Development of a nomogram to predict prognosis in ovarian cancer: a SEER-based study
Introduction
Ovarian cancer remains the most lethal gynecologic malignancy and the fifth leading cause of cancer-related mortality globally (1,2), with approximately 185,000 women died from the disease worldwide in 2018 (3,4). Epithelial ovarian cancer is the most common histologic type that encompasses a clinically and biologically heterogeneous class of tumors including several major subtypes (serous, mucinous, endometrioid and clear cell carcinoma (5). The remaining ovarian cancer types are mainly thought to originate from stromal granulosa, theca, and germ cells (6,7). The high mortality rate pf ovarian cancer patients due to asymptomatic disease onset and resulting late diagnosis (stage III or IV) with bowel obstruction and systemic involvement (8). Furthermore, the effectiveness of currently available treatments diminishes over time and relapse occurs in the majority of patients (9), despite a high initial response rate to platinum and taxanes therapy following cytoreductive surgery in cases of advanced cancer (10). Ovarian cancer subsequently develops into incurable disease for which treatment options remain limited (11) and the reported 5-year survival rate is ~40% (12). Comprehensive characterization of the mechanisms underlying ovarian cancer is therefore essential for developing effective therapeutic strategies.
Prognostic nomograms are graphical calculation scales for predictive models to maximize the accuracy of individual prognosis (13,14) via Kaplan-Meier survival analysis and multivariate Cox proportional hazards model (15). Currently, nomograms are widely used to assist surgeons in developing treatment plans and evaluating prognosis for various tumor types, including hepatocellular carcinoma (16), gastric cancer (17), nasopharyngeal cancer (18) and several other cancers (19,20). In the current study, we retrieved and used information available from the Surveillance, Epidemiology, and End Results (SEER) database with a view to identifying risk factors affecting overall survival (OS) and developing a nomogram for visually predicting prognosis of patients with ovarian cancer. We present the following article in accordance with the STROBE reporting checklist (available at http://dx.doi.org/10.21037/tcr-20-1238).
Methods
Data sources
We collected clinicopathological data from 75,921 patients with ovarian cancer from 2004 to 2016 available in the SEER program of the National Cancer Institute. The SEER database consists of 18 registries covering ~28% of the US population and includes collated information on cancer incidence, prevalence, mortality, population-based variables, primary tumor characteristics and treatments, excluding chemotherapy (21,22). The program has been commonly used by researchers to search for prognostic factors associated with various cancer types (23-26).
Study population
Patients diagnosed with ovarian cancer from 2004 to 2016 were identified from the SEER database. Tumor staging was manually restaged based on the lasted AJCC criteria. The following information was obtained for each patient: race, age, tumor laterality, histology, grade, stage, surgery, radiotherapy, chemotherapy, insurance, and marital status. Patients with missing data were excluded. Eligible cases were randomly divided into the training cohort and the validation cohort at a 7:3 ratio. Ethics approval was not required because that all the data of ovarian cancer patients in our study were gained from SEER database. The study was conducted in accordance with the Declaration of Helsinki (as revised in 2013).
Statistical analysis
The prognostic effect of each variable on survival was evaluated using the Kaplan-Meier method and log-rank test. Multivariate analyses were performed using the Cox proportional hazards regression model. Variance inflation factors (VIF) were calculated to evaluate the independence of each variable. Survival was calculated in months from the date of initial surgery to the last follow-up. Statistical analyses were performed using the software package R version 3.3.1 (http://www.r-project.org/).
A nomogram was formulated based on the results of multivariate analysis using the rms package in R version 3.3.1. The maximum score of each factor was set as 10. The performance of the nomogram was measured according to the concordance index (C-index) and assessed by comparing nomogram-predicted versus observed Kaplan-Meier estimates of survival probability. Accuracy was required to be validated by 500 times bootstrapping and 10-fold cross-validation measures internally and externally. The fitting degree was evaluated on the basis of concordance index (C-index) values and calibration plots, which were derived based on regression analysis. A probability (P) value of <0.05 was considered statistically significant.
Results
Patient characteristics
A total of 75,921 ovarian cancer patients were identified from the SEER database, of which 47,546 were excluded (Figure 1), resulting in the final inclusion of 28,375 patients (19,862 in the training cohort, 8,513 in the validation cohort). The clinical characteristics of our study patients are summarized in Table 1. Tumor pathology was categorized into six groups: serous, mucinous, endometrioid, clear-cell, sex cord-stromal and germ cell carcinoma.
Table 1
Characteristics | Total, n (%) | Training cohort, n (%) | Validation cohort, n (%) | P |
---|---|---|---|---|
Total No. | 28,375 | 19,862 | 8,513 | |
Race | 0.443 | |||
White | 23,597 (83.2) | 16,536 (83.3) | 7,061 (82.9) | |
Black | 1,898 (6.7) | 1,324 (6.7) | 574 (6.7) | |
Others | 2,880 (10.1) | 2,002 (10.1) | 878 (10.3) | |
Age, years | 0.307 | |||
≤30 | 1,086 (3.8) | 750 (3.8) | 336 (3.9) | |
31–40 | 1,585 (5.6) | 1,104 (5.6) | 481 (5.7) | |
41–50 | 4,942 (17.4) | 3,452 (17.4) | 1,490 (17.5) | |
51–60 | 8,039 (28.3) | 5,614 (28.3) | 2,425 (28.5) | |
61–70 | 7,083 (25) | 4,976 (25.1) | 2,107 (24.8) | |
71–80 | 4,160 (14.7) | 2,915 (14.7) | 1,245 (14.6) | |
>80 | 1,480 (5.2) | 1,051 (5.3) | 429 (5.0) | |
Laterality | 0.070 | |||
Unilateral | 16,007 (56.4) | 11,274 (56.8) | 4,733 (55.6) | |
Bilateral | 12,368 (43.6) | 8,588 (43.2) | 3,780 (44.4) | |
Histology | 0.876 | |||
Serous carcinoma | 18,538 (65.3) | 12,958 (65.2) | 5,580 (65.5) | |
Mucinous carcinoma | 2,285 (8.1) | 1,606 (8.1) | 679 (8.0) | |
Endometrioid carcinoma | 4,580 (16.1) | 3,203 (16.1) | 1,377 (16.2) | |
Clear cell carcinoma | 2,032 (7.2) | 1,432 (7.2) | 600 (7.0) | |
Sex cord-gonadal stromal tumor | 209 (0.7) | 154 (0.8) | 55 (0.6) | |
Germ cell tumor | 731 (2.6) | 509 (2.6) | 222 (2.6) | |
Grade | 0.907 | |||
Well differentiated | 3,491 (12.3) | 2,435 (12.3) | 1,056 (12.4) | |
Moderately differentiated | 5,526 (19.5) | 3,861 (19.4) | 1,665 (19.6) | |
Poorly differentiated | 12,127 (42.7) | 8,530 (42.9) | 3,597 (42.3) | |
Undifferentiated | 7,231 (25.5) | 5,036 (25.4) | 2,195 (25.8) | |
Stage | 0.857 | |||
I | 8,228 (29.0) | 5,752 (29.0) | 2,476 (29.1) | |
II | 2,875 (10.1) | 2,037 (10.3) | 838 (9.8) | |
III | 12,247 (43.2) | 8,533 (43) | 3,714 (43.6) | |
IV | 5,025 (17.7) | 3,540 (17.8) | 1,485 (17.4) | |
T stage | 0.889 | |||
T0 | 16 (0.1) | 12 (0.1) | 4 (0.0) | |
T1 | 8,707 (30.7) | 6,080 (30.6) | 2,627 (30.9) | |
T2 | 3,818 (13.5) | 2,703 (13.6) | 1,115 (13.1) | |
T3 | 15,834 (55.8) | 11,067 (55.7) | 4,767 (56.0) | |
N stage | 0.320 | |||
N0 | 21,674 (76.4) | 15,204 (76.5) | 6,470 (76.0) | |
N1 | 6,701 (23.6) | 4,658 (23.5) | 2,043 (24.0) | |
M stage | 0.588 | |||
M0 | 23,350 (82.3) | 16,322 (82.2) | 7,028 (82.6) | |
M1 | 5,025 (17.7) | 3,540 (17.8) | 1,485 (17.4) | |
Surgery | 0.551 | |||
Not performed | 490 (1.7) | 337 (1.7) | 153 (1.8) | |
Performed | 27,885 (98.3) | 19,525 (98.3) | 8,360 (98.2) | |
Radiotherapy | 0.553 | |||
Not performed | 28,061 (98.9) | 19,647 (98.9) | 8,414 (98.8) | |
Performed | 314 (1.1) | 215 (1.1) | 99 (1.2) | |
Chemotherapy | 0.297 | |||
No/unknown | 7,269 (25.6) | 5,053 (25.4) | 2,216 (26.0) | |
Performed | 21,106 (74.4) | 14,809 (74.6) | 6,297 (74.0) | |
Insurance status | 0.726 | |||
None or unknown | 7,237 (25.5) | 5,054 (25.4) | 2,183 (25.6) | |
Any | 21,138 (74.5) | 14,808 (74.6) | 6,330 (74.4) | |
Marital status | 0.011 | |||
Not married | 13,138 (46.3) | 9,294 (46.8) | 3,844 (45.2) | |
Married | 15,237 (53.7) | 10,568 (53.2) | 4,669 (54.8) |
Survival analysis
The median follow-up period was 38 (range, 17–71) months and overall 5-year survival rate was 55.5±0.3% (Figure 2). All 19,862 patients in the training cohort were subjected to univariate and multivariate analyses to determine predictors of survival (Table 2). Specific demographic data (race and age) significantly influenced patient prognosis (Figure 3). Clinicopathological factors, such as laterality, histology, grade and stage (AJCC), were additionally identified as risk factors influencing patient survival (Figure 4). Furthermore, TNM stage shows that tumor metastasis seriously affected the survival of patients with ovarian cancer (Figure S1). Survival outcomes differed in relation to the type of surgery, radiotherapy, and chemotherapy (Figure 5). Though patients with chemotherapy had better survival in a short time after being diagnosed, they had worse survival in a long time, perhaps due to the severe cancer condition of those patients. Finally, marital status had significant effects on survival in ovarian cancer, but insurance didn’t (Figure S2). Cox regression analysis was performed to further explore the effects of age, race, histology, stage, laterality, grade, surgery, chemotherapy, radiotherapy, and marital status. Each of the eleven factors was an independent risk factor for prognosis (P<0.001) (Table 2). All VIFs are far away from 10, indicating there are no multi-collinearity problem. The developed nomogram presented in Figure 6A is based on the significant risk factors identified using multivariate analyses for predicting 3- and 5-year OS. To calculate OS rates, we initially identified each factor based on the points scale at the top of the nomogram and subsequently summed the points of each factor. Finally, 3- and 5-year OS rates were obtained based on the bottom point scale of the nomogram. The calibration plots based on bootstrap resampling validation are illustrated in Figure 6B,C. The C-index of the nomogram was 0.752 (95% CI: 0.746–0.758).
Table 2
Characteristics | Univariate analyses | Multivariate analysis | VIF | |||
---|---|---|---|---|---|---|
HR (95% CI) | P | HR (95% CI) | P | |||
Total No. | ||||||
Race | <0.001 | <0.001 | 1.022 | |||
White | Reference | Reference | ||||
Black | 1.166 (1.073–1.267) | <0.001 | 1.190 (1.094–1.295) | <0.001 | ||
Others | 0.718 (0.662–0.779) | <0.001 | 0.904 (0.833–0.982) | 0.017 | ||
Age, years | <0.001 | <0.001 | 1.193 | |||
≤30 | Reference | Reference | ||||
31–40 | 2.204 (1.716–2.830) | <0.001 | 1.323 (1.014–1.726) | 0.039 | ||
41–50 | 3.260 (2.602–4.084) | <0.001 | 1.390 (1.086–1.778) | 0.009 | ||
51–60 | 4.154 (3.328–5.186) | <0.001 | 1.639 (1.284–2.093) | <0.001 | ||
61–70 | 5.651 (4.528–7.053) | <0.001 | 1.950 (1.528–2.490) | <0.001 | ||
71–80 | 7.917 (6.335–9.895) | <0.001 | 2.613 (2.044–3.341) | <0.001 | ||
>80 | 12.19 (9.688–15.33) | <0.001 | 4.326 (3.363–5.564) | <0.001 | ||
Laterality | <0.001 | <0.001 | 1.332 | |||
Unilateral | Reference | Reference | ||||
Bilateral | 2.112 (2.022–2.205) | <0.001 | 1.203 (1.148–1.261) | <0.001 | ||
Histology | <0.001 | <0.001 | 1.522 | |||
Serous carcinoma | Reference | Reference | ||||
Mucinous carcinoma | 0.403 (0.365–0.445) | <0.001 | 1.608 (1.432–1.804) | <0.001 | ||
Endometrioid carcinoma | 0.308 (0.285–0.332) | <0.001 | 0.835 (0.768–0.908) | <0.001 | ||
Clear cell carcinoma | 0.515 (0.467–0.567) | <0.001 | 1.439 (1.297–1.597) | <0.001 | ||
Sex cord-gonadal stromal tumor | 0.188 (0.121–0.292) | <0.001 | 0.785 (0.503–1.223) | 0.285 | ||
Germ cell tumor | 0.058 (0.039–0.088) | <0.001 | 0.285 (0.182–0.446) | <0.001 | ||
Grade | <0.001 | <0.001 | 1.296 | |||
Well differentiated | Reference | Reference | ||||
Moderately differentiated | 2.147 (1.920–2.400) | <0.001 | 1.571 (1.403–1.761) | <0.001 | ||
Poorly differentiated | 4.063 (3.671–4.498) | <0.001 | 1.750 (1.567–1.955) | <0.001 | ||
Undifferentiated | 3.940 (3.543–4.381) | <0.001 | 1.668 (1.486–1.874) | <0.001 | ||
Stage | <0.001 | <0.001 | 1.694 | |||
I | Reference | Reference | ||||
II | 2.238 (2.006–2.496) | <0.001 | 2.020 (1.801–2.265) | <0.001 | ||
III | 5.970 (5.533–6.442) | <0.001 | 5.168 (4.717–5.661) | <0.001 | ||
IV | 9.299 (8.572–10.088) | <0.001 | 7.649 (6.939–8.431) | <0.001 | ||
T stage | <0.001 | Not included | ||||
T0 | Reference | |||||
T1 | 0.136 (0.065–0.286) | 0.000 | ||||
T2 | 0.353 (0.168–0.743) | 0.066 | ||||
T3 | 0.855 (0.407–1.794) | 0.910 | ||||
N stage | <0.001 | Not included | ||||
N0 | Reference | |||||
N1 | 1.889 (1.804–1.979) | <0.001 | ||||
M stage | <0.001 | Not included | ||||
M0 | Reference | |||||
M1 | 2.737 (2.609–2.871) | <0.001 | ||||
Surgery | <0.001 | <0.001 | 1.022 | |||
Not performed | Reference | Reference | ||||
Performed | 0.188 (0.166–0.214) | <0.001 | 0.367 (0.322–0.417) | <0.001 | ||
Radiotherapy | 0.0021 | 0.001 | 1.004 | |||
Not performed | Reference | Reference | ||||
Performed | 1.355 (1.126–1.632) | 0.001 | 1.369 (1.136–1.650) | 0.001 | ||
Chemotherapy | <0.001 | <0.001 | 1.189 | |||
No/Unknown | Reference | Reference | ||||
Performed | 1.376 (1.305–1.449) | <0.001 | 0.746 (0.705–0.789) | <0.001 | ||
Insurance status | 0.146 | Not included | <0.001 | |||
None or unknown | Reference | |||||
Any | 0.966 (0.922–1.012) | 0.146 | ||||
Marital status | <0.001 | <0.001 | 1.015 | |||
Not married | Reference | Reference | ||||
Married | 0.892 (0.854–0.931) | <0.001 | 0.878 (0.84–0.917) | <0.001 |
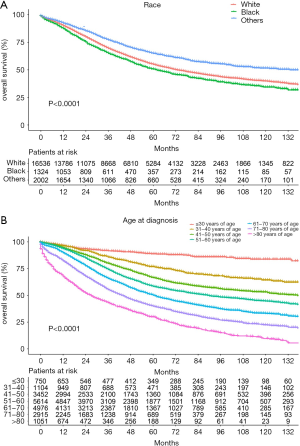
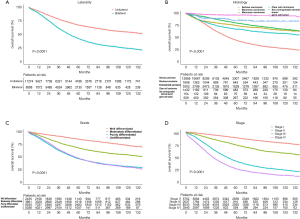
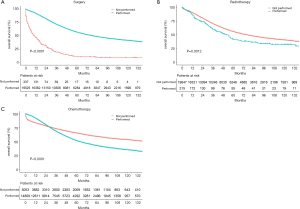
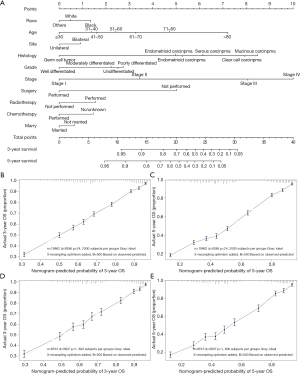
We further validated the nomogram using the data of the validation cohort. The calibration plots based on bootstrap resampling validation are illustrated in Figure 6D,E. The C-index was 0.755 (95% CI: 0.746–0.764), indicating good agreement between the nomogram and actual observation for predicting 3- and 5-year OS rates of patients with ovarian cancer.
In general, the OS rates were better for younger patients and poorer for black women. Advanced stage, paired site and high grade had a negative influence on OS. In terms of histological subtype, the germ cell tumor type was associated with best prognosis in general, followed by endometrial carcinoma, sex cord-gonadal stromal tumor, serous carcinoma, clear cell carcinoma, and mucinous carcinoma. Survival was superior in patients who underwent surgery and chemotherapy and inferior in those subjected to radiotherapy. With the aid of the newly developed nomogram, it was possible to effectively predict prognosis according to individual patient characteristics.
Discussion
Despite considerable progress in the development of both surgical procedures and novel medicines, the overall survival rates of ovarian cancer patients remain extremely low. International Federation of Gynecology and Obstetrics stage and residual tumor after debulking surgery are the most widely reported prognostic factors (27), but are insufficient for effective prognosis. A nomogram, commonly used in clinical oncology, is a convenient tool that quantifies risk by incorporating and illustrating the relative importance of various prognostic factors (28). The current study used data from more than 20,000 cases of ovarian cancer for developing a nomogram to predict the 3- and 5-year OS rates based on 10 significant factors (age, race, histology, stage, laterality, grade, surgery, chemotherapy, radiotherapy, and marital status) with the aim of effectively predicting prognosis according to specific characteristics. To our knowledge, no other researchers to date have conducted a comprehensive nomogram analysis based on the SEER database for predicting outcomes in ovarian cancer patients. The discrimination performance of the nomogram was evaluated using an internal bootstrap resampling method. The C-index demonstrated the capability of the nomogram to predict 3- and 5-year OS rates of patients with ovarian cancer.
Platinum/taxanes therapy following cytoreductive surgery is the standard therapeutic strategy for advanced ovarian cancer. Radiotherapy was largely discontinued after the introduction of platinum-based chemotherapy. In our analysis, radiotherapy was associated with poor prognosis in ovarian cancer, similar to the earlier findings of Patel et al. (29) who analyzed OS for clear cell, mucinous, and endometrioid histologies of stage I–III ovarian cancer from the SEER Program between 2004 and 2011. Patients receiving radiation therapy had lower cause-specific survival and ovarian cancer at 5 and 10 years. However, a number of studies have demonstrated the essential utility of radiotherapy as a feasible treatment modality for patients with persistent recurrent epithelial ovarian cancer (30), which indicated that radiotherapy is irreplaceable still and needed to be studied furthermore.
Marital status has recently been established as an independent predictor of survival in gastric cancer (17), colorectal cancer (31) and several other tumors. Determination of the relationship between marital status and survival in ovarian cancer would be beneficial for decision making by researchers, physicians as well as policy makers to improve the mortality rate. Our data showed that unmarried ovarian cancer patients (including widowed, single, divorced, and separated samples or those with domestic partners) generally have poorer prognosis although the marital status may have changed for some patients during the course of study.
The SEER database has provided the opportunity to perform large, population-based studies for many tumor types, such as laryngeal squamous cell carcinoma (32), malignant pleural mesothelioma (33), lung cancer (34) and brain cancer (35). However, several vital limitations require addressing and the results should be interpreted with caution. Firstly, our nomogram isn’t validated by the data of our own department or other databases, due to the lack of another large cohort. Secondly, the current study is a retrospective design and a larger randomized controlled trial may be required to validate our findings. Thirdly, the C-index of the nomogram is not entirely reliable. Several other factors additionally influence prognosis, such as family history and general health. Meanwhile, the follow-up period for some patients was extremely long and some factors may have changed over this time-period, such as marital status. Further systematic analyses are therefore required to improve the predictive accuracy of the nomogram.
Despite the obvious limitations of our study, the data clearly indicate that age, race, histology, stage, laterality, grade, surgery, chemotherapy, radiotherapy, and marital status are independent risk factors for survival of patients with ovarian cancer The nomogram developed could accurately predict the 3- and 5-year OS rates of our patient sample according to individual characteristics.
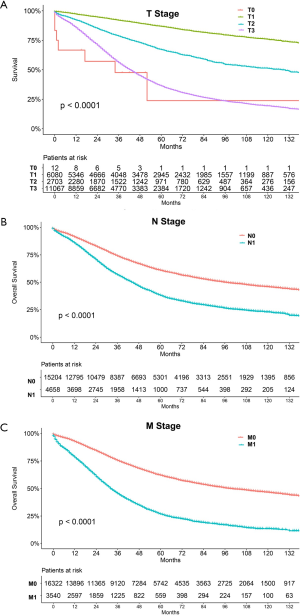
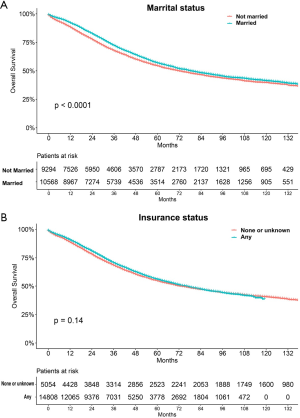
Acknowledgments
We thank our anonymous reviewers for their valuable comments, which have led to many improvements to our article.
Funding: This work was supported by
Footnote
Reporting Checklist: The authors have completed the STROBE reporting checklist. Available at http://dx.doi.org/10.21037/tcr-20-1238
Peer Review File: Available at http://dx.doi.org/10.21037/tcr-20-1238
Conflicts of Interest: All authors have completed the ICMJE uniform disclosure form (available at http://dx.doi.org/10.21037/tcr-20-1238). The authors have no conflicts of interest to declare.
Ethical Statement: The authors are accountable for all aspects of the work in ensuring that questions related to the accuracy or integrity of any part of the work are appropriately investigated and resolved. The study was conducted in accordance with the Declaration of Helsinki (as revised in 2013). Data of ovarian cancer were obtained from SEER database.
Open Access Statement: This is an Open Access article distributed in accordance with the Creative Commons Attribution-NonCommercial-NoDerivs 4.0 International License (CC BY-NC-ND 4.0), which permits the non-commercial replication and distribution of the article with the strict proviso that no changes or edits are made and the original work is properly cited (including links to both the formal publication through the relevant DOI and the license). See: https://creativecommons.org/licenses/by-nc-nd/4.0/.
References
- Siegel RL, Miller KD, Jemal A. Cancer Statistics, 2020. CA Cancer J Clin 2020;70:7-30. [Crossref] [PubMed]
- Lim W, Ryu S, Bazer FW, et al. Chrysin attenuates progression of ovarian cancer cells by regulating signaling cascades and mitochondrial dysfunction. J Cell Physiol 2018;233:3129-40. [Crossref] [PubMed]
- Mirza MR, Avall LE, Birrer MJ, et al. Niraparib plus bevacizumab versus niraparib alone for platinum-sensitive recurrent ovarian cancer (NSGO-AVANOVA2/ENGOT-ov24): a randomised, phase 2, superiority trial. Lancet Oncol 2019;20:1409-19. [Crossref] [PubMed]
- Ferlay J, Colombet M, Soerjomataram I, et al. Cancer incidence and mortality patterns in Europe: Estimates for 40 countries and 25 major cancers in 2018. Eur J Cancer 2018;103:356-87. [Crossref] [PubMed]
- Al-Niaimi AN, Ahmed M, Petersen CB. Epithelial ovarian cancer. Obstet Gynecol Clin North Am 2012;39:269-83. [Crossref] [PubMed]
- Shan W, Liu J. Epithelial ovarian cancer: focus on genetics and animal models. Cell Cycle 2009;8:731-5. [Crossref] [PubMed]
- Rosen DG, Yang G, Liu G, et al. Ovarian cancer: pathology, biology, and disease models. Front Biosci (Landmark Ed) 2009;14:2089-102. [Crossref] [PubMed]
- Feng W, Dean DC, Hornicek FJ, et al. Exosomes promote pre-metastatic niche formation in ovarian cancer. Mol Cancer 2019;18:124. [Crossref] [PubMed]
- Hanker LC, Loibl S, Burchardi N, et al. The impact of second to sixth line therapy on survival of relapsed ovarian cancer after primary taxane/platinum-based therapy. Ann Oncol 2012;23:2605-12. [Crossref] [PubMed]
- Jung JG, Shih IM, Park JT, et al. Ovarian Cancer Chemoresistance Relies on the Stem Cell Reprogramming Factor PBX1. Cancer Res 2016;76:6351-61. [Crossref] [PubMed]
- Maringe C, Walters S, Butler J, et al. Stage at diagnosis and ovarian cancer survival: evidence from the International Cancer Benchmarking Partnership. Gynecol Oncol 2012;127:75-82. [Crossref] [PubMed]
- Kurman RJ, Shih I. The Dualistic Model of Ovarian Carcinogenesis: Revisited, Revised, and Expanded. Am J Pathol 2016;186:733-47. [Crossref] [PubMed]
- Balachandran VP, Gonen M, Smith JJ, DeMatteo RP. Nomograms in oncology: more than meets the eye. Lancet Oncol 2015;16:e173-80. [Crossref] [PubMed]
- Iasonos A, Schrag D, Raj GV, et al. How to build and interpret a nomogram for cancer prognosis. J Clin Oncol 2008;26:1364-70. [Crossref] [PubMed]
- Wang F, Zhang H, Wen J, et al. Nomograms forecasting long-term overall and cancer-specific survival of patients with oral squamous cell carcinoma. Cancer Med 2018;7:943-52. [Crossref] [PubMed]
- Li J, Liu Y, Yan Z, et al. A nomogram predicting pulmonary metastasis of hepatocellular carcinoma following partial hepatectomy. Br J Cancer 2014;110:1110-7. [Crossref] [PubMed]
- Yuan SQ, Wu WJ, Qiu MZ, et al. Development and Validation of a Nomogram to Predict the Benefit of Adjuvant Radiotherapy for Patients with Resected Gastric Cancer. J Cancer 2017;8:3498-505. [Crossref] [PubMed]
- Cho JK, Lee GJ, Yi KI, et al. Development and external validation of nomograms predictive of response to radiation therapy and overall survival in nasopharyngeal cancer patients. Eur J Cancer 2015;51:1303-11. [Crossref] [PubMed]
- Wen J, Ye F, He X, et al. Development and validation of a prognostic nomogram based on the log odds of positive lymph nodes (LODDS) for breast cancer. Oncotarget 2016;7:21046-53. [Crossref] [PubMed]
- Huang Y, Yang X, Lu T, et al. Assessment of the prognostic factors in patients with pulmonary carcinoid tumor: a population-based study. Cancer Med 2018;7:2434-41. [Crossref] [PubMed]
- Warren JL, Klabunde CN, Schrag D, et al. Overview of the SEER-Medicare data: content, research applications, and generalizability to the United States elderly population. Med Care 2002;40:IV-3-18. [Crossref] [PubMed]
- Sun F, Ma K, Yang X, et al. A nomogram to predict prognosis after surgery in early stage non-small cell lung cancer in elderly patients. Int J Surg 2017;42:11-6. [Crossref] [PubMed]
- Koay EJ, Teh BS, Paulino AC, et al. A Surveillance, Epidemiology, and End Results analysis of small cell carcinoma of the bladder: epidemiology, prognostic variables, and treatment trends. Cancer 2011;117:5325-33. [Crossref] [PubMed]
- Khan HM, Gabbidon K, Saxena A, et al. Disparities in Cervical Cancer Characteristics and Survival Between White Hispanics and White Non-Hispanic Women. J Womens Health (Larchmt) 2016;25:1052-8. [Crossref] [PubMed]
- Lau CSM, Zywot A, Mahendraraj K, et al. Gallbladder Carcinoma in the United States: A Population Based Clinical Outcomes Study Involving 22,343 Patients from the Surveillance, Epidemiology, and End Result Database (1973-2013). HPB Surg 2017;2017:1532835. [Crossref] [PubMed]
- Lim YJ, Song C, Kim JS. Improved survival with postoperative radiotherapy in thymic carcinoma: A propensity-matched analysis of Surveillance, Epidemiology, and End Results (SEER) database. Lung Cancer 2017;108:161-7. [Crossref] [PubMed]
- Escayola C, Torrent JJ, Ferron G, et al. When and Who Should Perform Epithelial Ovarian Cancer Surgery? Int J Gynecol Cancer 2018;28:594-9. [Crossref] [PubMed]
- Wang JY, Zhu Y, Wang CF, et al. A nomogram to predict Gleason sum upgrading of clinically diagnosed localized prostate cancer among Chinese patients. Chin J Cancer 2014;33:241-8. [Crossref] [PubMed]
- Patel SC, Frandsen J, Bhatia S, et al. Impact on survival with adjuvant radiotherapy for clear cell, mucinous, and endometriod ovarian cancer: the SEER experience from 2004 to 2011. J Gynecol Oncol 2016;27:e45. [Crossref] [PubMed]
- Choi N, Chang JH, Kim S, et al. Radiation for persistent or recurrent epithelial ovarian cancer: a need for reassessment. Radiat Oncol J 2017;35:144-52. [Crossref] [PubMed]
- Wang L, Wilson SE, Stewart DB, et al. Marital status and colon cancer outcomes in US Surveillance, Epidemiology and End Results registries: does marriage affect cancer survival by gender and stage? Cancer Epidemiol 2011;35:417-22. [Crossref] [PubMed]
- Zhan C, Yang X, Song X, et al. Radiotherapy vs surgery for T1-2N0M0 laryngeal squamous cell carcinoma: A population-based and propensity score matching study. Cancer Med 2018;7:2837-47. [Crossref] [PubMed]
- Wang S, Ma K, Chen Z, et al. A Nomogram to Predict Prognosis in Malignant Pleural Mesothelioma. World J Surg 2018;42:2134-42. [Crossref] [PubMed]
- Liang W, He J, Shen Y, et al. Impact of Examined Lymph Node Count on Precise Staging and Long-Term Survival of Resected Non-Small-Cell Lung Cancer: A Population Study of the US SEER Database and a Chinese Multi-Institutional Registry. J Clin Oncol 2017;35:1162-70. [Crossref] [PubMed]
- Bytnar JA, Lin J, Shriver CD, et al. Racial differences in brain cancer characteristics and survival: an analysis of SEER data. Cancer Causes Control 2019;30:1283-91. [Crossref] [PubMed]