Identification of a 7-mRNA signature as a prognostic biomarker in pediatric osteosarcoma
Introduction
Osteosarcoma (OS) is the most common primary bone cancer. The global incidence is 0.2–3/100,000 per year for the general population and is 0.8–11/100,000 for people aged 15–19 years (1,2). OS is common among children, and has a high malignancy, disability, and recurrence rate, along with a poor prognosis. The incidence of OS generally increases with age. Although chemotherapy has increased the 3-year survival rate from 20% to 60–70% (3), there has been no significant progress in targeted OS treatment in the past few decades. Therefore, a further understanding of the genetic etiology of OS is required for progress to be made in treating this cancer.
Currently, a number of molecules have become therapeutic targets for certain cancers. For example, transferrin receptor 1 (TFR1), a member of the TFR family, is a membrane protein that regulates iron input (4,5). The uptake of iron by TFRs is important for cancer cells to absorb iron. There is increasing evidence that TFR1 is involved in the development of tumors, and its expression is significantly dysregulated in many cancers (6,7). The relationship between TFR1 and cancer has been extensively studied, and TFR1 is considered a valuable drug target for cancer intervention (8-11). Angiogenesis are regulated by the vascular endothelial growth factor (VEGF)and its receptor (VEGFR) signaling pathway and play a key role in tumor growth and metastasis. The selective inhibition of VEGFR kinase has been explored as a successful clinical cancer treatment strategy. Many VEGFR inhibitors have already been approved for clinical use, and many more are in various stages of development (12). Cyclin-dependent protein kinase 9 (CDK9) has been shown to play an important role in the pathogenesis of malignant tumors. A recent study demonstrated that high CDK9 expression was associated with significantly shorter survival in patients with OS following immunohistochemistry. This suggests that a high expression of CDK9 is an independent predictor of poor prognosis in patients with OS. It also indicates that CDK9 is a new prognostic marker and a promising therapeutic target for OS (13).
At present, there are no well-established prognostic markers for pediatric patients with OS. Individual heterogeneity makes the tumor-node-metastasis (TNM) staging system clinically ineffective for the prognosis of OS. Although the alkaline phosphatase tumor biomarker has been used to predict OS (14), and gene modules have been found to be associated with OS (15-18), to the best of our knowledge, there have been no previously published studies specifically focused on the prognosis of OS in pediatric patients. Studies have shown that mRNA plays a very important role in the development of pediatric OS (19-22), which indicates that mRNAs may be used as a prognostic marker in this disease.
The purpose of the present study was to evaluate the use of mRNAs as a prognostic marker of OS in pediatric patients by analyzing the expression of mRNAs available from the Therapeutically Available Research to Generate Effective Treatments (TARGET) OS database.
We present the following article in accordance with the MDAR reporting checklist (available at http://dx.doi.org/10.21037/tcr-20-2407).
Methods
Extraction of TARGET pediatric OS data
mRNA sequencing data and corresponding clinical target data were downloaded from the TARGET database (portal.gdc.cancer.gov/). Because the data are standardized, no further processing was required, and no data were deleted. There were 101 cases of gating in the database, including 0 normal samples and 101 OS samples. There were 39 females and 62 males, ranging in age from 4 to 23 years, with an average age of 16 years. Differential expression analysis was performed on level 3RNA sequencing data of OS tissues using the edgeR package based on R language. Genes with absolute log2 fold change >1 and P<0.05 in mRNA expression levels were considered to be differentially expressed. The study was conducted in accordance with the Declaration of Helsinki (as revised in 2013). Because the data are from a public database, no ethics committee approval was required.
Survival analysis
The mRNA expression data of pediatric patients with OS combined with clinical data from the TARGET database were used to determine the differential expression of mRNA signals that affect prognosis. The survival curve of the samples with differential mRNA expression was drawn with the Kaplan-Meier plot, and the total survival rate was determined. Univariate and multivariate Cox analyses were performed to calculate the risk ratio and P values of all differentially expressed mRNAs. The sensitivity and specificity of the risk score in predicting the overall survival rate of pediatric patients with OS were assessed based on the area under the curve (AUC) of the receiver-operating characteristic (ROC) analysis.
Pathway analysis
Pathway analyses were performed among the survival-related mRNAs from the univariate Cox analysis using Kyoto Encyclopedia of Genes and Genomes (KEGG) (http://www.genome.jp/kegg/pathway.html) pathway databases.
Statistical analysis
Pediatric patients with OS tumors were allocated to high- and low-expression groups according to the median expression level of each differentially expressed mRNA. Overall survival was determined using the Kaplan-Meier survival analysis. The difference in survival of the pediatric patients was determined using the log-rank test with the survival analysis in R package. A P value <0.05 was considered to be statistically significant.
Results
Single mRNA species were related to survival in pediatric patients with OS
A total of 19,496 mRNA species were found to be differentially expressed, including 104 downregulated mRNAs and 267 upregulated mRNAs. Kaplan-Meier and Cox P value survival analyses of the deferentially expressed mRNA species indicated that 33 genes with differential mRNA expression were significantly related to survival (P<0.05) (Table 1).
Table 1
Gene | Kaplan-Meier value | HR | HR.95L | HR.95H | Cox P value |
---|---|---|---|---|---|
MUC17 | 0.024729 | 1.000261 | 1.00006 | 1.000463 | 0.011048 |
SCGB1A1 | 0.002324 | 1.000069 | 1.000009 | 1.000129 | 0.023514 |
KRT83 | 0.005254 | 1.007786 | 1.00261 | 1.012988 | 0.003153 |
CFAP57 | 0.043358 | 1.007527 | 1.00099 | 1.014106 | 0.023945 |
SFTA3 | 0.014252 | 1.001505 | 1.000187 | 1.002826 | 0.025206 |
ADRB3 | 0.028427 | 1.003499 | 1.000898 | 1.006108 | 0.008347 |
AGR3 | 0.006579 | 1.002917 | 1.000391 | 1.005449 | 0.023561 |
RP11-598P20.5 | 0.034737 | 1.001679 | 1.000369 | 1.002991 | 0.011985 |
CYP4F8 | 0.037341 | 1.000538 | 1.000086 | 1.00099 | 0.019618 |
ALDH1A1 | 0.004767 | 1.000117 | 1.000041 | 1.000194 | 0.002573 |
TNXB | 0.03894 | 1.000203 | 1.000056 | 1.000351 | 0.006823 |
GABRB3 | 0.039065 | 1.000312 | 1.000038 | 1.000587 | 0.025833 |
CFAP73 | 0.006869 | 1.003911 | 1.000594 | 1.007239 | 0.020798 |
MYOC | 0.011729 | 1.005669 | 1.001098 | 1.01026 | 0.015002 |
RAB25 | 0.021731 | 1.004769 | 1.000384 | 1.009173 | 0.033002 |
C19orf33 | 0.039076 | 1.003781 | 1.000497 | 1.007076 | 0.023996 |
IGF2 | 0.00266 | 0.999955 | 0.999915 | 0.999996 | 0.029667 |
CLEC4M | 0.016707 | 1.004171 | 1.000466 | 1.00789 | 0.027301 |
HP | 0.038545 | 1.002115 | 1.000463 | 1.003771 | 0.012093 |
GABRA5 | 0.047178 | 1.001137 | 1.000161 | 1.002113 | 0.022432 |
TRIM49C | 0.017894 | 1.002371 | 1.000414 | 1.004332 | 0.017556 |
MEIS3 | 0.019896 | 0.997913 | 0.99598 | 0.999849 | 0.034657 |
MYC | 0.024538 | 1.000058 | 1.000026 | 1.000089 | 0.000315 |
TNFRSF21 | 0.004399 | 0.999503 | 0.999153 | 0.999853 | 0.00539 |
ACKR4 | 0.024598 | 1.007749 | 1.001252 | 1.014288 | 0.019331 |
HERC5 | 0.000518 | 0.997795 | 0.996052 | 0.999542 | 0.013366 |
TRIM16L | 0.007439 | 0.996127 | 0.993405 | 0.998857 | 0.00545 |
SYT2 | 0.042662 | 0.994201 | 0.988901 | 0.999528 | 0.032928 |
ST8SIA6 | 0.007765 | 0.980774 | 0.964442 | 0.997383 | 0.023464 |
HSD11B1 | 0.021879 | 1.012085 | 1.00466 | 1.019566 | 0.001387 |
DRD2 | 0.026452 | 0.983503 | 0.967999 | 0.999256 | 0.040187 |
NDST3 | 0.009417 | 0.988577 | 0.979798 | 0.997434 | 0.011583 |
UNC5A | 0.032069 | 1.001864 | 1.000307 | 1.003423 | 0.018925 |
MUC4 | 0.023129 | 1.00491 | 1.001114 | 1.00872 | 0.011196 |
TRABD2A | 0.019783 | 0.99763 | 0.995713 | 0.999551 | 0.015626 |
EFHC2 | 0.001406 | 0.991072 | 0.984883 | 0.997299 | 0.005012 |
ZNF488 | 0.008545 | 0.975283 | 0.95449 | 0.996528 | 0.022827 |
GDNF | 0.013354 | 1.00078 | 1.000016 | 1.001544 | 0.045517 |
A group of mRNA species was closely related to survival in pediatric patients with OS
Univariate Cox analysis was performed on all differentially expressed mRNAs, and 22 potential candidates were selected (Table 2). Multivariate Cox analysis was then performed on the candidate mRNA species to determine the mRNA species closely related to survival, with a cutoff threshold of significance set at 0.01 (P<0.01). Seven mRNA species were identified (SCGB3A1, MUC17, ADH1B, KRT83, RP1-37E16.12, FIGF, and SFTPD). The independent prognostic values of these seven hub mRNAs were generated by multivariate Cox analysis (Table 3). Five mRNAs were related to high mortality among pediatric patients with OS, including SCGB3A1, MUC17, ADH1B, KRT83, and RP1-37E16.12. The risk mortality was 63% higher in patients with a high expression of KRT83 than in patients with a low KRT83 expression; the risk of mortality was 52% higher in patients with a high expression of ADH1B than in patients with a low ADH1B expression. FIGF and SFTPD were associated with a low risk of mortality in pediatric patients with OS.
Table 2
Gene | HR | Z | P value |
---|---|---|---|
SCGB3A1 | 1.060302 | 0.547207 | 0.000424 |
FIGF | 0.982092 | –0.1604 | 0.000785 |
INMT | 1.236569 | 2.016269 | 0.001369 |
SLC34A2 | 1.124608 | 1.184404 | 0.001438 |
PGC | 1.16949 | 1.520409 | 0.001506 |
MUC17 | 1.189722 | 2.081223 | 0.002025 |
SFTPB | 1.115756 | 1.345639 | 0.00209 |
SCGB1A1 | 1.188812 | 2.662658 | 0.002559 |
TCF21 | 1.116229 | 1.238056 | 0.002667 |
NAPSA | 1.024285 | 0.209626 | 0.003016 |
ADAMTS8 | 1.07829 | 0.748342 | 0.003384 |
SFTPA2 | 1.0807 | 1.009918 | 0.005021 |
ADH1B | 1.213052 | 2.400327 | 0.005168 |
KRT83 | 1.453494 | 3.173515 | 0.005172 |
RP1-37E16.12 | 1.282076 | 2.675284 | 0.005424 |
HPSE2 | 1.187637 | 1.876842 | 0.006284 |
SFTPD | 0.853388 | –1.2376 | 0.006428 |
MAP1LC3C | 1.16887 | 1.191472 | 0.007302 |
PTGER1 | 1.222665 | 2.059195 | 0.007467 |
SCGB3A2 | 1.284029 | 2.292198 | 0.007753 |
SFTPC | 1.08178 | 1.140528 | 0.008273 |
C4BPA | 1.142128 | 1.43618 | 0.008797 |
CXCL17 | 1.045608 | 0.354362 | 0.009551 |
Table 3
ID | Coef. | Exp(coef.) | Se(coef.) | Z | Pr(>|z|) |
---|---|---|---|---|---|
SCGB3A1 | 0.242257 | 1.274122 | 0.136497 | 1.774825 | 0.075927 |
FIGF | –0.2991 | 0.741483 | 0.15569 | –1.92115 | 0.054713 |
MUC17 | 0.168999 | 1.184118 | 0.086462 | 1.954604 | 0.05063 |
ADH1B | 0.415514 | 1.515149 | 0.128483 | 3.234008 | 0.001221 |
KRT83 | 0.488864 | 1.630463 | 0.134531 | 3.633843 | 0.000279 |
RP1-37E16.12 | 0.360864 | 1.434568 | 0.113838 | 3.169984 | 0.001524 |
SFTPD | –0.39576 | 0.673166 | 0.163477 | –2.42091 | 0.015482 |
Pathways involved in survival
Through Reactome pathway analysis, six enriched pathways that were most likely involved in the survival of pediatric patients with OS were identified (Table 4): glycolysis/gluconeogenesis, arachidonic acid metabolism, cytokine-cytokine receptor interaction, neuroactive ligand-receptor interaction, tight junction, and complement and coagulation cascades.
Table 4
Category | Term | Count | P value |
---|---|---|---|
KEGG_PATHWAY | Glycolysis/Gluconeogenesis | 5 | 3.3E–2 |
KEGG_PATHWAY | Arachidonic acid metabolism | 5 | 2.4E–2 |
KEGG_PATHWAY | Cytokine-cytokine receptor interaction | 11 | 1.3E–2 |
KEGG_PATHWAY | Neuroactive ligand-receptor interaction | 13 | 4.3E–3 |
KEGG_PATHWAY | Tight junction | 5 | 7.3E–2 |
KEGG_PATHWAY | Complement and coagulation cascades | 5 | 3.6E–2 |
Seven-mRNA prognostic model
For each patient, a risk score was generated from the independent prognostic values of the seven mRNAs using the following formula: risk score = 0.242257 × SCGB3A1 + 0.168999 × MUC17 + 0.415514 × ADH1B + 0.488864 × KRT83 + 0.360864 × RP1-37E16.12 – 0.2991 × FIGF – 0.39576 × SFTPD. The distribution of patients with different survival risk scores of these seven mRNAs and mRNA-related survival time are shown in Figure 1A,B, respectively. The expression heatmap of the 7-mRNA signature is shown in Figure 1C. Pediatric patients with OS were assigned to low- and high-risk groups according to the risk scores (Figure 1D). Log-rank test indicated that pediatric patients with OS in the low-risk group had significantly longer survival (P<0.05) than patients in the high-risk group (Figure 1D). The area under the ROC curve was 0.858 (Figure 2). These findings indicate that the 7-mRNA prognostic model is promising, sensitive, and specific in predicting the survival outcomes of pediatric patients with OS.
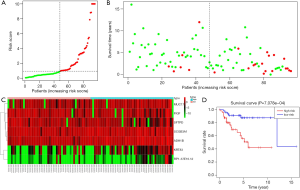
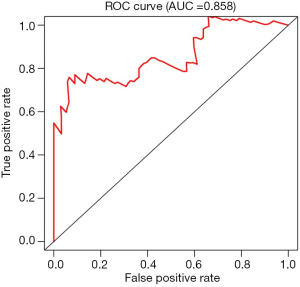
Discussion
In the present study, a 7-mRNA model was proposed to predict the prognosis of pediatric patients with OS. Of the genes with differential mRNA expression in the TARGET database, seven mRNA species (SCGB3A1, MUC17, ADH1B, KRT83, RP1-37E16.12, FIGF, and SFTPD) were found to be closely related to the survival of pediatric patients with OS. The combination of the seven mRNA species could sensitively and specifically predict survival outcomes in pediatric patients with OS. Further study and confirmation of this 7-mRNA model is required in the future to predict the prognosis of pediatric patients with OS.
The abnormal transcription process is one of the factors influencing the development of OS. Activated protein 1 complex (AP-1) is composed of fos and jun proteins, which are the products of c-fos and c-jun proto oncogenes, respectively. AP-1controls cell proliferation, differentiation, and bone metabolism (23-26). Leaner et al. recently found that AP-1-mediated transcriptional inhibition leads to a reduction of migration, invasion, and metastasis in OS mouse models (27). When delivered via nanoparticles, the enzyme dz13 cleaves human c-jun mRNA and inhibits the growth and progression of OS in mouse models (28). Myc is a transcription factor, and its amplification is related to the pathogenesis of OS and chemotherapy resistance (29). The overexpression of myc in bone marrow stromal cells leads to OS and fat loss. Myc has been found to be amplified in the U2OS cell line; it has high resistance to adriamycin, and is increased in the Saos-2 methotrexate-resistant cell line (30). In addition, myc is considered to be the target treatment of OS. The downregulation of myc enhances the therapeutic effect of methotrexate on OS cells (31). These findings suggest that mRNAs, especially mRNAs resulting from abnormal transcription processes, may be good markers for the prognosis of OS.
In the present study, SCGB3A1, MUC17, ADH1B, KRT83, RP1-37E16.12, FIGF, and SFTPD mRNA species were found to be highly related to the survival of pediatric patients with OS. MUC17 encodes mucin17, which functions in epithelial cells to provide cytoprotection and signal transduction, maintain luminal structure and homeostasis of the mucosal surface, and confer anti-adhesive properties to cancer cells that lose their apical/basal polarization (32). ADH1B encodes alcohol dehydrogenase 1B (class I), β polypeptide [which metabolizes a wide variety of substrates, including ethanol (alcohol beverage)], retinol, other aliphatic alcohols, hydroxysteroids, and lipid peroxidation products (33). SFTPD encodes pulmonary surfactant protein D, which is an innate immune system collect in (34). SCGB3A1 is a type of secretory immunoglobulin, and its biologic functions are mainly unknown (35). KRT83 is a hair keratin (36). FIGF or c-fos-induced growth factor is a VEGF that promotes the metastasis of cancers to lymph nodes (37). RP1-37e16.12 is a filamentous actin-binding protein that is associated with the guanine nucleotide exchange factor and regulates actin cytoskeleton organization (38). Although the roles of these genes in OS progression are not well understood, the KEGG pathway databases indicate that they are related to neuroactive ligand-receptor interaction, arachidonic acid metabolism, cytokine-cytokine receptor interaction, tight junction, and complement and coagulation cascade signaling pathways. The neuroactive ligand-receptor interaction pathway is related to OS tumorigenesis and poor prognosis (39,40). Although arachidonic acid is not carcinogenic (40-43), arachidonic acid can be converted into prostaglandin E2 and other prostaglandins by cyclooxygenase-2 (44), which plays a role in regulating the migratory and invasive behavior of cells during the development and progression of cancer (16,45). Abnormality in cytokine-cytokine receptor interaction is related to OS genesis and poor prognosis (46,47). Abnormality in tight junction promotes a malignant phenotype of OS cells and causes poor prognosis (48-50). Imbalanced complement and coagulation interaction promote tumor growth (51,52). These findings indicate that the seven identified mRNA species have the potential to be used as prognostic markers in pediatric patients with OS. Multivariate Cox analysis-generated independent prognostic values indicated that SCGB3A1, MUC17, ADH1B, KRT83, and RP1-37E16.12 are related to high mortality in pediatric patients with OS, and FIGF and SFTPD are associated with a low mortality rate. In particular, the high expression of KRT83 and ADH1B led to higher risk of mortality in these patients. These findings further suggest that these mRNA species might be able to serve as prognostic markers. The prognostic model using the sum of the independent prognostic values of the seven mRNA species could be used as prognostic markers among pediatric patients with OS, with high sensitivity and specificity.
Many approaches have the potential to improve the prognosis of OS patients, including immunotherapy in activating monocytes and macrophages against OS cells (53-56), the inhibition of the mammalian target of rapamycin-mediated signal transduction pathway (57-60), the inhibition of tyrosine kinases (61-63), and the use of novel antifolates. Combination target therapy may have the greatest potential for improvement in outcomes. However, whether this improvement in outcomes among OS patients is achieved by these approaches correlates with the 7-mRNA species predictive value requires further investigation.
The predictive value of the 7-mRNA model was not validated in the present study. Therefore, a validation study using OS tissues from pediatric patients is warranted.
Conclusions
Seven mRNA species were found to be closely related to overall survival in pediatric patients with OS. A prognostic model using a combination of these 7-mRNA species has the potential to predict the prognosis of pediatric patients with OS; however, this requires further verification.
Acknowledgments
The authors would like to thank Profs. Hui Wang and Zhixia Sun for helpful discussions and kind support.
Funding: None.
Footnote
Reporting Checklist: The authors have completed the MDAR reporting checklist. Available at http://dx.doi.org/10.21037/tcr-20-2407
Conflicts of Interest: All authors have completed the ICMJE uniform disclosure form (available at http://dx.doi.org/10.21037/tcr-20-2407). The authors have no conflicts of interest to declare.
Ethical Statement: The authors are accountable for all aspects of the work in ensuring that questions related to the accuracy or integrity of any part of the work are appropriately investigated and resolved. The study was conducted in accordance with the Declaration of Helsinki (as revised in 2013).
Open Access Statement: This is an Open Access article distributed in accordance with the Creative Commons Attribution-NonCommercial-NoDerivs 4.0 International License (CC BY-NC-ND 4.0), which permits the non-commercial replication and distribution of the article with the strict proviso that no changes or edits are made and the original work is properly cited (including links to both the formal publication through the relevant DOI and the license). See: https://creativecommons.org/licenses/by-nc-nd/4.0/.
References
- Bielack S, Carrle D, Casali PG. Osteosarcoma: ESMO clinical recommendations for diagnosis, treatment and follow-up. Ann Oncol 2009;20:137-9. [Crossref] [PubMed]
- Mirabello L, Troisi RJ, Savage SA. Osteosarcoma incidence and survival rates from 1973 to 2004: data from the surveillance, epidemiology, and end results program. Cancer 2009;115:1531-43. [Crossref] [PubMed]
- van Oosterwijk JG, Anninga JK, Gelderblom H, et al. Update on targets and novel treatment options for high-grade osteosarcoma and chondrosarcoma. Hematol Oncol Clin North Am 2013;27:1021-48. [Crossref] [PubMed]
- Zanganeh S, Hutter G, Spitler R, et al. Iron oxide nanoparticles inhibit tumour growth by inducing pro-inflammatory macrophage polarization in tumour tissues. Nat Nanotechnol 2016;11:986-94. [Crossref] [PubMed]
- Kim SE, Zhang L, Ma K, et al. Ultrasmall nanoparticles induce ferroptosis in nutrient-deprived cancer cells and suppress tumour growth. Nat Nanotechnol 2016;11:977-85. [Crossref] [PubMed]
- Jeong SM, Hwang S, Seong RH. Transferrin receptor regulates pancreatic cancer growth by modulating mitochondrial respiration and ROS generation. Biochem Biophys Res Commun 2016;471:373-9. [Crossref] [PubMed]
- Buas MF, Rho JH, Chai X, et al. Candidate early detection protein biomarkers for ER+/PR+ invasive ductal breast carcinoma identified using pre-clinical plasma from the WHI observational study. Breast Cancer Res Treat 2015;153:445-54. [Crossref] [PubMed]
- Senyilmaz D, Virtue S, Xu X, et al. Regulation of mitochondrial morphology and function by stearoylation of TFR1. Nature 2015;525:124-8. [Crossref] [PubMed]
- Moreno-Navarrete JM, Novelle MG, Catalán V, et al. Insulin resistance modulates iron-related proteins in adipose tissue. Diabetes Care 2014;37:1092-100. [Crossref] [PubMed]
- Daniels TR, Bernabeu E, Rodríguez JA, et al. The transferrin receptor and the targeted delivery of therapeutic agents against cancer. Biochim Biophys Acta 2012;1820:291-317. [Crossref] [PubMed]
- Nagai K, Nakahata S, Shimosaki S, et al. Development of a complete human anti-human transferrin receptor C antibody as a novel marker of oral dysplasia and oral cancer. Cancer Med 2014;3:1085-99. [Crossref] [PubMed]
- Zhang C, Tan C, Ding H, et al. Selective VEGFR inhibitors for anticancer therapeutics in clinical use and clinical trials. Curr Pharm Des 2012;18:2921-35. [Crossref] [PubMed]
- Ma H, Seebacher NA, Hornicek FJ, et al. Cyclin-dependent kinase 9 (CDK9) is a novel prognostic marker and therapeutic target in osteosarcoma. EBioMedicine 2019;39:182-93. [Crossref] [PubMed]
- Hao H, Chen L, Huang D. Meta-analysis of alkaline phosphatase and prognosis for osteosarcoma. Eur J Cancer Care (Engl) 2017; [Crossref] [PubMed]
- Zhang J, Lan Q, Lin J. Identification of key gene modules for human osteosarcoma by co-expression analysis. World J Surg Oncol 2018;16:89. [Crossref] [PubMed]
- Liu X, Hu AX, Zhao JL, et al. Identification of key gene modules in human osteosarcoma by co-expression analysis weighted gene co-expression network analysis (WGCNA). J Cell Biochem 2017;118:3953-59. [Crossref] [PubMed]
- Flores RJ, Li Y, Yu A, et al. A systems biology approach reveals common metastatic pathways in osteosarcoma. BMC Syst Biol 2012;6:50. [Crossref] [PubMed]
- Wang M, Xie R, Si H. Integrated bioinformatics analysis of miRNA expression in osteosarcoma. Artif Cells Nanomed Biotechnol 2017;45:936-43. [Crossref] [PubMed]
- Pan Y, Lu L, Chen J. Identification of potential crucial genes and construction of microRNA-mRNA negative regulatory networks in osteosarcoma. Hereditas 2018;155:21. [Crossref] [PubMed]
- Chen X, Xu P, Zhu J. Downregulation of NKD1 in human osteosarcoma and its clinical significance. Mol Med Rep 2018;17:1111-17. [PubMed]
- Cai H, Lin L, Cai H. Combined microRNA-340 and ROCK1 mRNA profiling predicts tumor progression and prognosis in pediatric osteosarcoma. Int J Mol Sci 2014;15:560-73. [Crossref] [PubMed]
- Tao Y, Xin M, Cheng H. TRIM37 promotes tumor cell proliferation and drug resistance in pediatric osteosarcoma. Oncol Lett 2017;14:6365-72. [Crossref] [PubMed]
- Wu JX, Carpenter PM, Gresens C, et al. The proto-oncogene c-fos is over-expressed in the majority of human osteosarcomas. Oncogene 1990;5:989-1000. [PubMed]
- Franchi A, Calzolari A, Zampi G. Immunohistochemical detection of c-fos and c-jun expression in osseous and cartilaginous tumours of the skeleton. Virchows Arch 1998;432:515-9. [Crossref] [PubMed]
- Gamberi G, Benassi MS, Bohling T, et al. C-myc and c-fos in human osteosarcoma: prognostic value of mRNA and protein expression. Oncology 1998;55:556-63. [Crossref] [PubMed]
- Wang ZQ, Liang J, Schellander K, et al. c-fos-induced osteosarcoma formation in transgenic mice: cooperativity with c-jun and the role of endogenous c-fos. Cancer Res 1995;55:6244-51. [PubMed]
- Leaner VD, Chick JF, Donninger H, et al. Inhibition of AP-1 transcriptional activity blocks the migration, invasion, and experimental metastasis of murine osteosarcoma. Am J Pathol 2009;174:265-75. [Crossref] [PubMed]
- Tan ML, Choong PF, Dass CR. Direct anti-metastatic efficacy by the DNA enzyme Dz13 and downregulated MMP-2, MMP-9 and MT1-MMP in tumours. Cancer Cell Int 2010;10:9. [Crossref] [PubMed]
- Shimizu T, Ishikawa T, Sugihara E, et al. c-MYC overexpression with loss of Ink4a/Arf transforms bone marrow stromal cells into osteosarcoma accompanied by loss of adipogenesis. Oncogene 2010;29:5687-99. [Crossref] [PubMed]
- Hattinger CM, Stoico G, Michelacci F, et al. Mechanisms of gene amplification and evidence of coamplification in drug-resistant human osteosarcoma cell lines. Genes Chromosomes Cancer 2009;48:289-309. [Crossref] [PubMed]
- Scionti I, Michelacci F, Pasello M, et al. Clinical impact of the methotrexate resistance-associated genes C-MYC and dihydrofolate reductase (DHFR) in high-grade osteosarcoma. Ann Oncol 2008;19:1500-8. [Crossref] [PubMed]
- Rogers MA, Langbein L, Praetzel S, et al. Sequences and differential expression of three novel human type-II hair keratins. Differentiation 1997;61:187-94. [Crossref] [PubMed]
- Yokoyama A, Taniki N, Nakamoto N, et al. Associations among liver disease, serum lipid profile, body mass index, ketonuria, meal skipping, and the alcohol dehydrogenase-1B and aldehyde dehydrogenase-2 genotypes in Japanese men with alcohol dependence. Hepatol Res 2020;50:565-77. [Crossref] [PubMed]
- Brandt EB, Mingler MK, Stevenson MD, et al. Surfactant protein D alters allergic lung responses in mice and human subjects. J Allergy Clin Immunol 2008;121:1140-7.e2. [Crossref] [PubMed]
- Naizhen X, Kido T, Yokoyama S, et al. Spatiotemporal expression of three secretoglobin proteins, SCGB1A1, SCGB3A1, and SCGB3A2, in mouse airway epithelia. J Histochem Cytochem 2019;67:453-63. [Crossref] [PubMed]
- Stacker SA, Caesar C, Baldwin ME, et al. VEGF-D promotes the metastatic spread of tumor cells via the lymphatics. Nat Med 2001;7:186-91. [Crossref] [PubMed]
- Hoffmann TJ, Keats BJ, Yoshikawa N, et al. A Large Genome-Wide Association Study of Age-Related Hearing Impairment Using Electronic Health Records. PLoS Genet 2016;12:e1006371. [Crossref] [PubMed]
- Xu J, Li D, Cai Z, et al. An integrative analysis of DNA methylation in osteosarcoma. J Bone Oncol 2017;9:34-40. [Crossref] [PubMed]
- Chen XG, Ma L, Xu JX. Abnormal DNA methylation may contribute to the progression of osteosarcoma. Mol Med Rep 2018;17:193-9. [PubMed]
- Schuurman AG, van den Brandt PA, Dorant E, et al. Association of energy and fat intake with prostate carcinoma risk: results from The Netherlands Cohort Study. Cancer 1999;86:1019-27. [Crossref] [PubMed]
- Leitzmann MF, Stampfer MJ, Michaud DS, et al. Dietary intake of n-3 and n-6 fatty acids and the risk of prostate cancer. Am J Clin Nutr 2004;80:204-16. [Crossref] [PubMed]
- Astorg P. Dietary fatty acids and colorectal and prostate cancers: epidemiological studies. Bull Cancer 2005;92:670-84. [PubMed]
- Whelan J, McEntee MF. Dietary (n-6) PUFA and intestinal tumorigenesis. The J Nutr 2004;134:3421S-6S. [Crossref] [PubMed]
- Cao Y, Prescott SM. Many actions of cyclooxygenase-2 in cellular dynamics and in cancer. J Cell Physiol 2002;190:279-86. [Crossref] [PubMed]
- Greenhough A, Smartt HJ, Moore AE, et al. The COX-2/PGE2 pathway: key roles in the hallmarks of cancer and adaptation to the tumour microenvironment. Carcinogenesis 2009;30:377-86. [Crossref] [PubMed]
- Tang Y, Gu Z, Fu Y, et al. CXCR3 from chemokine receptor family correlates with immune infiltration and predicts poor survival in osteosarcoma. Biosci Rep 2019;39:BSR20192134. [Crossref] [PubMed]
- Shen H, Wang W, Ni B, et al. Exploring the molecular mechanisms of osteosarcoma by the integrated analysis of mRNAs and miRNA microarrays. Int J Mol Med 2018;42:21-30. [Crossref] [PubMed]
- Zhang X, Wang X, Wang A, et al. CLDN10 promotes a malignant phenotype of osteosarcoma cells via JAK1/Stat1 signaling. J Cell Commun Signal 2019;13:395-405. [Crossref] [PubMed]
- Tian X, He Y, Han Z, et al. The cytoplasmic expression of CLDN12 predicts an unfavorable prognosis and promotes proliferation and migration of osteosarcoma. Cancer Manag Res 2019;11:9339-51. [Crossref] [PubMed]
- Hatakeyama N, Kojima T, Iba K, et al. IGF-I regulates tight-junction protein claudin-1 during differentiation of osteoblast-like MC3T3-E1 cells via a MAP-kinase pathway. Cell Tissue Res 2008;334:243-54. [Crossref] [PubMed]
- Markiewski MM, Nilsson B, Ekdah KN, et al. Complement and coagulation: strangers or partners in crime? Trends Immunol 2007;28:184-92. [Crossref] [PubMed]
- Pio R, Corrales L, Lambris JD. The role of complement in tumor growth. Adv Exp Med Biol 2014;772:229-62. [Crossref] [PubMed]
- Meyers PA. Muramyl tripeptide (mifamurtide) for the treatment of osteosarcoma. Expert Rev Anticancer Ther 2009;9:1035-49. [Crossref] [PubMed]
- Sone S, Mutsuura S, Ogawara M, et al. Potentiating effect of muramyl dipeptide and its lipophilic analog encapsulated in liposomes on tumor cell killing by human monocytes. J Immunol 1984;132:2105-10. [PubMed]
- Fidler IJ, Brown NO, Hart IR. Species variability for toxicity of free and liposome-encapsulated muramyl peptides administered intravenously. J Biol Response Mod 1985;4:298-309. [PubMed]
- Anderson PM, Markovic SN, Sloan JA, et al. Aerosol granulocyte macrophage-colony stimulating factor: a low toxicity, lung-specific biological therapy in patients with lung metastases. Clin Cancer Res 1999;5:2316-23. [PubMed]
- Inoki K, Corradetti MN, Guan KL. Dysregulation of the TSC-mTOR pathway in human disease. Nat Genet 2005;37:19-24. [Crossref] [PubMed]
- Zhou Q, Deng Z, Zhu Y, et al. mTOR/p70S6K Signal transduction pathway contributes to osteosarcoma progression and patients’ prognosis. Med Oncol 2010;27:1239-45. [Crossref] [PubMed]
- Wan X, Mendoza A, Khanna C, et al. Rapamycin inhibits ezrin-mediated metastatic behavior in a murine model of osteosarcoma. Cancer Res 2005;65:2406-11. [Crossref] [PubMed]
- Chawla SP, Tolcher AW, Staddon AP, et al. Updated results of a phase II trial of AP23573, a novel mTOR inhibitor, in patients with advanced soft tissue or bone sarcomas. J Clin Oncol 2006;24:9505. [Crossref]
- Abuzzahab MJ, Schneider A, Goddard A, et al. IGF-I receptor mutations resulting in intrauterine and postnatal growth retardation. N Engl J Med 2003;349:2211-22. [Crossref] [PubMed]
- Ouban A, Muraca P, Yeatman T, et al. Expression and distribution of insulin-like growth factor-1 receptor in human carcinomas. Hum Pathol 2003;34:803-8. [Crossref] [PubMed]
- Shimizu C, Hasegawa T, Tani Y, et al. Expression of insulin-like growth factor 1 receptor in primary breast cancer: immunohistochemical analysis. Hum Pathol 2004;35:1537-42. [Crossref] [PubMed]