Identification of hub genes correlated with the pathogenesis and prognosis in Pancreatic adenocarcinoma on bioinformatics methods
Introduction
Pancreatic adenocarcinoma (PC) is one of the common digestive system tumors. The mortality rate of PC ranks the fourth among all malignant tumors, and the 5-year relative survival rate was only 8%, which is the worst in the United States (1). Due to the lack of specific and sensitive diagnostic indicators, the screening diagnosis rate of patients with a family history of PC was usually less than 1% (2). The good choice to cure PC is surgical resection, but the recurrence rate of patients after operation was about 81% (3). PC is characterized by occult onset, early distant metastasis, and insensitive to a variety of traditional chemotherapy drugs (4). At present, the main clinical management is radiotherapy or chemotherapy, but such improvements are still usually measured in the range of weeks to months (5,6). According to reports, the whole genome and exon sequencing have proved that the genome of PC has significant and complex variation (7,8), and the classification of DNA and RNA is helpful to predict the drug sensitivity of each molecular subtype (9). Hence, it is of great significance to explore the molecular mechanism of the occurrence and development of PC from gene level, and to identify specific differential genes for the early detection of PC to increase OS. Unfortunately, as the currently published research was limited by small sample size, application of different technology platforms, the common disadvantage of mRNA expression profiling research is a lack of consistency, resulting in non-specific and insensitive biomarkers (10-12).
Bioinformatics analysis has been widely used to map the molecular basis of PC heterogeneity and malignant progression. Microarray technology can be used to analyze comprehensive mRNA expression profile, and to identify and research new biomarkers related to tumorigenesis of PC (13). The Cancer Genome Atlas (TCGA) and Gene Expression Omnibus (GEO) include varied cancer types for high-throughput sequencing and gene expression prediction data at the level of DNA, RNA, protein and epigenetics.
In our study, we used microarray data analysis of GEO and TCGA databases to obtain the expression signature of PC. It wound be a new potential biomarker to improve the early diagnosis, and find the therapeutic target and improve the prognosis. Furthermore, we verified the novel genes in PC by integrated bioinformatics approach, and aimed to provide a theoretical basis for future molecular mechanisms.
Methods
Data source
We downloaded eight publicly available gene expression profiles (GSE15471, GSE28735, GSE32676, GSE39751, GSE43795, GSE55643, GSE62165, and GSE62452) from GEO database, which met the following criteria: (I) Studies with PC tissue samples from human; (II) samples contained both PC and normal (or adjacent) tissues; (III) sample size was 25 or more. The larger the sample sizes are, the more persuasive the evidence is and the less biased the small sample events are. Furthermore, we obtained the gene expression profiles and clinical information of 178 PC patients from TCGA (http://tcga-data.nci.nih.gov). All of the data can be obtained for free online. Platform and series matrix files are downloaded in the form of txt files.
Integration of microarray data and key gene screening
The main sources of bias and variability are generally considered to be heterogeneity and potential variables (14). First of all, we combine all the GEO samples of eight datasets by “SVA” package of R, which can not only improve the number of samples, but also avoid producing unreliable results. In this way, the data were merged, calibrated and standardized. Next, we using “limma” package of R to identify the differentially expressed genes (DEGs) between normal pancreatic tissue and PC tissue. Genes with a |logFC|>2 and adjusted P value (FDR) <0.05 indicated statistically significance. The TCGA dataset was standardized and analyzed by the “edgR” package of R. The TCGA-DEGs were screened by the criteria of a false discovery rate (FDR) P<0.05 and |logFC|>2. The DEGs in GEO and TCGA PC were plotted by “volcano” package of R. The overlapped DEGs in the GEO and TCGA datasets were identified as key genes.
Expression and Survival analysis of key genes
The GEPIA (Gene Expression Profiling Interactive Analysis) (http://gepia.cancer-pku.cn/about.html) is used to analyze the RNA sequence expression data of 9,736 tumors and 8,587 normal samples in TCGA and GTEX projects. We utilized GEPIA to study the expression of key genes in PC patients. In order to determine whether the key genes are related to the prognosis of PC, Kaplan Meier analysis was carried out by “survival” package of R.
Key genes analysis use UALCAN online databases
UALCAN (http://ualcan.path.uab.eduis) is a user-friendly interactive web resource for analyzing cancer transcriptome data (TCGA and MET500 transcriptome sequencing) (15). We can apply UALCAN to distinguish biomarkers or implement computer verification of potential genes of interest. One of the user-friendly functions of the portal is that it can analyze the relative expression of query genes between tumor/normal specimen, and in different tumor molecular subtypes, such as individual age, gender, tumor stage or other clinicopathological features. Accordingly, based on the clinicopathological characteristics of various tumor molecular subtypes and PC, we probed into the relative expression of key genes via UALCAN.
Data processing of gene set enrichment analysis (GSEA)
Using GSEA4.0.0 software for gene enrichment analysis, utilizing c2.cp.kegg.v5.2.Symbols.gmt data set in MSigDB databank as the functional gene set, according to the expression level of KEY genes, they were separated into upregulated expression group and down regulated expression group. According to the method of default weighted enrichment statistics, the number of random combinations was 1,000, and the possible mechanism of key gene expression level on PC patients was analyzed. The gene set with P<0.05 and FDR (false discovery rates) <0.05 was used as the significant enrichment gene set.
Prognostic gene signature construction
The survival time and life status of PC patients can also be obtained from TCGA. patients with PC were established prognostic characteristics by integrating gene expression and survival information. The expression value of DEGs in TCGA was filtered into univariate Cox regression analysis. Genes based on P<0.05 are bound up with overall survival (OS) in datasets. Then, through further analysis of the genes with P<0.05 in univariate analysis, the OS for predicting gene signature was constructed in the multivariate Cox regression model. The risk score of the dataset was also obtained by multiplying the gene expression value with the correlation coefficient in the multivariate Cox regression model. The survival analysis and receiver operating characteristic curve (ROC) were constructed by predicting the performance of the risk score in predicting OS. Area under curve (AUC) analysis was performed to define the prediction capability.
Results
Identification of DEGs and key gene screening
The Pancreatic cancer expression microarray datasets GSE15471, GSE28735, GSE32676, GSE39751, GSE43795, GSE55643, GSE62165, and GSE62452 were standardized, among them, GSE15471 contained 39 PC samples and 39 normal samples, GSE28735 contained 45 PC samples and 45 normal samples, GSE32676 contained 25 PC samples and 7 normal samples, GSE39751 contained 12 PC samples and 12 normal samples, GSE43795 contained 26 PC samples and 5 normal samples, GSE55643 contained 45 PC samples and 8 normal samples, GSE62165 contained 118 PC samples and 13 normal samples, GSE62452 contained 69 PC samples and 61 normal samples. Besides, GSE15471 and GSE32676 were from platform GPL570 [(HG-U133_Plus_2) Affymetrix Human Genome U133 Plus 2.0 Array]. GSE28735 and GSE62452 were from platform GPL6244 [(HuGene-1_0-st) Affymetrix Human Gene 1.0 ST Array]. GSE39751 was from platform GPL5936 (HEEBO Human oligo array). GSE43795 was from platform GPL10558 (Illumina HumanHT-12 V4.0 expression beadchip). GSE55643 was from platform GPL6480 (Agilent-01485 0 Whole Human Genome Microarray 4x44K G4112F), and GSE62165 was based on platform GPL13667 [(HG-U219] Affymetrix Human Genome U219 Array]. Table 1 was listed for details of the datasets. After merging all the samples of 8 GEO datasets, we standardized them in batches. Then merging, calibrating and standardizing the data, we compared the tumor tissue with the normal tissue. According to the screening criteria of |logFC|>2, adjusting the P (FDR) <0.05, 41 DEGs were identified from 8 GEO gene expression profiles, including 19 up-regulated genes and 22 down-regulated genes (Figure 1A, Table S1). Then we use the “edgR” package of R to standardize and analyze the dataset of TCGA-PC, and used FDR-P<0.05 and |logFC|>2 to screen TCGA-DEGs, 446 DEGs were identified from TCGA-PC gene expression profile, including 26 up-regulated genes and 420 down-regulated genes (Figure 1B, Table S2). Venn diagram revealed that the overlapped DEGs in GEO and TCGA datasets was TSPAN1(Figure 1C). We predict that TSPAN1 is the key gene of PC.
Table 1
Dataset | Platform | Number of samples (tumor/control) | Tumor type |
---|---|---|---|
GSE15471 | GPL570[(HG-U133_Plus_2) Affymetrix Human Genome U133 Plus 2.0 Array] | 78 (39/39) | Pancreatic cancer |
GSE28735 | GPL6244[(HuGene-1_0-st) Affymetrix Human Gene 1.0 ST Array] | 90 (45/45) | Pancreatic cancer |
GSE32676 | GPL570[(HG-U133_Plus_2) Affymetrix Human Genome U133 Plus 2.0 Array] | 32 (25/7) | Pancreatic cancer |
GSE39751 | GPL5936 (HEEBO Human oligo array) | 24 (12/12) | Pancreatic cancer |
GSE43795 | GPL10558(Illumina HumanHT-12 V4.0 expression beadchip) | 31 (26/5) | Pancreatic cancer |
GSE55643 | GPL6480 (Agilent-01485 0 Whole Human Genome Microarray 4x44K G4112F) | 53 (45/8) | Pancreatic cancer |
GSE62165 | GPL13667[(HG-U219) Affymetrix Human Genome U219 Array] | 131 (118/13) | Pancreatic cancer |
GSE62452 | GPL6244[(HuGene-1_0-st) Affymetrix Human Gene 1.0 ST Array] | 130 (61/69) | Pancreatic cancer |
TCGA | Illumina HiSeq | 182 (178/4) | Pancreatic cancer |
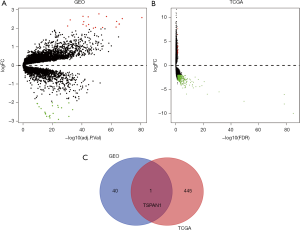
Expression and survival analysis of key genes
We used GEPIA to ascertain the expression level of the pivotal gene TSPAN1 in a variety of cancers and healthy people, and focused on the expression in PC, as shown in Figure 2, the expression of TSPAN1 is significant in a variety of tumors (Figure 2A), like Breast invasive carcinoma, Colon adenocarcinoma, Ovarian serous cystadenocarcinoma, and Endometrial cancer were significantly higher than that of normal tissues (Figure 2B). Then we utilized R software to analyze the relationship between mRNA expression and OS rate in PC patients. As presented in Figure 2C, the high expression of TSPAN1 (P<0.05), was closely related to a poor prognosis in patients with PC.
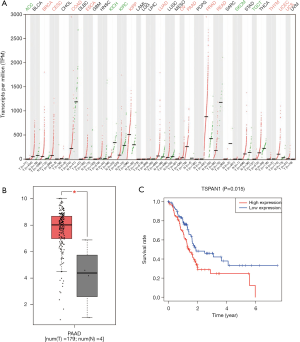
Key genes analysis use UALCAN online databases
We used UALCAN to detect the specific expression of the key gene TSPAN1 (Figure 3). The subgroup analysis of individual age, gender, cancer stage and tumor grade of PC in TCGA presented that the mRNA expression level of TSPAN1 gene was irrelevant to gender (Figure 3A), but the middle-aged and elderly patients with PC (age >41) were apparently higher than the control group (P<0.01) (Figure 3B). Moreover, high expression of TSPAN1 in PC patients was significantly associated with tumor grade 2, tumor grade 3, and tumor stage 2 (Figure 3C,D).
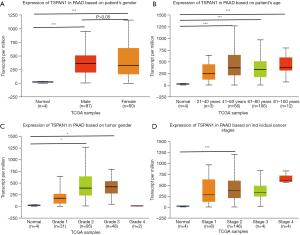
Data processing results of GSEA
As shown in Figure 4, the GSEA analysis results indicated that the high expression samples of TSPAN1 gene are mainly enriched in glycerophospholipid metabolism, glycolysis gluconeogenesis, O-glycan biosynthesis, p53 signaling pathway and tight junction (TJ). It suggested that the key gene TSPAN1 not only accelerates the proliferation of tumor cells but also affects the clinical symptoms and prognosis of patients through above biological processes. The details are shown in Table 2.
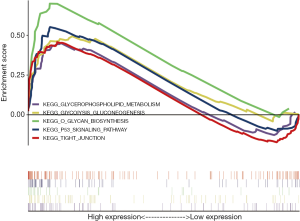
Table 2
Gene set | ES | NES | P | FDR |
---|---|---|---|---|
O Glycan Biosynthesis | 0.699 | 1.876 | 0.002 | 0.088 |
Tight Junction | 0.457 | 1.643 | 0.004 | 0.161 |
Glycerophospholipid Metabolism | 0.451 | 1.567 | 0.006 | 0.193 |
P53 Signaling Pathway | 0.552 | 1.750 | 0.011 | 0.100 |
Glycolysis Gluconeogenesis | 0.494 | 1.618 | 0.019 | 0.152 |
ES, enrichment score; NES, normalized enrichment score.
Prognostic gene signature
A total of 43 genes identified from univariate Cox regression model were remarkably correlated with survival time with P<0.05 (Table S3). In additional, a prognostic gene characteristic made up of four genes was detected through multivariate Cox regression analysis, containing AIM2, B3GNT3, MATK and BEND4. Among these genes, AIM2 and B3GNT3 with a hazard ratio (HR) of >1 were considered to be risk prognostic genes, while MATK and BEND4 with HR <1 were regarded as protective prognostic genes (P<0.05) (Table 3). 85 patients were divided into high-risk group, and the other 85 cases were divided into low-risk group according to the risk scores (Figure 5A,B,C). A highly prominent diversity in OS was revealed between the high- and low-risk groups (P=5.171e-08) (Figure 5D). Concretely, compared with 81.9% (95% CI: 74.1–90.7%), 46% (95% CI: 34.7–61.0%%) and 33.2% (95% CI: 20.8–53.1%) in low risk group, the high risk patients’ OS rate was 69.5% (95% CI: 60.2–80.3%), 24.7% (95% CI: 14.9–41.1%) and 24.7% (95% CI: 14.9–41.1%) for 1, 3, and 5 year, respectively. The gene signature exhibits a well efficiency in predicting patients’ survival (AUC 0.84) (Figure 5E).
Table 3
Gene symbol | Univariate analysis | Multivariate analysis | ||||
---|---|---|---|---|---|---|
HR (95%CI) | P value | HR (95%CI) | P value | Coefficient | ||
AIM2 | 1.1458 | 0.0168 | 1.2555 | 0.0056 | 0.2275 | |
B3GNT3 | 1.4389 | 0.0003 | 1.4298 | 0.0291 | 0.3576 | |
MATK | 0.8126 | 0.0424 | 0.7087 | 0.0321 | −0.3443 | |
BEND4 | 0.8783 | 0.0237 | 0.8084 | 0.0326 | −0.2127 |
HR, hazard ratio; CI, confidence interval.
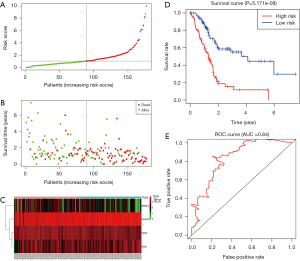
Discussion
From our research, we found that TSPAN1 was identified as the key gene of PC, which could be used as a potential targeted therapy to enhance the OS rate of patients.
Firstly, through microarray data analysis of GEO and TCGA databases, TSPAN1 was found that may be the promising key gene of PC. TSPAN1 increased in various tumors was found to be a new member of the tetraspanins group (16-18). In order to verify the effect of TSPAN1 on PC, we tested the expression of TSPAN1 gene in all tumors including PC on GEPIA and UALCAN website. It was found that TSPAN1 was over expressed in various cancers, such as prostate adenocarcinoma, kidney recurrent papillary cell carcinoma, and also in PC.
In the past, it has been found that the expression of TSPAN1 in human PC tissues and cell lines increased significantly. After siRNA targeted transfection, TSPAN1 obviously inhibited the migration and invasion of PC cells (19-21). In our research, we validated the impact of TSPAN1 on the survival rate of sufferers with PC by, and found that TSPAN1 is related to the poor prognosis of PC, which is of great significance to establish the risk scoring model of PC. ROC analysis indicated that the prediction of PC related survival rate was accurate and the increase of TSPAN1 was closely related to the clinicopathological characteristics and survival rate of PC patients, our results may add to the evidence, which is consistent with prior study.
At the same time, by studying the correlation between the TSPAN1 expression and PC progress, we found that the overall level of TSPAN1 was on the rise, the lowest expression level in the first stage and the highest expression level in the fourth stage, indicating that TSPAN1 was in direct proportion to the deterioration of PC, and could be used to assess the progressive PC in clinical stage.
At present, the main molecular mechanism of PC pathogenesis and development was unclear. Therefore, GSEA4.0.0 software was performed for gene enrichment analysis that participated in some vital pathways related to PC pathogenesis, such as glycerophospholipid metabolism, glycolysis gluconeogenesis, O glycan biosynthesis, p53 signaling pathway, TJ.
Glycerol phospholipid synthesis plays a significant part in cell proliferation (22). The first step of glycerol phospholipid pathway is to form lysophosphatidic acid (LPA) under the catalysis of glycerol-3-phosphate acyltransferase (GPAT), which is then catalyzed by several lysophosphatidic acid acyltransferase (LPAATs) (22). LPAAT-β is one of well-established LPAATs which is over-activating in certain organizations including pancreas, and a variety of tumor cell lines are specifically inhibited by it, reducing growth stagnation, apoptosis or necrosis (23). The previous study established that the most essential metabolites sub-pathway interrelated to glycerophospholipid metabolism is closely associated with PC (24). Unfortunately, there is no research focused on the use of various analytical techniques to evaluate the metabolic changes of PC in vivo and in vitro models.
Glycolysis gluconeogenesis: Tumor is more likely to use glycolysis to obtain energy, that is, aerobic glycolysis, also known as Warburg effect (25,26). According to Bailey and Collison’s classification criteria for PC based on expression profile, the quasi-mesenchymal subtype with the worst prognosis also showed significantly enhanced glycolysis activity (27,28). While, abundant researches have suggested that glycolysis of tumor cells is the key link of tumor development, and that the treatment of abnormal glycometabolism of tumor cells may be the crux to the management of PC (29-31). The analysis of gene enrichment indicated that the accumulation of TSPAN1 mRNA in the glycolysis gluconeogenesis pathway might affect the glycolysis ability of PC cells.
O-glycan biosynthesis is related to the production and transportation of nucleotide sugar donators, as well as the activity of glycosyltransferase and glycosidase, which starts after the late endoplasmic reticulum or a part of Golgi body protein folding and oligomerization (32,33). By revealing the biological significance of O-glycosylation in PC, it is helpful to decipher the molecular mechanism of tumor biology. One research suggests that elevated expression of specific O-glycosyltransferase promotes receptor modification in certain cancer cells, which may go hand in hand with the sensitivity of apoptotic ligand Apo2L/TRAIL induced by tumor necrosis factors (34). Furthermore, the abnormal O-glycosylation mediated by COSMC (C1GALT1C1) gene knockdown can promote the carcinogenesis of PC (35). However, the correlation between the expression levels of TSPAN1 and O-glycan is particularly rare in the research of PC.
p53 is a tumor suppressor that can indirectly regulate cell cycle checkpoint and apoptosis through trans activation of multiple genes, which is considered as a potential anti metastasis target of PC (36,37). However, through the interaction with homologous recombination (HR) protein and intermediate structure, p53 directly controls the beginning and end of HR and affects the transformation pathway of RAD51 gene, thus regulating the plasticity of genome (38,39). On the basis of these transformation, p53 consolidate a series of transcription steps, leading to different cell results. Recently, it was found that the inhibition of BAG3 mediated by p53 could facilitating the accumulation of p53 in the adaptation of cells to metabolic stress (40). Another study has shown that targeting NOP14 can effectively inhibit tumor invasion in a manner dependent on mutp53 (41). From these results, we can conclude that p53 has a massive impact on cell cycle and apoptosis through various pathways.
TJ is an epithelial to endothelial cell junction that regulates solute flow and maintains cell polarity through paracellular pathways (42), thereby functioning as a barrier of epithelial and endothelial cellular sheets (43). It is not a simple barrier, but a complex one, including the permeability selective barrier of steady-state regulation of many tissues, including pancreas (44). Claudin and occludin, as transmembrane proteins, are essential for the formation of TJ (43). The main function of tetraspanins is integrin-mediated adhesion strengthening (45). A study reveals that tetraspanins, such as CD9, CD81, CD82 etc, exist in DJs of epithelial cells, endothelial cells, as well as cancer cells (46). But the mechanism of TSPAN1 has not been clearly defined at endothelial cell-cell junctions.
Finally, we found four prognostic gene signatures through multivariate Cox regression analysis. The overexpressed AIM2, B3GNT3 and low-grade expressed MATK, BEND4 may be related with evaluation of therapeutic effect, recurrence rate and prognosis of PC.
Melanoma 2 (AIM2): in all stages of tumorigenesis, inflammasome signaling is involved in operating either tumor inhabited or pro-tumorigenic functions (47). The inflammasome mediated by AIM2 (a natural immune sensor), is assembled and activated in response to double-stranded DNA (48). The expression of ALM2 mRNA and protein in the pancreas of kcp1 and kcp2 mice was significantly up-regulated, and the activation of ALM2 inflammatory body further induced the expression of CD274/PD-L1 through the release of HMGB1 (high mobility group box 1) mediated by ALM2, which induces the occurrence and prognosis of PC (49). B3GNT3 pertains to the β 3glcnact gene family, which is composed of 8 and more various β 3glcnacts (50). Members of the B3GNT family participate in tumor malignant transformation. Rs265548, a single nucleotide polymorphism (SNP) near B3GNT3 gene at 19q13.1, as a novel loci related to the plasma concentration of tumor biomarker CA19-9, may be helpful for early screening of cancer risk in the general population (51). However, the molecular mechanism of B3GNT3 in PC’s cellular activity and clinical significance is still ambiguous.
Megakaryocyte-associated tyrosine kinase (MATK): it is also referred to as Csk-homologous kinase (CHK), which is a regulator of p60c-sre in megakaryocytic cells and is revolved in regulating cells proliferation (52). It has been confirmed that in human PC cells, the regulation of CHK is related to the expression of ErbB-2 (one of the most frequently highly activated proto-oncogenes) in the SH2 domain. CHK could downregulate Lyn kinase expression and significantly inhibit the multiplication and invasion of PANC-1 cells stimulated by epidermal growth factor (EGF). CHK negatively regulates ErbB-2 expression and Lyn kinase signal transduction in PC cells (53). However, further study is needed to confirm the significance of CHK in the prognosis of PC. Although the correlation between BEND4 and prognosis in PC has rarely been reported in previous study, it remains reasonable be identified as a prognostic biomarker because of its significance in our signature model and role in many other tumors (54,55). These new findings prompt researchers to further explore the molecular mechanism of malignancies to guide clinical practice.
Results of our study indicate that TSPAN1 regulated by complex molecular mechanism, as a key gene and a potential biomarker for efficient diagnosis and treatment, yet the prognostic value of AIM2, B3GNT3, MATK, BEND4 in PC provides hope for further clinical application of mRNA in PC. At the same time, only the key genes and its potential molecular mechanism were predicted in this study, therefore, it is necessary to further research the pathogenesis of PC through in vitro and in vitro experiments to verify these results.
Conclusions
In conclusion, our results suggest that the key gene TSPAN1, which is closely related to the pathogenesis, and these genes such as AIM2, B3GNT3 are considered to be associated with poor prognosis and recurrence of PC by analyzing eight chips and TCGA database. However, since our research was based on data analysis, further experiments are needed to confirm the prediction results in PC.
Table S1
DEGs | Gene names |
---|---|
Upregulated | LAMC2, SULF1, FN1, ITGA2, TSPAN1, LAMB3, POSTN, SLPI, SLC6A14, THBS2, FAP, COL10A1, CTSE, TMPRSS4, COL11A1, CST1, CEACAM6, CEACAM5, GABRP |
Downregulated | EGF, ALB, GNMT, TMED6, SERPINI2, IAPP, AQP8, PNLIPRP1, CTRL, KLK1, PDIA2, PNLIPRP2, CUZD1, GP2, CPA2, CTRC, CEL, CLPS, PLA2G1B, CPA1, REG1B, PNLIP |
GEO, Gene Expression Omnibus; DEGs, differentially expressed genes.
Table S2
DEGs | Gene names |
---|---|
Upregulated | AL121772.1, AL365356.4, B3GNT3, AC009065.5, ABCA12, AC020907.1, FXYD3,TSPAN1, AC093904.2, PPP1R14D, STYK1, SH3TC2, ZIC2, LINC00628, C2orf70, HIST1H2BD, CHRNA5, JPH1, SLC7A11, CKMT1A, HIST2H2BE, AFAP1-AS1, SPDEF, LINC00365, KCNK1, AC009065.2 |
Downregulated | CD5L, AC127496.1, AC091230.1, LMAN1L, FAM9C, SPIC, KCNT1, AC023154.1, STAB2, CD160, NKX2-5, SLC9A5, GPR182, CXCR2P1, AC008609.1, MMP12, NPIPA9, ITGAD, EEF1A1P24, IDI2-AS1, AC073850.1, KLRF1, SCN11A, ADRA1A, RF00100, LINC00996, UMODL1-AS1, DNASE1L3, GDF7, NR5A1, TBC1D27P, DNAH17-AS1, OR5G5P, H3F3C, FCRL3, PARP15, U62631.1, KLRD1, SIGLEC11, CCM2L, NCR1, AC245884.6, ABCA9-AS1, FGFBP2, AIF1L, LRTM1, LINC01894, KLHL14, CD22, LEFTY2, CRHBP, CR1, AP001605.1, TBX21, SNORD17, RN7SL752P, ATP4B, TRAPPC3L, KIR3DX1, AC009951.1, CR786580.1, AC007952.6, AL031658.1, TRG-AS1, FCRL2, AL137789.1, CLECL1, AC010970.1, LILRA1, TLR10, AC007728.2, CD36, TM4SF19, GLYCTK-AS1, AC012123.1, CHRNA4, FCRL6, AC112229.3, PTGDR, FCRL5, AC079015.1, KMO, IL9RP3, P2RX5, BFSP2, AL356234.1, ZBTB20-AS1, C11orf21, CASP16P, CFP, FGD2, KLRC4-KLRK1, PATL2, FGR, AC104809.1, LINC01537, LY86-AS1, TNFRSF13B, TLX1NB, LINC00528, LINC01684, AC010976.2, HIST2H3C, ADAD2, TEX101, ITGB2-AS1, FP671120.5, CD300LB, TDGF1, P2RX5-TAX1BP3, PARVG, BTG1P1, AC060234.2, SCIMP, HBA2, KRT72, TRDV1, BANK1, DEFA4, TXK, PZP, AC004687.1, NCF1C, MS4A1, NKG7, LILRB1, CHI3L1, MATK, FCRL1, Z84723.1, CNR2, NFIA-AS2, PAX5, ABHD17AP5, AL023653.1, KLRK1, ADGRE1, RPL7AP10, ZC3H12D, AC108206.1, AC018697.1, TSPAN32, POU2F2, TRGC1, OR5G3, HK3, RNU4-62P, LINC00173, CETP, WDFY4, SLC5A10, KIR2DL1, CD72, KCNJ10, TLX1, FAM53B-AS1, LINC01857, IL18RAP, DCSTAMP, LILRB5, NPY5R, KLRC4, AL137786.1, TMC8, CD19, KRT17P8, ITGAL, AC005332.2, CD52, CD244, Z97192.3, AC012645.3, LILRA4, HBB AL365475.1, KRT73, EOMES, AC004381.1, KEL, AC008033.3, AC018755.4, TRDC, HBA1, AL359885.1, PLEK, CD180, SASH3, CCL2, AC015660.4, ACAP1, BTK, MCOLN2, PSG9, AC010175.1, KLF1, PPP1R16B, UNC45B, COL4A3, C1QTNF1-AS1, CDHR1, AC008750.1, IL4I1, AL451067.1, IKZF3, BTLA, FCAMR, AC023510.2, TNFRSF13C, P2RY13, MAP4K1, LINC01781, AC008040.1, PRKCB, GDPD2, AP003086.1, BLK, SPATC1, HCG22, KRT75, AC243960.1, TPO, AMTN, BTBD6P1, TBC1D10C, LINC02245, C10orf90, CADM3-AS1, AC007386.1, MIR3609, FAM129C, SP140, SIGLEC22P, AC104809.2, FABP4, GPR18, GVINP1, HEATR9, TRGC2, GAPT, AC104024.1, NCF1, GPR55, AC099792.1, FCRLA, RASGRP2, GIMAP5, STAP1, AC063977.6, NPY1R, CDCA4P1, SEMA3D, MIR6774, CD37, RIPOR2, TEX53, AC079793.1, NOX5, TREML2, SCN4A, FAM30A, AC123912.4, CX3CR1, ACTG1P21, FAM238A, CARMIL2, SLC4A1, AC093010.1, AC064805.1, LINC00926, SIGLEC5, MTHFD2P1, SNORA73B, RN7SL5P, LINC00943, CCR6, GLYATL1B, LINC01645, TRAF3IP3, TTC24, LINC01215, E2F3P1, ZAP70, AL133371.2, RPL31P11, AC003957.1, AL121985.1, HLA-DOB, KRT79, AL161756.2, RSL24D1P11, AC023301.1, AC093010.2, LINC01010, SLC12A3, AL031595.1, AC119396.1, AC060764.1, AC016027.2, CELF2-AS1, SCARNA10, IRX6, LINC02422, AL096816.1, LINC01539, LINC00494, AL355076.3, PSG5, PIK3CD-AS1, HLA-DQB1-AS1, AL034397.3, AJAP1, PF4V1, AC103809.1, AL353801.1, DIRC3-AS1, AP000924.1, PTCRA, AL683807.1, CHI3L2, KIR3DL1, LTB, GGTLC1, AL356417.1, LINC01447, NCR3, LINC02137, SCNN1G, AC015909.3, AC092145.1, LINC02413, PGM5P3-AS1, OR52N4, FCMR, KIR2DL3, DDX11L10, HMGN2P19, TRGV4, AC079584.2, LINC02576, RETN, AC010247.1, CCL14, TNXA, CXCR5, AL645608.2, REELD1, AC119428.2, EIF3FP1, AC073072.1, AC098935.2, AC015911.6, ALAS2, LINC01800, ITPKB-IT1, AC103858.1, CXCL6, C12orf42, CD27, PRAMENP, AC009970.1, CAMP, CLEC17A, SIRPG-AS1, IGHJ3P, FCER2, GTSF1L, CPN2, CCDC26, SIGLEC14, CD79B, AC083949.1, TBATA, TRGV3, AC008649.1, AC016168.1, AC007381.1, AL512378.1, SCARNA5, PCDH11X, DEFA3, AIM2, ANKRD20A19P, SLC7A10, AL031733.2, SNORA54, AL121985.3, AC243960.7, FCRL4, AC104699.1, AL161781.2, MIR4538, AC008878.3, TM4SF19-TCTEX1D2, TRAV1-1 LINC02397, SERPINA9, IL13, SIRPB3P, HLA-DQA2, SPATA21, CD79A, ZNF560, IL24, BEND4, LINC02132, CR2, AC105105.1, MIR4537, AP002957.1, TNMD, SNORD15B, AP001994.1, AC104971.3, LINC02244, AL162457.1, DNAH8, AC006946.3, RNU4-2, AL031429.1, ZNF80 |
A total of 446 DEGs were identified, including 26 upregulated genes and 420 downregulated genes. TCGA, The Cancer Genome Atlas; DEGs, differentially expressed genes.
Table S3
Gene | HR | Z | P value |
---|---|---|---|
SCN11A | 0.703639506 | −3.75906501 | 0.00017055 |
B3GNT3 | 1.438868912 | 3.588130084 | 0.000333058 |
CRHBP | 0.776088386 | −3.57570495 | 0.000349285 |
STYK1 | 1.363523877 | 3.508204535 | 0.000451142 |
CARMIL2 | 0.756703186 | −3.503799083 | 0.000458671 |
TSPAN1 | 1.345648032 | 3.502539144 | 0.000460846 |
CCM2L | 0.66083542 | −3.443526972 | 0.000574179 |
KCNK1 | 1.400354154 | 3.357058121 | 0.000787766 |
SH3TC2 | 1.298382346 | 3.197651888 | 0.001385514 |
ABCA12 | 1.191687987 | 3.149919639 | 0.001633154 |
MMP12 | 1.179596007 | 3.091184704 | 0.001993596 |
SPDEF | 1.155409242 | 3.071293664 | 0.002131334 |
PPP1R14D | 1.203202385 | 2.998880938 | 0.002709732 |
DNASE1L3 | 0.835728686 | −2.993100039 | 0.002761592 |
CXCL6 | 1.140309517 | 2.776945264 | 0.005487242 |
SCN4A | 0.832208745 | −2.736346206 | 0.006212563 |
CHRNA4 | 0.749149411 | −2.711469636 | 0.006698568 |
CDHR1 | 0.825456763 | −2.703393517 | 0.006863544 |
KCNJ10 | 0.777298851 | −2.692655943 | 0.007088538 |
C12orf42 | 0.833587002 | −2.692401593 | 0.007093947 |
ACAP1 | 0.755658934 | −2.561809842 | 0.010412831 |
KRT79 | 1.181711465 | 2.488663846 | 0.012822414 |
SLC7A11 | 1.182505672 | 2.451743156 | 0.01421661 |
SLC7A10 | 0.867313614 | −2.405796106 | 0.016137268 |
AIM2 | 1.145824966 | 2.391443842 | 0.016782251 |
FXYD3 | 1.187844904 | 2.343005963 | 0.019129077 |
CHI3L1 | 1.144437783 | 2.326983781 | 0.019966128 |
BEND4 | 0.878285206 | −2.261701774 | 0.023715835 |
IL13 | 0.796884219 | −2.261683447 | 0.023716968 |
CFP | 0.827171237 | −2.24864359 | 0.024535181 |
CD160 | 0.740642374 | −2.234866064 | 0.025426143 |
TLX1 | 1.130492663 | 2.210796342 | 0.027049943 |
REELD1 | 0.828394267 | −2.20007862 | 0.027801317 |
LRTM1 | 0.72930414 | −2.171415905 | 0.029899748 |
CETP | 0.795211505 | −2.16555869 | 0.030344926 |
FGFBP2 | 0.82872131 | −2.134770338 | 0.032779774 |
TREML2 | 1.124525193 | 2.116437642 | 0.034307603 |
FAM129C | 0.901979278 | −2.053300195 | 0.040043472 |
PPP1R16B | 0.823390068 | −2.053220159 | 0.04005123 |
MATK | 0.812586746 | −2.029752065 | 0.042381748 |
GDF7 | 0.861947085 | −2.003268123 | 0.045148517 |
CD36 | 0.887148466 | −1.993301358 | 0.046228458 |
SLC4A1 | 0.837601622 | −1.980687455 | 0.047626334 |
Acknowledgments
Funding: This work is supported by Grants from
Footnote
Conflicts of Interest: All authors have completed the ICMJE uniform disclosure form (available at http://dx.doi.org/10.21037/tcr-19-2873). The authors have no conflicts of interest to declare.
Ethical Statement: The authors are accountable for all aspects of the work in ensuring that questions related to the accuracy or integrity of any part of the work are appropriately investigated and resolved. All of the data can be obtained for free online, so ethical review is exempt.
Open Access Statement: This is an Open Access article distributed in accordance with the Creative Commons Attribution-NonCommercial-NoDerivs 4.0 International License (CC BY-NC-ND 4.0), which permits the non-commercial replication and distribution of the article with the strict proviso that no changes or edits are made and the original work is properly cited (including links to both the formal publication through the relevant DOI and the license). See: https://creativecommons.org/licenses/by-nc-nd/4.0/.
References
- Siegel RL, Miller KD, Jemal A. Cancer statistics, 2018. CA Cancer J Clin 2018;68:7-30. [Crossref] [PubMed]
- Vasen H, Ibrahim I, Ponce C, et al. Benefit of Surveillance for Pancreatic Cancer in High-Risk Individuals: Outcome of Long-Term Prospective Follow-Up Studies From Three European Expert Centers. J Clin Oncol 2016;34:2010-9. [Crossref] [PubMed]
- Moletta L, Serafini S, Valmasoni M, et al. Surgery for Recurrent Pancreatic Cancer: Is It Effective? Cancers (Basel) 2019;11:991. [Crossref] [PubMed]
- Koren E, Fuchs Y. The bad seed: cancer stem cells in tumor development and resistance. Drug Resist. Updat 2016;28:1-12. [Crossref] [PubMed]
- Chiorean E, Cheung W, Giordano G, et al. Real-world comparative effectiveness of nab-paclitaxel plus gemcitabine FOLFIRINOX in advanced pancreatic cancer: a systematic review. Ther Adv Med Oncol 2019;11:1758835919850367. [Crossref] [PubMed]
- Papneja N, Zaidi A, Chalchal H, et al. Comparisons of Outcomes of Real-World Patients With Advanced Pancreatic Cancer Treated With FOLFIRINOX Versus Gemcitabine and Nab-Paclitaxel: a Population-Based Cohort Study. Pancreas 2019;48:920-6. [Crossref] [PubMed]
- Biankin A, Waddell N, Kassahn K, et al. Pancreatic cancer genomes reveal aberrations in axon guidance pathway genes. Nature 2012;491:399-405. [Crossref] [PubMed]
- Witkiewicz A, McMillan E, Balaji U, et al. Whole-exome sequencing of pancreatic cancer defines genetic diversity and therapeutic targets. Nat Commun 2015;6:6744. [Crossref] [PubMed]
- Birnbaum D, Bertucci F, Finetti P, et al. Molecular classification as prognostic factor and guide for treatment decision of pancreatic cancer. Biochim Biophys Acta Rev Cancer 2018;1869:248-55.
- Castillo L, Young A, Mawson A, et al. MCL-1 antagonism enhances the anti-invasive effects of dasatinib in pancreatic adenocarcinoma. Oncogene 2020;39:1821-9. [Crossref] [PubMed]
- Dart D, Arisan D, Owen S, et al. Wnt-11 Expression Promotes Invasiveness and Correlates with Survival in Human Pancreatic Ductal Adeno Carcinoma. Genes (Basel) 2019;10:921. [Crossref] [PubMed]
- Han L, Zan Y, Huang C, et al. NELFE promoted pancreatic cancer metastasis and the epithelial-to-mesenchymal transition by decreasing the stabilization of NDRG2 mRNA. Int J Oncol 2019;55:1313-23. [PubMed]
- Lytle N, Ferguson L, Rajbhandari N, et al. A Multiscale Map of the Stem Cell State in Pancreatic Adenocarcinoma. Cell 2019;177:572-86.e22. [Crossref] [PubMed]
- Zhang X, Zhang W, Jiang Y, et al. Identification of functional lncRNAs in gastric cancer by integrative analysis of GEO and TCGA data. J Cell Biochem 2019;120:17898-911. [Crossref] [PubMed]
- Chandrashekar D, Bashel B, Balasubramanya S, et al. UALCAN: a Portal for Facilitating Tumor Subgroup Gene Expression and Survival Analyses. Neoplasia 2017;19:649-58. [Crossref] [PubMed]
- Lee C, Im E, Moon P, et al. Discovery of a diagnostic biomarker for colon cancer through proteomic profiling of small extracellular vesicles. BMC Cancer 2018;18:1058. [Crossref] [PubMed]
- Munkley J, McClurg U, Livermore K, et al. The cancer-associated cell migration protein TSPAN1 is under control of androgens and its upregulation increases prostate cancer cell migration. Sci Rep 2017;7:5249. [Crossref] [PubMed]
- Duan J, Liu J, Liu Y, et al. miR-491-3p suppresses the growth and invasion of osteosarcoma cells by targeting TSPAN1. Mol Med Rep 2017;16:5568-74. [Crossref] [PubMed]
- Hou F, Lei X, Yao J, et al. Tetraspanin 1 is involved in survival, proliferation and carcinogenesis of pancreatic cancer. Oncol Rep 2015;34:3068-76. [Crossref] [PubMed]
- Zhang X, Shi G, Gao F, et al. TSPAN1 upregulates MMP2 to promote pancreatic cancer cell migration and invasion via PLCγ. Oncol Rep 2019;41:2117-25. [PubMed]
- Tian J, Zhang R, Piao H, et al. Silencing Tspan1 inhibits migration and invasion, and induces the apoptosis of human pancreatic cancer cells. Mol Med Rep 2018;18:3280-8. [PubMed]
- Dolce V, Cappello A, Lappano R, et al. Glycerophospholipid synthesis as a novel drug target against cancer. Curr Mol Pharmacol 2011;4:167-75. [Crossref] [PubMed]
- Hollenback D, Bonham L, Law L, et al. Substrate specificity of lysophosphatidic acid acyltransferase beta -- evidence from membrane and whole cell assays. J Lipid Res 2006;47:593-604. [Crossref] [PubMed]
- Zang H, Huang G, Ju H, et al. Integrative analysis of the inverse expression patterns in pancreas development and cancer progression. World J Gastroenterol 2019;25:4727-38. [Crossref] [PubMed]
- M de-Brito N, Duncan-Moretti J, C da-Costa H, et al. Aerobic glycolysis is a metabolic requirement to maintain the M2-like polarization of tumor-associated macrophages. Biochim Biophys Acta Mol Cell Res 2020;1867:118604.
- Hanahan D, Weinberg R. Hallmarks of cancer: the next generation. Cell 2011;144:646-74. [Crossref] [PubMed]
- Collisson E, Sadanandam A, Olson P, et al. Subtypes of pancreatic ductal adenocarcinoma and their differing responses to therapy. Nat Med 2011;17:500-3. [Crossref] [PubMed]
- Daemen A, Peterson D, Sahu N, et al. Metabolite profiling stratifies pancreatic ductal adenocarcinomas into subtypes with distinct sensitivities to metabolic inhibitors. Proc Natl Acad Sci U S A 2015;112:e4410-7. [Crossref] [PubMed]
- Liberti M, Locasale J. The Warburg Effect: How Does it Benefit Cancer Cells? Trends Biochem Sci 2016;41:211-8. [Crossref] [PubMed]
- Lu J. The Warburg metabolism fuels tumor metastasis. Cancer Metastasis Rev 2019;38:157-64. [Crossref] [PubMed]
- Guillaumond F, Iovanna J, Vasseur S. Pancreatic tumor cell metabolism: focus on glycolysis and its connected metabolic pathways. Arch Biochem Biophys 2014;545:69-73. [Crossref] [PubMed]
- Wopereis S, Lefeber D, Morava E, et al. Mechanisms in protein O-glycan biosynthesis and clinical and molecular aspects of protein O-glycan biosynthesis defects: a review. Clin Chem 2006;52:574-600. [Crossref] [PubMed]
- Moremen K, Tiemeyer M, Nairn A. Vertebrate protein glycosylation: diversity, synthesis and function. Nat Rev Mol Cell Biol 2012;13:448-62. [Crossref] [PubMed]
- Wagner K, Punnoose E, Januario T, et al. Death-receptor O-glycosylation controls tumor-cell sensitivity to the proapoptotic ligand Apo2L/TRAIL. Nat Med 2007;13:1070-7. [Crossref] [PubMed]
- Hofmann B, Schlüter L, Lange P, et al. COSMC knockdown mediated aberrant O-glycosylation promotes oncogenic properties in pancreatic cancer. Mol Cancer 2015;14:109. [Crossref] [PubMed]
- Hager K, Gu W. Understanding the non-canonical pathways involved in p53-mediated tumor suppression. Carcinogenesis 2014;35:740-6. [Crossref] [PubMed]
- Junttila M, Karnezis A, Garcia D, et al. Selective activation of p53-mediated tumour suppression in high-grade tumours. Nature 2010;468:567-71. [Crossref] [PubMed]
- Bertrand P, Saintigny Y, Lopez B. p53's double life: transactivation-independent repression of homologous recombination. Trends Genet 2004;20:235-43. [Crossref] [PubMed]
- Domínguez-Bendala J, Priddle H, Clarke A, et al. Elevated expression of exogenous Rad51 leads to identical increases in gene-targeting frequency in murine embryonic stem (ES) cells with both functional and dysfunctional p53 genes. Exp Cell Res 2003;286:298-307. [Crossref] [PubMed]
- Wang J, Liu B, Du Z, et al. p53-dependent transcriptional suppression of BAG3 protects cells against metabolic stress via facilitation of p53 accumulation. J Cell Mol Med 2020;24:562-72. [Crossref] [PubMed]
- Du Y, Liu Z, You L, et al. Pancreatic Cancer Progression Relies upon Mutant p53-Induced Oncogenic Signaling Mediated by NOP14. Cancer Res 2017;77:2661-73. [Crossref] [PubMed]
- Garcia M, Nelson W, Chavez N. Cell-Cell Junctions Organize Structural and Signaling Networks. Cold Spring Harb Perspect Biol 2018;10:a029181. [Crossref] [PubMed]
- Van I, Anderson J. Architecture of tight junctions and principles of molecular composition. Semin. Cell Dev Biol 2014;36:157-65. [Crossref] [PubMed]
- Rahner C, Mitic L, Anderson J. Heterogeneity in expression and subcellular localization of claudins 2, 3, 4, and 5 in the rat liver, pancreas, and gut. Gastroenterology 2001;120:411-22. [Crossref] [PubMed]
- Yáñez-Mó M, Barreiro O, Gordon-Alonso M, et al. Tetraspanin-enriched microdomains: a functional unit in cell plasma membranes. Trends Cell Biol 2009;19:434-46. [Crossref] [PubMed]
- Huang C, Fu C, Wren J, et al. Correction to: tetraspanin-enriched microdomains regulate digitation junctions. Cell Mol Life Sci 2018;75:4077. [Crossref] [PubMed]
- Karki R, Man S, Kanneganti T. Inflammasomes and Cancer. Cancer Immunol Res 2017;5:94-99. [Crossref] [PubMed]
- Sharma B, Karki R, Kanneganti T. Role of AIM2 inflammasome in inflammatory diseases, cancer and infection. Eur J Immunol 2019;49:1998-2011. [Crossref] [PubMed]
- Li C, Zhang Y, Cheng X, et al. PINK1 and PARK2 Suppress Pancreatic Tumorigenesis through Control of Mitochondrial Iron-Mediated Immunometabolism. Dev Cell 2018;46:441-55.e8. [Crossref] [PubMed]
- Mitoma J, Petryniak B, Hiraoka N, et al. Extended core 1 and core 2 branched O-glycans differentially modulate sialyl Lewis X-type L-selectin ligand activity. J Biol. Chem 2003;278:9953-61. [Crossref] [PubMed]
- Ho W, Che M, Chou C, et al. B3GNT3 expression suppresses cell migration and invasion and predicts favorable outcomes in neuroblastoma. Cancer Sci 2013;104:1600-8. [Crossref] [PubMed]
- Avraham S, Jiang S, Ota S, et al. Structural and functional studies of the intracellular tyrosine kinase MATK gene and its translated product. J Biol Chem 1995;270:1833-42. [Crossref] [PubMed]
- Fu Y, Zagozdzon R, Avraham R, et al. CHK negatively regulates Lyn kinase and suppresses pancreatic cancer cell invasion. Int J Oncol 2006;29:1453-8. [PubMed]
- Mori Y, Olaru A, Cheng Y, et al. Novel candidate colorectal cancer biomarkers identified by methylation microarray-based scanning. Endocr Relat Cancer 2011;18:465-78. [Crossref] [PubMed]
- Kettunen E, Hernandez-Vargas H, Cros M, et al. Asbestos-associated genome-wide DNA methylation changes in lung cancer. Int J Cancer 2017;141:2014-29. [Crossref] [PubMed]