Correlation analysis between metabolic tumor burden measured by positron emission tomography/computed tomography and the 2015 World Health Organization classification of lung adenocarcinoma, with a risk prediction model of tumor spread through air spaces
Introduction
The phenomenon of “spread through air spaces” (STAS), which was first identified by Kadota et al. (1), is defined as the spread of lung cancer tumor cells into the air spaces of the lung parenchyma adjacent to the main tumor. Kadota et al. (1) reported that the presence of STAS is a significant risk factor associated with the recurrence of small lung adenocarcinomas treated with limited resection. Lymphovascular invasion and high-grade morphologic pattern in main tumors were more frequently identified in STAS-positive tumors than in STAS-negative tumors; in contrast, the lepidic pattern was less frequently identified in STAS-positive tumors than in STAS negative tumors (1). These findings support their proposal that STAS should be formally recognized as a pattern of invasion of lung adenocarcinoma.
Morales-Oyarvide et al. (2) stated that since the publication of the International Lung Cancer Research Association, the American Thoracic Society and the European Respiratory Society (IASLC/ATS/ERS) classification, only a few new morphologic features, which appear to be prognostically important, have been identified. Cribriform pattern, tumor islands, and STAS are such examples (3,4). Tumor islands and STAS have been reported to have strong prognostic implications for recurrence and patient survival, especially among small early-stage tumors undergoing limited resection (e.g., wedge resection, segmentectomy) (1,3) and are now considered to be patterns of air space invasion (5). Warth et al. (6) proposed that the presence of STAS was intimately linked with specific growth patterns. STAS was significantly more prevalent in high-stage, nodal-positive adenocarcinoma with distant metastasis. Warth et al. (6) also posited that STAS was significantly associated with reduced overall and disease-free survival, which was growth pattern but not stage independently.
The World Health Organization (WHO) classification of lung adenocarcinoma in 2015 mainly emphasizes the significance of prognostic stratification. That is to say, it divides lung adenocarcinoma into pre-invasion lesions (AAH and AIS), microinvasive adenocarcinoma and invasive adenocarcinoma. The diagnosis of AIS and microinvasive adenocarcinoma mainly focused on single lesion with or within 3 cm in diameter; without vascular tumor thrombus, nerve invasion, pleural invasion or tumor necrosis. For invasive adenocarcinoma, it can be divided into five categories, including lepidic, papillary, acinar, solid with mucus secretion and micropapillary types. They also have different prognostic significance. The prognosis of lepidic is most favorable, solid type and micropapillary are the worst, while papillary and acinar adenocarcinoma are in the middle. In addition, a special type of invasion, STAS, is defined as the presence of free tumor cell clusters in the normal alveolar cavity around the tumor body (>3 alveolar spaces), which can exist in single, clusters, or micropapillary in the alveolar space (5).
Recently, positron emission tomography/computed tomography (PET/CT) with 2-deoxy-2-[18F]fluoro-D-glucose ([18F]FDG) has been widely used for tumor diagnosis, staging, prognosis prediction, and treatment evaluation. Several studies have already proved the prognostic and predictive value of maximum standard uptake value (SUVmax), metabolic tumor volume, and total lesion glycolysis in patients with non-small-cell lung carcinoma (7,8). Besides, tumor FDG uptake before surgery is strongly correlated with prognosis and may aid post-surgical treatment planning (9,10). There possible correlations between STAS and PET/CT metabolic tumor burden are unknown, as is the predictive value of PET/CT for early-stage tumor recurrence before surgery. This study aimed to assess the correlation between metabolic tumor burden measured by PET/CT and the 2015 WHO classification of lung adenocarcinoma, and to establish a risk prediction model of STAS in patients with stage I lung adenocarcinoma.
We present the following article in accordance with the STROBE reporting checklist (available at http://dx.doi.org/10.21037/tcr-20-1934).
Methods
Participants
The study was conducted in accordance with the Declaration of Helsinki (as revised in 2013). The study was approved by National Cancer Center’s Institutional Review Board (No. NCC2017G-060). The institutional review board of our hospital approved this retrospective study and waived the need for informed consent. Between June 2005 and June 2012, a total of 8,464 patients were pathologically diagnosed with primary malignant lung tumors at our hospital, including 4,612 adenocarcinomas, of which 851 patients had stage I adenocarcinomas. Finally, 127 patients with stage I adenocarcinoma with preoperative [18F]FDG PET/CT scans were enrolled in our study. The electronic medical records of all patients, including age, sex, surgical approach, histological grade, TNM staging, and clinical staging were reviewed. The distribution and nodule density of the tumor was determined by PET/CT imaging. A non-solid nodule was defined as a ground-glass opacity on computed tomography, which appears as a hazy area of increased opacity in the lung, with intact bronchial and vascular margins. A nodule with mixed solid and non-solid components was defined as a part-solid nodule. The lesions were divided into 3 types, according to the nodule density: non-solid, part-solid solid, and solid. Image analysis was performed independently by 3 blinded radiologists with 30, 17, and 14 years’ experience in radiology. Disagreements were resolved through discussion.
Pathology review
All Patients with a lung adenocarcinoma that had undergone complete anatomic resection, diagnosed with pT1a-T2aN0M0 consecutively, were enrolled. All specimens were reviewed according to the new 2015 WHO classification by an experienced pathologist with 15 years of working experience. The growth patterns were divided into five categories and three major grades: (I) low grade: lepidic predominant adenocarcinoma (LPA); (II) moderate grade: acinar predominant adenocarcinoma (APA), papillary predominant adenocarcinoma (PPA); (III) high grade: micropapillary predominant adenocarcinoma (MPPA), solid predominant adenocarcinoma (SPA). The phenomena of STAS were reviewed simultaneously. The correlations of clinical and pathological factors with 5-year progress-free survival (PFS) were evaluated.
All the 127 patients went through pathologic review and recorded by the predominant subtypes according to the 2015 WHO classification of lung cancer (11), among which 12 cases were excluded for not enough number for statistical analysis [1 case of minimally invasive adenocarcinoma (MIA), 3 MPPA and 3 mucinous adenocarcinomas] or not meeting the including criteria (2 cases of primarily diagnosed adenocarcinomas were revised as atypical neuroendocrine carcinoma, 2 cases were revised as with pleural metastasis, and 1 was not reviewed due to lack of archived slides), finally 115 cases were left for statistical analysis, including 58 APA, 24 LPA, 22 PPA, and 11 SPA.
On the other hand, total 121 cases went through STAS review, excluding 6 cases (2 cases of primarily diagnosed adenocarcinomas were revised as atypical neuroendocrine carcinoma, 2 cases were revised as with pleural metastasis, 1 was not reviewed due to lack of archived slides, and 1 case of MIA without STAS).
[18F]FDG PET/CT study
All patients underwent scanning with the same integrated PET/CT (Discovery ST, GE Healthcare) machine. Each patient’s serum glucose was maintained at normal level (120–200 mg/dL) before the scan. Each patient received 3.70–4.44 MBq/kg of [18F]FDG intravenously and then underwent whole-body three-dimensional PET/CT after 60–70 minutes. The PET images with a slice thickness of 3.27 mm were obtained with 3 minutes acquisition per bed. Scanning from the vertex of the skull to the upper-thigh required an acquisition time of 15–18 minutes. All PET images were reconstructed with an iterative algorithm (ordered-subset expectation maximization) for CT-based attenuation correction (12). The scan parameters for spiral CT were as follows: 120 kV tube voltage, 150 mA tube current, 3.75 mm slice thickness, 3.75 mm slice interval, and 0.8 s per rotation. Breath-hold chest CT was performed with a tube voltage of 120 kV, tube current of 205 mA, slice thickness of 5 mm/1.25 mm, with 5 mm/0.8 mm intervals, and 0.8 s per rotation.
Automated PET delineation methods and CT delineation method
Two PET delineation methods were used, according to the type of nodule (12).
Adaptive target volume delineation by PET Volume Computerized Assisted Reporting (PETVCAR, GE Healthcare) was used for solid lesions. The PET and CT co-registrations were assessed, once the images were loaded into the PETVCAR software. The primary lung cancer PET grayscale and PET/CT fused images were subsequently reviewed in the axial, sagittal, and coronal planes (13). A boundary box was placed on the image, which was used to auto-contour and segment the region of interest (ROI). A radiologist reviewed and adjusted the box to ensure that this three-dimensional cube contained all the [18F]FDG PET-positive areas and excluded the negative normal tissue. This process was repeated several times, until each [18F]FDG PET/CT-positive region had been optimized. The lesion metabolic volume was automatically calculated using an adaptive iterative algorithm in PETVCAR, which separated the target volume from the background tissue, by weighting the SUVmax and the SUVmean within the target volume with a weighting factor, represented as a Boolean variable (14) (Figure 1A).
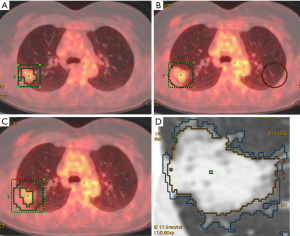
An adaptive threshold of 40% SUVmax (15) was used for non-solid and part-solid lesions, which adapts the threshold value within the selected volume of interest (VOI) relative to mean background (BG) SUV, by calculating the T value as thresholding: T =0.4 (SUVmax − BG) +BG. This delineation method requires information on BG uptake. Since the BG of the lung is heterogeneous, mean BG SUV shows discrepancies at different regions (such as the apex, and central and peripheral regions). The radiologist copied the ROI and pasted it on the same location, at the contralateral lung (Figure 1B,C).
The computed tomography volume was measured using the Lung Volume Computerized Assisted Reporting software (Lung VCAR, GE Healthcare) with the 1.25-mm slice thickness images. Lung VCAR, which uses GE’s Volume Viewer software, is an image analysis software package for Advantage Workstation systems (12). The analysis mode was used which offers a combination of two-dimensional reformatted views, with corresponding volume rendering views centering on the bookmarked spot. In this mode, the software can zoom on the VOI, automatically calculate the volume of the suspected spot and display the calculated volume on the view. Moreover, it displays the consistency of the detected nodules, depending on the protocol chosen. The actual volume measurement is performed with an automatic nodule sizing algorithm. This metric can be used to assess the evolution of a nodule’s volume by repeating the measurements several times (Figure 1D).
Statistical analysis
Continuous data were expressed as mean ± SD, and categorical data were presented as frequencies. Continuous data analysis was performed with the independent t-test for parametric variables. Multiple groups were compared using analysis of variance and pairwise comparisons were performed with the least significant difference method. Nonparametric variables analysis was performed with the Mann-Whitney U test. Categorical data were calculated with Pearson’s Chi-squared test.
Five-year progression-free survival (PFS) was compared with the Kaplan-Meier method and the Cox proportional-hazard model. The cut-off point of each continuous parameter was determined through the receiver operating characteristic curve (ROC) and Youden index, using PFS as the classification status. The Kaplan-Meier was used for univariate analysis. The Cox proportional-hazard model was used for multivariate analysis.
All risk factors were analyzed by multivariate logistic regression analysis; regression coefficients and odds ratios were calculated for the independent risk factors. A STAS risk prediction model was created using the regression coefficients to determine the predictive probability (PP).
P<0.05 was considered statistically significant. The Statistical Package for the Social Sciences 13.0 was used for statistical analysis (SPSS version 13.0, SPSS, Chicago, IL, USA).
Results
A total of 127 patients were evaluated (mean age 60 years; 60 males, 67 females). The diameter of 52 lesions are smaller than 2.0 cm (41%), 50 lesions are 2.0–3.0 cm (39%) and 25 lesions are larger than 3.0 cm (20%). The median followed-up time was 5 years (60 months). At the end of the follow-up period, 96 patients (76%) were alive (mean PFS time of 58.7±23.9 months, the median of 60 months) and 31 patients (24%) had a recurrence (mean PFS time of 21.6±10.5 months, the median of 22 months). Recurrence was reported as follows: 4 cases of local recurrence (bronchial stump), 11 cases of regional recurrence (3 pleural seeding, 2 mediastinal lymph node and 6 lung metastasis), 12 cases of distant recurrence (3 liver, 5 brain and 4 bone), and 4 cases with multi-metastasis (4 pleural, 3 lymph node, 1 lung and 1 bone simultaneously).
Survival analysis
In the Kaplan-Meier univariate analysis, 2015 WHO classification was significantly correlated with PFS (P=0.000, χ2=18.129). LPA was correlated with better survival than APA (P=0.026, χ2=4.959), but without statistical significance with PPA (P=0.285, χ2=1.144). The prognostic of SPA was worse than the others (all P<0.05) (Figure 2). In short, the high pathological grade was correlated with shorter PFS.
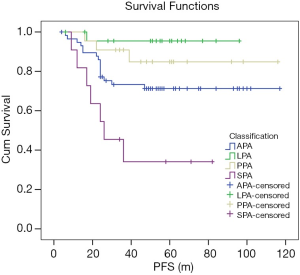
The nodule types were significantly correlated with 2015 WHO pathologic categories (P=0.000, χ2=32.067) (Figure 3, Table 1). Table 1 demonstrated that in LPA with good prognosis, the occurrence of non-solid nodule plus part-solid nodule were more than that of solid type (83.8% vs. 16.7%); in PPA and APA with moderate prognosis, the occurrence of solid type was gradually increased; in SPA with poor prognosis, the occurrence of solid type was of the great majority (100%). In these cases (4/24) which LPA appeared as a solid nodule, large amount of fibrosis and congestion were seen under the microscope (Figure 4).
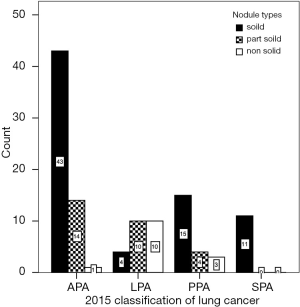
Table 1
Nodule type | LPA (n=24) | PPA (n=22) | APA (n=58) | SPA (n=11) |
---|---|---|---|---|
NSN+PSN | 83.3% | 31.8% | 25.9% | 0 |
SN | 16.7% | 68.2% | 74.1% | 100% |
NSN, non-solid nodule; PSN, part-solid nodule; SN, solid nodule; APA, acinar predominant adenocarcinoma; LPA, lepidic predominant adenocarcinoma; PPA, papillary predominant adenocarcinoma; SPA, solid predominant adenocarcinoma; WHO, World Health Organization.
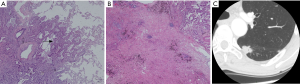
The 2015 WHO classification were significantly correlated with SUVmax (P=0.001, t=6.252), SUVmean (P=0.001, t=5.754), SUVpeak (P=0.001, t=5.490), TLG (P=0.027, t=3.177), and CTV (P=0.029, t=3.108), but not with MTV (P=0.105, t=2.093) and diameter (P=0.168, t=1.715). LPA had the lowest SUVmax and TLG comparing with APA, PPA, and SPA (P-SUV=0.000, 0.002 and 0.002; P-TLG=0.006, 0.011 and 0.057). The prediction incidence of LPA was analyzed using the ROC curve (AUC =0.859, P=0.000), the cut off point of SUVmax was 1.235. The sensitivity, specificity, and accuracy of the prediction of SUVmax were 75.0%, 90.1%, and 87.0%, respectively, higher than TLG and CTV.
Tumor STAS
In the 121 cases with STAS review (Figure 5), STAS was positive in 51 cases, negative in 70 cases (Figure 6A). In the 70 negative cases, there were 36 solid nodules, 20 part-solid nodules and 14 non-solid nodules. In the 51 positive cases, there were 42 solid nodules, 9 part-solid nodules and 0 non-solid nodules. There were significant differences in STAS distribution among different nodule types (P=0.000). In the 51 positive cases, 7 cases were with STAS ≤5, 44 cases were with STAS >5, and STAS >5 was more common in solid nodule type (Figure 6B). All of the non-solid nodules were without STAS. In the 29 recurrences, STAS was positive in only 8.3% LPA cases, far less than in APA (44.8%), PPA (59.1%), SPA (63.6%), and MPPA (66.6%) (Figure 6C).
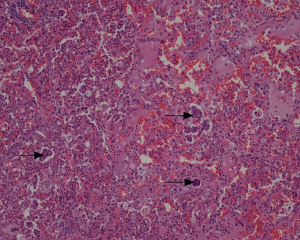
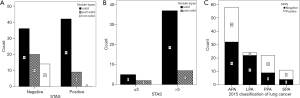
STAS was significantly correlated with SUVmax (P=0.000, t=−4.241), SUVmean (P=0.000, t=−4.441), SUVpeak (P=0.000, t=−4.296), TLG (P=0.001, t=−3.264) and diameter (P=0.044, t=−2.035), but not with MTV (P=0.064, t=−1.875) and CTV (P=0.105, t=−1.634). The prediction incidence of STAS was analyzed using the ROC curve, the cut off point of SUVmax was 2.795 (AUC =0.729, P=0.000), the cut off point of TLG was 9750 (AUC =0.711, P=0.000). The sensitivity, specificity, and accuracy of the prediction of SUVmax were 66.7%, 72.9%, and 70.2%, respectively; of TLG were 54.9%, 78.6%, and 68.6%, respectively.
Risk prediction model
The risk prediction model of STAS used the following regression analysis formula:
Note: PP, predictive probability; A, MTV; B, SUVmax; C, SUVmean; D, TLG; E, SUVpeak; F, Nodule types; G, long diameter; H, short diameter; I, CTV.
The PP of STAS and the incidence of STAS were analyzed using the ROC curve (Figure 7) (AUC =0.759, P=0.000). The cut-off point of PP was 0.553. The sensitivity, specificity, and accuracy of the predictive model for STAS were 47.1%, 88.6%, and 71.1%, respectively.
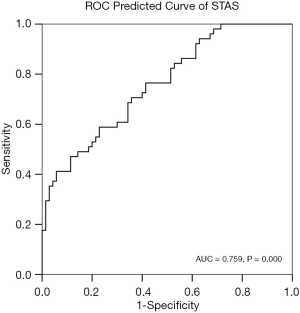
Discussion
Despite the favorable prognosis of stage IA NSCLC, the disease recurs after complete surgical resection in 20–30% of patients (9). It may correlate to the high heterogeneity of lung cancer. STAS has been reported having strong prognostic implications for recurrence and patient survival. Shiono et al. (16) reviewed 318 stage I lungs adenocarcinomas, investigated the characteristics of STAS and analyzed the relationship between STAS and prognosis. They proposed that STAS is a prognosticator related to local recurrence. Kang et al. (17) considered that FDG uptake and STAS are significant prognostic markers in stage I NSCLC adenocarcinoma treated with surgical resection. Yang et al. (18) considered that STAS predicts a worse survival in patients with stage I adenocarcinomas >2 cm after radical lobectomy. Therefore, if we can predict the possibility of STAS before the surgery, it would be very helpful to those patients who should undergo lobectomy surgery instead of limited resection, and who may be potential candidates for postoperative adjuvant chemotherapy.
However, up to now, there were no possible imaging examination methods to achieve this purpose. To our knowledge, we are the first one reporting the correlation between the phenomenon of STAS and FDG metabolic tumor burden by PET/CT, besides, we established a risk prediction model of STAS using logistic regression analysis.
In our study, different predominant histological subtype was found correlated with different prognosis (LPA favors for a good prognosis, while APA and PPA for moderate one, SPA and MPPA for poor prognosis), which was consistent with the previous reports (19). In the correlation analysis of STAS and pathological categories and nodule types, LPA was usually showed as subsolid nodules without STAS. We assume that it is according to the pathological basis of lung tumor cells growth patterns with lepidic growing, monolayer tumor cells arranged neatly, with a clear pole. However, in several cases, LPA with a large amount of fibrosis or congestion might appear as solid nodule. In our study, no STAS was seen in non-solid nodules, but more common in solid nodules; STAS was positive in only 8.3% LPA cases, far less than in APA (44.8%), PPA (59.1%), SPA (63.6%), and MPPA (66.6%). Therefore, we considered it is safety that the limited resection performed in non-solid nodules (pure ground glass nodule) without STAS.
An interesting coexisting phenomenon was found along with STAS, which was pulmonary congestion and/or alveolar edema and expansion at the edge of the main tumor, and the STAS was normally observed in the congestion background, whether it is correlated with PET/CT features needs to be further investigated.
Our result showed that SUV and TLG were significantly correlated with STAS and pathological histology major subtype. In most cases, LPA is along with low FDG uptake and with a low incidence of STAS; while SPA is along with high FDG uptake and with a high incidence of STAS. The reason for this might be as follows: firstly, the FDG uptake will be higher in the lesions which contain more active and poor differentiated tumor cells such as MPPA and SPA. Secondly, malignant tumors are more aggressive and gain greater malignant potential during growth, i.e. evolution, which may lead to more FDG uptake. Moreover, Kadota et al. (20) reported that the histological grade was positively correlated with the SUVmax in stage I lung adenocarcinoma developed by IASLC/ATS/ERS.
In our study, nine risk factors, including SUVmax, SUVmean, SUVpeak, MTV, TLG, nodule type, long diameter, short diameter, and CTV, were used for the STAS risk prediction model. This model integrates multiple risk factors to determine the probability of STAS. The nine risk factors were determined through our previous study (13). In univariate analysis of our previous studies, the histological grade, nodule type, diameter, CTV, SUV, MTV, and TLG were significantly associated with 5-year PFS (all P value <0.05) (13). Although no prediction method can ever reach 100% accuracy, this model predicts STAS with a high degree of accuracy (AUC =0.759). Furthermore, the prediction model proposed in our study has not been previously reported.
The significance of the risk prediction model of STAS is as following. Firstly, it’s the first time to establish the correlations between STAS and PET/CT metabolic tumor burden. Secondly, it would be very helpful to those patients who should undergo lobectomy surgery instead of limited resection and who may be potential candidates for postoperative adjuvant chemotherapy. Thirdly, it’s a non-invasively method for STAS predicting, which can help patients who cannot obtain surgical pathology.
This article is a preliminary study with small sample size. The limitations of this study exist in that it is a retrospective study with intrinsic biases, and the enrolled sample was not big enough for cross-validation. The insufficient numbers of non-solid and part-solid nodules and the heterogeneous distribution in three nodule types maybe cause some biases. A large scale prospective study is necessary for external validation for the predictive model.
Clinical practice points
The LPA appeared as non-solid nodule with low SUV without STAS has a good prognosis.
STAS is an important pattern of invasion and impacts the frequency and location of recurrence. SUV and TLG are valuable predictive indices in the prediction of STAS.
Conclusions
The LPA is more likely to appear as a subsolid nodule with low SUV, without STAS, and has a good prognosis. SUV is significantly correlated with 2015 WHO classification of lung adenocarcinoma. SUV and TLG are valuable predictive indices in the prediction of STAS. This risk prediction model has good specificity and accuracy for predicting the incidence of STAS.
Acknowledgments
A huge number of people helped to investigate and analyze the patients in the past few years. We wish to express our gratitude to all of them. Amongst them are Dr. Rong Zheng, Dr. Wen-Jie Zhang, Dr. Xiao-Meng Li, Dr. Yan Fang, Dr. Lv Lv, Dr. Jian-Hua Geng, technician Shao-Meng Du and all staffs of PET-CT center.
Funding: This study was funded by
Footnote
Reporting Checklist: The authors have completed the STROBE reporting checklist. Available at http://dx.doi.org/10.21037/tcr-20-1934
Data Sharing Statement: Available at http://dx.doi.org/10.21037/tcr-20-1934
Conflicts of Interest: All authors have completed the ICMJE uniform disclosure form (available at http://dx.doi.org/10.21037/tcr-20-1934). The authors have no conflicts of interest to declare.
Ethical Statement: The authors are accountable for all aspects of the work in ensuring that questions related to the accuracy or integrity of any part of the work are appropriately investigated and resolved. The study was conducted in accordance with the Declaration of Helsinki (as revised in 2013). The study was approved by National Cancer Center’s Institutional Review Board (No. NCC2017G-060). The institutional review board of our hospital approved this retrospective study and waived the need for informed consent.
Open Access Statement: This is an Open Access article distributed in accordance with the Creative Commons Attribution-NonCommercial-NoDerivs 4.0 International License (CC BY-NC-ND 4.0), which permits the non-commercial replication and distribution of the article with the strict proviso that no changes or edits are made and the original work is properly cited (including links to both the formal publication through the relevant DOI and the license). See: https://creativecommons.org/licenses/by-nc-nd/4.0/.
References
- Kadota K, Nitadori J, Sima CS, et al. Tumor Spread through Air Spaces is an Important Pattern of Invasion and Impacts the Frequency and Location of Recurrences after Limited Resection for Small Stage I Lung Adenocarcinomas. J Thorac Oncol 2015;10:806-14. [Crossref] [PubMed]
- Morales-Oyarvide V, Mino-Kenudson M. Tumor islands and spread through air spaces: Distinct patterns of invasion in lung adenocarcinoma. Pathol Int 2016;66:1-7. [Crossref] [PubMed]
- Onozato ML, Kovach AE, Yeap BY, et al. Tumor islands in resected early-stage lung adenocarcinomas are associated with unique clinicopathologic and molecular characteristics and worse prognosis. Am J Surg Pathol 2013;37:287-94. [Crossref] [PubMed]
- Kadota K, Yeh YC, Sima CS, et al. The cribriform pattern identifies a subset of acinar predominant tumors with poor prognosis in patients with stage I lung adenocarcinoma: a conceptual proposal to classify cribriform predominant tumors as a distinct histologic subtype. Mod Pathol 2014;27:690-700. [Crossref] [PubMed]
- Travis WD, Brambilla E, Nicholson AG, et al. The 2015 World Health Organization Classification of Lung Tumors: Impact of Genetic, Clinical and Radiologic Advances Since the 2004 Classification. J Thorac Oncol 2015;10:1243-60. [Crossref] [PubMed]
- Warth A, Muley T, Kossakowski CA, et al. Prognostic Impact of Intra-alveolar Tumor Spread in Pulmonary Adenocarcinoma. Am J Surg Pathol 2015;39:793-801. [Crossref] [PubMed]
- Hyun SH, Ahn HK, Kim H, et al. Volume-based assessment by (18)F-FDG PET/CT predicts survival in patients with stage III non-small-cell lung cancer. Eur J Nucl Med Mol Imaging 2014;41:50-8. [Crossref] [PubMed]
- Liao S, Penney BC, Wroblewski K, et al. Prognostic value of metabolic tumor burden on 18F-FDG PET in nonsurgical patients with non-small cell lung cancer. Eur J Nucl Med Mol Imaging 2012;39:27-38. [Crossref] [PubMed]
- Park SY, Cho A, Yu WS, et al. Prognostic value of total lesion glycolysis by 18F-FDG PET/CT in surgically resected stage IA non-small cell lung cancer. J Nucl Med 2015;56:45-9. [Crossref] [PubMed]
- Domachevsky L, Groshar D, Galili R, et al. Survival Prognostic Value of Morphological and Metabolic variables in Patients with Stage I and II Non-Small Cell Lung Cancer. Eur Radiol 2015;25:3361-7. [Crossref] [PubMed]
- Zugazagoitia J, Enguita AB, Nunez JA, et al. The new IASLC/ATS/ERS lung adenocarcinoma classification from a clinical perspective: current concepts and future prospects. J Thorac Dis 2014;6:S526-36. [PubMed]
- Wang XY, Zhao YF, Liu Y, et al. Comparison of different automated lesion delineation methods for metabolic tumor volume of 18F-FDG PET/CT in patients with stage I lung adenocarcinoma. Medicine (Baltimore) 2017;96:e9365. [Crossref] [PubMed]
- Wang XY, Zhao YF, Liu Y, et al. Prognostic value of metabolic variables of [18F]FDG PET/CT in surgically resected stage I lung adenocarcinoma. Medicine (Baltimore) 2017;96:e7941. [Crossref] [PubMed]
- Moule RN, Kayani I, Prior T, et al. Adaptive 18fluoro-2-deoxyglucose positron emission tomography/computed tomography-based target volume delineation in radiotherapy planning of head and neck cancer. Clin Oncol (R Coll Radiol) 2011;23:364-71. [Crossref] [PubMed]
- Firouzian A, Kelly MD, Declerck JM. Insight on automated lesion delineation methods for PET data. EJNMMI Res 2014;4:69. [Crossref] [PubMed]
- Shiono S, Yanagawa N. Spread through air spaces is a predictive factor of recurrence and a prognostic factor in stage I lung adenocarcinoma. Interact Cardiovasc Thorac Surg 2016;23:567-72. [Crossref] [PubMed]
- Kang YK, Song YS, Cho S, et al. Prognostic stratification model for patients with stage I non-small cell lung cancer adenocarcinoma treated with surgical resection without adjuvant therapies using metabolic features measured on F-18 FDG PET and postoperative pathologic factors. Lung Cancer 2018;119:1-6. [Crossref] [PubMed]
- Yang L, Yang Y, Ma P, et al. Spread through air spaces predicts a worse survival in patients with stage I adenocarcinomas >2 cm after radical lobectomy. J Thorac Dis 2018;10:5308-17. [Crossref] [PubMed]
- Zhao ZR, Xi SY, Li W, et al. Prognostic impact of pattern-based grading system by the new IASLC/ATS/ERS classification in Asian patients with stage I lung adenocarcinoma. Lung Cancer 2015;90:604-9. [Crossref] [PubMed]
- Kadota K, Colovos C, Suzuki K, et al. FDG-PET SUVmax combined with IASLC/ATS/ERS histologic classification improves the prognostic stratification of patients with stage I lung adenocarcinoma. Ann Surg Oncol 2012;19:3598-605. [Crossref] [PubMed]