Expression and regulatory network of miRNAs in head and neck squamous cell carcinoma
Introduction
The incidence rate of head and neck squamous cell carcinoma (HNSCC) is rising. With about 650,000 new cases annually, HNSCC has the sixth-highest global incidence rate of all malignant tumors (1). Complex anatomical types and extensive molecular changes induce significant heterogeneity in HNSCC, which affects the prognosis of patients and further limits the progress of molecular mechanism research (2). To date, many researchers have attempted to explore the significant number of functional molecules and molecular mechanisms in HNSCC and have obtained exciting results concerning the genome, transcriptome, and proteome (3-5).
microRNAs (miRNAs), as a significant part of transcriptome, serve critical roles in regulating the progression of multiple tumors, including HNSCC (5-7). It has been widely reported that, miRNAs could bind to the 3' untranslated region (3'UTR) of target mRNAs, and subsequently induce mRNA degradation or translation inhibition (8,9). Even, the same miRNA could target different mRNAs, thus participating in the regulation of diverse signaling pathways or molecular functions (10-13). The significance is different in tumor progression for each pathway or molecular function, considering that there are various pathways or molecular functions exerted by a specific miRNA, and all play complicated roles in the regulatory networks in tumor progression. However, perhaps a certain pathway or molecular function plays a pivotal role in this process. Furthermore, it is essential to screen out the critical mechanisms involved in tumor progression for specific miRNAs, which is more effective and profitable for targeted intervention treatments in certain tumors. Hence, it is still beneficial to explore more mechanisms of the reported miRNAs.
In the present study, miRNAs reported to be associated with HNSCC were retrieved and filtered from the National Center for Biotechnology Information (NCBI)/PubMed database. Further, enrolled miRNAs were classified into three grades according to the research content of the relevant reports. Target mRNAs for each miRNA were then predicted and screened. After that, the miRNAs-mRNAs regulatory networks were constructed and further analyzed for each grade. We aimed to provide a valuable reference for understanding the additional mechanisms of HNSCC that are-related to the miRNAs.
Methods
Ethics, consent, and permission
The present study was authorized by the Ethics Committees of the Affiliated Hospital of Hebei University (No. HDFY-LL-2020-008) and the First Hospital of Hebei Medical University (No. 2017012004). Written consent and permission from the patients were not needed.
Literature retrieval and filtering
Original research published in the preceding 5 years that was related to miRNAs in HNSCC was retrieved from the PubMed database. We searched the database using the terms “HNSCC” or “head and neck squamous cell carcinoma” or “Squamous Cell Carcinoma of the Head and Neck” or “Squamous Cell Carcinoma, Head And Neck” or “Carcinoma, Squamous Cell of Head and Neck” and “miRNA” or “microRNA” (retrieval date, December 5, 2019, back to January 1, 2014, considering feasibility and timeliness). The retrieved articles were filtered using the following criteria: (I) original research on HNSCC and published in English; and (II) research focused on the clinical relevance, molecular functions or mechanisms of specific miRNAs, not an expression profile analysis based on a microarray or high-throughput sequencing. Re-analysis articles based on published studies, including reviews, meta-analysis, and bioinformatics analysis based on public databases, were excluded.
Research content evaluation and miRNAs extraction
The enrolled studies were classified into three grades according to the research content and the evidence provided with experimental methods after a detailed analysis and evaluation. Mainly, the criteria included: (I) correlation analyses of miRNA expression levels and clinical features; (II) effects of miRNA on cellular functions; and (III) regulation of miRNA on its exact targeted mRNA and verified by luciferase assay. Grade A was of high confidence. The miRNAs contained in these articles were expounded in detail, including criteria I, II, and III. Grade B was of medium confidence. The miRNAs contained in these articles were elaborated on but were limited to criteria I and II, not III. Grade C was of low confidence. The miRNAs contained in these articles were analyzed based only on criteria I, not II, or III. Simultaneously, extracted miRNAs were also divided into A, B, and C grades following the grades of the source articles.
Target mRNAs prediction and screening
The target mRNAs of included miRNAs were predicted using the TargetScan (RRID: SCR_010845) (14), miRDB (RRID: SCR_010848) (15), and miRWalk (RRID: SCR_016509) (16) online databases. Afterward, the intersections of the target mRNAs in the three databases were calculated using Venn diagram (http://bioinformatics.psb.ugent.be/webtools/Venn/) for further analyses.
Co-expression networks construction and further analyses
Regulatory networks between miRNAs and target mRNAs (included in the intersection) were constructed through Cytoscape v3.6.0 based on grades (RRID: SCR_003032) (17). The Database for Annotation, Visualization, and Integrated Discovery (DAVID) v6.8 (RRID: SCR_001881) (18,19) and Gene Expression Profiling Interactive Analysis (GEPIA) (RRID: SCR_018294) (20) were adopted for use in the co-expression network analyses.
Results
A total of 201 eligible types of research were enrolled in the present study
According to the search terms, 447 articles were retrieved from the PubMed database; of them, 201 eligible articles were enrolled in subsequent analyses after evaluation and screening. The articles were divided into three grades considering the research content and the evidence provided by the experimental methods, as follows: A [124], B [47], C [30] (Figure 1).
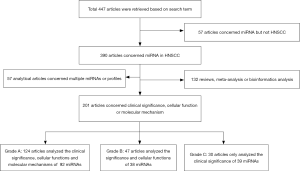
A total of 130 miRNAs were included in the subsequent analyses
All 169 miRNAs involved in these analyses were extracted and divided into three grades in line with the articles through elaborately analyzing the content of each included study (Figure 1). The miRNA was extracted and classified into the same grade as the study. For instance, a miRNA derived from a Grade A study, should be classified into a Grade A miRNA with high confidence, accordingly. However, the same miRNA may have been reported by several studies successively, and these studies were classified into different grades due to wide ranging degrees of credibility. Hence, the same miRNA might simultaneously be located at different grades with distinct confidences. Through a comparison of the different grades, some of the miRNAs that existed in different groups were the same and were only assigned to a high-level grade to provide more valuable references. Consequently, if miRNAs were classified into three grades concurrently, the miRNAs located in the medium or low grade were further excluded and only the miRNAs located in the high grade were retained. Finally, a total of 130 miRNAs were included in the present study and were distributed into grade A [92], B [18], and C [20], respectively.
Predicting target mRNAs and constructing co-expression networks
To acquire more reliable target mRNAs of miRNAs, three independent databases (TargetScan, miRDB, and miRWalk) were used to predict the primary target mRNAs. Next, the intersections of the three databases were selected as the final target of the mRNAs for further analysis. After, the final target mRNAs for each miRNA were sorted by the miRDB target score, and the top 10 mRNAs were selected for co-expression network construction. Co-expression networks based on the interaction of miRNAs-mRNAs were constructed using Cytoscape v3.6.0 software in three grades, respectively, to further explore the regulation of miRNAs to potential target mRNAs (Figures S1-S3).
Co-expression network analyses of Grade A
Gene ontology (GO) and Kyoto Encyclopedia of Genes and Genomes (KEGG) pathway enrichment analyses of target mRNAs included in the network were carried out through the DAVID v6.8 database to further explore the biological functions and possible mechanisms of the miRNAs and target mRNAs involved. Enrichment analyses suggested that miRNAs and target mRNAs were involved in complex and diverse biological functions and provided valuable information as a reference for further studies (Figure 2). For instance, SMAD family member 3 (SMAD3), could participate in multiple biological processes as a putative target of miR-145-p (transcription DNA-templates and the regulation of transcription from RNA polymerase II promoters) and molecular functions (protein binding, DNA binding, and RNA polymerase II core promoter proximal region sequence-specific DNA binding) in different cellular components such as the nucleus, cytoplasm, and nuclear chromatin. More importantly, the KEGG pathway enrichment analysis presented that enrolled miRNAs could take part in multiple pathways through target mRNAs. Among them, the PI3K-Akt, MAPK, and Wnt signaling pathways, which are key regulatory pathways of epithelial-mesenchymal transition (EMT), should be paid attention to. The scanning of the three signal pathways is shown in Table 1; certain mRNAs were located in different pathways simultaneously, including 13 mRNAs: activating transcription factor 2 (ATF2), cyclin D2 (CCND2), fibroblast growth factor 2 (FGF2), fibroblast growth factor 9 (FGF9), inhibitor of nuclear factor kappa B kinase subunit beta (IKBKB), mitogen-activated protein kinase 1 (MAP2K1), mitogen-activated protein kinase 3 (MAPK3), nuclear factor of activated T cells 1 (NFATC1), protein phosphatase 3 regulatory subunit B, alpha (PPP3R1), protein kinase cAMP-activated catalytic subunit beta (PRKACB), protein kinase C alpha (PRKCA), protein kinase C beta (PRKCB), and SOS Ras/Rac guanine nucleotide exchange factor 1 (SOS1). All of the 13 mRNAs were projected into the co-expression network (shown as a yellow node in Figure S1) to trace the associated miRNAs. A sub-network was extracted from the original co-expression network using Cytoscape (Figure 3). The IKBKB, located on the PI3K-Akt and MAPK signaling pathways, is regulated by miR-195-5p and miR-199b-5p simultaneously from the sub-network, while miR-195-5p could regulate IKBKB and FGF2. In the meantime, it also found on the PI3K-Akt and MAPK signaling pathways. These findings demonstrate the potential pivotal effects of miR-195-5p and miR-199b-5p on the PI3K-Akt and MAPK signaling pathways. The above analyses only reflected a part of the information contained in the co-expression network, and more valuable references could be established with additional, further analysis.
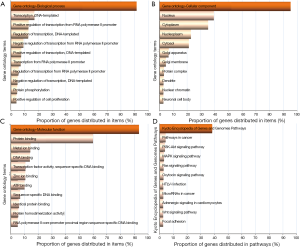
Table 1
KEGG pathway | Count | P value | Genes | Fold enrichment | FDR |
---|---|---|---|---|---|
hsa04151:PI3K-Akt signaling pathway | 32 | 2.57×10–4 | FGF9*, KITLG, RPS6KB1, FOXO3, PTEN, ATF2*, PDPK1, ITGB8, BCL2, SOS1*, CREB3L2, PRKAA2, FGF2*, PRKCA*, COL4A2, MAP2K1*, CREB1, CREB5, IL6R, HGF, BCL2L11, VEGFC, CDKN1A, EIF4E, TSC1, CCND2*, MAPK3*, GNB5, PPP2R5E, EFNA5, IKBKB*, PPP2R2A | 2.000164 | 0.329683 |
hsa04010:MAPK signaling pathway | 26 | 2.47×10–4 | FGF9*, MKNK2, PPP3R1*, DUSP10, CACNB2, CACNB4, ATF2*, ELK4, SOS1*, JUND, PRKACB*, RAPGEF2, FGF2*, NFATC1*, PRKCA*, CACNA2D1, MAP2K1*, TGFBR1, CACNA2D2, PRKCB*, MAP4K3, MAP4K4, DUSP3, RASGRF1, MAPK3*, IKBKB* | 2.216090 | 0.316678 |
hsa04310:Wnt signaling pathway | 21 | 4.57×10–6 | PRKCA*, DVL3, TBL1XR1, NKD1, VANGL1, ROCK2, CREBBP, PPP3R1*, LEF1, SKP1, DAAM2, PRKCB*, PRICKLE1, CCND2*, CAMK2D, LRP6, SIAH1, PRKACB*, NFATC2, FOSL1, NFATC1* | 3.281518 | 0.005860 |
* means the mRNA included in two or three pathways. KEGG, Kyoto Encyclopedia of Genes and Genomes; FDR, false discovery rate.
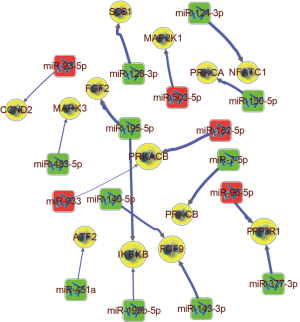
Co-expression network analyses of Grade B
GO and KEGG pathway analyses were used to explore the potential functions and mechanisms of Grade B miRNAs-target mRNAs using the same methods as Grade A. The enrichment analyses revealed that included miRNA exerted significant effects on multiple biological processes and molecular functions in different cellular components via putative targeting of mRNAs, including certain signaling pathways. Of them, the HIF-1 signaling pathway, which is a pivotal cancer-related signaling pathway (21,22), and the eight mRNAs included in it [mitogen-activated protein kinase 1 (MAPK1), von Hippel-Lindau tumor suppressor (VHL), RELA proto-oncogene, NF-kB subunit (RELA), calcium/calmodulin dependent protein kinase II delta (CAMK2D), phosphatidylinositol-4,5-bisphosphate 3-kinase catalytic subunit alpha (PIK3CA), insulin receptor (INSR), phosphoinositide-3-kinase regulatory subunit 3 (PIK3R3), and hexokinase 1 (HK1)], which may mediate the significant regulatory effects related to miRNAs and tumor progression through the pathway, were analyzed. The further analysis projected the eight mRNAs to the co-expression network of Grade B (yellow node in Figure S2) and traced the upstream miRNAs (red circle in Figure S2). The results showed that eight miRNAs may be involved in the regulation of the HIF-1 signaling pathway through the eight mRNAs mentioned above. The study further analyzed the putative target mRNAs with GEPIA to explore the significance of the miRNAs. Of them, seven mRNAs were up-regulated in HNSCC, while only HK1 was down-regulated (Figure 4A). More importantly, of the eight mRNAs, HK1 and PIK3R3 were associated with the overall survival rate of HNSCC patients (Figure 4B). The analyses above prompted the theory that HK1 and PIK3R3 may mediate the regulation of miR-150-3p and miR-365a-3p to the HIF-1 signaling pathway separately, which may further affect the development and overall survival rate of HNSCC.
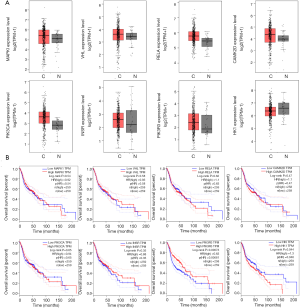
Co-expression network analyses of Grade C
The information contained in the network could be extracted and screened directly from it after the methods of analyzing the former co-expression networks through functional enrichment analyses. The co-expression network of Grade C showed that different miRNAs might have some synergistic or antagonistic effects on some biological functions through the common target mRNAs (yellow edges in Figure S3). Furthermore, to further analyze these findings, a sub-network was extracted (Figure 5A). Unexpectedly, the same mRNA was regulated by two miRNAs concurrently, and even the two miRNAs tended to present an opposite expression. For instance, PIK3R1 is a putative target of the up-regulated miR-5100. Meanwhile, it may still be regulated by a down-regulated miR-486-5p. It is well known that miRNA could result in the degradation or translation inhibition of a target mRNA. Hence, the mRNA should present the opposite expression tendency to miRNA. All of these factors show that using a database to make predictions has some limitations and the returned results need to be further filtered and verified. Given these results, further analyses were conducted based on the sub-network. The mRNAs expression levels in the HNSCC were first measured by GEPIA (Figure 5B) and then traced back to the relevant miRNAs. The study speculated that the down-regulated clock circadian regulator (CLOCK) might be regulated by up-regulated miR-34b-3p, not the down-regulated miR-15b-5p, considering those mentioned above. Consistently, thyroid hormone receptor beta (THRB) and PIK3R1 are the putative targets of miR-134b-5p and miR-5100 separately, not miR-138-5p and miR-486-5p.
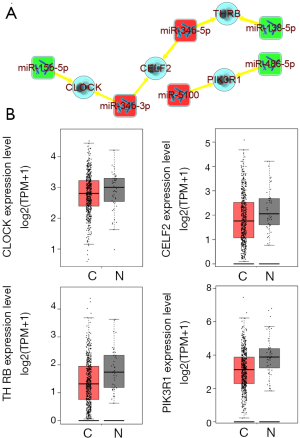
Discussion
The present study partly summarized the reported miRNAs to screen the ectopically expressed miRNAs and their related regulatory mechanisms in HNSCC to supply references for diagnosis and therapy. Through a series of analyses, the study showed that multiple miRNAs participated in the occurrence and development of HNSCC. Furthermore, the study also determined the potential regulatory roles of the PI3K-Akt, MAPK, Wnt signaling pathways, and other significant pathways or functions exerted by ectopic miRNAs. All of these contributed to the development of further research on miRNA in HNSCC.
It is regulated by multiple regulatory elements concurrently for a certain biomolecule or biological process. Furthermore, the regulatory effects of the different regulatory elements are diverse, such as the regulation of EMT (23-25). Meanwhile, the same regulatory elements may exert different regulatory roles in different biomolecules or biological processes, such as the regulatory roles of miR-129-5p, for instance (10-13). It is imperative and significant that the critical roles or biological processes of these regulatory elements are explored, especially for the reported functional molecules. These purposes also brought significance to the present study. miRNAs are involved in multifarious biological processes by targeting diverse mRNAs as pivotal functional molecules. For instance, miR-6089 could suppress the Wnt/β-catenin signaling pathway and EMT through targeting the myosin heavy chain 9 (MYH9) (26). Similarly, miR-129-5p could inhibit the progression of the malignant phenotype of multiple tumors by directly down-regulating different target mRNAs, including calcium/calmodulin dependent protein kinase IV (CAMK4), ZIC family member 2 (ZIC2), E2F transcription factor 7 (E2F7), and many others (10-13,27). After proving the complexity and diversity of miRNA biological functions, these studies also showed that the same miRNA could regulate multiple mRNAs and participate in different biological processes. These findings are consistent with our present analyses. miR-194-5p, miR-4295, miR-218-5p, and other included miRNAs all have multiple putative target mRNAs as they are present in co-expression networks. Further, the GO and KEGG pathway analyses suggested that these target mRNAs may be involved in different biological processes or molecular functions. Also, it is noteworthy that the co-expression networks also showed that different miRNAs target the same mRNA simultaneously; for instance, miR-224-5p, miR-375-3p, miR-186-5p, miR-34a-5p, and miR-199a-5p all target the zinc finger and BTB domain containing 20 (ZBTB20). The phenomenon suggests that these miRNAs may exert synergistic or antagonistic effects through the same mRNA, which has been reported in the earlier study. Relevant studies demonstrated that miR-16 could act as an adjuvant to enhance the inhibition of miR-302/367 cluster on EMT (28). Besides, miR-124 and miR-203 synergistically suppressed the EMT progress through down-regulating the common target zinc finger E-Box binding homeobox 2 (ZEB2) (29). The above analyses not only elucidated that there is a diversity of miRNA function mechanisms, but it also confirmed that there are interactions between miRNA and mRNA, as well as between miRNA and miRNA. Additionally, it further illustrated the significance of miRNAs as regulatory molecules.
All of the information mentioned above impelled this study to further explore more mechanisms of miRNAs by constructing co-expression networks. Based on the research contents of earlier studies, miRNAs included in the present study were grouped into three levels and separately were analyzed. Each level gives different references for later studies. The roles of Grade A miRNAs in HNSCC obtained the strongest evidence in the support-luciferase assay, which makes further research on these miRNAs more possible. However, it does not signify that there is no reference significance for other grades of miRNAs with a reduced amount of evidence.
On the contrary, more research should be applied to further explore the functions and mechanisms for Grade B and C miRNAs which have been confirmed to be associated with HNSCC. These miRNAs participate in the regulation of various biological processes, molecular functions, and signal pathways through acting on different target mRNAs precisely as presented by co-expression networks and functional enrichment analyses.
However, it is noteworthy that there are inherent limitations on the predictions and analyses based on these databases. The co-expression network shows that one mRNA simultaneously receives the regulations from two or more miRNAs, but these miRNA expression trends are opposite. Therefore, it is necessary to screen and validate the real upstream miRNA following the mRNA expression level and eliminate all of the false-positives. The analysis with the GEPIA database was performed simply to meet this need. The false-positives from database prediction are acceptable for the main purpose of supplying a feasible reference for subsequent study. The co-expression networks, based on some reported miRNAs, have a tremendous amount of valuable information.
Conclusions
Our study partly summarized the miRNAs altered in HNSCC and constructed miRNA-mRNA co-expression networks according to different evidence levels based on the information above. Further, the present study analyzed and extracted the information contained in these networks from different perspectives to provide a better reference for the subsequent research and to fully reflect the significance of these studies. The analyses also showed that multiple miRNAs participated in the occurrence and development of HNSCC through different molecular functions, biological processes, and signaling pathways. Exploring the mechanisms of miRNA in HNSCC is of great significance for understanding the pathogenesis and pursuing improved targeted therapy methods of HNSCC.
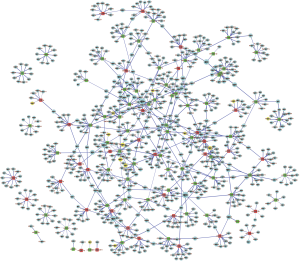
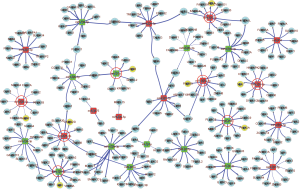
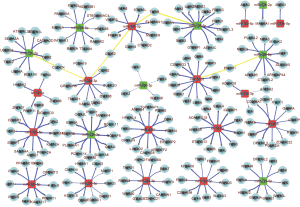
Acknowledgments
Funding: This study was funded by
Footnote
Conflicts of Interest: All authors have completed the ICMJE uniform disclosure form (available at http://dx.doi.org/10.21037/tcr-20-1145). The authors have no conflicts of interest to declare.
Ethical Statement: The authors are accountable for all aspects of the work in ensuring that questions related to the accuracy or integrity of any part of the work are appropriately investigated and resolved. The present study was authorized by the Ethics Committees of the Affiliated Hospital of Hebei University (No. HDFY-LL-2020-008) and the First Hospital of Hebei Medical University (No. 2017012004). The present study did not involve patients or specimens. No informed consent was need.
Open Access Statement: This is an Open Access article distributed in accordance with the Creative Commons Attribution-NonCommercial-NoDerivs 4.0 International License (CC BY-NC-ND 4.0), which permits the non-commercial replication and distribution of the article with the strict proviso that no changes or edits are made and the original work is properly cited (including links to both the formal publication through the relevant DOI and the license). See: https://creativecommons.org/licenses/by-nc-nd/4.0/.
References
- Ghanizada M, Jakobsen KK, Gronhoj C, et al. The effects of checkpoint inhibition on head and neck squamous cell carcinoma: a systematic review. Oral Oncol 2019;90:67-73. [Crossref] [PubMed]
- Leemans CR, Snijders PJF, Brakenhoff RH. The molecular landscape of head and neck cancer. Nat Rev Cancer 2018;18:269-82. [Crossref] [PubMed]
- Zhang W, Cheng J, Diao P, et al. Therapeutically targeting head and neck squamous cell carcinoma through synergistic inhibition of LSD1 and JMJD3 by TCP and GSK-J1. Br J Cancer 2020;122:528-38. [Crossref] [PubMed]
- Ma H, Chang H, Yang W, et al. A novel IFNalpha-induced long noncoding RNA negatively regulates immunosuppression by interrupting H3K27 acetylation in head and neck squamous cell carcinoma. Mol Cancer 2020;19:4. [Crossref] [PubMed]
- Zhuang Z, Yu P, Xie N, et al. MicroRNA-204-5p is a tumor suppressor and potential therapeutic target in head and neck squamous cell carcinoma. Theranostics 2020;10:1433-53. [Crossref] [PubMed]
- Hu M, Lu Y, Zeng H, et al. MicroRNA-21 maintains hematopoietic stem cell homeostasis through sustaining the NF-kappaB signaling pathway in mice. Haematologica 2020; [Epub ahead of print]. [Crossref] [PubMed]
- Tang Z, Xu T, Li Y, et al. Inhibition of CRY2 by STAT3/miRNA-7-5p promotes osteoblast differentiation through upregulation of CLOCK/BMAL1/P300 expression. Mol Ther Nucleic Acids 2020;19:865-76. [Crossref] [PubMed]
- Majumder M, Palanisamy V. RNA binding protein FXR1-miR301a-3p axis contributes to p21WAF1 degradation in oral cancer. PLoS Genet 2020;16:e1008580. [Crossref] [PubMed]
- Sereno M, Hasko J, Molnar K, et al. Downregulation of circulating miR 802-5p and miR 194-5p and upregulation of brain MEF2C along breast cancer brain metastasization. Mol Oncol 2020;14:520-38. [Crossref] [PubMed]
- Moradimotlagh A, Arefian E, Rezazadeh Valojerdi R, et al. MicroRNA-129 inhibits glioma cell growth by targeting CDK4, CDK6, and MDM2. Mol Ther Nucleic Acids 2020;19:759-64. [Crossref] [PubMed]
- Yu D, Han GH, Zhao X, et al. MicroRNA-129-5p suppresses nasopharyngeal carcinoma lymphangiogenesis and lymph node metastasis by targeting ZIC2. Cell Oncol (Dordr) 2020;43:249-61. [Crossref] [PubMed]
- Wu C, Miao C, Tang Q, et al. MiR-129-5p promotes docetaxel resistance in prostate cancer by down-regulating CAMK2N1 expression. J Cell Mol Med 2020;24:2098-108. [Crossref] [PubMed]
- Li Z, Lu J, Zeng G, et al. MiR-129-5p inhibits liver cancer growth by targeting calcium calmodulin-dependent protein kinase IV (CAMK4). Cell Death Dis 2019;10:789. [Crossref] [PubMed]
- Agarwal V, Bell GW, Nam JW, et al. Predicting effective microRNA target sites in mammalian mRNAs. ELife 2015;4:e05005. [Crossref] [PubMed]
- Chen Y, Wang X. miRDB: an online database for prediction of functional microRNA targets. Nucleic Acids Res 2020;48:D127-31. [Crossref] [PubMed]
- Sticht C, De La Torre C, Parveen A, et al. miRWalk: an online resource for prediction of microRNA binding sites. PLoS One 2018;13:e0206239. [Crossref] [PubMed]
- Shannon P, Markiel A, Ozier O, et al. Cytoscape: a software environment for integrated models of biomolecular interaction networks. Genome Res 2003;13:2498-504. [Crossref] [PubMed]
- Huang W, Sherman BT, Lempicki RA. Bioinformatics enrichment tools: paths toward the comprehensive functional analysis of large gene lists. Nucleic Acids Res 2009;37:1-13. [Crossref] [PubMed]
- Huang W, Sherman BT, Lempicki RA. Systematic and integrative analysis of large gene lists using DAVID bioinformatics resources. Nat Protoc 2009;4:44-57. [Crossref] [PubMed]
- Tang Z, Li C, Kang B, et al. GEPIA: a web server for cancer and normal gene expression profiling and interactive analyses. Nucleic Acids Res 2017;45:W98-W102. [Crossref] [PubMed]
- Wang LM, Zhang LL, Wang LW, et al. Influence of miR-199a on rats with non-small cell lung cancer via regulating the HIF-1alpha/VEGF signaling pathway. Eur Rev Med Pharmacol Sci 2019;23:10363-9. [PubMed]
- Kung-Chun Chiu D, Pui-Wah Tse A, Law CT, et al. Hypoxia regulates the mitochondrial activity of hepatocellular carcinoma cells through HIF/HEY1/PINK1 pathway. Cell Death Dis 2019;10:934. [Crossref] [PubMed]
- Wang X, Xu Z, Sun J, et al. Cisplatin resistance in gastric cancer cells is involved with GPR30-mediated epithelial-mesenchymal transition. J Cell Mol Med 2020;24:3625-33. [Crossref] [PubMed]
- Kong P, Xu E, Bi Y, et al. Novel ESCC-related gene ZNF750 as potential Prognostic biomarker and inhibits Epithelial-Mesenchymal Transition through directly depressing SNAI1 promoter in ESCC. Theranostics 2020;10:1798-813. [Crossref] [PubMed]
- Liu W, Jiang D, Gong F, et al. miR-210-5p promotes epithelial-mesenchymal transition by inhibiting PIK3R5 thereby activating oncogenic autophagy in osteosarcoma cells. Cell Death Dis 2020;11:93. [Crossref] [PubMed]
- Liu L, Ning Y, Yi J, et al. miR-6089/MYH9/beta-catenin/c-Jun negative feedback loop inhibits ovarian cancer carcinogenesis and progression. Biomed Pharmacother 2020;125:109865. [Crossref] [PubMed]
- Wan P, Bai X, Yang C, et al. miR-129-5p inhibits proliferation, migration, and invasion in rectal adenocarcinoma cells through targeting E2F7. J Cell Physiol 2020;235:5689-701. [Crossref] [PubMed]
- Hoseinbeyki M, Taha MF, Javeri A. miR-16 enhances miR-302/367-induced reprogramming and tumor suppression in breast cancer cells. IUBMB Life 2020;72:1075-86. [Crossref] [PubMed]
- Chen J, Zhong Y, Li L. miR-124 and miR-203 synergistically inactivate EMT pathway via coregulation of ZEB2 in clear cell renal cell carcinoma (ccRCC). J Transl Med 2020;18:69. [Crossref] [PubMed]