Identification of biomarkers in colon cancer based on bioinformatic analysis
Introduction
Globally, colon cancer is one of the most common cancers affecting the digestive tract and is the fourth most common cause of cancer-related deaths. Estimates in 2020 revealed 104,610 new colon cancer cases and 53,200 colorectal cancer-related deaths in the United States (1). Although some patients can initially respond to anticancer therapies, such as chemotherapy and targeted therapy, patients with advanced colon cancer eventually succumb to this disease (2). Thus, identifying the molecular mechanisms underlying colon cancer will facilitate the development of novel treatment strategies.
The diagnosis and treatment of colon cancer are aided by certain biomarkers, ranging from the early microsatellite instability (MSI) status to the recently discovered Ras family, B-Raf proto-oncogene, and serine/threonine kinase (BRAF) (3). Recent studies have markedly advanced the characterization of genetic changes in the malignant transformation process. Caudal-type homeobox 2 (CDX2) was identified as a prognostic biomarker of stage II and stage III colon cancer (4). Aldehyde dehydrogenase 1B1 (ALDH1B1) is a potential biomarker of human colon cancer (5). The epidermal growth factor receptor (EGFR) signal transduction pathway is one of the major pathways involved in the pathogenesis of colon cancer and is now widely targeted for the effective treatment of colon cancer. The initiation and progression of colon cancer are associated with the continuous activation of downstream Ras-RAF-MAPK and PI3K-AKT-mTOR pathways, which are mediated by EGFR (6). Thus, there is a need to identify novel biomarkers of colon cancer.
The objective of this study was to identify novel biomarkers of colon cancer through bioinformatics analysis of microarray data. We downloaded two mRNA microarray datasets for colon cancer from the Gene Expression Omnibus (GEO) database. Additionally, we obtained the most recent colon cancer dataset (COAD) in The Cancer Genome Atlas (TCGA) database. The differentially expressed genes (DEGs) between colon cancer and adjacent normal tissues were identified in these three datasets. Further, the DEGs were examined using Gene Ontology (GO) and Kyoto Encyclopedia of Genes and Genomes (KEGG) enrichment analyses and protein-protein interaction (PPI) network analysis. Finally, we investigated the key molecular events and functional pathways in colon cancer, which may provide the theoretical basis for the development of improved treatment strategies for colon cancer.
Methods
Data screening from the GEO database
The GEO database (http://www.ncbi.nlm.nih.gov/geo) (7) in the National Center for Biotechnology Information (NCBI) platform was utilized for screening the gene expression data, chip-based data, and microarray data. The inclusion criteria for the target data set are as follows: (I) the selected dataset included samples of both colon cancer tissue and adjacent normal tissue; (II) the dataset contains gene expression information; (III) study conducted over the last 10 years. Exclusion criteria: (I) study data from cell or animal experiments rather than from colon cancer patient tissues; (II) studies on colorectal cancer (one study includes both colon cancer and rectal cancer); (III) studies of rectal cancer or caecum cancer.
According to the above standards, we qualified the search strategy as “colon AND normal” in the GEO Datasets sub-database, selected “entry type” as “series” and limited “Publication dates” to after January 1, 2010. Then the retrieval results were screened one by one. Finally, we downloaded two gene expression datasets (GSE44076 and GSE47074) from the GEO database that satisfied the screening criteria. The probes were converted to the corresponding gene symbols based on the annotation information available on the platform. The GSE44076 dataset comprised 98 colon cancer and 98 adjacent normal tissues, while the GSE47074 dataset comprised 4 colon cancer and 4 adjacent normal tissues.
Data screening from the TCGA database
The mRNA array gene expression data from the colon cancer dataset (COAD) were downloaded from the TCGA database (https://tcga-data.nci.nih.gov/tcga/) using the R package Bioconductor/RTCGAToolbox (8). The TCGA COAD database (2016-01-28) contains 153 colon samples and 19 normal samples for analysis.
The mRNA expression profile data in GEO and TCGA databases are publicly available for downloading. So that this study does not require the ethics approval.
Identification of DEGs
The GSE44076 and GSE47074 datasets were used to identify the DEGs between colon cancer and adjacent normal tissues based on the interactive web tool GEO2R (http://www.ncbi.nlm.nih.gov/geo/http://www.ncbi.nih.gov/geo/geo2r/). The differences were considered statistically significant when |log fold change (FC)| was greater than 1 and P value was less than 0.01. In addition, the genes that were found to be dysregulated in the TCGA COAD datasets were loaded using the get DiffExpressedGene function package in R. The cut-off values were adjusted to obtain P value <0.05 and log FC >2. The analysis identified 889 upregulated genes and 550 downregulated genes in the colon cancer tissues. Finally, the common DEGs among the three datasets were selected for further analysis.
KEGG and GO enrichment analyses of DEGs
The KEGG (9) database contains information on genomes, biological pathways, diseases, and chemical compounds. GO (10) enrichment analysis is mainly used for annotating the genes and gene products. GO terms are grouped into three categories: biological processes (BP), cellular composition (CC), and molecular function (MF). We used the Database for Annotation, Visualization, and Integrated Discovery (DAVID; http://david.ncifcrf.gov) (version 6.8) (11) to analyze the KEGG pathways and GO terms associated with the identified DEGs. The difference was considered statistically significant when the adjusted P value was less than 0.05. The results were visualized using the ggplot2 package in R to generate the bubble map of the KEGG pathway analysis and the histogram of the GO enrichment analysis.
PPI network construction and visualization
The functional interactions between DEGs were evaluated using the protein interaction database (STRING) (12). The interactions with a combined score of greater than 0.4 were considered statistically significant. Cytoscape (version 3.7.0) was used to visualize the PPI network (13) based on the STRING results, and MCODE was used to identify the densely connected regions (14). The following parameters were used in the analysis: MCODE scores >5, degree cut-off =2, node score cut-off =0.2, max depth =100, and k-score =2. Additionally, KEGG and GO enrichment analyses were performed on the selected meaningful models. The difference was considered statistically significant when the P value was less than 0.05.
Selection and analysis of hub genes
The genes with degree ≥10 were defined as hub genes (15). We used cBioportal (http://www.cbioportal.org) (16,17) to analyze the genes that were co-expressed with the hub genes. Next, hierarchical clustering of the hub genes was performed using the UCSC Cancer Genomics Browser (http://genome-cancer.ucsc.edu) (18). Using the starBase (http://starbase.sysu.edu.cn/) (19) Pan-Cancer Analysis platform, the prognostic values of the hub genes were analyzed to identify the genes whose survival curves were statistically significant and those that exhibit potential as biomarkers of colon cancer. Finally, the Oncomine database (http://www.oncomine.com) was used to verify the correlation between expression patterns and tumor grades, tumor histological type and other characteristics.
Validation by quantitative real-time polymerase chain reaction real-time fluorescence quantitative PCR (qRT-PCR)
We evaluated the expression levels of hub genes using 3 groups of the colon cancer cells (HCT-8, SW620, and SW480) and a normal colon cell (HT-29). The colon cancer cells and normal colon cell were obtained from Cell Bank of the Typical Culture Preservation Committee of the Chinese Academy of Sciences (Shanghai, China). Total RNA was extracted from the samples using the Trizol reagent (Invitrogen, Carlsbad, CA, USA), following the manufacturer’s instructions. The reverse transcription of mRNA was performed using the FastQuant RT Kit (TaKaRa, Beijing China), following the manufacturer’s instructions. qRT-PCR was performed using the ABI 7500 Real-time PCR Detection System. Relative gene expression was analyzed using 2−ΔCt and 2−ΔCtΔCt method.
Results
Identification of DEGs
Differential expression analysis identified 2,677 and 517 DEGs in the GSE44076 and GSE44074 datasets respectively, as well as 1,439 DEGs in TCGA datasets. We obtained 150 genes that were common among the three datasets, which are presented as a Venn diagram (Figure 1).
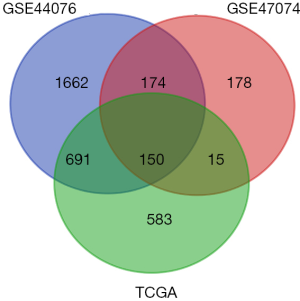
GO term and KEGG pathway enrichment
The top ten terms with P<0.05 in the BP and CC categories were selected for visualization. All terms with P<0.05 in the MF category are shown in Figure 2. The BP terms were predominantly related to cell division, DNA damage repair, and positive regulation of RNA transcription. The MF terms were mainly related to ATP binding. The CC terms were predominantly associated with the cytoplasm (Figure 2). KEGG pathway analysis revealed that the DEGs were mainly related to the cell cycle, microRNAs in cancer, and other signaling pathways, such as the p53 signaling pathway (Figure 3).
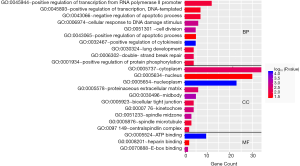
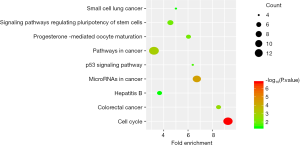
PPI network
In total, 148 proteins were identified from the STRING database based on the DEGs, which involved 807 interaction pairs. The average node degree was 10.9. Next, the PPI network was constructed using Cytoscape (Figure 4A). The most important module in the network, which comprised 34 nodes, was extracted using MCODE (Figure 4B). Functional analysis of the genes involved in this module revealed that these genes were predominantly enriched in cell division, ATP binding, and cell cycle-associated functions and pathways (Table 1).

Table 1
Term | Description | Count | P value |
---|---|---|---|
BP | |||
GO:0051301 | Cell division | 11 | 1.86E-13 |
GO:0032467 | Positive regulation of cytokinesis | 4 | 1.88E-05 |
GO:0007080 | Mitotic metaphase plate congression | 4 | 3.22E-05 |
GO:0031536 | Positive regulation of exit from mitosis | 3 | 3.94E-05 |
GO:0000281 | Mitotic cytokinesis | 3 | 0.0011 |
GO:0000086 | G2/M transition of mitotic cell cycle | 3 | 0.0013 |
GO:0006302 | Double-strand break repair | 3 | 0.0036 |
GO:0007018 | Microtubule-based movement | 3 | 0.0055 |
GO:0046602 | Regulation of mitotic centrosome separation | 2 | 0.0081 |
CC | |||
GO:0005634 | Nucleus | 15 | 2.39E-04 |
GO:0030496 | Midbody | 5 | 2.83E-05 |
GO:0000776 | Kinetochore | 4 | 2.30E-04 |
GO:0051233 | Spindle midzone | 3 | 5.65E-04 |
GO:0005876 | Spindle microtubule | 3 | 0.0023 |
GO:0005871 | Kinesin complex | 3 | 0.0049 |
GO:0097149 | Central spindlin complex | 2 | 0.0067 |
MF | |||
GO:0005524 | ATP binding | 12 | 5.90E-06 |
GO:0019901 | Protein kinase binding | 5 | 1.48E-05 |
GO:0004842 | Ubiquitin-protein transferase activity | 4 | 0.0053 |
KEGG | |||
04110 | Cell cycle | 8 | 2.91E-09 |
04914 | Progesterone-mediated oocyte maturation | 4 | 7.68E-04 |
04114 | Oocyte meiosis | 4 | 0.0016 |
04115 | p53 signaling pathway | 3 | 0.0096 |
BP, biological processes; CC, cellular composition; MF, molecular function; KEGG, pathways in Kyoto Encyclopedia of Genes and Genomes (KEGG) database.
Hub genes
In the most important module, the degree value of the 34 genes was ≥25, which indicated close interaction between the nodes. Thus, the 34 genes were considered as the hub genes for preliminary analysis. A co-expression network of the hub genes was constructed using the cBioPortal online platform (Figure 4C). The co-expression network revealed the connection between the hub genes and some relevant genes in colon cancer. The results of hierarchical clustering of the hub genes using UCSC are shown in Figure 5. The hub genes were weakly expressed in the normal tissues and strongly expressed in the primary colon cancer tissues. However, we did not observe significant correlation between the expression levels of the hub genes and gender. Moreover, for the MSI entry, although most samples did not provide MSI detection data, it was observed that more samples with MSI appeared in samples with strong expression of hub genes.
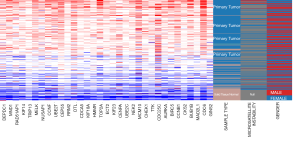
Next, the prognostic values of these hub genes were analyzed using the starBase Pan-Cancer Analysis Platform. As shown in Figure 6, the survival curves of eight genes were statistically significant (P value <0.05). These eight genes were CCNB1, CHEK1, DEPDC1, ECT2, GINS2, HMMR, KIF14, and KIF18A. However, the generated predictions using starBase suggested that the weak expression of these eight genes was associated with poor prognosis.
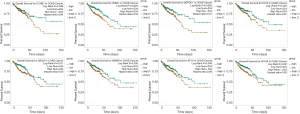
Finally, validation analysis using the Oncomine database revealed that whether in a single study or in the meta-analysis results of multiple studies, the expression levels of the eight genes were upregulated in the cancer samples when compared to those in the non-cancer samples (Figures 7,8). In Oncomine database, data from Kaiser et al. reported that the expression of GINS2 was strongly upregulated in the colon small cell carcinoma and colon signet ring cell adenocarcinoma when compared to that in other types of colon cancers, whereas the other seven genes were strongly upregulated in the colon small cell carcinoma (Figure 9). Furthermore, the expression levels of these eight genes were higher in patients with grade 1 cancer than those in patients with grades 2-3 cancer (Figure 10).
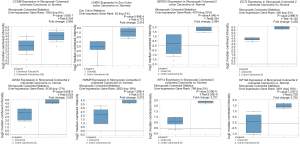
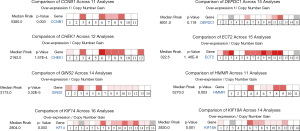
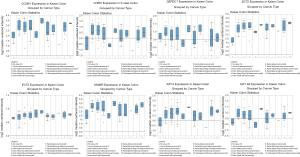
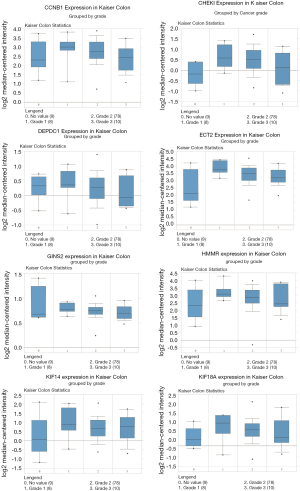
Finally, we selected five specific genes (DEPDC1, ECT2, GINS2, HMMR, KIF18A) to verify the analysis. qRT-PCR was used to detect the expression differences between colon cancer cells (HCT-8, SW620, SW480) and normal colon cells (HT-29) in each group. The results of qRT-PCR showed that the expression levels of DEPDC1, ECT2, GINS2, HMMR, and KIF18A were increased in colon cancer cells compared with normal colon tissues (Figure 11 and Table 2), which was consistent with the analysis results of GEO and TCGA data sets, as well as the Oncomine and UCSC database validation results.
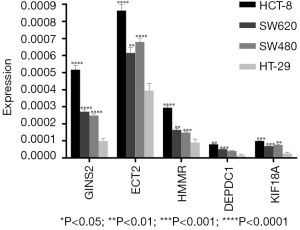
Table 2
Gene | Group | 2–△CT (mean ± SD) | △CT | 2–△△CT | P value |
---|---|---|---|---|---|
GINS2 | HCT-8 | 0.000539±1.51E-05 | 10.918 | 5.220 | <0.0001 |
SW620 | 0.000271±6.984E-06 | 11.849 | 2.737 | <0.0001 | |
SW480 | 0.000248±6.173E-06 | 11.977 | 2.505 | <0.0001 | |
HT-29 | 0.000099±1.152E-05 | 13.302 | |||
ECT2 | HCT-8 | 0.000863±2.076E-05 | 10.178 | 2.185 | <0.0001 |
SW620 | 0.000615±1.885E-05 | 10.667 | 1.558 | 0.0019 | |
SW480 | 0.0006798±1.359E-05 | 10.522 | 1.722 | <0.0001 | |
HT-29 | 0.000394±3.391E-05 | 11.310 | |||
HMMR | HCT-8 | 0.000294±6.119E-06 | 11.732 | 3.292 | <0.0001 |
SW620 | 0.000164±4.807E-06 | 12.574 | 1.837 | 0.0041 | |
SW480 | 0.000150±4.359E-06 | 12.712 | 1.669 | 0.0005 | |
HT-29 | 8.93E-05±1.658E-05 | 13.451 | |||
DEPDC1 | HCT-8 | 7.979E-05±1.924E-06 | 13.613 | 5.582 | 0.0012 |
SW620 | 5.116E-05±1.269E-06 | 14.253 | 3.581 | 0.0004 | |
SW480 | 4.109E-05±1.079E-06 | 14.571 | 2.875 | 0.0805 | |
HT-29 | 1.43E-05±4.302E-06 | 16.094 | |||
KIF18A | HCT-8 | 9.902E-05±1.768E-06 | 13.302 | 3.823 | 0.0004 |
SW620 | 6.97E-05±1.539E-06 | 13.808 | 2.692 | 0.0008 | |
SW480 | 7.628E-05±2.16E-06 | 13.678 | 2.947 | 0.0024 | |
HT-29 | 2.59E-05±6.362E-06 | 15.237 |
Discussion
Recent studies have demonstrated the importance of targeting key molecules in a network for the diagnosis and treatment of colon cancer. Therefore, there is an urgent need to identify novel biomarkers of colon cancer. Bioinformatics analysis of gene expression profiles is widely employed to identify DEGs as biomarkers for the occurrence and progression of cancer. This has facilitated the development of effective diagnostic and therapeutic strategies.
In this study, we analyzed two mRNA microarray datasets from the GEO database and one mRNA microarray dataset from the TCGA database. Our analysis identified 150 DEGs between colon cancer and adjacent normal tissues. GO enrichment analysis revealed that these DEGs were enriched in functions related to accelerating the cell cycle, promoting tumor cell accumulation, promoting cell division, positively regulating cell division, and negatively regulating apoptosis. Enhanced cell division and inhibition of apoptosis of cancer cells are generally recognized as important markers of colon cancer (20). KEGG pathway analysis revealed that the DEGs were also involved in the cell cycle pathway, which concurred with the results of GO enrichment analysis. Furthermore, we identified 34 hub genes in the PPI network, which were enriched in the cell cycle pathway. These findings indicated the critical role of the cell cycle in colon cancer progression.
As shown in Figure 5, the expression of hub genes is not affected by gender factors. However, the expression levels of the hub genes were correlated with the MSI status, which is one of the important mechanisms for the formation of colon cancer. MSI induces carcinogenesis by activating the oncogenes or by inactivating the tumour suppressor genes (21). Our results indicate that high expression of hub genes is associated with MSI status, suggesting that hub genes may play a synergistic role with MSI in the development of colon cancer. Studies have shown that colorectal cancer patients with MSI status benefit less from chemotherapy and are more likely to acquire 5-FU resistance (22). But for immunotherapy, colon cancer patients with MSI-H have a higher response to immune checkpoint inhibitors (23). In our study, more samples with MSI appeared in samples with strong expression of hub genes, suggesting that these hub genes may guide the treatment of colon cancer in adjuvant chemotherapy and immunotherapy.
Prognostic analysis of the 34 hub genes revealed that the low expression levels of the eight genes (CCNB1, CHEK1, DEPDC1, ECT2, GINS2, HMMR, KIF14, and KIF18A) were significantly correlated with the poor prognosis of colon cancer (P value <0.05). The role of these eight genes in colon cancer was validated using relevant studies from the Oncomine database. The results of the Skrzypczak’s and Zou’s experiments included in the database showed that these genes were up-regulated in colon cancer tissue relative to normal colon tissue, respectively. In addition, a meta-analysis of all colon cancer related studies in Oncomine database was performed online to demonstrate the result again. For the tissue type of colon cancer, the eight genes were strongly expressed in small cell carcinoma of colon cancer. Small cell carcinoma of the gastrointestinal tract is rare (24) and shares many characteristics with squamous cell carcinoma of the lungs, including neuroendocrine features, regional lymph node involvement, high metastatic potential, and poor prognosis (25). However, because of the limited number of studies in the Oncomine database that involve these 8 genes and colon cancer tissue type, the sample size of various tissue types involved is relatively small only through the analysis of Kaiser’s study. So more large-sample studies are still needed to support this conclusion. Furthermore, we observed that in the study of tumor pathological grading, the expression levels of these 8 genes in Grade 1 (well differentiated) patients were higher than those in Grade 2 (moderately differentiated) and Grade 3 (poorly differentiated) cancer patients. This suggested that the upregulation of the eight hub genes was not associated with the malignancy of colon cancer. Hence, these eight genes might be potential biomarkers of early colon cancer.
Among the eight genes, checkpoint kinase 1 (CHEK1) and DEP domain containing 1 (DEPDC1) exhibited the most statistically significant survival curves (P<0.01). CHEK1 is strongly expressed in many tumors, such as colon cancer (26), breast cancer (27), and liver cancer (28). CHEK1, a checkpoint kinase of the G2/M phase during cell division, helps repair DNA damage in cells and plays a crucial role in the regulation of tumorigenesis. In colon cancer, CHEK1 not only promotes the cell proliferation, but also attenuates the DNA damage of tumor cells induced by radiotherapy and chemotherapy (29). Hence, treatment with CHEK1 inhibitors blocks the colon cancer cell cycle at the G2 phase and enhances the sensitivity of tumor cells to various chemotherapy drugs by inhibiting the DNA damage repair (30). CHEK1 inhibitors are currently being tested as novel anti-tumor drugs for several types of tumors, including colon cancer (30). In recent years, DEPDC1 was reported as a novel cell cycle-related gene that regulates mitotic progression in several human cancers, such as bladder cancer (31), nasopharyngeal cancer (32), gastric cancer (33), glioblastoma (34), and liver cancer (35). Additionally, several studies suggest that DEPDC1 promotes cell proliferation and inhibits apoptosis of cancer cells by binding to the zinc finger protein 224 and activating the NF-κB signaling pathway (36). Therefore, the expression of DEPDC1 may be associated with the rapid proliferation of colon cancer cells.
To validate our bioinformatics findings, we selected DEPDC1, ECT2, GINS2, HMMR, and KIF18A gene for qRT-PCR analysis. Our analysis revealed that the expression levels of all five genes in the colon cancer cells were upregulated when compared to those in the normal colon cell. The results obtained from qRT-PCR analysis were concurred with those obtained from the Oncomine and UCSC databases validation results.
Epithelial cell transforming sequence 2 (ECT2) is highly evolutionarily conserved and is an oncogene known to be closely associated with cell proliferation and apoptosis. Additionally, ECT2 can induce malignant transformation of epithelial cells (37). ECT2 is a Rho guanine nucleotide exchange factor (RhoGEF) that can promote the dissociation of GDP and the replacement of GDP with GTP, which activates the Ras analogue Rho GTPase in the cell signal transduction pathway. Therefore, ECT2 can affect the cytoskeletal dynamics, cell morphogenesis, and regulation of cell movement in colon cancer. Additionally, ECT2 can affect malignant transformation, growth, invasion, and metastasis of tumor cells (38). Our protein co-expression data suggest that ECT2 acts on RHOBTB2, which was identified recently as a member of the Rho family, and inhibits tumor cell proliferation (39). ECT2 may promote the proliferation of colon cancer by inhibiting the expression of RHOBTB2. A recent study analyzed the RNA samples extracted from tumor cells in the peripheral blood circulation of 90 patients with colorectal cancer and 151 healthy donors. The analysis revealed that ECT2 had a high detection rate, even in patients with carcinoembryonic antigen level lower than 5 ng/mL. This indicated that ECT2 is a potential diagnostic marker of colorectal cancer and exhibits high sensitivity for the detection of circulating tumor cells in blood compared to that for the detection of carcinoembryonic antigen (40).
GINS complex subunit 2 (GINS2) is a helicase that plays an important role in various BP, such as DNA replication, cross-chain repair, and maintenance of chromosome condensation (41). GINS2 promotes the growth of breast cancer cells by regulating the cell cycle progression to G2/M phase (42). In ovarian cancer, GINS2 knockdown resulted in the induction of cell cycle arrest at the S phase and not at the G2 phase (43). Based on our bioinformatics analysis, we hypothesized that the enhanced expression of GINS2 in colon cancer is also involved in cell cycle regulation. However, this hypothesis must be experimentally verified. Previous studies have demonstrated that GINS2 expression levels are downregulated after exposure to 5-fluorouracil (5-FU) in a colorectal adenocarcinoma cell line. This suggested that GINS2 can be a novel potential biomarker of 5-FU chemosensitivity/resistance for human colon cancer as they inhibit cell proliferation (44).
Hyaluronan-mediated motility receptor (HMMR) is located on chromosome 5. The HMMR gene is downstream of IC-1 and is overexpressed in various cancers, such as breast cancer (45), lung adenocarcinoma (46), and epithelial ovarian cancer (47). HMMR affects the tumor microenvironment in the tumor budding cells and promotes single-cell invasion and metastatic dissemination of colon cancer. The enhanced expression levels of HMMR is correlated with the high tumor grade, lymphatic infiltration, and lymph node metastasis in colorectal cancer. This suggested that HMMR expression may drive the progression of cancer in the early phase. Therefore, targeting the HMMR receptor could be a novel therapeutic strategy for colorectal cancer (48).
Kinesin family member 18A (KIF18A) is a member of the kinesin superfamily proteins, which belong to the microtubules depolymerase Kip3 family. During mitosis, KIF18A is directly involved in mid plate assembly and separation of sister chromatids (49). Dysregulated protein expression of KIF18A leads to abnormal separation of sister chromatids during mitosis resulting in aneuploidy, which promotes tumorigenesis. KIF18A is highly expressed in breast cancer and is associated with poor prognosis (50). In colon cancer, the enhanced expression of KIF18A induces proliferation and inhibits apoptosis of colon cancer cells. Additionally, KIF18A induces Akt phosphorylation, mediates PI3K-Akt signaling pathway, and promotes colon cancer progression (51).The co-expression network analysis using the cBioPortal database revealed that KIF18A was associated with the expression of several genes, including BIRC5, DSN1, CENPF, CENPA, CDCA5 and BUB1B. Additionally, the analysis revealed that CCNB1 can act on KIF18A.These genes were associated with proliferation and apoptosis of colon cancer.
Conclusions
In conclusion, DEPDC1, ECT2, GINS2, HMMR, and KIF18A were upregulated in colon cancer, and promoted the progression of colon cancer by regulating the cell cycle, which was consistent with our GO enrichment and KEGG pathway analyses. The results of our bioinformatics analysis and those of other studies indicate that DEPDC1, ECT2, GINS2, HMMR, and KIF18A may serve as biomarkers for the early diagnosis and progression of colon cancer. However, further studies are needed to elucidate the specific mechanism of action of these genes in colon cancer.
Acknowledgments
The authors acknowledge the assistance of Professor Hui Chai from the University of Zhejiang Chinese Medical University and Kaibo Guo, Guan Feng from First Clinical College of Zhejiang Chinese Medical University in the review and preparation of this manuscript.
Funding: This study was supported by grants from
Footnote
Peer Review File: Available at http://dx.doi.org/10.21037/tcr-20-845
Conflicts of Interest: All authors have completed the ICMJE uniform disclosure form (available at http://dx.doi.org/10.21037/tcr-20-845). The authors have no conflicts of interest to declare.
Ethical Statement: The authors are accountable for all aspects of the work in ensuring that questions related to the accuracy or integrity of any part of the work are appropriately investigated and resolved. The mRNA expression profile data in GEO and TCGA databases are publicly available for downloading. So that this study does not require the ethics approval.
Open Access Statement: This is an Open Access article distributed in accordance with the Creative Commons Attribution-NonCommercial-NoDerivs 4.0 International License (CC BY-NC-ND 4.0), which permits the non-commercial replication and distribution of the article with the strict proviso that no changes or edits are made and the original work is properly cited (including links to both the formal publication through the relevant DOI and the license). See: https://creativecommons.org/licenses/by-nc-nd/4.0/.
References
- Siegel RL, Miller KD, Goding Sauer A, et al. Colorectal cancer statistics, 2020. CA Cancer J Clin 2020;70:145-64. [Crossref] [PubMed]
- Xie J, Gu D, Song R. A novel fusion gene responsible for colon cancer drug resistance. AACR; 2018.
- Taieb J, Kourie HR, Emile JF, et al. Association of Prognostic Value of Primary Tumor Location in Stage III Colon Cancer With RAS and BRAF Mutational Status. JAMA Oncol 2018;4:e173695. [Crossref] [PubMed]
- Dalerba P, Sahoo D, Paik S, et al. CDX2 as a prognostic biomarker in stage II and stage III colon cancer. N Engl J Med 2016;374:211-22. [Crossref] [PubMed]
- Chen Y, Orlicky DJ, Matsumoto A, et al. Aldehyde dehydrogenase 1B1 (ALDH1B1) is a potential biomarker for human colon cancer. Biochem Biophys Res Commun 2011;405:173-9. [Crossref] [PubMed]
- Palomba G, Doneddu V, Cossu A, et al. Prognostic impact of KRAS, NRAS, BRAF, and PIK3CA mutations in primary colorectal carcinomas: a population-based study. J Transl Med 2016;14:292. [Crossref] [PubMed]
- Edgar R, Domrachev M, Lash AE. Gene Expression Omnibus: NCBI gene expression and hybridization array data repository. Nucleic Acids Res 2002;30:207-10. [Crossref] [PubMed]
- Samur MK. RTCGAToolbox: a new tool for exporting TCGA Firehose data. PLoS One 2014;9:e106397. [Crossref] [PubMed]
- Kanehisa M. The KEGG database. Novartis Found Symp 2002;247:91-101; discussion -3, 19-28, 244-52.
- Ashburner M, Ball CA, Blake JA, et al. Gene ontology: tool for the unification of biology. The Gene Ontology Consortium. Nat Genet 2000;25:25-9. [Crossref] [PubMed]
- Huang DW, Sherman BT, Tan Q, et al. The DAVID Gene Functional Classification Tool: a novel biological module-centric algorithm to functionally analyze large gene lists. Genome Biol 2007;8:R183. [Crossref] [PubMed]
- Szklarczyk D, Franceschini A, Wyder S, et al. STRING v10: protein-protein interaction networks, integrated over the tree of life. Nucleic Acids Res 2015;43:D447-52. [Crossref] [PubMed]
- Shannon P, Markiel A, Ozier O, et al. Cytoscape: a software environment for integrated models of biomolecular interaction networks. Genome Res 2003;13:2498-504. [Crossref] [PubMed]
- Bandettini WP, Kellman P, Mancini C, et al. MultiContrast Delayed Enhancement (MCODE) improves detection of subendocardial myocardial infarction by late gadolinium enhancement cardiovascular magnetic resonance: a clinical validation study. J Cardiovasc Magn Reson 2012;14:83. [Crossref] [PubMed]
- Li L, Lei Q, Zhang S, et al. Screening and identification of key biomarkers in hepatocellular carcinoma: Evidence from bioinformatic analysis. Oncol Rep 2017;38:2607-18. [Crossref] [PubMed]
- Cerami E, Gao J, Dogrusoz U, et al. The cBio cancer genomics portal: an open platform for exploring multidimensional cancer genomics data. Cancer Discov 2012;2:401-4. [Crossref] [PubMed]
- Gao J, Aksoy BA, Dogrusoz U, et al. Integrative analysis of complex cancer genomics and clinical profiles using the cBioPortal. Sci Signal 2013;6:pl1. [Crossref] [PubMed]
- Casper J, Zweig AS, Villarreal C, et al. The UCSC Genome Browser database: 2018 update. Nucleic Acids Res 2018;46:D762-9. [Crossref] [PubMed]
- Li JH, Liu S, Zhou H, et al. starBase v2.0: decoding miRNA-ceRNA, miRNA-ncRNA and protein-RNA interaction networks from large-scale CLIP-Seq data. Nucleic Acids Res 2014;42:D92-7. [Crossref] [PubMed]
- Hanahan D, Weinberg RA. Hallmarks of cancer: the next generation. Cell 2011;144:646-74. [Crossref] [PubMed]
- Koenig JL, Toesca DAS, Harris JP, et al. Microsatellite Instability and Adjuvant Chemotherapy in Stage II Colon Cancer. Am J Clin Oncol 2019;42:573-80. [Crossref] [PubMed]
- Leicher LW, Lammertink MHA, Offerman SR, et al. Consequences of testing for mismatch repair deficiency of colorectal cancer in clinical practice. Scand J Gastroenterol 2018;53:632-6. [Crossref] [PubMed]
- Baretti M, Le DT. DNA mismatch repair in cancer. Pharmacol Ther 2018;189:45-62. [Crossref] [PubMed]
- Saif MW. Small cell carcinoma of the colon arising in a carcinoid tumor. Anticancer Res 2013;33:1713-5. [PubMed]
- Balasubramanyam S, O'Donnell BP, Musher BL, et al. Evaluating Treatment Patterns for Small Cell Carcinoma of the Colon Using the National Cancer Database (NCDB). J Gastrointest Cancer 2019;50:244-53. [Crossref] [PubMed]
- Stawinska M, Cygankiewicz A, Trzcinski R, et al. Alterations of Chk1 and Chk2 expression in colon cancer. Int J Colorectal Dis 2008;23:1243-9. [Crossref] [PubMed]
- Kim HJ, Min A, Im SA, et al. Anti-tumor activity of the ATR inhibitor AZD6738 in HER2 positive breast cancer cells. Int J Cancer 2017;140:109-19. [Crossref] [PubMed]
- Xie Y, Wei RR, Huang GL, et al. Checkpoint kinase 1 is negatively regulated by miR-497 in hepatocellular carcinoma. Med Oncol 2014;31:844. [Crossref] [PubMed]
- Zhang Y, Hunter T. Roles of Chk1 in cell biology and cancer therapy. Int J Cancer 2014;134:1013-23. [Crossref] [PubMed]
- Herudkova J, Paruch K, Khirsariya P, et al. Chk1 Inhibitor SCH900776 Effectively Potentiates the Cytotoxic Effects of Platinum-Based Chemotherapeutic Drugs in Human Colon Cancer Cells. Neoplasia 2017;19:830-41. [Crossref] [PubMed]
- Obara W, Ohsawa R, Kanehira M, et al. Cancer peptide vaccine therapy developed from oncoantigens identified through genome-wide expression profile analysis for bladder cancer. Jpn J Clin Oncol 2012;42:591-600. [Crossref] [PubMed]
- Feng X, Zhang C, Zhu L, et al. DEPDC1 is required for cell cycle progression and motility in nasopharyngeal carcinoma. Oncotarget 2017;8:63605-19. [Crossref] [PubMed]
- Fujiwara Y, Okada K, Omori T, et al. Multiple therapeutic peptide vaccines for patients with advanced gastric cancer. Int J Oncol 2017;50:1655-62. [Crossref] [PubMed]
- Kikuchi R, Sampetrean O, Saya H, et al. Functional analysis of the DEPDC1 oncoantigen in malignant glioma and brain tumor initiating cells. J Neurooncol 2017;133:297-307. [Crossref] [PubMed]
- Yuan SG, Liao WJ, Yang JJ, et al. DEP domain containing 1 is a novel diagnostic marker and prognostic predictor for hepatocellular carcinoma. Asian Pac J Cancer Prev 2014;15:10917-22. [Crossref] [PubMed]
- Li A, Wang Q, He G, et al. DEP domain containing 1 suppresses apoptosis via inhibition of A20 expression, which activates the nuclear factor kappaB signaling pathway in HepG2 cells. Oncol Lett 2018;16:949-55. [PubMed]
- Fields AP, Justilien V. The guanine nucleotide exchange factor (GEF) Ect2 is an oncogene in human cancer. Adv Enzyme Regul 2010;50:190-200. [Crossref] [PubMed]
- Aspenstrom P. Activated Rho GTPases in Cancer-The Beginning of a New Paradigm. Int J Mol Sci 2018;19:3949. [Crossref] [PubMed]
- Choi YM, Kim KB, Lee JH, et al. DBC2/RhoBTB2 functions as a tumor suppressor protein via Musashi-2 ubiquitination in breast cancer. Oncogene 2017;36:2802-12. [Crossref] [PubMed]
- Chen CJ, Sung WW, Chen HC, et al. Early Assessment of Colorectal Cancer by Quantifying Circulating Tumor Cells in Peripheral Blood: ECT2 in Diagnosis of Colorectal Cancer. Int J Mol Sci 2017;18:743. [Crossref] [PubMed]
- Ouyang F, Liu J, Xia M, et al. GINS2 is a novel prognostic biomarker and promotes tumor progression in early-stage cervical cancer. Oncol Rep 2017;37:2652-62. [Crossref] [PubMed]
- Peng L, Song Z, Chen D, et al. GINS2 regulates matrix metallopeptidase 9 expression and cancer stem cell property in human triple negative Breast cancer. Biomed Pharmacother 2016;84:1568-74. [Crossref] [PubMed]
- Yan T, Liang W, Jiang E, et al. GINS2 regulates cell proliferation and apoptosis in human epithelial ovarian cancer. Oncol Lett 2018;16:2591-8. [PubMed]
- Lee SH, Nam JK, Park JK, et al. Differential protein expression and novel biomarkers related to 5-FU resistance in a 3D colorectal adenocarcinoma model. Oncol Rep 2014;32:1427-34. [Crossref] [PubMed]
- Bidadi B, Liu D, Kalari KR, et al. Pathway-Based Analysis of Genome-Wide Association Data Identified SNPs in HMMR as Biomarker for Chemotherapy- Induced Neutropenia in Breast Cancer Patients. Front Pharmacol 2018;9:158. [Crossref] [PubMed]
- Song YJ, Tan J, Gao XH, et al. Integrated analysis reveals key genes with prognostic value in lung adenocarcinoma. Cancer Manag Res 2018;10:6097-108. [Crossref] [PubMed]
- Chu ZP, Dai J, Jia LG, et al. Increased expression of long noncoding RNA HMMR-AS1 in epithelial ovarian cancer: an independent prognostic factor. Eur Rev Med Pharmacol Sci 2018;22:8145-50. [PubMed]
- Koelzer VH, Huber B, Mele V, et al. Expression of the hyaluronan-mediated motility receptor RHAMM in tumor budding cells identifies aggressive colorectal cancers. Hum Pathol 2015;46:1573-81. [Crossref] [PubMed]
- Locke J, Joseph AP, Pena A, et al. Structural basis of human kinesin-8 function and inhibition. Proc Natl Acad Sci U S A 2017;114:E9539-48. [Crossref] [PubMed]
- Alfarsi LH, Elansari R, Toss MS, et al. Kinesin family member-18A (KIF18A) is a predictive biomarker of poor benefit from endocrine therapy in early ER+ breast cancer. Breast Cancer Res Treat 2019;173:93-102. [Crossref] [PubMed]
- Zhu H, Xu W, Zhang H, et al. Targeted deletion of Kif18a protects from colitis-associated colorectal (CAC) tumors in mice through impairing Akt phosphorylation. Biochem Biophys Res Commun 2013;438:97-102. [Crossref] [PubMed]