Identification of tumor microenvironment-related genes in lower-grade gliomas by mining TCGA database
Introduction
Lower-grade gliomas (LGG) are ubiquitous and fatal branches of brain neoplasm. The World Health Organization has classified LGG as grade II or grade III tumors, including three subtypes: (I) astrocytoma, IDH wild-type (Astro-wIDH); (II) astrocytoma, IDH-mutant and 1p/19q non-codeleted (Astro-mIDH-1p/19q non-codel); (III) oligodendroglioma, IDH-mutant and 1p/19q-codeleted (Oligo-mIDH-1p/19q codel) (1). The median survival time (MST) of LGG patients is 1.7–8.0 years (2). One way to meet the needs of better cancer diagnosis, treatment, and prevention was to establish TCGA dataset, which has provided a vast amount of available data for researchers around the world (3). In that regard, we downloaded clinical data and the mRNA expression profiling of LGG from TCGA for further analysis.
Tumor growth is closely linked to the surrounding environment, including epithelial and stromal cells, vascular and lymphatic vessels, immune cells and inflammatory cells (4). In tumor microenvironment, immune and stromal cells play a central role and make significant contributions to tumor progression and clinical outcomes (5-7). For improvement in detecting tumor purity in tumor tissues, an algorithm called ESTIMATE (Estimation of Stromal and Immune cells in MAlignant Tumor tissues using Expression data) was designed; it uses specific gene expression signatures to predict the extent of infiltrating stromal and immune cells (8).
In the current study, we first explored the correlation between immune/stromal scores and prognosis in different subgroups of LGG. Our result revealed that only in Astro-wIDH subgroup the scores were notably related to prognosis. Furtherly, a comprehensive bioinformatics analysis was employed, accordingly, a series of genes that have significant impacts on prognosis and are significantly associated with the tumor microenvironment were discovered. Lastly, we used the data in the CGGA database to validate the selected genes.
Methods
Data obtaining from the TCGA dataset and CGGA dataset
The gene expression data (level 3 data) from LGG patient samples were downloaded from TCGA dataset (http://gdc-portal.nci.nih.gov). The data obtained from TCGA also included phenotype, mutation, and prognosis scores for the downloaded data described above. Gene expression profiles and clinical information of LGG patients were collected from CGGA dataset (http://www.cgga.org.cn/) and used to verify the reliability of the TCGA data. Two batches were found (mRNAseq_693 and mRNAseq_325), and their platforms are all the Illumina HiSeq 4,000.
DEGs identification
Using the limma package, we selected DEGs between the high-score group and the low-score group. In the current study, we set the cut-off standard as Fold change >2 and P<0.05 to identify DEGs.
Clustering analysis
Heatmaps and clustering analysis were performed to show the hierarchical clustering of DEGs for samples with high scores and low scores by applying web-based tools of the Morpheus (https://software.broadinstitute.org/morpheus/).
Functional enrichment analysis
DAVID, a web-based tool for functional annotation, was used to explore the biological property of DEGs. GO analysis was conducted, including the biological process, molecular function, and cellular component. KEGG analysis was also performed using DAVID.
Survival analysis
The Kaplan-Meier method was used to identify genes significantly associated with OS, and the significance of the relationship between gene expression level and the OS of LGG patients was calculated using the log-rank test.
Protein-protein interaction network generation and module analysis
The PPI network was searched from the STRING database to establish potential relationships between DEGs. A confidence score ≥0.4 was set as the cut-off standard to reserve the credible PPIs. Subsequently, the PPIs from the STRING database were imported into Cytoscape to reconstruct the PPIs network of selected DEGs. MCODE, a useful app of the Cytoscape plug-in, was used to screen hub modules in the PPI network. Modules with nodes ≥10 and score ≥4 were reserved for further analysis. We also calculated the degree of each node in the network. For screening based on detected modules and the degree, hub genes were selected.
Results
Immune/stromal scores are significantly associated with Astro-wIDH prognosis but not other subtypes
The clinical data of 530 LGG patient samples were acquired from the TCGA dataset. Among these samples, 238 (44.9%) were female, 291 (54.9%) were male, and 1 (0.19%) was unlabeled. The proportions of the various subtypes in these samples were 97 Astro-wIDH cases, 264 Astro-mIDH-1p/19q non-codel cases, and 169 Oligo-mIDH-1p/19q codel cases. Immune and stromal scores were calculated using the ESTIMATE algorithm; immune scores ranged from −2,012.46 to 2,236.6, and stromal scores from −1,832.4 to 1,759.22. Astro-wIDH cases had the highest average immune and stromal scores, followed by Astro-mIDH-1p/19q non-codel, and lastly by Oligo-mIDH-1p/19q codel with the lowest scores (Figure 1A,B, P<0.0001). The MST of patients with each subtype was 1.7 years for Astro-wIDH, 6.3 years for Astro-mIDH-1p/19q non-codel, and 8.0 years for Oligo-mIDH-1p/19q codel (2). Interestingly, the rank order of MST is consistent with the order of immune and stromal scores. To figure out whether OS was related to immune scores and/or stromal scores, the Kaplan-Meier survival analysis was performed. First, we assigned the all samples into high-score and low-score groups. The Kaplan-Meier survival analysis shown that immune/stromal scores correlated with OS, and the low-score group had higher median survival than that of the high-score group in both cases, that is, stromal scores (4,068 vs. 2,052 d, P=0.0024 Figure 1C) and immune scores (2,907 vs. 2,052 d, P=0.0146 Figure 1D). Similarly, we performed further survival analysis for each subtype based on immune scores and stromal scores. The result showed that the immune/stromal scores were only significantly associated with OS in Astro-wIDH subtype (Figure 1E,F,G,H,I,J). Overall, these analyses indicated that immune/stromal scores were significantly associated with LGG subtypes, and immune/stromal scores only correlated notably with Astro-wIDH prognosis but not other subtypes.
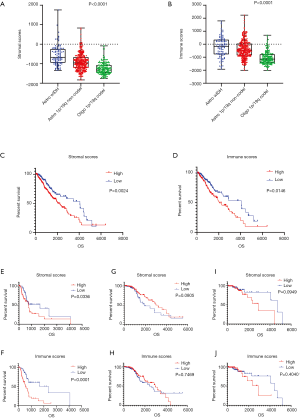
Identification DEGs
Patients with Astro-wIDH subtype of LGG whose tumor mRNA had been sequenced were collected from TCGA dataset, and the effect of immune and stromal scores on mRNA expression in the LGG tumors was examined. The Bioconductor package, limma was applied in the selection of DEGs between the high-score and the low-score group, setting Fold change >2 and P<0.05 as the cut-off. Based on immune scores, a total of 1,432 DEGs were detected, containing 920 upregulated genes and 512 downregulated genes. Similarly, 858 genes were upregulated and 382 were downregulated in high stromal score group contrast with low stromal score group. All the genes were plotted, such that red dots represented the DEGs, and black dots represented genes that were screened out, as shown in Figure 2A,B.
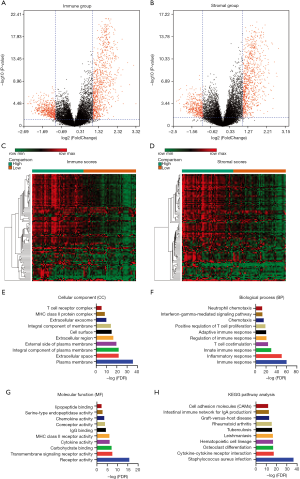
Next, a cluster analysis using the Morpheus web-tool was performed by comparing gene expression profiles between the high-score group and the low-score group, and these genes were well clustered as shown in Figure 2C,D. A functional enrichment and pathway analysis were performed of 743 DEGs upregulated in both the high immune score group and the high stromal score group to determine the potential function of DEGs. For the GO analysis, DEGs were significantly enriched in the extracellular space, immune/inflammatory response and receptor activity (Figure 2 E,F,G,H).
Survival analysis
We used log-rank test and the Kaplan-Meier survival method to examine the relationship between the 743 upregulated DEGs and the OS of LGG patients in a bit to explore DEGs’ ability to affect patient prognosis. According to this analysis, the presence of a total of 486 DEGs correlated significantly with OS (P<0.05, Figure 3).
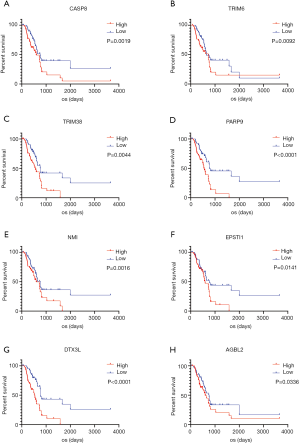
PPI network construction and Mcode analysis
To better investigate the interaction among the selected DEGs, we submitted the DEGs screened out by the survival analysis to the STRING database. The network included 573 nodes, and 6,469 edges were obtained using the cutoff standard of a median confidence ≥0.4. Based on the Mcode analysis, 7 modules were established in the network. The top three modules with the highest credibility scores were detected for further investigation and plotted in Figure 4. Among three modules, IL10, TLR2, ITGB2, and SPI1 in module A, PTPRC, CD86, ITGAM, and TYROBP in module B, and LCP2, BTK, and SYK in module C, were the hub nodes with higher degrees.
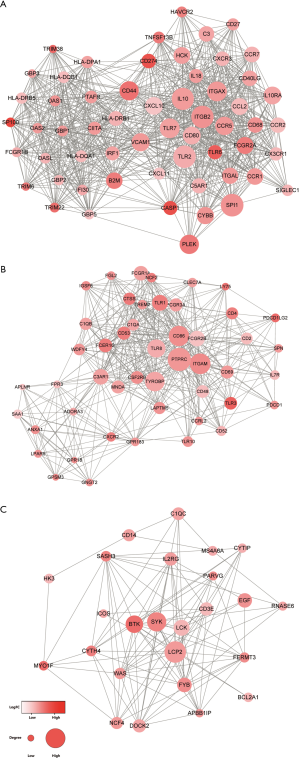
Functional analysis of selected modules
All the genes in the three modules obtained through the PPI network analysis were submitted to DAVID, as shown in Figure 5. For cell component, DEGs were significantly enriched in the plasma membrane, cell surface, and receptor of complex (Figure 5A). Biological process mainly displayed immune and inflammatory responses (Figure 5B). Molecular function included chemokine receptor activity and cytokine binding (Figure 5C). Additionally, the KEGG analysis showed that genes were mostly involved in cell adhesion molecules (Figure 5D).
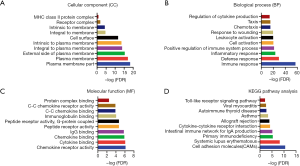
Validation using CGGA database
We obtained two batches of gene expression profiles and corresponding clinical information of LGG patient samples from the CGGA database to examine whether the DEGs selected from TCGA database were also significantly associated with prognosis in other databases. A total of 25 genes were verified to be notably associated with prognosis. Based on the result of the PPI network analysis, we selected 8 genes with higher degree values from the validation of DEGs for further analysis (P<0.05, Figure 6).
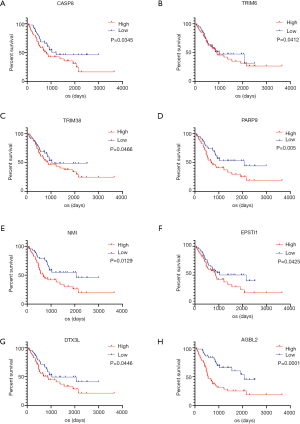
Discussion
Currently, we have obtained DEGs related to tumor microenvironment by mining the TCGA database. Among them, 486 DEGs were significantly associated with prognosis, and 25 were validated by the CGGA database.
Firstly, we originally explored the association between immune/stromal scores and prognosis in different subtypes of LGG. The result shown that immune/stromal scores were strongly associated with Astro-wIDH prognosis, but not in other subtypes. Thus, further analyses were all performed in astrocytoma, IDH-wildtype cases. Our discussion of LGG subtypes also provides an idea for similar bioinformatics analysis. Secondly, we analyzed 743 differentially expressed genes obtained by comparing high and low scores. GO analysis showed that DEGs were primarily enriched by tumor microenvironments, including extracellular space, immune/inflammatory response, and Cytokine-cytokine receptor interaction. Our result was consistent with previous reports that tumor microenvironment was significantly associated with the progression of gliomas (9-11).
Next, we performed survival analysis on 743 DEGs, and a total of 486 DEGs were screened for significant prognosis. We also built 7 PPI modules using the STRING database and Cytoscape. Furthermore, GO analysis and KEGG pathway analysis were performed on the top three significant modules, and the result of enrichment analyses demonstrated that all modules were significantly associated with immune response. IL10, TLR2, and ITGB2, all remarkable nodes in the PPI modules, have been shown to be involved in the tumorigenesis of gliomas (12-17).
Finally, we validated DEGs based on the CGGA database. A total of 25 genes, which strongly correlated with prognosis both in the TCGA and CGGA databases, were screened. Based on the result of the PPI network analysis, we selected 8 genes with higher degree values from validated DEGs (Figure 6). These selected genes included the caspase CASP8, Tripartite Motif Containing TRIM6 and TRIM38, poly(ADP-ribose) polymerase family PARP9, N-myc and STAT interactor NMI, Epithelial Stromal Interaction EPSTO1, Deltex E3 Ubiquitin Ligase DTX3L and ATP/GTP Binding Protein AGBL2.
CASP8, a canonical cysteine protease, is known for its roles in death receptor-induced programmed cell death. However, CASP8 also plays a number of non-apoptotic roles, such as correlated with the progress of cell adhesion and cell migration (18,19) and promoting NF-κB activity (20). Moreover, CASP8 was found to promote the expression and secretion of VEGF, IL6 and IL8 through activating NF-kB in human GBM cell lines, resulting in neovascularization and increased resistance to Temozolomide (21). Accumulating evidence indicates that CASP8 may have potential prognostic value for gliomas.
N-myc and STAT interactor, NMI, was originally recognized as an interactor of N-myc and C-myc oncogenes (22). Zhu et al. furtherly demonstrated that NMI cooperate with several members of STAT and augmented the JAK/STAT pathway (23), which has been found to be positively related to tumor growth, progression and metastasis (24). Moreover, it also shown that higher expression of NMI promotes tumor growth by regulating G1/S phase progression, leading to poor prognosis in human glioblastoma (25).
AS a ubiquitin ligase, DTX3L was found to be critically related to the tumorigenesis of various tumors (26,27). Furthermore, it was reported that DTX3L was overexpressed in glioma and correlated significantly with glioma progression (28). PARP9, also known as BAL1, was first identified as an upregulated gene in malignant B cell lymphoma (29), and further found to correlate with metastasis, progression and recurrence in a variety of tumors (30-32). Previous study has shown that DTX3L and PARP9 were jointly involved in the STAT1 signaling (33). Interestingly, an overexpression of STAT1 has been found in glioblastoma, which predicted poor prognosis (34). Up to now, the exact role of TRIM6, TRIM38, EPSTI1 and ATGB2 in gliomas have not been elucidated, which may serve as potential targets for the diagnosis and treatment of gliomas.
Conclusions
Based on the ESTIMATE algorithm, we first explored the relationship between immune/stromal scores and prognosis in different subtypes of LGG and the result shown that the scores were only strongly associated with the prognosis of astrocytoma, IDH-wildtype. Furtherly, a comprehensive bioinformatics analysis of the gene expression profiles of Astro-wIDH was conducted, and crucial genes were identified. These genes are validated in the CGGA database and were significantly associated with prognosis. All DEGs may be involved in the occurrence, development, and invasion of LGG, but some previously unreported genes identified here could serve as potential biomarkers for LGG. However, in addition to exploratory research in bioinformatics, definitive work is still needed to determine the function of these genes.
Acknowledgments
Funding: We are grateful for the support of
Footnote
Conflicts of Interest: Both authors have completed the ICMJE uniform disclosure form (available at http://dx.doi.org/10.21037/tcr-20-1079). The authors have no conflicts of interest to declare.
Ethical Statement: The authors are accountable for all aspects of the work in ensuring that questions related to the accuracy or integrity of any part of the work are appropriately investigated and resolved.
Open Access Statement: This is an Open Access article distributed in accordance with the Creative Commons Attribution-NonCommercial-NoDerivs 4.0 International License (CC BY-NC-ND 4.0), which permits the non-commercial replication and distribution of the article with the strict proviso that no changes or edits are made and the original work is properly cited (including links to both the formal publication through the relevant DOI and the license). See: https://creativecommons.org/licenses/by-nc-nd/4.0/.
References
- Wesseling P, Capper D. WHO 2016 Classification of gliomas. Neuropathol Appl Neurobiol 2018;44:139-50. [Crossref] [PubMed]
- Brat DJ, Verhaak RG, Aldape KD, et al. Comprehensive, Integrative Genomic Analysis of Diffuse Lower-Grade Gliomas. N Engl J Med 2015;372:2481-98. [Crossref] [PubMed]
- Tomczak K, Czerwinska P, Wiznerowicz M. The Cancer Genome Atlas (TCGA): an immeasurable source of knowledge. Contemp Oncol (Pozn) 2015;19:A68-77. [Crossref] [PubMed]
- Coussens LM, Werb Z. Inflammation and cancer. Nature 2002;420:860-7. [Crossref] [PubMed]
- Li H, Fan X, Houghton J. Tumor microenvironment: the role of the tumor stroma in cancer. J Cell Biochem 2007;101:805-15. [Crossref] [PubMed]
- Fridman WH, Dieu-Nosjean MC, Pages F, et al. The immune microenvironment of human tumors: general significance and clinical impact. Cancer Microenviron 2013;6:117-22. [Crossref] [PubMed]
- Chen L, Bi S, Hou J, et al. Targeting p21-activated kinase 1 inhibits growth and metastasis via Raf1/MEK1/ERK signaling in esophageal squamous cell carcinoma cells. Cell Commun Signal 2019;17:31. [Crossref] [PubMed]
- Yoshihara K, Shahmoradgoli M, Martinez E, et al. Inferring tumour purity and stromal and immune cell admixture from expression data. Nat Commun 2013;4:2612. [Crossref] [PubMed]
- Silver DJ, Sinyuk M, Vogelbaum MA, et al. The intersection of cancer, cancer stem cells, and the immune system: therapeutic opportunities. Neuro Oncol 2016;18:153-9. [Crossref] [PubMed]
- Pickup MW, Mouw JK, Weaver VM. The extracellular matrix modulates the hallmarks of cancer. EMBO Rep 2014;15:1243-53. [Crossref] [PubMed]
- Peranzoni E, Rivas-Caicedo A, Bougherara H, et al. Positive and negative influence of the matrix architecture on antitumor immune surveillance. Cell Mol Life Sci 2013;70:4431-48. [Crossref] [PubMed]
- Li C, Ma L, Liu Y, et al. TLR2 promotes development and progression of human glioma via enhancing autophagy. Gene 2019;700:52-9. [Crossref] [PubMed]
- Qian J, Luo F, Yang J, et al. TLR2 Promotes Glioma Immune Evasion by Downregulating MHC Class II Molecules in Microglia. Cancer Immunol Res 2018;6:1220-33. [PubMed]
- Hishii M, Nitta T, Ishida H, et al. Human glioma-derived interleukin-10 inhibits antitumor immune responses in vitro. Neurosurgery 1995;37:1160-6; discussion 1166-7. [Crossref] [PubMed]
- Huettner C, Czub S, Kerkau S, et al. Interleukin 10 is expressed in human gliomas in vivo and increases glioma cell proliferation and motility in vitro. Anticancer Res 1997;17:3217-24. [PubMed]
- Rajaraman P, Brenner AV, Butler MA, et al. Common variation in genes related to innate immunity and risk of adult glioma. Cancer Epidemiol Biomarkers Prev 2009;18:1651-8. [Crossref] [PubMed]
- Wagner S, Czub S, Greif M, et al. Microglial/macrophage expression of interleukin 10 in human glioblastomas. Int J Cancer 1999;82:12-6. [Crossref] [PubMed]
- Graf RP, Keller N, Barbero S, et al. Caspase-8 as a regulator of tumor cell motility. Curr Mol Med 2014;14:246-54. [Crossref] [PubMed]
- Keller N, Ozmadenci D, Ichim G, et al. Caspase-8 function, and phosphorylation, in cell migration. Semin Cell Dev Biol 2018;82:105-17. [Crossref] [PubMed]
- Su H, Bidere N, Zheng L, et al. Requirement for caspase-8 in NF-kappaB activation by antigen receptor. Science 2005;307:1465-8. [Crossref] [PubMed]
- Fianco G, Mongiardi MP, Levi A, et al. Caspase-8 contributes to angiogenesis and chemotherapy resistance in glioblastoma. Elife 2017;6:e22593. [Crossref] [PubMed]
- Bao J, Zervos AS. Isolation and characterization of Nmi, a novel partner of Myc proteins. Oncogene 1996;12:2171-6. [PubMed]
- Zhu M, John S, Berg M, et al. Functional association of Nmi with Stat5 and Stat1 in IL-2- and IFNgamma-mediated signaling. Cell 1999;96:121-30. [Crossref] [PubMed]
- Stark GR, Darnell JE Jr. The JAK-STAT pathway at twenty. Immunity 2012;36:503-14. [Crossref] [PubMed]
- Meng D, Chen Y, Yun D, et al. High expression of N-myc (and STAT) interactor predicts poor prognosis and promotes tumor growth in human glioblastoma. Oncotarget 2015;6:4901-19. [Crossref] [PubMed]
- Yan Q, Xu R, Zhu L, et al. BAL1 and its partner E3 ligase, BBAP, link Poly(ADP-ribose) activation, ubiquitylation, and double-strand DNA repair independent of ATM, MDC1, and RNF8. Mol Cell Biol 2013;33:845-57. [Crossref] [PubMed]
- Thang ND, Yajima I, Kumasaka MY, et al. Deltex-3-like (DTX3L) stimulates metastasis of melanoma through FAK/PI3K/AKT but not MEK/ERK pathway. Oncotarget 2015;6:14290-9. [Crossref] [PubMed]
- Xu P, Tao X, Zhao C, et al. DTX3L is upregulated in glioma and is associated with glioma progression. Int J Mol Med 2017;40:491-8. [Crossref] [PubMed]
- Aguiar RC, Yakushijin Y, Kharbanda S, et al. BAL is a novel risk-related gene in diffuse large B-cell lymphomas that enhances cellular migration. Blood 2000;96:4328-34. [Crossref] [PubMed]
- Juszczynski P, Kutok JL, Li C, et al. BAL1 and BBAP are regulated by a gamma interferon-responsive bidirectional promoter and are overexpressed in diffuse large B-cell lymphomas with a prominent inflammatory infiltrate. Mol Cell Biol 2006;26:5348-59. [Crossref] [PubMed]
- Bachmann SB, Frommel SC, Camicia R, et al. DTX3L and ARTD9 inhibit IRF1 expression and mediate in cooperation with ARTD8 survival and proliferation of metastatic prostate cancer cells. Mol Cancer 2014;13:125. [Crossref] [PubMed]
- Tang X, Zhang H, Long Y, et al. PARP9 is overexpressed in human breast cancer and promotes cancer cell migration. Oncol Lett 2018;16:4073-7. [PubMed]
- Zhang Y, Mao D, Roswit WT, et al. PARP9-DTX3L ubiquitin ligase targets host histone H2BJ and viral 3C protease to enhance interferon signaling and control viral infection. Nat Immunol 2015;16:1215-27. [Crossref] [PubMed]
- Thota B, Arimappamagan A, Kandavel T, et al. STAT-1 expression is regulated by IGFBP-3 in malignant glioma cells and is a strong predictor of poor survival in patients with glioblastoma. J Neurosurg 2014;121:374-83. [Crossref] [PubMed]