The correlations between socioeconomic status and intrahepatic cholangiocarcinoma in the United States: a population-based study
Introduction
Primary liver cancer is one of the most common types of cancer and is a leading cause of cancer-related mortality globally. Approximately 35,660 patients are diagnosed with this malignancy in the United States (US) annually (1). Among primary liver cancer cases, approximately 15% are cases of intrahepatic cholangiocarcinoma (ICC). Over the past three decades, the incidence of ICC has increased by 165% from approximately 0.35 cases per 100,000 in the US, as indicated by the National Cancer Institute’s Surveillance, Epidemiology, and End Results (SEER) program database. ICC is typically advanced or fatal when diagnosed, making it more difficult to treat (2-5). Over the past two decades, management strategies have been developed for with continuous progress in surgical methods, systemic chemotherapy and targeted radiation therapy keeping update (6). Choosing a best appropriate treatment for individualized cancer therapy needs precise staging and prognostic stratification to distinguish risk populations.
Accumulating findings have indicated that several risk factors can be induced biliary malignancies, including congenital abnormalities, sclerosing cholangitis (PSC) intrahepatic lithiasis, parasitic infection, toxic or occupational exposures, chronic liver disease, metabolic abnormalities, and cirrhosis. Additionally, patients without exposure to known risk factors may also suffer from ICC (7). Recently, some studies have indicated that patients with lower socioeconomic factors (SES) may be at higher risk of developing cancer (8,9). In addition, there two studies reported that SES influenced ICC treatment decisions and patient survival (10,11). To help further stratify at-risk populations and optimize ICC screening, therefore, it is vital to study the effects of SES on ICC incidence and survival,
In this study, we aimed to show the correlations between SES and ICC in the US, on the Surveillance, Epidemiology, and End Results (SEER) database. Using disadvantageous SES, we generated a prognostic score model for risk stratification for ICC. We hypothesis that low-risk socioeconomic status (SES) confers protective effects in ICC. We present the following article in accordance with the STROBE reporting checklist (available at http://dx.doi.org/10.21037/tcr-20-2506).
Methods
Data source
The SEER database is supported by the US National Cancer Institute, and it collects information on patient demographics and case characteristics from 18 population-based cancer registries. Data on cancer incidence and survival are published using this database. The dataset used for the current retrospective study was the April 2018 release of SEER whose patients diagnosed 1973–2015, which includes data about 30% of the US population. SEER is an open-access database, and thus it is available for research. We obtained permission to access the data files with the reference number 12948-Nov2015. The study was conducted in accordance with the Declaration of Helsinki (as revised in 2013) and the study approved by the ethics committee of the 4th Affiliated Hospital of Harbin Medical University, Harbin, China (Ethic No. 2020-SCILLSC-05). As the data extracted from this database were anonymized and de-identified before release, participants were not needed to supply informed consent.
Study population
We included ICC patients identified with using the International Classification of Diseases for Oncology, 3rd Edition (ICD-O-3), topography code C22.0 (primary liver cancer) and morphology codes 8160 and 8161, or by topography code C22.1 (intrahepatic bile duct cancer) and morphology codes 8010, 8020, 8140, 8160, and 8161, which diagnostic criteria for ICC were consisted with previous study (12). We will define cut-points for “% unemployment rate” and “%< High School Education” quintiles for all US counties combined using 2011–2015 ACS data. To be consistent in our analyses, we will use the same US cut-points for the incidence and survival, as described in https://seer.cancer.gov/seerstat/tutorials/rate5/webprint/. Thus, we excluded patients who were diagnosed before 2011. All the included patients were diagnosed between 2011 and 2015. We excluded ineligible cases using the following criteria: (I) patients with more than one primary tumor, (II) patients aged <18 years or with an unknown age at diagnoses, and (III) patients missing data on TNM category, insurance records, or marital status.
Demographic characteristics and clinical variables included age at diagnosis, sex, race, TNM stage, and first-course surgery. The races included white, black, and other (American Indian/Alaska Native and Asian/Pacific Islander). TNM stages were measured using the 6th edition of the American Joint Committee on Cancer staging system. We classified treatment strategies as binary values, namely first-course surgery (i.e., surgery) or no treatment, as there was insufficient relevant information on first-course surgery. The socioeconomic factors assessed in the present study included marital status, insurance status, median household income, unemployment rate, residence, and county educational levels. Data on median household income, unemployment rate, and county educational levels were obtained using the country attribute ACS-2011-2015 datasets. County educational levels were assessed for patients aged ≥25 years, who had at least a high school diploma. Area of residence was classified as a metro area, non-metro urban area, or non-metro rural area based on the 2003 Rural-Urban Continuum Codes. Marital status was classified as married and unmarried (including single, separated/divorced, and widowed) while insurance status was classified as insured and uninsured.
The primary outcomes of interest were age-adjusted incidence rates, cause-specific survival (CSS), and overall survival (OS). ICC incidence rates per 100,000 persons were directly age-adjusted to the 2000 US population. For the analysis of CSS, deaths attributed to ICC were events, while deaths from other causes were censored, and surviving patients were censored at last follow-up. OS represent for the time from the date of diagnosis to the date of death. There were no restrictions on the cause of death.
Statistical analysis
For continuous variables, descriptive statistics were converted into categorical variables based on the interquartile range (IQR) (i.e., median household income, unemployment rates, and county educational levels). Follow-up times were reported as medians and IQRs. ICC incidence rates performed with rate session of SEER*Stat. all steps were carried out as described by the official website of SEER. Univariate and multivariate Cox regression analyses were performed to quantify the impact of socioeconomic factors on survival outcomes. Disadvantageous SES was used to set up a prognostic score model for risk stratification, then the Kaplan-Meier method and the log-rank test were performed to identify the influence of SES on for ICC CSS/OS. The stratified analysis were performed at gender or age at diagnosis subgroup to assess the potential reasons for the survival disparity between the patient subgroups with different scores. Statistical analyses were performed using SEER*Stat 8.3.5, or SPSS, version 21.0 (SPSS Inc., Chicago, IL, USA). Two-sided tests were considered significant if P<0.05.
Results
Patient baseline characteristics and effect of socioeconomic factors on ICC incidence rates and rates ratio
Overall, 3,456 ICC patients diagnosed between 2011 and 2015 were enrolled in this study (Figure 1). Table 1 displays the baseline characteristics of the enrolled patients with ICC. The median follow-up time was 6 (IQR, 2–16) months, and the median age was 66 (IQR, 57–74) years. The last follow-up date was December 31, 2015. The male-to-female ratio was 1.06:1. The rate ratios (RR) for ICC incidence in females was lower than that in males (0.806 vs. 1, P<0.001). Among different races, the RR in American Indian/Alaska Native (AI) was 1.243, which was higher than that in other races and lowest in white (P<0.001). The RR in a non-metro urban area was lower than that in the metro area (0.836 vs. 1, P=0.003). RR for ICC incidence increased monotonically with ages (P<0.001). RRs decrease with increasing county education levels for ICC(P<0.001). The RR in high unemployment rate (> Quartile 3) was 0.805, which was lower than that in other stratums (P=0.007). The RR in the high median housed income rate (> Quartile 3) was 1.357, which was the highest in these strata (P<0.001) (Table 1).
Table 1
Variable | N | Pop | Rate | RR | 95% CI | P |
---|---|---|---|---|---|---|
Sex | ||||||
Male | 2,529 | 172,930,831 | 1.494 | 1 | Reference | |
Female | 2,455 | 180,430,159 | 1.203 | 0.806 | 0.761–0.853 | <0.001 |
Age | ||||||
18–44 | 205 | 151,600,151 | 0.150 | 1 | Reference | |
45–54 | 504 | 61,074,142 | 0.806 | 5.391 | 4.573–6.377 | <0.001 |
55–64 | 1,247 | 52,973,324 | 2.341 | 15.662 | 13.500–18.255 | <0.001 |
65–74 | 1,412 | 32,552,039 | 4.393 | 29.384 | 25.358–34.212 | <0.001 |
>75 | 1,615 | 25,101,436 | 6.428 | 43.001 | 37.156–50.007 | <0.001 |
Race | ||||||
White | 3,950 | 265,798,955 | 1.329 | 1 | Reference | |
Black | 392 | 43,383,759 | 1.045 | 0.786 | 0.704–0.876 | <0.001 |
API | 36 | 5,577,534 | 0.844 | 0.635 | 0.434–0.895 | 0.007 |
AI | 594 | 38,600,742 | 1.651 | 1.243 | 1.136–1.357 | <0.001 |
Education level‡ | ||||||
< Quartile 1 | 1,351 | 71,345,128 | 1.723 | 1 | Reference | |
< Quartile 2 | 1,401 | 84,916,625 | 1.555 | 0.902 | 0.835–0.974 | 0.009 |
< Quartile 3 | 1,036 | 76,342,535 | 1.301 | 0.755 | 0.694–0.820 | <0.001 |
> Quartile 3 | 1,195 | 90,696,804 | 1.303 | 0.756 | 0.698–0.819 | <0.001 |
Unemployment level‡ | ||||||
< Quartile 1 | 194 | 11,231,121 | 1.422 | 1 | Reference | |
< Quartile 2 | 1,074 | 65,451,933 | 1.539 | 1.082 | 0.925–1.271 | 0.345 |
< Quartile 3 | 2,126 | 140,234,874 | 1.399 | 0.984 | 0.846–1.148 | 0.851 |
> Quartile 3 | 1,596 | 136,641,258 | 1.145 | 0.805 | 0.691–0.942 | 0.007 |
Median housed income‡ | ||||||
< Quartile 1 | 240 | 24,901,604 | 0.803 | 1 | Reference | |
< Quartile 2 | 452 | 39,653,072 | 1.007 | 1.254 | 1.066–1.478 | 0.006 |
< Quartile 3 | 552 | 54,133,604 | 0.945 | 1.176 | 1.005–1.379 | 0.043 |
> Quartile 3 | 3,741 | 321,806,008 | 1.090 | 1.357 | 1.187–1.557 | <0.001 |
Resident‡ | ||||||
Metro area | 4,446 | 394,77,102 | 1.066 | 1 | Reference | |
Non-metro urban area | 465 | 40,321,396 | 0.891 | 0.836 | 0.756–0.922 | 0.003 |
Non-metro rural area | 73 | 4,813,276 | 1.059 | 0.993 | 0.993–0.772 | 1.000 |
‡, all data are county-level; education level is the percentage of patients aged ≥25 years with at least a high school diploma. CI, confidence interval; RR, rates ratios; SES, socioeconomic status.
The effect of socioeconomic factors on CSS and OS
Using univariate Cox regression analysis, we observed that most variables had significant differences (P<0.05; Table 2). Especially for socioeconomic factors, compared with married patients, unmarried patients showed poorer CSS and OS (HR, 1.230; 95% CI, 1.134–1.335; HR, 1.225; 95% CI, 1.132–1.326; respectively). In addition, compared with uninsured patients, insured showed better CSS and OS (HR, 0.810; 95% CI, 0.728–0.900; HR, 0.785; 95% CI, 0.710–0.869; respectively). Moreover, compared with lower household income (< Quartile 1), higher household income had better CSS and OS, respectively. Then, we carried out the multivariate Cox regression analysis. The results showed that compared with married patients, unmarried patients showed poorer CSS and OS (HR, 1.229; 95% CI, 1.097–1.377; HR, 1.205; 95% CI, 1.079–1.345; respectively). In addition, compared with uninsured patients, insured showed better CSS and OS (HR, 0.846; 95% CI, 0.731–0.979; HR, 0.813; 95% CI, 0.706–0.936; respectively). Moreover, compared with lower household income (< Quartile 1), higher household income had better CSS and OS, respectively.
Table 2
Variable | Patient No. (%) | CSS | OS | ||||
---|---|---|---|---|---|---|---|
Univariate analysis, HR (95% CI) | Multivariate analysis, HR (95% CI) | Univariate analysis, HR (95% CI) | Multivariate analysis, HR (95% CI) | ||||
Gender | |||||||
Male | 1,763 (51.0) | Reference | Reference | Reference | Reference | ||
Female | 1,693 (49.0) | 0.837 (0.771–0.910)** | 0.807 (0.723–0.902)** | 0.901 (0.833–0.975)* | 0.807 (0.726–0.897)** | ||
Age at diagnosis, year | |||||||
<45 | 168 (4.9) | Reference | Reference | Reference | Reference | ||
45–65 | 1,461 (42.3) | 1.349 (1.100–1.654)* | 1.397 (1.072–1.819)* | 1.330 (1.091–1.622)* | 1.401 (1.080–1.817)* | ||
65+ | 1,827 (52.9) | 1.801 (1.472–2.205)** | 1.845 (1.417–2.403)** | 1.827 (1.501–2.222)** | 1.919 (1.480–2.487)** | ||
Race | |||||||
White | 2,698 (78.1) | Reference | Reference | Reference | Reference | ||
Black | 285 (8.2) | 1.107 (0.957–1.280) | 1.050 (0.868–1.270) | 1.116 (0.970–1.285) | 1.113 (0.928–1.336) | ||
Other | 468 (13.5) | 0.953 (0.845–1.074) | 0.977 (0.828–1.152) | 0.968 (0.863–1.087) | 0.976 (0.833–1.146) | ||
Marital status | |||||||
Married | 2,041 (59.1) | Reference | Reference | Reference | Reference | ||
Unmarried | 1,415 (40.9) | 1.230 (1.134–1.335)** | 1.229 (1.097–1.377)** | 1.225 (1.132–1.326)** | 1.205 (1.079–1.345)* | ||
Insurance status | |||||||
Uninsured | 611 (17.7) | Reference | Reference | Reference | Reference | ||
Insured | 2,845 (82.3) | 0.810 (0.728–0.900)** | 0.846 (0.731–0.979)* | 0.785 (0.710–0.869)** | 0.813 (0.706–0.936)* | ||
Education level‡ | |||||||
< Quartile 1 (9.53%) | 2,645 (76.5) | Reference | Reference | Reference | Reference | ||
< Quartile 2 (13.06%) | 307 (8.9) | 1.190 (1.034–1.369)* | 1.119 (0.924–1.355) | 1.191 (1.039–1.365)* | 1.135 (0.942–1.369) | ||
< Quartile 3 (18.74%) | 450 (13.0) | 1.193 (1.057–1.347)* | 1.200 (0.981–1.467) | 1.229 (1.094–1.381)* | 1.022 (0.955–1.210) | ||
≥ Quartile 3 (18.74%) | 54 (1.6) | 1.324 (0.985–1.780) | 1.136 (0.779–1.656) | 1.333 (1.001–1.776)* | 1.178 (0.817–1.697) | ||
Unemployment rate‡ | |||||||
< Quartile 1 | 125 (3.6) | Reference | Reference | ||||
< Quartile 2 | 743 (21.5) | 1.128 (0.892–1.426) | – | 1.129 (0.898–1.420) | – | ||
< Quartile 3 | 1484 (42.9) | 1.129 (0.900–1.416) | – | 1.146 (0.9149–1.429) | – | ||
≥ Quartile 3 | 1104 (31.9) | 1.219 (0.969–1.533) | – | 1.241 (0.993–1.552) | – | ||
Median household income‡ | |||||||
<Quartile 1 (US $5,289) | 865 (25.0) | Reference | Reference | Reference | Reference | ||
<Quartile 2 (US $5,995) | 892 (25.8) | 0.887 (0.793–0.992)* | 0.793 (0.672–0.936)* | 0.903 (0.810–0.989)* | 0.806 (0.709–0.915)* | ||
<Quartile 3 (US $7,446) | 852 (24.7) | 0.835 (0.746–0.934)* | 0.853 (0.725–0.946)* | 0.840 (0.753–0.937)* | 0.813 (0.713–0.927)* | ||
≥Quartile 3 (US $7,446) | 847 (24.5) | 0.774 (0.690–0.868)** | 0.749 (0.630–0.891)* | 0.804 (0.720–0.869)** | 0.801 (0.696–0.921)* | ||
Residence‡ | |||||||
Metro area | 3,099 (89.7) | Reference | Reference | Reference | Reference | ||
Non–metro urban area | 241 (7.0) | 1.164 (0.999–1.355) | 1.167 (0.946–1.440) | 1.135 (0.978–1.318) | 1.160 (0.943–1.427) | ||
Non–metro rural area | 93 (2.7) | 1.350 (1.067–1.708)* | 1.229 (0.892–1.692) | 1.337 (1.063–1.680)* | 1.213 (0.884–1.664) | ||
T category | |||||||
T1 | 891 (25.8) | Reference | Reference | Reference | Reference | ||
T2 | 400 (11.6) | 1.057 (0.929–1.202) | 1.042 (0.881–1.2338) | 1.030 (0.910–1.167)** | 1.273 (1.122–1.444)** | ||
T3 | 772 (22.3) | 1.381 (1.225–1.558)** | 1.228 (1.077–.1402)* | 1.339 (1.192–1.505)** | 1.303 (1.179–1.440)** | ||
T4 | 406 (11.7) | 1.438 (1.247–1.658)** | 1.255 (1.074–1.467)* | 1.397 (1.216–1.604)** | 1.257 (1.110–1.422)** | ||
N category | |||||||
N0 | 2,089 (60.4) | Reference | Reference | Reference | Reference | ||
N1 | 820 (23.7) | 1.165 (1.056–1.285)* | 1.034 (0.917–1.167) | 1.141 (1.037–1.256)* | 1.035 (0.920–1.164) | ||
M category | |||||||
M0 | 1,672 (48.4) | Reference | Reference | Reference | Reference | ||
M1 | 1,461 (42.3) | 1.903 (1.744–2.076)** | 1.478 (1.320–1.656)** | 1.841 (1.692–2.003)** | 1.437 (1.287–1.605)** | ||
First–course surgery | |||||||
No | 2,872 (83.1) | Reference | Reference | Reference | Reference | ||
Yes | 577 (16.7) | 0.215 (0.186–0.250)** | 0.284 (0.240–0.337)** | 0.220 (0.190–0.254)** | 0.228 (0.244–0.339)** |
‡, all data are county-level; education level is the percentage of patients aged ≥25 years with at least a high school diploma. *, P<0.050; **, P value ≤0.001. CI, confidence interval. Other races (American Indian/AK Native, Asian/Pacific Islander). CSS, cause-specific survival; ICC, intrahepatic cholangiocarcinoma; OS, overall survival; TNM, tumor-node metastasis; SES, socioeconomic status
Establishment and application of a prognostic score model
Three disadvantageous socioeconomic factors: unmarried status, uninsured status, and median household income <USD$5,289 were used to set up a prognostic score model. Consequently, the scores for patients with ICC were 0, 1, 2, or 3, showing an increased risk of mortality in degrees. For the subgroups with scores of 0 (n=1,335), 1 (n=1,446), 2 (n=580), and 3 (n=95), the 1-year cumulative OS rates were 41.0%, 31.0%, 29.3%, and 14.9%, respectively. The 3-year cumulative OS rates were 13.7%, 10.2%, 7.0%, and 0%, respectively. The 1-year cumulative CSS rates were 43.1%, 33.0%, 30.6%, and 17.0%, respectively. The 3-year cumulative CSS rates were 15.4%, 11.2%, 8.1%, and 0%, respectively (P<0.001; Figure 2). To assess the potential reasons for the survival disparity between the patient subgroups with different scores, we further explored the effect of gender and age at diagnosis on survival. We used Kaplan-Meier analysis to calculate the OS and CSS of ICC patients in the male and female subgroups (Figure 3). Figure 4 presents the Kaplan-Meier analysis to calculate the OS and CSS of ICC patients in the age at diagnosis subgroups. The results showed that, compared with the high scores patients, the lower scores patients had better OS and CSS outcome.
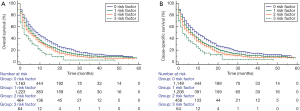
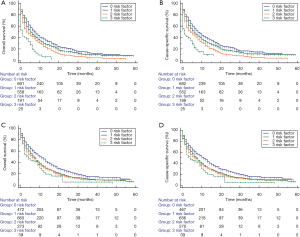
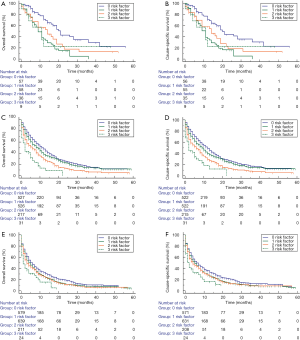
Discussion
In this study, we assess the correlations between SES and ICC incidence and survival in the US. The result shows that low county education levels may be associated with increased ICC risk. Importantly, we found that median household income, insurance status, and marital status were significant predictors of survival outcomes. Establishment and application of a prognostic score model, we found that ICC patients with low-risk SES had better survival outcomes.
The US is a nation with a robust medical system. However, the associations between SES and ICC incidence and survival remain not very clear. Socioeconomic barriers may increase the cancer burden at the early diagnosis stage, and disparities in income are associated with delayed diagnosis of more advanced cancers (13). Studies have indicated that individuals with a higher-level SES are more likely to participate in screening or have better access to high-quality treatment throughout disease progression (8). Conversely, those with a lower level SES, including those with universal health care (14,15), are expected to have relatively worse cancer survival rates and to have an increased risk of mortality due to obstacles to obtaining treatment before cancer has become incurable (16). The data for each patient were collected in a unified, standardized manner by the SEER program. Therefore, the SEER database was suitable to assess the impact of socioeconomic factors on the incidence and survival of ICC in this study.
Our study also reveals the incidence of ICC in men is slightly higher than that in women, which is consist with previous stduy(17), and men had more reduced survival than females for most cancers (18,19). Some studies have reported that increased cancer risk in men may be associated with their larger body sizes and higher basal metabolic rates (BMR) (20). Increased cell proliferation in men could also be associated with a higher risk of cancer (21). However, the larger body size is not a decisive factor in determining the increased risk of cancers, and associations include not only sex but also other causal factors (22). Most studies have concentrated on body mass index or weight, which might not be specific or reliable markers of cell numbers (20). Some studies have pointed out that some possible biological determinants vary significantly in sex. There are sex-related differences in susceptibility, sex steroid hormone levels, chronic antigenic stimuli and immune response to infectious agents (23). Some studies have demonstrated that being married is protective against many malignant tumors and have better survival (24-27). Such studies support the belief that, as compared with unmarried patients, patients who are married may have better access to health care and more significant financial resources for timely detection and treatment. This phenomenon has been explained by some psychological, biological, and social theories (28). Conversely, it is believed that unmarried and widowed patients are at higher risk of experiencing mental depression and psychological distress, as such patients lack sufficient social and emotional support (29,30). Therefore, patients with adequate emotional support might have better persistence with prescribed treatments and healthier lifestyles than unmarried or widowed patients (31). The potential mechanisms driving this relationship might be related to immune and endocrine function (31).
In our study, we found that median household income, insurance status, and marital status were independent predictors of survival outcomes. Using above three disadvantageous SES, we generated a prognostic score model for risk stratification, then we found that ICC patients with low-risk SES had better survival outcomes. Those with a higher income or with insurance can obtain better financial support, enabling them to receive adequate treatment promptly. A report from National Inpatient Sample Database (1997–2012) demonstrated that the mean hospitalization costs per patient increased from $36,460 to $77,753 over the study period (32). Patients with high household income living in a suitable environment may have better access to care that could improve their prognosis. Further, they may have a higher capacity to improve their health status because of their knowledge, social connections, financial abilities, and other resources. In contrast, social isolation, depression, and occupational stress in individuals with low household incomes may make it more challenging for them to gain helpful opinions from relatives or friends (33). Some studies have demonstrated that being insured is correlated with cancer-specific mortality, even in patients with non-metastatic disease. Patients without insurance may suffer more significant financial distress and inability to afford cancer care; such difficulties may result in giving up medical care (34).
Our findings that race, unemployment, place of residence, and county educational level are not significantly associated with survival outcomes for ICC were not consistent with previous studies, which reported the significant effects of these factors on patients with ICC (35,36). These discrepancies may be caused by the different geographical settings of the studies, which may be affected by various factors, such as the types of residences, unbalanced county educational backgrounds, and other socioeconomic factors. Furthermore, categorical variables (e.g., residence) in the earlier studies were classified based on standards that were specific to each data source, which may also have led to different outcomes.
This study has some limitations that should be taken into consideration. First, the SEER database does not provide all characteristics that are potentially correlated with socioeconomic factors. Furthermore, detailed etiological and treatment data were not recorded in the SEER database, such as the lifestyles of patients, occupational exposures, and chemotherapy regimens. These and similar factors may be correlated with the incidence and survival of patients with ICC. Second, unpredictable changes in socioeconomic factors, such as marital status, may have occurred after registration or during therapy. As previously discussed, marital status has a significant impact on health, and thus, changes in marital status are likely to have impacted the associations investigated in this study (37,38). Third, it is necessary to point out that education levels, unemployment rates and median housed income used in our analysis were measured at the county-level, which may vary significantly across census tracts or neighborhoods within a given county. They are contextual-level variables, not the individuals in this study.
Conclusions
The rates of ICC incidence and mortality are high in the US and have been increasing. There is a need for further studies to identify groups that are at high risk of ICC and predict their survival outcomes, who might benefit from screening and surveillance programs focused on earlier diagnosis and timely treatment. Therefore, SES may have significance for predicting incidence and survival outcomes.
Acknowledgments
Funding: None.
Footnote
Reporting Checklist: The authors have completed the STROBE reporting checklist. Available at http://dx.doi.org/10.21037/tcr-20-2506
Conflicts of Interest: Both authors have completed the ICMJE uniform disclosure form (available at http://dx.doi.org/10.21037/tcr-20-2506). The authors have no conflicts of interest to declare.
Ethical Statement: The authors are accountable for all aspects of the work in ensuring that questions related to the accuracy or integrity of any part of the work are appropriately investigated and resolved. The study was conducted in accordance with the Declaration of Helsinki (as revised in 2013) and the study was approved by the ethics committee of the 4th Affiliated Hospital of Harbin Medical University, Harbin, China (Ethic No. 2020-SCILLSC-05). As the data extracted from this database were anonymized and de-identified before release, participants were not needed to supply informed consent.
Open Access Statement: This is an Open Access article distributed in accordance with the Creative Commons Attribution-NonCommercial-NoDerivs 4.0 International License (CC BY-NC-ND 4.0), which permits the non-commercial replication and distribution of the article with the strict proviso that no changes or edits are made and the original work is properly cited (including links to both the formal publication through the relevant DOI and the license). See: https://creativecommons.org/licenses/by-nc-nd/4.0/.
References
- Siegel RL, Miller KD, Jemal A. Cancer statistics, 2015. CA Cancer J Clin 2015;65:5-29. [Crossref] [PubMed]
- Brandi G. Hot topics in cholangiocarcinoma. Transl Cancer Res 2019;8:S219-22. [Crossref]
- Shaib YH, Davila JA, McGlynn K, et al. Rising incidence of intrahepatic cholangiocarcinoma in the United States: a true increase? J Hepatol 2004;40:472-7. [Crossref] [PubMed]
- Lee AJ, Chun YS. Intrahepatic cholangiocarcinoma: the AJCC/UICC 8th edition updates. Chin Clin Oncol 2018;7:52.
- Ding Y, Sun Z, You W, et al. Lymphoepithelioma-like intrahepatic cholangiocarcinoma with Epstein-Barr virus infection: report of a rare case. Ann Transl Med 2019;7:497. [Crossref] [PubMed]
- Doherty B, Nambudiri VE, Palmer WC. Update on the Diagnosis and Treatment of Cholangiocarcinoma. Curr Gastroenterol Rep 2017;19:2. [Crossref] [PubMed]
- Plentz RR, Malek NP. Clinical presentation, risk factors and staging systems of cholangiocarcinoma. Best Pract Res Clin Gastroenterol 2015;29:245-52. [Crossref] [PubMed]
- Clegg LX, Reichman ME, Miller BA, et al. Impact of socioeconomic status on cancer incidence and stage at diagnosis: selected findings from the surveillance, epidemiology, and end results: National Longitudinal Mortality Study. Cancer Causes Control 2009;20:417-35. [Crossref] [PubMed]
- Donnelly DW, Hegarty A, Sharp L, et al. The Impact of Adjustment for Socioeconomic Status on Comparisons of Cancer Incidence between Two European Countries. J Cancer Epidemiol 2013;2013:612514.
- Uhlig J, Sellers CM, Cha C, et al. Intrahepatic Cholangiocarcinoma: Socioeconomic Discrepancies, Contemporary Treatment Approaches and Survival Trends from the National Cancer Database. Ann Surg Oncol 2019;26:1993-2000. [Crossref] [PubMed]
- Lee RM, Liu Y, Gamboa AC, et al. Race, ethnicity, and socioeconomic factors in cholangiocarcinoma: What is driving disparities in receipt of treatment? J Surg Oncol 2019;120:611-23. [PubMed]
- Welzel TM, Graubard BI, Zeuzem S, et al. Metabolic syndrome increases the risk of primary liver cancer in the United States: a study in the SEER-Medicare database. Hepatology 2011;54:463-71. [Crossref] [PubMed]
- Woods LM, Rachet B, Coleman MP. Origins of socio-economic inequalities in cancer survival: a review. Ann Oncol 2006;17:5-19. [Crossref] [PubMed]
- Wu CC, Chang CM, Hsu TW, et al. The effect of individual and neighborhood socioeconomic status on esophageal cancer survival in working-age patients in Taiwan. Medicine (Baltimore) 2016;95:e4140. [Crossref] [PubMed]
- Chang CM, Su YC, Lai NS, et al. The combined effect of individual and neighborhood socioeconomic status on cancer survival rates. PLoS One 2012;7:e44325. [Crossref] [PubMed]
- Kogevinas M, Marmot MG, Fox AJ, et al. Socioeconomic differences in cancer survival. J Epidemiol Community Health 1991;45:216-9. [Crossref] [PubMed]
- Bergquist A, von Seth E. Epidemiology of cholangiocarcinoma. Best Pract Res Clin Gastroenterol 2015;29:221-32. [Crossref] [PubMed]
- Innos K, Padrik P, Valvere V, et al. Sex differences in cancer survival in Estonia: a population-based study. BMC Cancer 2015;15:72. [Crossref] [PubMed]
- Cook MB, McGlynn KA, Devesa SS, et al. Sex disparities in cancer mortality and survival. Cancer Epidemiol Biomarkers Prev 2011;20:1629-37. [Crossref] [PubMed]
- Kizer NT, Thaker PH, Gao F, et al. The effects of body mass index on complications and survival outcomes in patients with cervical carcinoma undergoing curative chemoradiation therapy. Cancer 2011;117:948-56. [Crossref] [PubMed]
- Tomasetti C, Li L, Vogelstein B. Stem cell divisions, somatic mutations, cancer etiology, and cancer prevention. Science 2017;355:1330-4. [Crossref] [PubMed]
- Roberts DL, Dive C, Renehan AG. Biological mechanisms linking obesity and cancer risk: new perspectives. Annu Rev Med 2010;61:301-16. [Crossref] [PubMed]
- Whitacre CC. Sex differences in autoimmune disease. Nat Immunol 2001;2:777-80. [Crossref] [PubMed]
- Costa LJ, Brill IK, Brown EE. Impact of marital status, insurance status, income, and race/ethnicity on the survival of younger patients diagnosed with multiple myeloma in the United States. Cancer 2016;122:3183-90. [Crossref] [PubMed]
- He XK, Lin ZH, Qian Y, et al. Marital status and survival in patients with primary liver cancer. Oncotarget 2016;8:64954-63. [Crossref] [PubMed]
- Shi RL, Qu N, Lu ZW, et al. The impact of marital status at diagnosis on cancer survival in patients with differentiated thyroid cancer. Cancer Med 2016;5:2145-54. [Crossref] [PubMed]
- Qiu M, Yang D, Xu R. Impact of marital status on survival of gastric adenocarcinoma patients: Results from the Surveillance Epidemiology and End Results (SEER) Database. Sci Rep 2016;6:21098. [Crossref] [PubMed]
- Aizer AA, Chen MH, McCarthy EP, et al. Marital status and survival in patients with cancer. J Clin Oncol 2013;31:3869-76. [Crossref] [PubMed]
- Goldzweig G, Andritsch E, Hubert A, et al. Psychological distress among male patients and male spouses: what do oncologists need to know? Ann Oncol 2010;21:877-83. [Crossref] [PubMed]
- Baine M, Sahak F, Lin C, et al. Marital status and survival in pancreatic cancer patients: a SEER based analysis. PLoS One 2011;6:e21052. [Crossref] [PubMed]
- Garssen B, Goodkin K. On the role of immunological factors as mediators between psychosocial factors and cancer progression. Psychiatry Res 1999;85:51-61. [Crossref] [PubMed]
- Wadhwa V, Jobanputra Y, Thota PN, et al. Healthcare utilization and costs associated with cholangiocarcinoma. Gastroenterol Rep (Oxf) 2017;5:213-8. [PubMed]
- Wang JJ, Snyder M, Kaas M. Stress, loneliness, and depression in Taiwanese rural community-dwelling elders. Int J Nurs Stud 2001;38:339-47. [Crossref] [PubMed]
- Kent EE, Forsythe LP, Yabroff KR, et al. Are survivors who report cancer-related financial problems more likely to forgo or delay medical care? Cancer 2013;119:3710-7. [Crossref] [PubMed]
- Galobardes B, Shaw M, Lawlor DA, et al. Indicators of socioeconomic position (part 1). J Epidemiol Community Health 2006;60:7-12. [Crossref] [PubMed]
- Mosadeghi S, Liu B, Bhuket T, et al. Sex-specific and race/ethnicity-specific disparities in cholangiocarcinoma incidence and prevalence in the USA: An updated analysis of the 2000-2011 Surveillance, Epidemiology and End Results registry. Hepatol Res 2016;46:669-77. [Crossref] [PubMed]
- Zeng C, Wen W, Morgans AK, et al. Disparities by Race, Age, and Sex in the Improvement of Survival for Major Cancers: Results From the National Cancer Institute Surveillance, Epidemiology, and End Results (SEER) Program in the United States, 1990 to 2010. JAMA Oncol 2015;1:88-96. [Crossref] [PubMed]
- Jaremka LM, Glaser R, Malarkey WB, et al. Marital distress prospectively predicts poorer cellular immune function. Psychoneuroendocrinology 2013;38:2713-9. [Crossref] [PubMed]