Prognostic effect of systemic inflammation in patients undergoing surgery for hepatocellular carcinoma: comparison of composite ratios and cumulative scores
Introduction
Worldwide, liver cancer is the sixth most common tumor and the fourth leading cause of cancer-related deaths (1). China is a high-risk area for primary liver cancer (PLC), in which more than half of the world’s new cases of liver cancer occur each year (2). Hepatocellular carcinoma (HCC) is the most important type of PLC. At present, surgical resection is still the first choice for the treatment of early liver cancer, and the radical hepatectomy is an important means of obtaining long-term survival, however, the overall 5-year survival rate of patients after surgery is only 25% to 50% (3,4). The clinical stage of the tumor can be used as a basis for determining the treatment plan and predicting the prognosis of the patient. Clarification of the postoperative high-risk subgroup of patients will help to optimize the postoperative adjuvant therapy. However, the current clinical staging systems of various PLCs have limitations and lack universality (5).
There is increasing evidence shows that systemic inflammatory responses play a crucial role in the development and progression of tumors and are associated with overall survival (OS); therefore, these responses can help clinically identify patients with poor prognosis. Recently, systemic inflammation has been linked to poorer outcomes and increased tumor progression. Such systemic inflammatory responses have been investigated using indexes such as the elevation of the neutrophil-lymphocyte ratio (NLR) and platelet-lymphocyte ratio (PLR). Inflammatory indexes have been shown to be prognostic markers in other gastroenterological malignancies as well as in HCC. It is known that inflammation can increase tumor risk and influence all tumor stages, triggering the initial genetic mutation or epigenetic mechanism, promoting tumor initiation, metastasis and progression. Thus, inflammation parameter is a powerful candidate to predict cancer outcome. Composite ratios and cumulative scores based on preoperative serum inflammatory levels. It may work as a better prognostic marker for long-term outcomes in HCC patients receiving hepatectomy. We conducted this retrospective study in a large cohort of HCC patients undergoing curative resection, attempting to investigate the prognostic effect of the systemic inflammatory indexes in HCC patients undergoing curative resection.
To date, studies systematically evaluating whether these inflammatory indicators predict the OS rate of postoperative tumors in patients with liver cancer are rare. Therefore, the aim of this study was to evaluate the prognostic effect of the composite ratio and cumulative scores in patients receiving curative resection for HCC.
We present the following article in accordance with the STROBE reporting checklist (available at http://dx.doi.org/10.21037/tcr-20-2089).
Methods
Patients
The study was conducted in accordance with the Declaration of Helsinki (as revised in 2013). This study was approved by the Ethics Committee of Fudan University Shanghai Cancer Center (Protocol: 202003017) and informed consent was taken from all the patients. From January 2015 to December 2017, patients receiving curative resection for HCC in FUSCC were enrolled. The inclusion criteria were: postoperative pathology shows HCC; complete clinical basic information and postoperative follow-up data; patients with PLC stage I-III according to the eighth edition of AJCC (American Joint Committee on Cancer) TNM staging; and complete laboratory test results for neutrophils, lymphocytes, platelets, C-reactive protein, and albumin obtained one week prior to surgery. The exclusion criteria included pathology-confirmed nonhepatocellular cell carcinoma; preoperative neoadjuvant therapy; radiation therapy; infectious diseases preoperatively; autoimmune diseases and other malignant tumors; loss of follow-up; and lack of perioperative hematology records. The data were collected from the database of the FUSCC clinical information system. The medical information of each patient was reviewed and recorded, including demographic information, primary diagnosis, medical history, operative details (procedure type and venous invasion), anesthesia method, tumor differentiation and pathological staging. The strategy for follow-up is as follows: follow-up every 3 months in the first 1–2 years and then every 6 months in the third years. The follow-up included routine examination, chest tumor markers, and abdominal enhanced CT. The endpoint of this study was OS. The OS is defined as the period from the date of surgery to the date of death or last follow-up. Follow-up was continued until December 2018 or until the patient died.
Methods for calculate inflammatory scores
Indicators related to inflammatory responses, such as the NLR and the PLR, have been proposed as prognostic indexes for tumors. These prognostic indexes of systemic inflammatory response are usually based on two main approaches (6). One approach is to utilize the composite ratios of the white cell count and then apply the prediction threshold to the ratio so that the results are effectively layered. In addition to NLR and PLR, the most repeatedly validated examples of this approach are the lymphocyte-monocyte ratio (LMR) and the C-reactive protein/albumin ratio (CAR). Another method is the cumulative prognostic score, where indexes of the systemic inflammatory response are specified as normal or as abnormal based on their laboratory reference ranges so that two indexes with normal values score lowest and relate with the best outcomes, and two indexes with abnormal values score highest and relate with the poorest outcomes. The most widely confirmed example of this approach is the Glasgow prognostic score (mGPS) based on the acute phase proteins, C-reactive protein and albumin. Lately, the neutrophil-platelet score (NPS) using neutrophils and platelets has been reported (7). Similarly, the cumulative score can also be applied to the ratios described above, such as the neutrophil-lymphocyte score (NLS), platelet-lymphocyte score (PLS) and NPS (Table 1) (6).
Table 1
Variables | Ratio/score |
---|---|
NLR | |
Neutrophil count to lymphocyte count ratio | ≤3 |
Neutrophil count to lymphocyte count ratio | 3–5 |
Neutrophil count to lymphocyte count ratio | >5 |
PLR | |
Platelet count to lymphocyte count ratio | ≤150 |
Platelet count to lymphocyte count ratio | >150 |
LMR | |
Lymphocyte count to monocyte count ratio | ≤2.40 |
Lymphocyte count to monocyte count ratio | >2.40 |
CAR | |
C-reactive protein to albumin ratio | ≤0.22 |
C-reactive protein to albumin ratio | >0.22 |
NLS | |
Neutrophil count ≤7.5×109/L combined with lymphocyte count ≥1.5×109/L | 0 |
Neutrophil count >7.5×109/L combined with lymphocyte count ≥1.5×109/L | 1 |
Neutrophil count ≤7.5×109/L combined with lymphocyte count <7.5×109/L | 1 |
Neutrophil count >7.5×109/L combined with lymphocyte count <7.5×109/L | 2 |
PLS | |
Platelet count ≤400×109/L combined with lymphocyte count ≥1.5×109/L | 0 |
Platelet count >400×109 /L combined with lymphocyte count ≥1.5×109/L | 1 |
Platelet count ≤400×109/L combined with lymphocyte count <1.5×109/L | 1 |
Platelet count >400×109/L combined with lymphocyte count <1.5×109/L | 2 |
NPS | |
Neutrophil count ≤7.5×109/L combined with platelet count <400×109/L | 0 |
Neutrophil count >7.5×109/L combined with platelet count <400×109/L | 1 |
Neutrophil count >7.5×109/L combined with platelet count >400×109/L | 1 |
Neutrophil count >7.5×109/L combined with platelet count >400×109/L | 2 |
mGPS | |
C-reactive protein ≤10 mg/L combined with albumin ≥35 g/L | 0 |
C-reactive protein >10 mg/L combined with albumin ≥35 g/L | 2 |
NLR, neutrophil to lymphocyte ratio; NLS, neutrophil and lymphocyte score; CAR, C-reactive protein to albumin ratio; mGPS, modified Glasgow prognostic score; NPS, neutrophil and platelet score; LMR, lymphocyte to monocyte ratio; PLR, platelet to lymphocyte ratio; PLS, platelet and lymphocyte score.
Statistical analysis
All data were analyzed using SPSS version 17.0 (SPSS Inc., Chicago, IL, USA), and a P value <0.05 was considered to be statistically significant.
First, we used the composition ratio to represent the basic information of the sample and the receiver operating curve (ROC) analysis method to determine the optimal threshold values of LMR, NLR and PLR; we used Pearson’s chi-square test to determine the relationship between the scoring index and general data. When the P value was less than 0.05, the data were considered to be statistically significant; the median was used to describe the level of the inflammatory index at different levels of different indicators; ROC analysis was used to analyze the predictive value of various inflammatory indicators for survival prognosis; finally, COX regression was performed to analyze and plot the Kaplan-Meier survival curve, determine the HR, and compare the survival rate differences of different levels. A Chi-square test was used to compare the differences between categorical variables. Statistically significant variables from the univariate analysis were included in the multivariate analysis.
Results
Patients clinical characteristic
In this retrospective study, 595 patients with primary HCC who underwent elective hepatic tumor resection were enrolled; most patients were between 45 and 60 years of age (49.1%); male (66.9%); had normal weight (67.8%); and underwent open surgery (61.1%). The ratio of general anesthesia to general anesthesia combined with epidural anesthesia was approximately equivalent (53.7% vs. 46.3%). For most patients, the TNM staging was stage I (57%), and tumor differentiation was moderate to good (75.2%). Most patients had no vascular invasion (73.2%), and 148 patients died during follow-up (Table 2).
Table 2
Characteristics | Category | n=595 (%) |
---|---|---|
Gender | Male | 398 (66.9) |
Female | 197 (33.1) | |
Age | <45 | 142 (23.9) |
45–60 | 292 (49.1) | |
>60 | 161 (27.0) | |
BMI | <18.5 | 16 (2.7) |
18.5–23.9 | 403 (67.8) | |
24–27.9 | 168 (28.2) | |
≥28 | 8 (0.7) | |
pT | 1 | 275 (46.3) |
2 | 171 (28.7) | |
3 | 149 (25.0) | |
pN | 0 | 392 (65.8) |
1 | 203 (34.2) | |
TNM | 1 | 340 (57.0) |
2 | 179 (30.9) | |
3 | 76 (12.1) | |
Surgery type | Open | 362 (61.1) |
Laparoscopic | 233 (38.9) | |
Anesthesia method | General anesthesia | 319 (53.7) |
General combined epidural | 276 (46.3) | |
Differentiation | Poor | 147 (24.8) |
Well | 448 (75.2) | |
Venous invasion | No | 436 (73.2) |
Yes | 159 (26.8) |
BMI, body mass index; TNM, tumor node metastasis.
The relationship between cumulative scores and composite ratios and the clinicopathological characteristics of patients receiving elective surgery for HCC is shown in Table 3. There was a statistically significant correlation between BMI and NPS, CAR, and mGPS (P<0.05). The degree of tumor differentiation was correlated with NLR, PLR, LMR, NLS, CAR, PLS, NPS and mGPS (P<0.05). Vascular invasion was associated with NLR, PLR, LMR, NPS, CAR, and mGPS (P<0.05). TNM was associated with NLS, PLS, PLS, NPS, CAR, and mGPS (P<0.05) (Table 3 ).
Table 3
Variables | Gender | Age | BMI | T | N | Differentiation | Venous invasion | TNM |
---|---|---|---|---|---|---|---|---|
NLR | 0.482 | 0.355 | 0.274 | 0.623 | 0.501 | 0.000 | 0.000 | 0.868 |
PLR | 0.651 | 0.088 | 0.453 | 0.021 | 0.490 | 0.000 | 0.001 | 0.061 |
LMR | 0.475 | 0.228 | 0.321 | 0.182 | 0.996 | 0.000 | 0.000 | 0.463 |
CAR | 0.769 | 0.842 | 0.014 | 0.003 | 0.653 | 0.001 | 0.001 | 0.010 |
NLS | 0.682 | 0.671 | 0.924 | 0.000 | 0.125 | 0.000 | 0.218 | 0.000 |
PLS | 0.562 | 0.760 | 0.843 | 0.000 | 0.110 | 0.000 | 0.361 | 0.000 |
NPS | 0.504 | 0.592 | 0.010 | 0.000 | 0.653 | 0.002 | 0.004 | 0.010 |
mGPS | 0.901 | 0.526 | 0.007 | 0.001 | 0.530 | 0.000 | 0.006 | 0.033 |
P<0.05 is considered to be significant. NLR, neutrophil to lymphocyte ratio, NLS, neutrophil and lymphocyte score, CAR, C-reactive protein to albumin ratio, mGPS, modified Glasgow prognostic score, NPS, neutrophil and platelet score, LMR, lymphocyte to monocyte ratio, PLR, platelet to lymphocyte ratio, PLS, platelet and lymphocyte score.
The relationship between composite ratios and component values and corresponding cumulative scores in patients undergoing surgery for HCC is shown in Table 4. Most patients were not in an inflammatory state before undergoing surgery: NLR <3 (69.8%), NLS =0 (51.7%), PLR ≤150 (63.8%), PLS =0 (51.7%), NPS =0 (98%), CAR ≤0.22 (96%), mGPS =0 (95.3%).
Table 4
Variables | Category | n (%) | Hazard ratio, median (range) neutrophil | Hazard ratio, median (range) lymphocyte |
---|---|---|---|---|
NLR | ≤3 | 415 (69.8) | Neutrophil: 2.8 (0.6–3.9) | Lymphocyte: 1.7 (1.3–3.0) |
3–5 | 116 (19.5) | Neutrophil: 4.3 (3.9–5.0) | Lymphocyte: 1.1 (1.0–1.2) | |
>5 | 64 (10.7) | Neutrophil: 6.2 (5.1–10.8) | Lymphocyte: 0.8 (0.4–1.0) | |
PLR | ≤150 | 379 (63.8) | Platelet: 144 (27.0–195.0) | Lymphocyte: 1.8 (1.3–3.0) |
>150 | 216 (36.2) | Platelet: 226.5 (103.0–641.0) | Lymphocyte: 1.1 (0.4–1.3) | |
LMR | ≤2.40 | 463 (77.9) | Lymphocyte: 1.6 (1.2–3.0) | Monocyte: 0.4 (0.1–0.5) |
>2.4 | 132 (22.1) | Lymphocyte: 1.0 (0.4–1.1) | Monocyte: 0.6 (0.5–1.5) | |
CAR | ≤0.22 | 571 (96.0) | C-protein: 1.5 (0.1–6.8) | Albumin: 43.7 (34.9–58.5) |
>0.22 | 24 (4.0) | C-protein: 12.3 (9.8–34.5) | Albumin: 32.6 (29.5–34.6) | |
NLS | 0 | 308 (51.7) | Neutrophil: 2.5 (0.6–3.2) | Lymphocyte: 1.9 (1.5–3.0) |
1 | 287 (48.3) | Neutrophil: 4 (3.2–10.8) | Lymphocyte: 1.2 (0.4–1.4) | |
PLS | 0 | 308 (51.7) | Platelet: 123.0 (27.0–161) | Lymphocyte: 1.9 (1.5–3) |
1 | 287 (48.3) | Platelet: 214 [103–641] | Lymphocyte: 1.2 (0.4–1.4) | |
NPS | 0 | 583 (98.0) | Neutrophil: 3.2 (0.6–7.1) | Platelet: 161.5 [27–375] |
1 | 12 (2.0) | Neutrophil: 9.8 (9.7–10.8) | Platelet: 475 [103–641] | |
mGPS | 0 | 567 (95.3) | C-protein: 1.5 (0.1–6.8) | Albumin: 43.7 (35.1–58.5) |
1 | 28 (4.7) | C-protein: 11.0 (6.8–34.5) | Albumin: 32.9 (29.5–34.9) |
NLR, neutrophil to lymphocyte ratio; NLS, neutrophil and lymphocyte score; CAR, C-reactive protein to albumin ratio; mGPS, modified Glasgow prognostic score; NPS, neutrophil and platelet score; LMR, lymphocyte to monocyte ratio; PLR, platelet to lymphocyte ratio; PLS, platelet and lymphocyte score.
The median values for the components of the scores and ratios are shown in Table 4. When the NLR ratio was less than 3, the median neutrophil count was 2.8×109/L, and the median lymphocyte count was 1.7×109/L. Both values were within the normal range, but when the NLR ratio was 3–5 or greater than 5, the median neutrophil count and median lymphocyte count were also in the normal range. A PLR >150 was associated with a median platelet count of 226.5×109/L and a median lymphocyte count of 1.1×109/L; both were within the normal reference range. An LMR >2.4 was associated with a median lymphocyte count of 1.0×109/L and a median monocyte count of 0.6×109/L; both were within the normal reference range. When CAR was >0.22, the C-reactive protein count was 12.3 mg/L, which was above the normal range, and the albumin count was 32.6 g/L, which was below the normal range. The neutrophil, lymphocyte and platelet counts corresponding to different NLS and PLS are within the normal range. However, when the NPS was 1, the neutrophil and platelet counts exceeded the normal value. When the mGPS was 1, the C-reactive protein count was 11.0 mg/L, which was above the normal range, and the albumin count was 32.9 g/L, which was below the normal range. However, when NPS and mGPS were 1, the proportion of patients corresponding to them was lower, at 2.0% and 4.7%, respectively.
ROC curve analysis
The predictive ability between component values and composite ratios in patients undergoing surgery for HCC is shown in Figure 1 and Table 5. On ROC analysis using standard thresholds and OS as an end-point, the area under the ROC curve (AUC) for TNM stage was 0.767 (95% CI, 0.674–0.86), NLR was 0.574 (95% CI, 0.467–0.681), PLR was 0.679 (95% CI, 0.58–0.779), LMR was 0.622 (95% CI, 0.515–0.729), CAR was 0.575 (95% CI, 0.465–0.685), NLS was 0.673 (95% CI, 0.510–0.851), PLS was 0.728 (95% CI, 0.641–0.815), NPS was 0.538 (95% CI, 0.43–0.645), mGPS was 0.588 (95% CI, 0.477–0.698). The AUC of all the values were greater than 0.5, of which the AUC of PLS was greater than 0.7, indicating that these indicators have predictive value for prognosis.
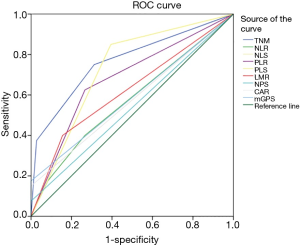
Table 5
Variables | Category | AUC (95% CI) | Univariate | Multivariate (adjusted for TNM stage) | |||
---|---|---|---|---|---|---|---|
HR (95% CI) | P value | HR (95% CI) | P value | ||||
TNM | 1 | 0.767 (0.674–0.86) | |||||
2 | 4.31 (1.92–9.68) | 0.000 | |||||
3 | 10.70 (4.67–24.51) | 0.000 | |||||
NLR | ≤3 | 0.574 (0.467–0.681) | |||||
3–5 | 0.94 (0.44–2.03) | 0.875 | 0.94 (0.44–2.03) | 0.573 | |||
>5 | 1.50 (0.65–3.49) | 0.346 | 1.50 (0.65–3.49) | 0.196 | |||
LMR | ≤2.40 | 0.622 (0.515–0.729) | |||||
>2.4 | 2.47 (1.30–4.68) | 0.006 | 2.01 (1.05–3.82) | 0.034 | |||
PLR | ≤150 | 0.679 (0.58–0.779) | |||||
>150 | 2.58 (1.36–4.90) | 0.004 | 2.19 (1.15–4.18) | 0.017 | |||
CAR | ≤0.22 | 0.575 (0.465–0.685) | |||||
>0.22 | 5.27 (2.18–12.75) | 0.000 | 3.16 (1.28–7.78) | 0.012 | |||
NLS | 0 | 0.673 (0.510–0.851) | |||||
1 | 5.66 (2.10–13.56) | 0.000 | 3.45 (1.38–8.62) | 0.004 | |||
PLS | 0 | 0.728 (0.641–0.815) | |||||
1 | 6.04 (2.54–14.41) | 0.000 | 3.67 (1.47–9.12) | 0.005 | |||
NPS | 0 | 0.538 (0.43–0.645) | |||||
1 | 13.17 (3.74–46.35) | 0.000 | 5.35 (1.48–19.31) | 0.010 | |||
mGPS | 0 | 0.588 (0.477–0.698) | |||||
1 | 3.95 (1.74–8.97) | 0.001 | 3.18 (1.39–7.3) | 0.006 |
NLR, neutrophil-lymphocyte ratio; NLS, neutrophil-lymphocyte score; CAR, C-reactive protein albumin ratio; mGPS, modified Glasgow prognostic score; NPS, neutrophil-platelet score; LMR, lymphocyte-monocyte ratio; PLR, platelet-lymphocyte ratio; PLS, platelet-lymphocyte score; TNM, tumor node metastasis.
Univariate analysis of OS
For univariate analysis, except for NLR (P>0.5), TNM (P=0.000, HR 4.31, 95% CI, 1.92–9.68), PLR (P=0.004, HR 2.58, 95% CI, 1.36–4.9), LMR (P=0.006, HR 2.47, 95% CI, 1.3–4.68), CAR (P=0.000, HR 5.27, 95% CI, 2.18–12.75), NLS (P=0.000, HR 5.66, 95% CI, 2.10–13.56), PLS (P=0.000, HR 6.04, 95% CI, 2.54–14.41), NPS (P=0.000, HR 13.17, 95% CI, 3.74–46.35), and mGPS (P=0.001, HR 3.95, 95% CI, 1.74–8.97) were significantly associated with postoperative OS. When multivariate analysis was adjusted according to TNM, except for NLR (P>0.5), PLR >150 (P=0.017, HR 2.19, 95% CI, 1.15–4.18), LMR >2.4 (P=0.034, HR 2.01, 95% CI, 1.05–3.82), CAR >0.22 (P=0.012, HR 3.16, 95% CI, 1.28–7.78), NLS =1 (P=0.004, HR 3.45, 95% CI, 1.38–8.62), PLS =1 (P=0.005, HR 3.67, 95% CI, 1.47–9.12), NPS =1 (P=0.010, HR 5.35, 95% CI, 1.48–19.31), and mGPS =1 (P=0.006, HR 3.18, 95% CI, 1.39–7.3) were significantly associated with postoperative OS. These results indicate that NLS, PLR, PLS, LMR, NPS, CAR, and mGPS can be used as independent predictors of prognosis, but not NLR (Table 5).
Kaplan-Meier analysis of OS
Different levels of NLR, PLR, LMR, NLS, CAR, PLS, NPS, mGPS and postoperative OS time curves were plotted by the Kaplan-Meier method (Figures 2-5). The results showed that except for NLR (P>0.05), patients with PLR >150, LMR >2.4, CAR >0.22, NLS 1, PLS 1, NPS 1, and mGPS 1 had significantly lower postoperative survival rates (P<0.001, respectively).
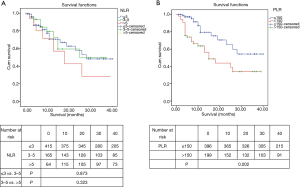
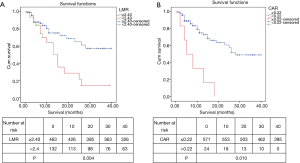
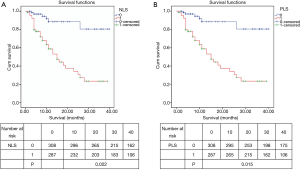
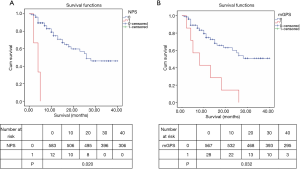
Discussion
The prognosis of patients with liver cancer is related to clinical pathological features, including the number of tumors, tumor size, depth of invasion, lymph node involvement, distant metastasis, and histological type (8). At present, TNM staging is considered to be one of the main criteria for the clinical evaluation of prognosis in patients with liver cancer (5). However, even patients with the same TNM staging have different prognoses, indicating that there is a deficiency in the prognosis of patients when only considering TNM staging (9). Therefore, from a clinical perspective, we have been looking for a supplementary diagnosis and treatment method with high sensitivity and specificity, economy and simple detection to guide the treatment of liver cancer patients. In this study, we compared the predictive effects of commonly used inflammatory markers on the prognosis of patients with liver cancer. The results showed that a PLR of >150, an LMR of >2.4, a CAR of >0.22, an NLS of 1, a PLS of 1, an NPS of 1, mGPS of 1 were negatively correlated with postoperative OS in patients with liver cancer.
Inflammation has been recognized as one of the biological characteristics of malignant tumors, and inflammation plays an important role in the different stages of tumor development (10). In the tumor microenvironment and systemic circulation, tumor-associated inflammation is related to the progression and prognosis of many tumors (11). Although there are many methods for grading liver cancer, these grading methods focus more on tumor-related factors, liver function and general patient condition and less on the degree of inflammatory reaction in patients (12).
The levels of circulating immune cells and some circulating proteins, such as C-reactive protein and albumin, are associated with tumor-associated inflammatory responses, reflecting the state of systematic antitumor immune function and predicting the patient’s prognosis. In addition to eliminating tumor cells, neutrophils also play an important role in tumor growth stimulation, mainly through the secretion of different cytokines, growth factors and proteases (13). Lymphocytes are the main component of antitumor immunity. The decrease in the number of lymphocytes leads to a decrease in the activity of lysing and killing tumor cells, thereby promoting the proliferation and migration of tumor cells (14,15). The level of monocytes in the circulation is supposed to be related to the level of tumor-associated macrophages (TAM), which may reflect the level of formation and presence, and the tumor burden is also considered to be high when monocytes in peripheral blood increase (16,17). Platelets are derived from mature megakaryocyte cytoplasm, which can stop bleeding and promote wound healing. Platelets are also actively involved in the development of tumors, including extravasation, growth, metastasis, neovascularization and immune escape (18,19). CRP is an acute phase protein synthesized after tissue damage or tissue infection. In tumor patients, CRP can be induced by cytokines, such as IL-6, in the tumor and tumor microenvironment, and high CRP levels are associated with the poor prognosis of various types of tumors (20-22). Albumin is the most abundant protein in plasma, and it is an important indicator of the nutritional status of patients. Albumin also reflects the immune status and is related to the prognosis of cancer patients (23). However, the single indicator was not so sensitive. In our study, the single preoperative index of most patients was within the normal range. Therefore, based on these single indicators, some systemic inflammation indicators have been proposed, which can provide a reference for improving the risk stratification of cancer patients and providing more targeted treatments (24-27).
There are several limitations in this study. First, it was retrospectively analyzed, and the study was limited to one hospital. The clinical materials analyzed in this study were obtained solely from patients in China. Second, most of the patients included in the study had early liver cancer. Larger prospective trials including diverse ethnic populations are warranted to further confirm the validity of these biomarkers and to assess their prognostic and predictive potential for liver cancer patients.
Conclusions
In summary, our analysis identified that PLR, LMR, CAR, NLS, PLS, NPS and mGPS are associated with postoperative OS in patients with liver cancer, except for NLR. Furthermore, cumulative scores are more relevant than composite ratios for obtaining prognoses in patients with liver cancer. Our present study directly compares, the prognostic value of composite ratios and cumulative scores of the systemic inflammatory response. These ratios and scores, whether composed of white cells from lymphoid/myeloid tissue or from acute phase proteins from the liver, had prognostic value, independent of TNM stage, in patients with HCC. However, cumulative scores, based on normal reference ranges, are simpler and more consistent for clinical use.
Acknowledgments
Funding: This work was supported by
Footnote
Reporting Checklist: The authors have completed the STROBE reporting checklist. Available at http://dx.doi.org/10.21037/tcr-20-2089
Data Sharing Statement: Available at http://dx.doi.org/10.21037/tcr-20-2089
Conflicts of Interest: All authors have completed the ICMJE uniform disclosure form (available at http://dx.doi.org/10.21037/tcr-20-2089). The authors have no conflicts of interest to declare.
Ethical Statement: The authors are accountable for all aspects of the work in ensuring that questions related to the accuracy or integrity of any part of the work are appropriately investigated and resolved. The study was conducted in accordance with the Declaration of Helsinki (as revised in 2013). The study was approved by ethics board of Fudan University Shanghai Cancer Center (Protocol: 202003017) and informed consent was taken from all the patients.
Open Access Statement: This is an Open Access article distributed in accordance with the Creative Commons Attribution-NonCommercial-NoDerivs 4.0 International License (CC BY-NC-ND 4.0), which permits the non-commercial replication and distribution of the article with the strict proviso that no changes or edits are made and the original work is properly cited (including links to both the formal publication through the relevant DOI and the license). See: https://creativecommons.org/licenses/by-nc-nd/4.0/.
References
- Bray F, Ferlay J, Soerjomataram I, et al. Global cancer statistics 2018: GLOBOCAN estimates of incidence and mortality worldwide for 36 cancers in 185 countries. CA Cancer J Clin 2018;68:394-424. [Crossref] [PubMed]
- Chen W, Zheng R, Baade PD, et al. Cancer statistics in China, 2015. CA Cancer J Clin 2016;66:115-32. [Crossref] [PubMed]
- Marrero JA, Kulik LM, Sirlin CB, et al. Diagnosis, Staging, and Management of Hepatocellular Carcinoma: 2018 Practice Guidance by the American Association for the Study of Liver Diseases. Hepatology 2018;68:723-50. [Crossref] [PubMed]
- Vogel A, Cervantes A, Chau I, et al. Hepatocellular carcinoma: ESMO Clinical Practice Guidelines for diagnosis, treatment and follow-up. Ann Oncol 2018;29:iv238-iv255. [Crossref]
- Liu PH, Hsu CY, Hsia CY, et al. Prognosis of hepatocellular carcinoma: Assessment of eleven staging systems. J Hepatol 2016;64:601-8. [Crossref] [PubMed]
- Dolan RD, McSorley ST, Park JH, et al. The prognostic value of systemic inflammation in patients undergoing surgery for colon cancer: comparison of composite ratios and cumulative scores. Br J Cancer 2018;119:40-51. [Crossref] [PubMed]
- Dolan RD, Lim J, McSorley ST, et al. The role of the systemic inflammatory response in predicting outcomes in patients with operable cancer: Systematic review and meta-analysis. Sci Rep 2017;7:16717. [Crossref] [PubMed]
- Kulik L, El-Serag HB. Epidemiology and Management of Hepatocellular Carcinoma. Gastroenterology 2019;156:477-491.e1. [Crossref] [PubMed]
- Kim DY, Han KH. Staging for hepatocellular carcinoma in light of tumor heterogeneity: Time to change or update? Hepatology 2018;67:2076-8. [Crossref] [PubMed]
- Hanahan D, Weinberg RA. Hallmarks of cancer: the next generation. Cell 2011;144:646-74. [Crossref] [PubMed]
- Sylman JL, Mitrugno A, Atallah M, et al. The Predictive Value of Inflammation-Related Peripheral Blood Measurements in Cancer Staging and Prognosis. Front Oncol 2018;8:78. [Crossref] [PubMed]
- Bruix J, Reig M, Sherman M. Evidence-Based Diagnosis, Staging, and Treatment of Patients with Hepatocellular Carcinoma. Gastroenterology 2016;150:835-53. [Crossref] [PubMed]
- Galdiero MR, Varricchi G, Loffredo S, et al. Roles of neutrophils in cancer growth and progression. J Leukoc Biol 2018;103:457-64. [Crossref] [PubMed]
- Ray-Coquard I, Cropet C, Van Glabbeke M, et al. Lymphopenia as a prognostic factor for overall survival in advanced carcinomas, sarcomas, and lymphomas. Cancer Res 2009;69:5383-91. [Crossref] [PubMed]
- Suzuki R, Lin SH, Wei X, et al. Prognostic significance of pretreatment total lymphocyte count and neutrophil-to-lymphocyte ratio in extensive-stage small-cell lung cancer. Radiother Oncol 2018;126:499-505. [Crossref] [PubMed]
- Shibutani M, Maeda K, Nagahara H, et al. The peripheral monocyte count is associated with the density of tumor-associated macrophages in the tumor microenvironment of colorectal cancer: a retrospective study. BMC Cancer 2017;17:404. [Crossref] [PubMed]
- Hayashi T, Fujita K, Nojima S, et al. Peripheral blood monocyte count reflecting tumor-infiltrating macrophages is a predictive factor of adverse pathology in radical prostatectomy specimens. Prostate 2017;77:1383-8. [Crossref] [PubMed]
- Franco AT, Corken A, Ware J. Platelets at the interface of thrombosis, inflammation, and cancer. Blood 2015;126:582-8. [Crossref] [PubMed]
- Schlesinger M. Role of platelets and platelet receptors in cancer metastasis. J Hematol Oncol 2018;11:125. [Crossref] [PubMed]
- Allin KH, Nordestgaard BG. Elevated C-reactive protein in the diagnosis, prognosis, and cause of cancer. Crit Rev Clin Lab Sci 2011;48:155-70. [Crossref] [PubMed]
- Shiels MS, Pfeiffer RM, Hildesheim A, et al. Circulating inflammation markers and prospective risk for lung cancer. J Natl Cancer Inst 2013;105:1871-80. [Crossref] [PubMed]
- Kim WR, Han YD, Min BS. C-Reactive Protein Level Predicts Survival Outcomes in Rectal Cancer Patients Undergoing Total Mesorectal Excision After Preoperative Chemoradiation Therapy. Ann Surg Oncol 2018;25:3898-905. [Crossref] [PubMed]
- Liu X, Meng QH, Ye Y, et al. Prognostic significance of pretreatment serum levels of albumin, LDH and total bilirubin in patients with non-metastatic breast cancer. Carcinogenesis 2015;36:243-8. [Crossref] [PubMed]
- Soyano AE, Dholaria B, Marin-Acevedo JA, et al. Peripheral blood biomarkers correlate with outcomes in advanced non-small cell lung Cancer patients treated with anti-PD-1 antibodies. J Immunother Cancer 2018;6:129. [Crossref] [PubMed]
- Bilen MA, Martini DJ, Liu Y, et al. The prognostic and predictive impact of inflammatory biomarkers in patients who have advanced-stage cancer treated with immunotherapy. Cancer 2019;125:127-34. [Crossref] [PubMed]
- Yeh YC, Lei HJ, Chen MH, et al. C-Reactive Protein (CRP) is a Promising Diagnostic Immunohistochemical Marker for Intrahepatic Cholangiocarcinoma and is Associated with Better Prognosis. Am J Surg Pathol 2017;41:1630-41. [Crossref] [PubMed]
- He L, Li H, Cai J, et al. Prognostic Value of the Glasgow Prognostic Score or Modified Glasgow Prognostic Score for Patients with Colorectal Cancer Receiving Various Treatments: A Systematic Review and Meta-Analysis. Cell Physiol Biochem 2018;51:1237-49. [Crossref] [PubMed]