Comprehensive analysis of transcriptome data stemness indices identifies key genes for controlling cancer stem cell characteristics in gastric cancer
Introduction
Gastric cancer (GC) is one of the leading causes of death worldwide, with approximately 1.2 million incident cases and about 865,000 deaths (1). Although many achievements have been obtained for GC treatment, the 5-year survival rate of GC patients with advanced stage still remains low (2,3).
Cancer stem cells (CSCs), the cancer cell-of-origin, are characterized by having the capabilities of self-renewal or differentiation, which may serve as a trigger of tumorigenesis, malignant progression, metastasis and multi-drug resistance (4-7). Cancer stemness index (SI), a parameter for assessing the degree of oncogenic dedifferentiation, has been widely studied with artificial intelligence and deep learning methods. Recently, it has been reported two independent stemness indices, namely, mRNA SI and mDNA SI, can be used to distinguish the molecular profiles of tumor cells with different stemness degrees. mRNA SI is a reflective of gene expression, while mDNA SI can reflect epigenetic changes (8). Both stemness indices are correlated to the biological processes in CSCs, tumor dedifferentiation, and histopathological classification.
The Cancer Genome Atlas (TCGA), is an open-access integrated database that provides genomic, epigenomic, transcriptomic, and proteomic data along with histopathological and clinical information (9). It has been demonstrated that the specific molecular subtypes of tumors obtained from TCGA are associated with mRNA SI and mDAN SI features (8).
Weighted gene co-expression network analysis (WGCNA), is mainly used to construct gene network by identifying and weighing the gene pairs according to the correlation between expression levels (10,11). Additionally, WGCNA can be applied to discern network topology and subnetwork, also known as modules, in order to measure the similarity between genes. Only genes that have a strong weighted connection with each other in the network can establish a gene expression module. In other words, the genes involved in the module are even more significantly associated with sample traits compared to the comparative genes. Thus far, the role of mRNA SI in several cancers has been evaluated to identify stemness-related genes by comprehensive analysis of TCGA data with WGCNA (12-14). Bai and their colleagues (12) identified 21 key genes as candidate therapeutic targets to inhibit the stemness of liver cancer through WGCNA on TCGA database. Pan Shen and their colleagues (13) also identified 13 key genes that could play vital roles in the maintenance of bladder CSCs through WGCNA using TCGA database. However, the key genes that involved in the stemness maintenance of GC stem cells are still not fully understood.
In this work, mRNA SI score and WGCNA were applied to identify potential stemness-related genes in GC patients. Our novel findings indicated a considerable number of genes associated with GC stemness and offered new insights into the molecular features of GC stem cells. The study was based on the TCGA Research Network: https://www.cancer.gov/tcga, thus, ethical approval was not needed.
Methods
Data acquisition and procession
The transcriptomic data of 32 normal tissue and 375 GC samples were obtained from TCGA database (http://tcga-data.nci.nih.gov/tcga/). Subsequently, the gene IDs were converted to a matrix of gene symbol with the use of Ensembl database (http://asia.ensembl.org/index.html). Next, the clinical data of 443 cases were also retrieved from TCGA database for the subsequent analysis.
mRNA SI scores and clinical significance
To assess the prognostic values of mRNA SI score, survival analysis was carried out by using the “survival” package in R language. Statistical significance was determined by log-rank tests. Next, we evaluated the correlation between mRNA SI scores and GC patients’ clinicopathologic characteristics, including tumor grade, lymph node metastasis, TNM stage, and overall survival (OS).
Differential expression analysis
Differentially expressed genes (DEGs) between normal stomach and GC tissues were determined using the following selection criteria: (I) |log2 fold change| >1; and (II) false discovery rate (FDR) <0.05. For genes with similar names, the fold-change values were averaged. This procedure was completed using “edgeR” package in R.
WGCNA analysis
WGCNA and module preservation
WGCNA analysis was conducted by using the WGCNA package in R software. To ensure the accuracy and heterogeneity of subsequent co-expression network analysis, the genes with the greatest variance (25%) in expression were selected for WGCNA analysis. First, RNA-seq data filtering was carried out to remove outliers. The absolute values of the correlations among mRNA expression levels were determined by a co-expression similarity matrix. The paired genes were constructed through a Pearson’s correlation matrix. A power function amn=|cmn|β (where amn = adjacency between gene m and gene n; cmn =Pearson’s correlation between gene m and gene n) was adopted to construct the weighted adjacency matrix. The parameter β is a soft thresholding estimator that defines a correlation power, and was applied to emphasize and penalize the strong and weak relationships between genes, respectively. Then, a proper β value was chosen to enhance the similarity matrix and provide a scale-free co-expression network. Next, the adjacency matrix was converted into a topological overlap matrix (TOM) to measure the network connectivity among genes, as denoted as the sum of adjacent genes obtained from all other networks. Through the use of TOM-based dissimilarity measure, an average linkage hierarchical clustering was carried out with a minimum size (gene group) of 30. Finally, their dissimilarity was calculated through further analysis of modules, and the module dendrograms were constructed.
Confirmation of significant modules
To explore the association between genes and sample traits, gene significance (GS) was calculated to evaluate the significance of each module. For each gene module, module eigengenes (MEs) were regarded as the pivotal components in the principal component analysis. Next, the expression pattern of each gene was encapsulated as a unitary feature expression profile with specific modules. Based on the linear regression between clinical data and gene expression, GS was interpreted as the log10 conversion of the P value (GS = lgP). Then, module significance (MS) was used to explore the relationship between sample traits and each module, which was defined as the average GS within the module. A cutoff value of less than 0.25 was set to merge all modules with the same length for the sake of increasing the capacity of each module. According to a report by Malta et al. (8), mRNA SI and epigenetically regulated mRNA SI were selected as the clinical phenotypes. Finally, the association between clinical phenotypes and gene expression modules was evaluated.
Identification of key genes
Firstly, modules of interest were selected. Then, GS and module membership (MM, the relationship of the module’s genes and expression profiles) were calculated according to the following threshold values: cor.gene GS >0.5 and cor.gene MM >0.8.
Validation of key genes
To validate the differential mRNA expression levels of key genes between normal tissue and GC tumors, “ggpubr” package in R software was used to calculate their differences. The scatter distribution plot of key genes was then presented in a heatmap.
Gene ontology (GO) and Kyoto encyclopedia of genes and genomes (KEGG) analysis
Through the use of “clusterProfiler” package in R software, GO functional annotation and KEGG pathway enrichment analysis were performed with the following threshold values: P<0.01 and FDR <0.05.
Protein-protein interaction (PPI) analysis
PPI was analyzed by using STRING version 11.0 (https://www.string-db.org,) with multi-seed based regulatory modules.
Gene co-expression analysis
The co-expression relationships between the key genes were determined by Pearson’s correlation analysis. All the calculations were completed using “corrplot” package in R.
Statistical analysis
All statistical analyses in the present study were carried out in R version 3.6.1. The Kaplan-Meier curve was drawn to analyze the survival difference between the normal group and the tumor group. The correlation between key genes was analyzed by Pearson’s correlation analysis. P value <0.05 was considered to be statistically significant.
Results
mRNA SI is correlated with clinical characteristics of GC
By analyzing the transcriptomic data of 32 normal tissue and 375 GC samples, mRNA SI score was found to be markedly increased in tumor tissue compared to normal tissue (Figure 1A). High mRNA SI score was remarkably associated with higher pathological grade, more advanced stage and higher T stage (Figure 1B,C,D). Further, we performed survival analysis between low and high mRNA SI scores. Similar to a recent report by Pan and co-workers (13), GC patients with higher mRNA SI scores exhibited improved OS compared those with lower mRNA SI scores (Figure 1E), which are opposed to our understanding of CSCs.
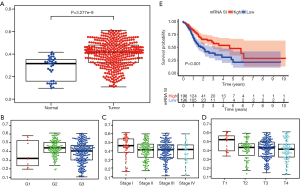
Screening of DEGs
By comparing the tumor and normal tissues of GC patients, 6,739 DEGs were identified, of which 5,592 were upregulated, and 1,147 were downregulated. Volcano map (Figure 2A) shows the distribution of all DEGs in the dimensions of -log (FDR) and log FC; while heatmap (Figure 2B) demonstrates the numerical data representing the expression profiles of DEGs.
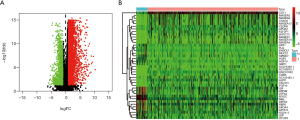
WGCNA analysis
After eliminating outlier samples, the 6,739 DEGs with top 25% of variance were selected for a module. As shown in Figure 3A,B,C, after considering a soft threshold of β=4 (scale-free R2=0.910), 16 modules were obtained for subsequent analysis.
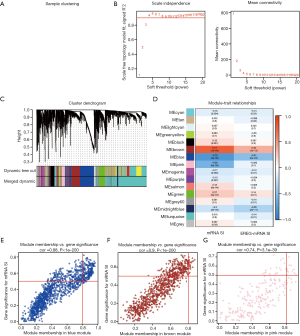
MS was employed to assess the correlation between gene expression modules and clinical phenotypes. Notably, the most remarkable association was found between mRNA SI and blue module, with a correlation value of −0.78. The brown and pink modules, with the correlation values of 0.77 and −0.56 respectively, also exhibited relatively high correlations with mRNA SI (Figure 3D). As a result, the blue module was chosen for subsequent analysis.
To screen the potential key genes, the threshold values of mRNA SI group were set as cor.MM >0.8 and cor.GS >0.5. A total of 19 key genes, namely, BUB1 mitotic checkpoint serine/threonine kinase (BUB1), BUB1 mitotic checkpoint serine/threonine kinase B (BUB1B), kinesin family member 14 (KIF14), non-SMC condensing I complex subunit H (NCAPH), Rac GTPase activating protein 1 (RACGAP1), kinesin family member 15 (KIF15), centromere protein F (CENPF), TPX2 microtubule nucleation factor (TPX2), RAD 54 like (RAD54L), kinesin family member 18B (KIF18B), TTK protein kinase (TTK), kinesin family member 4A (KIF4A), shugoshin 2 (SGO2), polo like kinase 4 (PLK4), Rho GTPase activating protein 11A (ARHGAP11A), X-ray repair cross complementing 2 (XRCC2), chromosome 1 open reading frame 112 (C1orf112), non-SMC condensing I complex subunit G (NCAPG), origin recognition complex subunit 6 (ORC6) were successfully screened out (Figure 3E,F,G).
Validation of the expression changes in key genes
All the 19 key genes were highly expressed in GC tissues compared to normal samples (Figure 4A). The heatmap demonstrated the scatter distribution plot of key genes (Figure 4B).
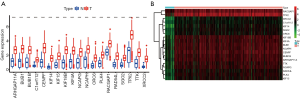
GO and KEGG analysis
GO analysis showed that the 19 key genes were related to biological process (e.g., sister chromatid segregation and nuclear chromosome segregation) as well as cellular component (e.g., spindle, chromosome and centromeric region), suggesting that these key genes are involved in cell proliferation (Figure 5A). In addition, KEGG analysis revealed that these 19 key genes are mainly associated with the pathways in cell cycle (Figure 5B).
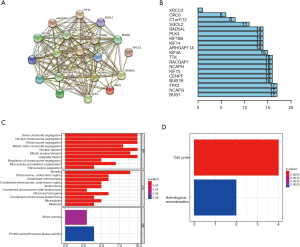
PPI analysis
The PPI relationships among the 19 key genes were analyzed by STRING, and the results demonstrated a strong relationship between these key genes (Figure 5C). Barplot figure showed that BUB1, NCAPG, TPX2 had the most nodes with other genes (Figure 5D).
Gene co-expression analysis
A strong co-expression association was found among the 19 key genes at the transcriptional level (Figure 6). Notably, the highest correlation (0.88) was observed between KIF14 and CENPF, while the lowest correlation (0.52) was between ORC6 and KIF4A.
Discussion
CSCs are considered to be tumorigenic, along with the capabilities of self-renewal and differentiation (4,7). CSCs are believed to have progenitor/stem cell-like features with the loss-of-differentiation state, which play crucial roles in tumor progression, metastasis, and recurrence (4-7). In the present study, CSC stemness-related key genes were identified from WGCNA analysis based on mRNA SI scores. Notably, mRNA SI scores were significantly higher in tumor samples than in normal tissues. Higher mRNA SI scores were associated with more progressive pathological grade, clinical outcomes, and pathological T stage, indicating that CSCs are involved in tumorigenesis and progression.
Furtherly, WGCNA were performed to construct a DEGs co-expression network, in order to uncover biological gene modules relying on average linkage hierarchical clustering and to identify genes associated with GC stemness characteristics. The parameter β in WGCNA was selected as 4 (scale-free R2=0.910) for constructing a scale-free network. To identify the modules of interest, GS and MM were calculated for each gene. To further explore the association between gene module and mRNA SI, MS was defined as the overall gene expression level of a certain module for the subsequent analysis. As illustrated in Figure 3D, the most remarkable association was found between mRNA SI and blue module, with a correlation value of −0.78. Then, in accordance with other reports, the threshold values of cor.gene GS (0.5) and cor.gene MM (0.8) was established to identify key genes in the blue module, and the results showed that a considerable number of genes were identified. The interactions among the proteins encoded by key genes and co-expression association at the transcriptional level were both strong. These findings are consistent with clinical observations demonstrating that these 19 key genes can affect GC development and progression.
As opposed to our understanding of CSCs, GC patients with higher mRNA SI scores exhibited improved OS compared to those with lower mRNA SI scores, which are similar to the findings of Pan and co-workers (13). They also found that mRNA SI is a stemness comprehensive index, which can be affected by the purity of tumor (13). However, the influence of tumor purity can be eliminated by the corrected mRNA SI (mRNAsi/tumor purity). It has been reported that the patients with higher corrected mRNA SI scores may exhibit significantly worse OS. The corrected mRNA SI scores can be calculated based on tumor purity derived from previous findings (15). However, we failed to calculate the corrected mRNA SI scores in GC due to a lack of necessary data.
GO analysis revealed that the 19 key genes were mainly involved in cell proliferation, while KEGG analysis revealed that the 19 key genes were primarily involved in the pathways of cell cycle. All these key genes have been shown to participate in cancer development and pathogenesis. BUB1 is involved in chromosome segregation, and the target inhibition of BUB1 kinase can sensitize tumor cells toward taxanes (16). BUB1B is a vital mitotic spindle checkpoint player, and its overexpression may enhance the progression of prostate cancer (PCa) and correlated with unfavorable clinical features (17). Yang and colleagues have reported that KIF14 is highly expressed in GC samples and cell line, thus promoting GC progression and metastasis (18). Upregulation of NCAPH has been found in colorectal cancer tissue. Silencing NCAPH can suppress colon cancer cell migration and proliferation both in vitro and in vivo, as well as promote cell cycle arrest at G2/M phase (19). RACGAP1 is overexpressed in several cancer cell lines following radiation, and the knockdown of RACGAP1 can inhibit cell viability and invasiveness after radiotherapy (20). KIF15 is highly expressed in pancreatic tumor samples, and its overexpression is associated with shorter survival times and pancreatic cancer growth (21). CENPF is involved in cancer proliferation and metastasis (22,23). Knocking down of CENPF can alter the metabolic profiles of PCa cells and thus inhibit their proliferation (23). TPX2, also known as TPX2 microtubule nucleation factor, has been found to promote cancer cell progression. Silencing of TPX2 exerts anti-cancer effects on various cancer cells (24-26). RAD54L has been found to be associated with tumorigenesis and radioresistance in glioblastoma patients (27). KIF18B has been reported to be upregulated in cutaneous melanoma and correlated with poor clinical outcomes (28). Functional experiments have suggested that KIF18B can aggravate the progression of cervical cancer (29). TTK is associated with the malignancy of PCa, and is thought to be a potential therapeutic target for cancer therapy (30). KIF4A is considered to be a potential gene in mediating tumorigenesis and cancer progression, which is related with shorter OS in a wide variety of tumors (31,32). SGO2 is highly expressed in cutaneous T-cell lymphomas (33). PLK4 is a promising target with potential clinical application, which promotes cancer invasion and metastasis (34,35). Both ARHGAP11A and XRCC2 are highly expressed in hepatocellular carcinoma (36,37), and knockdown of C1orf112 can suppress the growth of HeLa cells (38), indicating these genes may be involved in cancer development. NCAPG has been found to be correlated with shorter disease-free survival and advanced clinical stage. Silencing of NCAPG can suppress the aggressiveness cancer cells (39). ORC6 has been demonstrated to be highly expressed in colorectal cancer tissues, and downregulated expression of ORC6 sensitizes colon cancer cells to chemotherapy (40).
More importantly, some of these key genes have been found to play vital roles in maintaining the stemness of CSCs. Han and colleagues have reported that silencing of BUB1 can reduce the CSC potential of breast cancer cells, demonstrating that this gene is involved in the function of CSCs (41). BUB1B has been found to participate in the stemness maintenance of brain tumor-initiating cells (42). Huang and co-workers have report that TPX2 is associated with the development of breast CSCs, and lovastatin can sensitize breast CSCs to radiotherapy by affecting TPX2 gene expression (43). In addition, RACGAP1 and KIF18B are considered to play critical roles in the regulation of bladder CSCs (13).
Some limitations to the present study should be highlighted. First, the expression and function of these key genes that play vital roles in the stemness maintenance of CSCs in GC patients should be validated in cellular and molecular experiments. Indeed, we will validate our results in the further experiments as soon as possible. Next, non-coding RNA have been recognized to be crucial in maintaining the stemness of CSCs (44-48); however, our study focused on the identification of key genes that controlling stemness of GC stem cells, thus non-coding RNA was not included in our analysis.
Conclusions
In summary, 19 key genes were identified to play a critical role in the stemness maintenance of gastric CSCs. These key genes can be chosen as therapeutic targets for suppressing the stemness of CSCs in GC patients. Nevertheless, further experimental studies are still required to validate our findings.
Acknowledgments
The authors would like to express their gratitude to Editsprings (www.editsprings.com) for the expert linguistics services provided.
Funding: This study was supported by
Footnote
Conflicts of Interest: Both authors have completed the ICMJE uniform disclosure form (available at http://dx.doi.org/10.21037/tcr-20-704). XX and YL report grants from National Natural Science Foundation of China, grants from Hunan Provincial Natural Science Foundation, grants from Hunan Provincial Health Department Project, grants from Hunan Administration Traditional Chinese Medicine Foundation, outside the submitted work.
Ethical Statement: The authors are accountable for all aspects of the work in ensuring that questions related to the accuracy or integrity of any part of the work are appropriately investigated and resolved. The study was based on the TCGA Research Network: https://www.cancer.gov/tcga, thus, ethical approval was not needed. The study was conducted in accordance with the Declaration of Helsinki (as revised in 2013).
Open Access Statement: This is an Open Access article distributed in accordance with the Creative Commons Attribution-NonCommercial-NoDerivs 4.0 International License (CC BY-NC-ND 4.0), which permits the non-commercial replication and distribution of the article with the strict proviso that no changes or edits are made and the original work is properly cited (including links to both the formal publication through the relevant DOI and the license). See: https://creativecommons.org/licenses/by-nc-nd/4.0/.
References
- Global Burden of Disease Cancer Collaboration. Global, Regional, and National Cancer Incidence, Mortality, Years of Life Lost, Years Lived With Disability, and Disability-Adjusted Life-Years for 29 Cancer Groups, 1990 to 2017: A Systematic Analysis for the Global Burden of Disease Study. JAMA Oncol 2019;5:1749-68. [Crossref] [PubMed]
- Coutzac C, Pernot S, Chaput N, et al. Immunotherapy in advanced gastric cancer, is it the future? Crit Rev Oncol Hematol 2019;133:25-32. [Crossref] [PubMed]
- Lordick F, Smyth EC. Two steps forward and one step back. Nat Rev Clin Oncol 2019;16:69-70. [Crossref] [PubMed]
- Clarke MF. Clinical and Therapeutic Implications of Cancer Stem Cells. N Engl J Med 2019;380:2237-45. [Crossref] [PubMed]
- Marzagalli M, Raimondi M, Fontana F, et al. Cellular and molecular biology of cancer stem cells in melanoma: Possible therapeutic implications. Semin Cancer Biol 2019;59:221-35. [Crossref] [PubMed]
- Sharifzad F, Ghavami S, Verdi J, et al. Glioblastoma cancer stem cell biology: Potential theranostic targets. Drug Resist Updat 2019;42:35-45. [Crossref] [PubMed]
- Steinbichler TB, Savic D, Dudas J, et al. Cancer stem cells and their unique role in metastatic spread. Semin Cancer Biol 2020;60:148-56. [Crossref] [PubMed]
- Malta TM, Sokolov A, Gentles AJ, et al. Machine Learning Identifies Stemness Features Associated with Oncogenic Dedifferentiation. Cell 2018;173:338-54.e15. [Crossref] [PubMed]
- Linehan WM, Ricketts CJ. The Cancer Genome Atlas of renal cell carcinoma: findings and clinical implications. Nat Rev Urol 2019;16:539-52. [Crossref] [PubMed]
- Langfelder P, Horvath S. WGCNA: an R package for weighted correlation network analysis. BMC Bioinformatics 2008;9:559. [Crossref] [PubMed]
- Deffur A, Wilkinson RJ, Coussens AK. Tricks to translating TB transcriptomics. Ann Transl Med 2015;3:S43. [PubMed]
- Bai KH, He SY, Shu LL, et al. Identification of cancer stem cell characteristics in liver hepatocellular carcinoma by WGCNA analysis of transcriptome stemness index. Cancer Med 2020;9:4290-98. [Crossref] [PubMed]
- Pan S, Zhan Y, Chen X, et al. Identification of Biomarkers for Controlling Cancer Stem Cell Characteristics in Bladder Cancer by Network Analysis of Transcriptome Data Stemness Indices. Front Oncol 2019;9:613. [Crossref] [PubMed]
- Zhang Y, Tseng JT, Lien IC, et al. mRNAsi Index: Machine Learning in Mining Lung Adenocarcinoma Stem Cell Biomarkers. Genes (Basel) 2020;11:257. [Crossref] [PubMed]
- Robertson AG, Kim J, Al-Ahmadie H, et al. Comprehensive Molecular Characterization of Muscle-Invasive Bladder Cancer. Cell 2017;171:540-56 e25.
- Siemeister G, Mengel A, Fernandez-Montalvan AE, et al. Inhibition of BUB1 Kinase by BAY 1816032 Sensitizes Tumor Cells toward Taxanes, ATR, and PARP Inhibitors In Vitro and In Vivo. Clin Cancer Res 2019;25:1404-14. [Crossref] [PubMed]
- Fu X, Chen G, Cai ZD, et al. Overexpression of BUB1B contributes to progression of prostate cancer and predicts poor outcome in patients with prostate cancer. Onco Targets Ther 2016;9:2211-20. [PubMed]
- Yang Z, Li C, Yan C, et al. KIF14 promotes tumor progression and metastasis and is an independent predictor of poor prognosis in human gastric cancer. Biochim Biophys Acta Mol Basis Dis 2019;1865:181-92. [Crossref] [PubMed]
- Yin L, Jiang LP, Shen QS, et al. NCAPH plays important roles in human colon cancer. Cell Death Dis 2017;8:e2680. [Crossref] [PubMed]
- Wu PH, Onodera Y, Recuenco FC, et al. Lambda-Carrageenan Enhances the Effects of Radiation Therapy in Cancer Treatment by Suppressing Cancer Cell Invasion and Metastasis through Racgap1 Inhibition. Cancers (Basel) 2019;11:1192. [Crossref] [PubMed]
- Wang J, Guo X, Xie C, et al. KIF15 promotes pancreatic cancer proliferation via the MEK-ERK signalling pathway. Br J Cancer 2017;117:245-55. [Crossref] [PubMed]
- Chen EB, Qin X, Peng K, et al. HnRNPR-CCNB1/CENPF axis contributes to gastric cancer proliferation and metastasis. Aging (Albany NY) 2019;11:7473-91. [Crossref] [PubMed]
- Shahid M, Kim M, Lee MY, et al. Downregulation of CENPF Remodels Prostate Cancer Cells and Alters Cellular Metabolism. Proteomics 2019;19:e1900038. [Crossref] [PubMed]
- Huang DH, Jian J, Li S, et al. TPX2 silencing exerts antitumor effects on hepatocellular carcinoma by regulating the PI3K/AKT signaling pathway. Int J Mol Med 2019;44:2113-22. [PubMed]
- Yang Y, Li DP, Shen N, et al. TPX2 promotes migration and invasion of human breast cancer cells. Asian Pac J Trop Med 2015;8:1064-70. [Crossref] [PubMed]
- Zou J, Huang RY, Jiang FN, et al. Overexpression of TPX2 is associated with progression and prognosis of prostate cancer. Oncol Lett 2018;16:2823-32. [PubMed]
- Li Q, Xie W, Wang N, et al. CDC7-dependent transcriptional regulation of RAD54L is essential for tumorigenicity and radio-resistance of glioblastoma. Transl Oncol 2018;11:300-6. [Crossref] [PubMed]
- Yan H, Zhu C, Zhang L. Kinesin family member 18B: A contributor and facilitator in the proliferation and metastasis of cutaneous melanoma. J Biochem Mol Toxicol 2019;33:e22409. [Crossref] [PubMed]
- Wu Y, Wang A, Zhu B, et al. KIF18B promotes tumor progression through activating the Wnt/beta-catenin pathway in cervical cancer. Onco Targets Ther 2018;11:1707-20. [Crossref] [PubMed]
- Chen S, Wang J, Wang L, et al. Silencing TTK expression inhibits the proliferation and progression of prostate cancer. Exp Cell Res 2019;385:111669. [Crossref] [PubMed]
- Hou G, Dong C, Dong Z, et al. Upregulate KIF4A Enhances Proliferation, Invasion of Hepatocellular Carcinoma and Indicates poor prognosis Across Human Cancer Types. Sci Rep 2017;7:4148. [Crossref] [PubMed]
- Hu G, Yan Z, Zhang C, et al. FOXM1 promotes hepatocellular carcinoma progression by regulating KIF4A expression. J Exp Clin Cancer Res 2019;38:188. [Crossref] [PubMed]
- Gantchev J, Martinez Villarreal A, Xie P, et al. The Ectopic Expression of Meiosis Regulatory Genes in Cutaneous T-Cell Lymphomas (CTCL). Front Oncol 2019;9:429. [Crossref] [PubMed]
- Kazazian K, Go C, Wu H, et al. Plk4 Promotes Cancer Invasion and Metastasis through Arp2/3 Complex Regulation of the Actin Cytoskeleton. Cancer Res 2017;77:434-47. [Crossref] [PubMed]
- Zhao Y, Wang X. PLK4: a promising target for cancer therapy. J Cancer Res Clin Oncol 2019;145:2413-22. [Crossref] [PubMed]
- Dai B, Zhang X, Shang R, et al. Blockade of ARHGAP11A reverses malignant progress via inactivating Rac1B in hepatocellular carcinoma. Cell Commun Signal 2018;16:99. [Crossref] [PubMed]
- Sarathi A, Palaniappan A. Novel significant stage-specific differentially expressed genes in hepatocellular carcinoma. BMC Cancer 2019;19:663. [Crossref] [PubMed]
- van Dam S, Cordeiro R, Craig T, et al. GeneFriends: an online co-expression analysis tool to identify novel gene targets for aging and complex diseases. BMC Genomics 2012;13:535. [Crossref] [PubMed]
- Arai T, Okato A, Yamada Y, et al. Regulation of NCAPG by miR-99a-3p (passenger strand) inhibits cancer cell aggressiveness and is involved in CRPC. Cancer Med 2018;7:1988-2002. [Crossref] [PubMed]
- Gavin EJ, Song B, Wang Y, et al. Reduction of Orc6 expression sensitizes human colon cancer cells to 5-fluorouracil and cisplatin. PLoS One 2008;3:e4054. [Crossref] [PubMed]
- Han JY, Han YK, Park GY, et al. Corrigendum: Bub1 is required for maintaining cancer stem cells in breast cancer cell lines. Sci Rep 2016;6:33106. [Crossref] [PubMed]
- Ding Y, Hubert CG, Herman J, et al. Cancer-Specific requirement for BUB1B/BUBR1 in human brain tumor isolates and genetically transformed cells. Cancer Discov 2013;3:198-211. [Crossref] [PubMed]
- Huang C, Han Z, Wu D. Effects of TPX2 gene on radiotherapy sensitization in breast cancer stem cells. Oncol Lett 2017;14:1531-5. [Crossref] [PubMed]
- Zhan Y, Chen Z, He S, et al. Long non-coding RNA SOX2OT promotes the stemness phenotype of bladder cancer cells by modulating SOX2. Mol Cancer 2020;19:25. [Crossref] [PubMed]
- Luo H, Zhu G, Xu J, et al. HOTTIP lncRNA Promotes Hematopoietic Stem Cell Self-Renewal Leading to AML-like Disease in Mice. Cancer Cell 2019;36:645-659.e8. [Crossref] [PubMed]
- Shao M, Yang Q, Zhu W, et al. LncHOXA10 drives liver TICs self-renewal and tumorigenesis via HOXA10 transcription activation. Mol Cancer 2018;17:173. [Crossref] [PubMed]
- Wang Y, Zhu P, Luo J, et al. LncRNA HAND2-AS1 promotes liver cancer stem cell self-renewal via BMP signaling. EMBO J 2019;38:e101110. [Crossref] [PubMed]
- Wu J, Zhu P, Lu T, et al. The long non-coding RNA LncHDAC2 drives the self-renewal of liver cancer stem cells via activation of Hedgehog signaling. J Hepatol 2019;70:918-29. [Crossref] [PubMed]