.0237717 | Cell cycle G2/M phase transition | ZB | PD |
GO:0033003 | 0.0243556 | Regulation of mast cell activation | ZB | PD |
GO:0043551 | 0.0243556 | Regulation of phosphatidylinositol 3-kinase activity | ZB | PD |
GO:1903727 | 0.0252945 | Positive regulation of phospholipid metabolic process | ZB | PD |
GO:0032885 | 0.0253146 | Regulation of polysaccharide biosynthetic process | MSKCC | PD |
GO:0009409 | 0.0262411 | Response to cold | ZB | PD |
GO:1903307 | 0.0262411 | Positive regulation of regulated secretory pathway | ZB | PD |
GO:0032752 | 0.0274151 | Positive regulation of interleukin-3 production | MSKCC | PD |
GO:0042223 | 0.0274151 | Interleukin-3 biosynthetic process | MSKCC | PD |
GO:0043366 | 0.0274151 | Beta selection | MSKCC | PD |
GO:0045399 | 0.0274151 | Regulation of interleukin-3 biosynthetic process | MSKCC | PD |
GO:0045401 | 0.0274151 | Positive regulation of interleukin-3 biosynthetic process | MSKCC | PD |
GO:0008584 | 0.0282742 | Male gonad development | ZB | PD |
GO:0046546 | 0.0282742 | Development of primary male sexual characteristics | ZB | PD |
GO:0002351 | 0.0282742 | Serotonin production involved in inflammatory response | ZB | PD |
GO:0002442 | 0.0282742 | Serotonin secretion involved in inflammatory response | ZB | PD |
GO:0002554 | 0.0282742 | Serotonin secretion by platelet | ZB | PD |
GO:0032252 | 0.0282742 | Secretory granule localization | ZB | PD |
GO:0032672 | 0.0282742 | Regulation of interleukin-3 production | ZB | PD |
GO:0045425 | 0.0282742 | Positive regulation of granulocyte macrophage colony-stimulating factor biosynthetic process | ZB | PD |
GO:0045588 | 0.0282742 | Positive regulation of gamma-delta T cell differentiation | ZB | PD |
GO:1901843 | 0.0282742 | Positive regulation of high voltage-gated calcium channel activity | ZB | PD |
GO:0007257 | 0.0285333 | Activation of JUN kinase activity | MSKCC | PD |
GO:0032881 | 0.0285333 | Regulation of polysaccharide metabolic process | MSKCC | PD |
GO:0042129 | 0.0307477 | Regulation of T cell proliferation | ZB | PD |
GO:0051321 | 0.0309104 | Meiotic cell cycle | MSKCC | PD |
GO:0035272 | 0.0318221 | Exocrine system development | ZB | PD |
GO:0043550 | 0.0318221 | Regulation of lipid kinase activity | ZB | PD |
GO:0033003 | 0.0322116 | Regulation of mast cell activation | MSKCC | PD |
GO:0048146 | 0.0343113 | Positive regulation of fibroblast proliferation | ZB | PD |
GO:0010897 | 0.0343113 | Negative regulation of triglyceride catabolic process | ZB | PD |
GO:0032632 | 0.0343113 | Interleukin-3 production | ZB | PD |
GO:0045423 | 0.0343113 | Regulation of granulocyte macrophage colony-stimulating factor biosynthetic process | ZB | PD |
GO:0060699 | 0.0343113 | Regulation of endoribonuclease activity | ZB | PD |
GO:0007405 | 0.0343407 | Neuroblast proliferation | ZB | PD |
GO:0009409 | 0.0344727 | Response to cold | MSKCC | PD |
GO:1903307 | 0.0344727 | Positive regulation of regulated secretory pathway | MSKCC | PD |
GO:0002351 | 0.0359801 | Serotonin production involved in inflammatory response | MSKCC | PD |
GO:0002442 | 0.0359801 | Serotonin secretion involved in inflammatory response | MSKCC | PD |
GO:0002554 | 0.0359801 | Serotonin secretion by platelet | MSKCC | PD |
GO:0032252 | 0.0359801 | Secretory granule localization | MSKCC | PD |
GO:0032672 | 0.0359801 | Regulation of interleukin-3 production | MSKCC | PD |
GO:0045425 | 0.0359801 | Positive regulation of granulocyte macrophage colony-stimulating factor biosynthetic process | MSKCC | PD |
GO:0045588 | 0.0359801 | Positive regulation of gamma-delta T cell differentiation | MSKCC | PD |
GO:1901843 | 0.0359801 | Positive regulation of high voltage-gated calcium channel activity | MSKCC | PD |
GO:0010518 | 0.040339 | Positive regulation of phospholipase activity | MSKCC | PD |
GO:0042129 | 0.0411271 | Regulation of T cell proliferation | MSKCC | PD |
GO:0010897 | 0.0437818 | Negative regulation of triglyceride catabolic process | MSKCC | PD |
GO:0032632 | 0.0437818 | Interleukin-3 production | MSKCC | PD |
GO:0045423 | 0.0437818 | Regulation of granulocyte macrophage colony-stimulating factor biosynthetic process | MSKCC | PD |
GO:0060699 | 0.0437818 | Regulation of endoribonuclease activity | MSKCC | PD |
GO:0007405 | 0.0450633 | Neuroblast proliferation | MSKCC | PD |
GO:0019637 | 0.045281 | Organophosphate metabolic process | MSKCC | PD |
GO:0042542 | 1.365E-07 | Response to hydrogen peroxide | MSKCC | MD |
GO:0014812 | 1.111E-06 | Muscle cell migration | MSKCC | MD |
GO:0042493 | 1.147E-06 | Response to drug | MSKCC | MD |
GO:1901652 | 1.569E-06 | Response to peptide | ZB | MD |
GO:0044092 | 1.981E-06 | Negative regulation of molecular function | ZB | MD |
GO:0043434 | 5.246E-06 | Response to peptide hormone | ZB | MD |
GO:0000302 | 1.172E-05 | Response to reactive oxygen species | MSKCC | MD |
GO:0034504 | 1.315E-05 | Protein localization to nucleus | MSKCC | MD |
GO:0033365 | 1.481E-05 | Protein localization to organelle | MSKCC | MD |
GO:0070301 | 2.532E-05 | Cellular response to hydrogen peroxide | MSKCC | MD |
GO:0009607 | 3.12E-05 | Response to biotic stimulus | MSKCC | MD |
GO:0034614 | 4.066E-05 | Cellular response to reactive oxygen species | MSKCC | MD |
GO:1904705 | 4.887E-05 | Regulation of vascular smooth muscle cell proliferation | MSKCC | MD |
GO:1990874 | 4.887E-05 | Vascular smooth muscle cell proliferation | MSKCC | MD |
GO:0003279 | 6.629E-05 | Cardiac septum development | MSKCC | MD |
GO:0051707 | 6.996E-05 | Response to other organism | MSKCC | MD |
GO:0043207 | 7.068E-05 | Response to external biotic stimulus | MSKCC | MD |
GO:1901652 | 7.61E-05 | Response to peptide | MSKCC | MD |
GO:0051223 | 9.568E-05 | Regulation of protein transport | MSKCC | MD |
GO:0060411 | 0.0001143 | Cardiac septum morphogenesis | MSKCC | MD |
GO:0014909 | 0.0001298 | Smooth muscle cell migration | MSKCC | MD |
GO:0032496 | 0.0001321 | Response to lipopolysaccharide | MSKCC | MD |
GO:0045682 | 0.0001383 | Regulation of epidermis development | MSKCC | MD |
GO:0006273 | 0.0001448 | Lagging strand elongation | MSKCC | MD |
GO:0043434 | 0.0001755 | Response to peptide hormone | MSKCC | MD |
GO:0002237 | 0.0001807 | Response to molecule of bacterial origin | MSKCC | MD |
GO:0003179 | 0.000188 | Heart valve morphogenesis | MSKCC | MD |
GO:0050864 | 0.0002231 | Regulation of B cell activation | MSKCC | MD |
GO:0003170 | 0.0002759 | Heart valve development | MSKCC | MD |
GO:0051570 | 0.0003745 | Regulation of histone H3-K9 methylation | ZB | MD |
GO:0044092 | 0.0004357 | Negative regulation of molecular function | MSKCC | MD |
GO:0042100 | 0.0004756 | B cell proliferation | MSKCC | MD |
GO:0000302 | 0.0005009 | Response to reactive oxygen species | ZB | MD |
GO:1904019 | 0.0005234 | Epithelial cell apoptotic process | MSKCC | MD |
GO:0006266 | 0.0005342 | DNA ligation | ZB | MD |
GO:0034614 | 0.00056 | Cellular response to reactive oxygen species | ZB | MD |
GO:2001242 | 0.0005685 | Regulation of intrinsic apoptotic signaling pathway | MSKCC | MD |
GO:0046879 | 0.0006353 | Hormone secretion | MSKCC | MD |
GO:0006271 | 0.0006777 | DNA strand elongation involved in DNA replication | MSKCC | MD |
GO:0003205 | 0.0007089 | Cardiac chamber development | MSKCC | MD |
GO:0003007 | 0.0007878 | Heart morphogenesis | MSKCC | MD |
GO:0009914 | 0.0008096 | Hormone transport | MSKCC | MD |
GO:0034504 | 0.0008947 | Protein localization to nucleus | ZB | MD |
GO:0051567 | 0.000944 | Histone H3-K9 methylation | ZB | MD |
GO:0030888 | 0.001 | Regulation of B cell proliferation | MSKCC | MD |
GO:0032611 | 0.001 | Interleukin-1 beta production | MSKCC | MD |
GO:0033157 | 0.001093 | Regulation of intracellular protein transport | ZB | MD |
GO:1902042 | 0.001286 | Negative regulation of extrinsic apoptotic signaling pathway via death domain receptors | ZB | MD |
GO:0050852 | 0.0012901 | T cell receptor signaling pathway | MSKCC | MD |
GO:0006266 | 0.0012907 | DNA ligation | MSKCC | MD |
GO:0032845 | 0.0013576 | Negative regulation of homeostatic process | MSKCC | MD |
GO:0003281 | 0.0014821 | Ventricular septum development | MSKCC | MD |
GO:0033143 | 0.0014821 | Regulation of intracellular steroid hormone receptor signaling pathway | MSKCC | MD |
GO:0022616 | 0.001571 | DNA strand elongation | MSKCC | MD |
GO:0071887 | 0.0016635 | Leukocyte apoptotic process | ZB | MD |
GO:1904035 | 0.0017433 | Regulation of epithelial cell apoptotic process | MSKCC | MD |
GO:0032612 | 0.0019145 | Interleukin-1 production | MSKCC | MD |
GO:0097306 | 0.0019363 | Cellular response to alcohol | ZB | MD |
GO:0034968 | 0.0021658 | Histone lysine methylation | ZB | MD |
GO:0061647 | 0.002279 | Histone H3-K9 modification | ZB | MD |
GO:0033146 | 0.002285 | Regulation of intracellular estrogen receptor signaling pathway | MSKCC | MD |
GO:0022408 | 0.0023527 | Negative regulation of cell-cell adhesion | MSKCC | MD |
GO:0033157 | 0.0023985 | Regulation of intracellular protein transport | MSKCC | MD |
GO:0051223 | 0.0024086 | Regulation of protein transport | ZB | MD |
GO:0032446 | 0.0024086 | Protein modification by small protein conjugation | ZB | MD |
GO:0051573 | 0.0028681 | Negative regulation of histone H3-K9 methylation | ZB | MD |
GO:0018022 | 0.0028725 | Peptidyl-lysine methylation | ZB | MD |
GO:0046824 | 0.0028725 | Positive regulation of nucleocytoplasmic transport | ZB | MD |
GO:0031648 | 0.0030756 | Protein destabilization | MSKCC | MD |
GO:0009636 | 0.0032692 | Response to toxic substance | ZB | MD |
GO:0006261 | 0.0033055 | DNA-dependent DNA replication | MSKCC | MD |
GO:0006273 | 0.0033374 | Lagging strand elongation | ZB | MD |
GO:0002223 | 0.0035118 | Stimulatory C-type lectin receptor signaling pathway | ZB | MD |
GO:0002220 | 0.0036986 | Innate immune response activating cell surface receptor signaling pathway | ZB | MD |
GO:0051103 | 0.0038896 | DNA ligation involved in DNA repair | ZB | MD |
GO:0003007 | 0.0040602 | Heart morphogenesis | ZB | MD |
GO:0016571 | 0.0042866 | Histone methylation | ZB | MD |
GO:0097306 | 0.0044943 | Cellular response to alcohol | MSKCC | MD |
GO:0031060 | 0.0045733 | Regulation of histone methylation | ZB | MD |
GO:0023061 | 0.0047426 | Signal release | MSKCC | MD |
GO:0051573 | 0.0047426 | Negative regulation of histone H3-K9 methylation | MSKCC | MD |
GO:0001666 | 0.0047462 | Response to hypoxia | MSKCC | MD |
GO:0006284 | 0.0050431 | Base-excision repair | MSKCC | MD |
GO:0018205 | 0.0051017 | Peptidyl-lysine modification | ZB | MD |
GO:0036293 | 0.0053204 | Response to decreased oxygen levels | MSKCC | MD |
GO:0061647 | 0.0053204 | Histone H3-K9 modification | MSKCC | MD |
GO:0006261 | 0.0055276 | DNA-dependent DNA replication | ZB | MD |
GO:2001020 | 0.0055567 | Regulation of response to DNA damage stimulus | MSKCC | MD |
GO:0032446 | 0.0057176 | Protein modification by small protein conjugation | MSKCC | MD |
GO:0014909 | 0.0058867 | Smooth muscle cell migration | ZB | MD |
GO:0070664 | 0.0058867 | Negative regulation of leukocyte proliferation | ZB | MD |
GO:0090316 | 0.0059508 | Positive regulation of intracellular protein transport | MSKCC | MD |
GO:0032651 | 0.0061433 | Regulation of interleukin-1 beta production | MSKCC | MD |
GO:0006304 | 0.0062479 | DNA modification | MSKCC | MD |
GO:0051103 | 0.0062941 | DNA ligation involved in DNA repair | MSKCC | MD |
GO:0030520 | 0.0064205 | Intracellular estrogen receptor signaling pathway | MSKCC | MD |
GO:0042093 | 0.0064205 | T-helper cell differentiation | MSKCC | MD |
GO:0045936 | 0.0065605 | Negative regulation of phosphate metabolic process | ZB | MD |
GO:0010563 | 0.0066057 | Negative regulation of phosphorus metabolic process | ZB | MD |
GO:0045604 | 0.0067768 | Regulation of epidermal cell differentiation | MSKCC | MD |
GO:0031061 | 0.0067822 | Negative regulation of histone methylation | ZB | MD |
GO:0034968 | 0.0067993 | Histone lysine methylation | MSKCC | MD |
GO:0002294 | 0.0071277 | CD4-positive, alpha-beta T cell differentiation involved in immune response | MSKCC | MD |
GO:0002287 | 0.0074349 | alpha-beta T cell activation involved in immune response | MSKCC | MD |
GO:0002293 | 0.0074349 | alpha-beta T cell differentiation involved in immune response | MSKCC | MD |
GO:0031663 | 0.0074349 | Lipopolysaccharide-mediated signaling pathway | MSKCC | MD |
GO:0038034 | 0.0077505 | Signal transduction in absence of ligand | ZB | MD |
GO:0097192 | 0.0077505 | Extrinsic apoptotic signaling pathway in absence of ligand | ZB | MD |
GO:0034284 | 0.0078981 | Response to monosaccharide | MSKCC | MD |
GO:0006271 | 0.0081662 | DNA strand elongation involved in DNA replication | ZB | MD |
GO:0046660 | 0.0082366 | Female sex differentiation | MSKCC | MD |
GO:0014812 | 0.008241 | Muscle cell migration | ZB | MD |
GO:0003283 | 0.0088126 | Atrial septum development | ZB | MD |
GO:0023019 | 0.0088126 | Signal transduction involved in regulation of gene expression | ZB | MD |
GO:0007259 | 0.0089793 | JAK-STAT cascade | ZB | MD |
GO:0097696 | 0.0089793 | STAT cascade | ZB | MD |
GO:0018022 | 0.0091089 | Peptidyl-lysine methylation | MSKCC | MD |
GO:0046824 | 0.0091089 | Positive regulation of nucleocytoplasmic transport | MSKCC | MD |
GO:0001541 | 0.0092698 | Ovarian follicle development | MSKCC | MD |
GO:0070301 | 0.0095041 | Cellular response to hydrogen peroxide | ZB | MD |
GO:0002292 | 0.0096977 | T cell differentiation involved in immune response | MSKCC | MD |
GO:1903533 | 0.0097929 | Regulation of protein targeting | ZB | MD |
GO:0006479 | 0.0099192 | Protein methylation | ZB | MD |
GO:0008213 | 0.0099192 | Protein alkylation | ZB | MD |
GO:0050852 | 0.0099192 | T cell receptor signaling pathway | ZB | MD |
GO:0032845 | 0.0101971 | Negative regulation of homeostatic process | ZB | MD |
GO:0031060 | 0.0104978 | Regulation of histone methylation | MSKCC | MD |
GO:0032652 | 0.0104978 | Regulation of interleukin-1 production | MSKCC | MD |
GO:0008625 | 0.0108141 | Extrinsic apoptotic signaling pathway via death domain receptors | ZB | MD |
GO:0032496 | 0.0108141 | Response to lipopolysaccharide | ZB | MD |
GO:0009743 | 0.0108748 | Response to carbohydrate | MSKCC | MD |
GO:0001947 | 0.0108748 | Heart looping | MSKCC | MD |
GO:0048678 | 0.0108748 | Response to axon injury | MSKCC | MD |
GO:0072091 | 0.0108748 | Regulation of stem cell proliferation | MSKCC | MD |
GO:1903533 | 0.0110206 | Regulation of protein targeting | MSKCC | MD |
GO:0002223 | 0.0110206 | Stimulatory C-type lectin receptor signaling pathway | MSKCC | MD |
GO:0031061 | 0.0110206 | Negative regulation of histone methylation | MSKCC | MD |
GO:0043367 | 0.0112179 | CD4-positive, alpha-beta T cell differentiation | MSKCC | MD |
GO:0002220 | 0.0115502 | Innate immune response activating cell surface receptor signaling pathway | MSKCC | MD |
GO:1904705 | 0.0117161 | Regulation of vascular smooth muscle cell proliferation | ZB | MD |
GO:1990874 | 0.0117161 | Vascular smooth muscle cell proliferation | ZB | MD |
GO:0042100 | 0.0117593 | B cell proliferation | ZB | MD |
GO:1904019 | 0.0124536 | Epithelial cell apoptotic process | ZB | MD |
GO:0006471 | 0.0125445 | Protein ADP-ribosylation | ZB | MD |
GO:0002237 | 0.0127762 | Response to molecule of bacterial origin | ZB | MD |
GO:0061371 | 0.0130477 | Determination of heart left/right asymmetry | MSKCC | MD |
GO:0016571 | 0.0132027 | Histone methylation | MSKCC | MD |
GO:0022616 | 0.0132769 | DNA strand elongation | ZB | MD |
GO:0008585 | 0.0132769 | Female gonad development | ZB | MD |
GO:0003143 | 0.0134719 | Embryonic heart tube morphogenesis | MSKCC | MD |
GO:0008589 | 0.0134719 | Regulation of smoothened signaling pathway | MSKCC | MD |
GO:0035710 | 0.0134719 | CD4-positive, alpha-beta T cell activation | MSKCC | MD |
GO:0070664 | 0.0134719 | Negative regulation of leukocyte proliferation | MSKCC | MD |
GO:0071301 | 0.0136096 | Cellular response to vitamin B1 | ZB | MD |
GO:0090347 | 0.0136096 | Regulation of cellular organohalogen metabolic process | ZB | MD |
GO:0090348 | 0.0136096 | Regulation of cellular organofluorine metabolic process | ZB | MD |
GO:0090349 | 0.0136096 | Negative regulation of cellular organohalogen metabolic process | ZB | MD |
GO:0090350 | 0.0136096 | Negative regulation of cellular organofluorine metabolic process | ZB | MD |
GO:1904404 | 0.0136096 | Response to formaldehyde | ZB | MD |
GO:0003279 | 0.0136766 | Cardiac septum development | ZB | MD |
GO:0046545 | 0.0139992 | Development of primary female sexual characteristics | ZB | MD |
GO:0009743 | 0.0140538 | Response to carbohydrate | ZB | MD |
GO:0043086 | 0.0143878 | Negative regulation of catalytic activity | ZB | MD |
GO:0003283 | 0.0143938 | Atrial septum development | MSKCC | MD |
GO:0023019 | 0.0143938 | Signal transduction involved in regulation of gene expression | MSKCC | MD |
GO:0007389 | 0.015078 | Pattern specification process | MSKCC | MD |
GO:0006304 | 0.0153019 | DNA modification | ZB | MD |
GO:0051100 | 0.0154442 | Negative regulation of binding | MSKCC | MD |
GO:0045936 | 0.0155204 | Negative regulation of phosphate metabolic process | MSKCC | MD |
GO:0051570 | 0.015525 | Regulation of histone H3-K9 methylation | MSKCC | MD |
GO:0010563 | 0.01554 | Negative regulation of phosphorus metabolic process | MSKCC | MD |
GO:0046634 | 0.0155981 | Regulation of alpha-beta T cell activation | MSKCC | MD |
GO:0071301 | 0.0155981 | Cellular response to vitamin B1 | MSKCC | MD |
GO:0090347 | 0.0155981 | Regulation of cellular organohalogen metabolic process | MSKCC | MD |
GO:0090348 | 0.0155981 | Regulation of cellular organofluorine metabolic process | MSKCC | MD |
GO:0090349 | 0.0155981 | Negative regulation of cellular organohalogen metabolic process | MSKCC | MD |
GO:0090350 | 0.0155981 | Negative regulation of cellular organofluorine metabolic process | MSKCC | MD |
GO:1904404 | 0.0155981 | Response to formaldehyde | MSKCC | MD |
GO:0033146 | 0.0159353 | Regulation of intracellular estrogen receptor signaling pathway | ZB | MD |
GO:0051051 | 0.0167241 | Negative regulation of transport | MSKCC | MD |
GO:0038034 | 0.0167508 | Signal transduction in absence of ligand | MSKCC | MD |
GO:0097192 | 0.0167508 | Extrinsic apoptotic signaling pathway in absence of ligand | MSKCC | MD |
GO:0046660 | 0.0191336 | Female sex differentiation | ZB | MD |
GO:0031648 | 0.01952 | Protein destabilization | ZB | MD |
GO:0006471 | 0.0196997 | Protein ADP-ribosylation | MSKCC | MD |
GO:0043086 | 0.0202833 | Negative regulation of catalytic activity | MSKCC | MD |
GO:0003179 | 0.0203264 | Heart valve morphogenesis | ZB | MD |
GO:0003230 | 0.0203264 | Cardiac atrium development | ZB | MD |
GO:0033365 | 0.0219486 | Protein localization to organelle | ZB | MD |
GO:0042542 | 0.0231473 | Response to hydrogen peroxide | ZB | MD |
GO:0010868 | 0.0231473 | Negative regulation of triglyceride biosynthetic process | ZB | MD |
GO:0071460 | 0.0231473 | Cellular response to cell-matrix adhesion | ZB | MD |
GO:0090345 | 0.0231473 | Cellular organohalogen metabolic process | ZB | MD |
GO:0090346 | 0.0231473 | Cellular organofluorine metabolic process | ZB | MD |
GO:0050864 | 0.0232449 | Regulation of B cell activation | ZB | MD |
GO:0044262 | 0.0234314 | Cellular carbohydrate metabolic process | MSKCC | MD |
GO:0003170 | 0.0234325 | Heart valve development | ZB | MD |
GO:0008625 | 0.0238195 | Extrinsic apoptotic signaling pathway via death domain receptors | MSKCC | MD |
GO:0043029 | 0.0244019 | T cell homeostasis | ZB | MD |
GO:0046825 | 0.0252439 | Regulation of protein export from nucleus | ZB | MD |
GO:0018205 | 0.0254654 | Peptidyl-lysine modification | MSKCC | MD |
GO:0090316 | 0.0255328 | Positive regulation of intracellular protein transport | ZB | MD |
GO:0022408 | 0.0260472 | Negative regulation of cell-cell adhesion | ZB | MD |
GO:0006284 | 0.0260472 | Base-excision repair | ZB | MD |
GO:0010883 | 0.0260472 | Regulation of lipid storage | ZB | MD |
GO:0007259 | 0.0262577 | JAK-STAT cascade | MSKCC | MD |
GO:0097696 | 0.0262577 | STAT cascade | MSKCC | MD |
GO:0051567 | 0.0262577 | Histone H3-K9 methylation | MSKCC | MD |
GO:0010868 | 0.0262577 | Negative regulation of triglyceride biosynthetic process | MSKCC | MD |
GO:0071460 | 0.0262577 | Cellular response to cell-matrix adhesion | MSKCC | MD |
GO:0090345 | 0.0262577 | Cellular organohalogen metabolic process | MSKCC | MD |
GO:0090346 | 0.0262577 | Cellular organofluorine metabolic process | MSKCC | MD |
GO:0044262 | 0.0265122 | Cellular carbohydrate metabolic process | ZB | MD |
GO:0042493 | 0.0271005 | Response to drug | ZB | MD |
GO:0006479 | 0.0275738 | Protein methylation | MSKCC | MD |
GO:0008213 | 0.0275738 | Protein alkylation | MSKCC | MD |
GO:0008585 | 0.0277022 | Female gonad development | MSKCC | MD |
GO:0051100 | 0.029382 | Negative regulation of binding | ZB | MD |
GO:0032651 | 0.0295013 | Regulation of interleukin-1 beta production | ZB | MD |
GO:0043525 | 0.0295013 | Positive regulation of neuron apoptotic process | ZB | MD |
GO:0051055 | 0.0295013 | Negative regulation of lipid biosynthetic process | ZB | MD |
GO:0007389 | 0.0295013 | Pattern specification process | ZB | MD |
GO:0023061 | 0.0295013 | Signal release | ZB | MD |
GO:0030520 | 0.0295013 | Intracellular estrogen receptor signaling pathway | ZB | MD |
GO:0042093 | 0.0295013 | T-helper cell differentiation | ZB | MD |
GO:0009822 | 0.0295013 | Alkaloid catabolic process | ZB | MD |
GO:0010266 | 0.0295013 | Response to vitamin B1 | ZB | MD |
GO:0033076 | 0.0295013 | Isoquinoline alkaloid metabolic process | ZB | MD |
GO:0071494 | 0.0295013 | Cellular response to UV-C | ZB | MD |
GO:0098760 | 0.0295013 | Response to interleukin-7 | ZB | MD |
GO:0098761 | 0.0295013 | Cellular response to interleukin-7 | ZB | MD |
GO:1990785 | 0.0295013 | Response to water-immersion restraint stress | ZB | MD |
GO:0071887 | 0.029714 | Leukocyte apoptotic process | MSKCC | MD |
GO:0045604 | 0.0298719 | Regulation of epidermal cell differentiation | ZB | MD |
GO:0046545 | 0.0305378 | Development of primary female sexual characteristics | MSKCC | MD |
GO:1902042 | 0.0306947 | Negative regulation of extrinsic apoptotic signaling pathway via death domain receptors | MSKCC | MD |
GO:0002294 | 0.0309829 | CD4-positive, alpha-beta T cell differentiation involved in immune response | ZB | MD |
GO:0002287 | 0.0320739 | alpha-beta T cell activation involved in immune response | ZB | MD |
GO:0002293 | 0.0320739 | alpha-beta T cell differentiation involved in immune response | ZB | MD |
GO:0031663 | 0.0320739 | Lipopolysaccharide-mediated signaling pathway | ZB | MD |
GO:0003230 | 0.0321242 | Cardiac atrium development | MSKCC | MD |
GO:2001242 | 0.0323501 | Regulation of intrinsic apoptotic signaling pathway | ZB | MD |
GO:0051051 | 0.0324454 | Negative regulation of transport | ZB | MD |
GO:0051707 | 0.0338559 | Response to other organism | ZB | MD |
GO:0043207 | 0.033997 | Response to external biotic stimulus | ZB | MD |
GO:0009822 | 0.0354738 | Alkaloid catabolic process | MSKCC | MD |
GO:0010266 | 0.0354738 | Response to vitamin B1 | MSKCC | MD |
GO:0033076 | 0.0354738 | Isoquinoline alkaloid metabolic process | MSKCC | MD |
GO:0071494 | 0.0354738 | Cellular response to UV-C | MSKCC | MD |
GO:0098760 | 0.0354738 | Response to interleukin-7 | MSKCC | MD |
GO:0098761 | 0.0354738 | Cellular response to interleukin-7 | MSKCC | MD |
GO:1990785 | 0.0354738 | Response to water-immersion restraint stress | MSKCC | MD |
GO:0003205 | 0.0358613 | Cardiac chamber development | ZB | MD |
GO:0001541 | 0.0363016 | Ovarian follicle development | ZB | MD |
GO:0007089 | 0.0363016 | Traversing start control point of mitotic cell cycle | ZB | MD |
GO:0010989 | 0.0363016 | Negative regulation of low-density lipoprotein particle clearance | ZB | MD |
GO:0014042 | 0.0363016 | Positive regulation of neuron maturation | ZB | MD |
GO:0035799 | 0.0363016 | Ureter maturation | ZB | MD |
GO:0042997 | 0.0363016 | Negative regulation of Golgi to plasma membrane protein transport | ZB | MD |
GO:0070427 | 0.0363016 | Nucleotide-binding oligomerization domain containing 1 signaling pathway | ZB | MD |
GO:2000048 | 0.0363016 | Negative regulation of cell-cell adhesion mediated by cadherin | ZB | MD |
GO:0046879 | 0.0364954 | Hormone secretion | ZB | MD |
GO:0002292 | 0.0371894 | T cell differentiation involved in immune response | ZB | MD |
GO:0030888 | 0.0371894 | Regulation of B cell proliferation | ZB | MD |
GO:0032611 | 0.0371894 | Interleukin-1 beta production | ZB | MD |
GO:0043029 | 0.0386747 | T cell homeostasis | MSKCC | MD |
GO:0032652 | 0.0392552 | Regulation of interleukin-1 production | ZB | MD |
GO:2001020 | 0.039929 | Regulation of response to DNA damage stimulus | ZB | MD |
GO:0046825 | 0.0400926 | Regulation of protein export from nucleus | MSKCC | MD |
GO:0001947 | 0.040316 | Heart looping | ZB | MD |
GO:0048678 | 0.040316 | Response to axon injury | ZB | MD |
GO:0072091 | 0.040316 | Regulation of stem cell proliferation | ZB | MD |
GO:0009914 | 0.0407189 | Hormone transport | ZB | MD |
GO:0009607 | 0.0410816 | Response to biotic stimulus | ZB | MD |
GO:0043367 | 0.0414048 | CD4-positive, alpha-beta T cell differentiation | ZB | MD |
GO:0010883 | 0.0416577 | Regulation of lipid storage | MSKCC | MD |
GO:0007089 | 0.0432135 | Traversing start control point of mitotic cell cycle | MSKCC | MD |
GO:0010989 | 0.0432135 | Negative regulation of low-density lipoprotein particle clearance | MSKCC | MD |
GO:0014042 | 0.0432135 | Positive regulation of neuron maturation | MSKCC | MD |
GO:0035799 | 0.0432135 | Ureter maturation | MSKCC | MD |
GO:0042997 | 0.0432135 | Negative regulation of Golgi to plasma membrane protein transport | MSKCC | MD |
GO:0070427 | 0.0432135 | Nucleotide-binding oligomerization domain containing 1 signaling pathway | MSKCC | MD |
GO:2000048 | 0.0432135 | Negative regulation of cell-cell adhesion mediated by cadherin | MSKCC | MD |
GO:0003281 | 0.0437357 | Ventricular septum development | ZB | MD |
GO:0033143 | 0.0437357 | Regulation of intracellular steroid hormone receptor signaling pathway | ZB | MD |
GO:0060411 | 0.0437357 | Cardiac septum morphogenesis | ZB | MD |
GO:0061371 | 0.0447239 | Determination of heart left/right asymmetry | ZB | MD |
GO:0009636 | 0.0455879 | Response to toxic substance | MSKCC | MD |
GO:0003143 | 0.0456721 | Embryonic heart tube morphogenesis | ZB | MD |
GO:0008589 | 0.0456721 | Regulation of smoothened signaling pathway | ZB | MD |
GO:0035710 | 0.0456721 | CD4-positive, alpha-beta T cell activation | ZB | MD |
GO:0001666 | 0.0462698 | Response to hypoxia | ZB | MD |
GO:0043525 | 0.0463606 | Positive regulation of neuron apoptotic process | MSKCC | MD |
GO:0051055 | 0.0463606 | Negative regulation of lipid biosynthetic process | MSKCC | MD |
GO:0045682 | 0.0465784 | Regulation of epidermis development | ZB | MD |
GO:1904035 | 0.0465784 | Regulation of epithelial cell apoptotic process | ZB | MD |
GO:0034284 | 0.0473956 | Response to monosaccharide | ZB | MD |
GO:0032612 | 0.0473956 | Interleukin-1 production | ZB | MD |
GO:0036293 | 0.047653 | Response to decreased oxygen levels | ZB | MD |
GO:0046634 | 0.0493719 | Regulation of alpha-beta T cell activation | ZB | MD |
GO:1905005 | 0.0303648 | Regulation of epithelial to mesenchymal transition involved in endocardial cushion formation | ZB | WD |
GO:0003198 | 0.0462411 | Epithelial to mesenchymal transition involved in endocardial cushion formation | ZB | WD |
GO:0034110 | 0.0498585 | Regulation of homotypic cell-cell adhesion | ZB | WD |
GO:1905005 | 0.027475 | Regulation of epithelial to mesenchymal transition involved in endocardial cushion formation | MSKCC | WD |
GO:0003198 | 0.0414965 | Epithelial to mesenchymal transition involved in endocardial cushion formation | MSKCC | WD |
GO:0034110 | 0.0441837 | Regulation of homotypic cell-cell adhesion | MSKCC | WD |
Note: dataset, our dataset was named ZB. PD, poor differentiated; MD, moderate differentiated; WD, well differentiated.
Acknowledgments
Funding: None.
Footnote
Conflicts of Interest: All authors have completed the ICMJE uniform disclosure form (available at http://dx.doi.org/10.21037/tcr.2020.03.32). The work was carried out as part of the employment of the corresponding author at the Affiliated Hospital of Qingdao University. The Affiliated Hospital of Qingdao University was not involved in the manuscript writing, editing, approval, or decision to publish. The other authors have no conflicts of interest to declare.
Ethical Statement: The authors are accountable for all aspects of the work in ensuring that questions related to the accuracy or integrity of any part of the work are appropriately investigated and resolved. This study was approved by Shandong Provincial Hospital Affiliated to Shandong University and The Affiliated Hospital of Qingdao University. Each participant provided written informed consent. The study was conducted in accordance with the Declaration of Helsinki (as revised in 2013).
Open Access Statement: This is an Open Access article distributed in accordance with the Creative Commons Attribution-NonCommercial-NoDerivs 4.0 International License (CC BY-NC-ND 4.0), which permits the non-commercial replication and distribution of the article with the strict proviso that no changes or edits are made and the original work is properly cited (including links to both the formal publication through the relevant DOI and the license). See: https://creativecommons.org/licenses/by-nc-nd/4.0/.
References
- Cillo U, Giuliani T, Polacco M, et al. Prediction of hepatocellular carcinoma biological behavior in patient selection for liver transplantation. World J Gastroenterol 2016;22:232-52. [Crossref] [PubMed]
- Schadendorf D, Hodi FS, Robert C, et al. Pooled Analysis of Long-Term Survival Data From Phase II and Phase III Trials of Ipilimumab in Unresectable or Metastatic Melanoma. J Clin Oncol 2015;33:1889-94. [Crossref] [PubMed]
- Kobayashi M, Sawada K, Nakamura K, et al. Exosomal miR-1290 is a potential biomarker of high-grade serous ovarian carcinoma and can discriminate patients from those with malignancies of other histological types. J Ovarian Res 2018;11:81. [Crossref] [PubMed]
- Skoog P, Ohlsson M, Ferno M, et al. Tumor tissue protein signatures reflect histological grade of breast cancer. PLoS One 2017;12:e0179775. [Crossref] [PubMed]
- Cole AJ, Dwight T, Gill AJ, et al. Assessing mutant p53 in primary high-grade serous ovarian cancer using immunohistochemistry and massively parallel sequencing. Sci Rep 2016;6:26191. [Crossref] [PubMed]
- Deng L, Xiong P, Luo Y, et al. Association between IDH1/2 mutations and brain glioma grade. Oncol Lett 2018;16:5405-9. [PubMed]
- Wodziński D, Wosiak A, Pietrzak J, et al. Does the expression of the ACVR2A gene affect the development of colorectal cancer? Genet Mol Biol 2019;42:32-9. [Crossref] [PubMed]
- Edmondson HA, Steiner PE. Primary carcinoma of the liver: a study of 100 cases among 48,900 necropsies. Cancer 1954;7:462-503. [Crossref] [PubMed]
- Martin M. Cutadapt removes adapter sequences from high-throughput sequencing reads. EMBnet Journal 2011;17:3. [Crossref]
- Li H, Durbin R. Fast and accurate short read alignment with Burrows-Wheeler transform. Bioinformatics 2009;25:1754-60. [Crossref] [PubMed]
- McKenna A, Hanna M, Banks E, et al. The Genome Analysis Toolkit: a MapReduce framework for analyzing next-generation DNA sequencing data. Genome Res 2010;20:1297-303. [Crossref] [PubMed]
- Cibulskis K, Lawrence MS, Carter SL, et al. point mutations in impure and heterogeneous cancer samples. Nat Biotechnol 2013;31:213-9. [Crossref] [PubMed]
- Koboldt DC, Chen K, Wylie T, et al. VarScan: variant detection in massively parallel sequencing of individual and pooled samples. Bioinformatics 2009;25:2283-5. [Crossref] [PubMed]
- Karczewski KJ, Weisburd B, Thomas B, et al. The ExAC browser: displaying reference data information from over 60 000 exomes. Nucleic Acids Res 2017;45:D840-D845. [Crossref] [PubMed]
- Lek M, Karczewski KJ, Minikel EV, et al. Analysis of protein-coding genetic variation in 60,706 humans. Nature 2016;536:285-91. [Crossref] [PubMed]
- 1000 Genomes Project Consortium, Abecasis GR, Altshuler D, et al. A map of human genome variation from population-scale sequencing. Nature 2010;467:1061-73.
- NHLBI. Exome Variant Server. Available online: https://evs.gs.washington.edu. 2017/7/1.
- Wang K, Li M, Hakonarson H. ANNOVAR: functional annotation of genetic variants from high-throughput sequencing data. Nucleic Acids Res 2010;38:e164. [Crossref] [PubMed]
- Li H. FermiKit: assembly-based variant calling for Illumina resequencing data. Bioinformatics 2015;31:3694-6. [PubMed]
- Talevich E, Shain AH, Botton T, et al. CNVkit: Genome-Wide Copy Number Detection and Visualization from Targeted DNA Sequencing. PLoS Comput Biol 2016;12:e1004873. [Crossref] [PubMed]
- Murtaza M, Dawson SJ, Pogrebniak K, et al. Multifocal clonal evolution characterized using circulating tumour DNA in a case of metastatic breast cancer. Nat Commun 2015;6:8760. [Crossref] [PubMed]
- Jiang T, Shi W, Wali VB, et al. Predictors of Chemosensitivity in Triple Negative Breast Cancer: An Integrated Genomic Analysis. PLoS Med 2016;13:e1002193. [Crossref] [PubMed]
- Edge SB, Byrd D, Compton C, et al. Digestive system. In: Trotti A. editor. AJCC cancer staging manual, ed 7th. New York: Springer, 2010.
- Schrock AB, Pavlick D, Klempner SJ, et al. Hybrid Capture-Based Genomic Profiling of Circulating Tumor DNA from Patients with Advanced Cancers of the Gastrointestinal Tract or Anus. Clin Cancer Res 2018;24:1881-90. [Crossref] [PubMed]
- Lee JS. The mutational landscape of hepatocellular carcinoma. Clin Mol Hepatol 2015;21:220-9. [Crossref] [PubMed]
- Ding XX, Zhu QG, Zhang SM, et al. Precision medicine for hepatocellular carcinoma: driver mutations and targeted therapy. Oncotarget 2017;8:55715-30. [PubMed]
- Alexandrov LB, Nik-Zainal S, Wedge DC, et al. Signatures of mutational processes in human cancer. Nature 2013;500:415-21. [Crossref] [PubMed]
- Kandoth C, McLellan MD, Vandin F, et al. Mutational landscape and significance across 12 major cancer types. Nature 2013;502:333-9. [Crossref] [PubMed]
- Boichard A, Pham TV, Yeerna H, et al. APOBEC-related mutagenesis and neo-peptide hydrophobicity: implications for response to immunotherapy. Oncoimmunology 2018;8:1550341. [Crossref] [PubMed]
- Morishita A, Iwama H, Fujihara S, et al. Targeted sequencing of cancer-associated genes in hepatocellular carcinoma using next-generation sequencing. Oncol Lett 2018;15:528-32. [PubMed]
- Zhou C, Yuan Z, Ma W, et al. Clinical utility of tumor genomic profiling in patients with high plasma circulating tumor DNA burden or metabolically active tumors. J Hematol Oncol 2018;11:129. [Crossref] [PubMed]
- Badowska-Kozakiewicz AM, Liszcz A, Sobol M, et al. Retrospective evaluation of histopathological examinations in invasive ductal breast cancer of no special type: an analysis of 691 patients. Arch Med Sci 2017;13:1408-15. [Crossref] [PubMed]
- Kancherla V, Abdullazade S, Matter MS, et al. Genomic Analysis Revealed New Oncogenic Signatures in TP53-Mutant Hepatocellular Carcinoma. Front Genet 2018;9:2. [Crossref] [PubMed]