Identification of key candidate genes associated with prognosis of lung adenocarcinoma by integrated bioinformatical analysis
Introduction
Lung adenocarcinoma (LUAD), the most frequent histologic type of lung cancer, is also one of the most frequently diagnosed carcinoma and one of the leading cause of cancer-related death in the world (1). There is a great improvement in the therapy of LUAD in recent years, but the prognosis of LUAD is still poor with less than 18% of 5-year survival rates (2). We all know that the development of lung cancer, including LUAD, is a multifactor process, and numerous genes participate in the process. Therefore, identification of the key genes of LUAD is crucial for understanding the mechanism of LUAD and provides possible therapeutic targets for further clinical application.
The rapid progress in proteomics, genomics and bioinformatics, especially gene-expression profiling by microarray have promoted the discovery of mechanism and key genes in many kinds of diseases, especially in tumors (3-5). Using gene microarray chips can conveniently detect the genes expression information and is very useful for screening differentially expressed genes (DEGs) (6). This method has been widely used to explore the mechanism of diseases, and a large number of microarray datasets have been produced in recent years and a great number of these datasets have been stored in the public databases, such as NCBI-Gene Expression Omnibus database (NCBI-GEO) and The Cancer Genome Atlas (TCGA) (7). Re-analyzing and integrating the datasets of these public databases can produce useful clues for our research.
In recent years, a great number of microarray studies of LUAD have been carried out, and thousands of DEGs (8,9) have been identified. But due to the heterogeneity of the tissues and samples in independent studies, these results are always limited or inconsistent. Many results also are produced from single cohort study and the sample size is too small. All of these effects cause a poor reliability of the results. However, using integrated bioinformatics methods might decrease these disadvantages. In this study, we downloaded three original human LUAD microarray datasets [GSE43458 (10), GSE32863 (11), GSE10072 (12)] from the NCBI-GEO (available online: https://www.ncbi.nlm.nih.gov/geo). A total of 196 LUAD samples and 137 normal samples were available from these three datasets. We first analyzed DEGs by GEOR2 and identified shared DEGs in all three datasets, and then developed Gene ontology, wiki-pathway enrichment analysis. In order to identify hub genes for DEGs, Cytoscape software were used to construct protein-protein interaction (PPI) network (http://string-db.org)and calculate node degrees. We further verified the differential expressions and the association with the prognosis of LUAD of these hub genes in the TCGA and the GTEx projects, using GEPIA (http://gepia.cancer-pku.cn/index.html) (13), which contain 483 LUAD patients and 374 normal individuals. Besides, we also analyzed the association with the prognosis of LUAD of those hub genes by Kaplan-Meier analysis, an online tool Kaplan-Meier Plotter (http://kmplot.com/analysis/) (14). The genetic alterations of these hub genes in LUAD patients were studied by using cBioPortal. Identifying DEGs and finding the key candidate genes will help us to find more accurate and reliable targets for early diagnosis and therapy of LUAD.
We present the following article in accordance with the MDAR checklist (available at http://dx.doi.org/10.21037/tcr-20-2110).
Methods
The information of microarray datasets and identification of DEGs
Three gene expression profiles of LUAD and normal or adjacent non-tumor lung tissues (GSE43458, GSE32863, GSE10072) were obtained from NCBI-GEO. The microarray data of GSE43458 was based on GPL6244, including 80 LUAD and 30 normal lung tissues (10). The GSE32863 dataset was based on Platforms GPL6884, including 58 LUAD and 58 adjacent normal lung tissues (11). The GSE10072 dataset was based on GPL96 Platforms, including 58 LUAD and 49 normal lung tissues (12). For data analysis we usedGEO2R, an online tool of GEO. The criteria of DEGs was adjust P value <0.05 and |logFC| >1. The study was conducted in accordance with the Declaration of Helsinki (as revised in 2013).
Gene ontology, WikiPathway enrichment analysis, integration of PPI network and identification of hub genes
The functional annotation including Gene Ontology analysis and WikiPathway analysis of DEGs was done by CluoGO APP of Cytoscape software platform (15) with P<0.05 as the cut-off criterion. The PPI network of the DEGs was constructed by a widely used online tools of The Search Tool for the Retrieval of Interacting Genes/Proteins (STRING) (http://string-db.org) (16), the threshold of PPIs was confidence score >0.4. The PPI network was reconstructed by Cytoscape software platform (15). Hub genes of the network were identified by a plug-in of Cytoscape software platform called cytoHubba, which has a powerful function to explore subnetworks and important nodes in a given network by calculating the connectivity of nodes with several topological algorithms, in this study two kinds of topological algorithms (MCC, Degree) was respectively used to calculate the top 25 nodes in the PPI network, and we identified 20 shared nodes as the most significant candidate genes in both methods.
Verify the hub genes in the TCGA and the GTEx projects
We verify the differential expressions of these hub genes in the TCGA and the GTEx projects, using GEPIA(http://gepia.cancer-pku.cn/index.html) (13), which contain 483 LUAD patients and 374 normal individuals. The criteria of DEGs was adjust P value <0.001 and |logFC| >1.
Verify the association between hub genes and prognosis of LUAD
We verify the association between hub genes and prognosis of LUAD using GEPIA (http://gepia.cancer-pku.cn/index.html) (13), which contain 483 LUAD patients’ survival data. Then further analyzed the association between the prognosis of LUAD and these hub genes by Kaplan-Meier analysis, an online tool Kaplan-Meier Plotter (http://kmplot.com/analysis/) (14), which contain 2,437 lung cancer patients with relapse-free and overall survival data. The criteria of significant association was log-rank P value <0.05.
The genetic alterations of these hub genes in LUAD patients
We investigate the genetic alterations of these hub genes in LUAD patients by an online tool The cBioPortal for Cancer Genomics (http://www.cbioportal.org/), we choose four studies about LUAD, which contain 1,847 LUAD patients totally.
The expression of CENPF in different subgroups of LUAD patients
The online tool UALCAN (http://ualcan.path.uab.edu) can provide useful information about different subgroups of genes in 31 cancer types according to age, gender, race, smoking condition and cancer stage groups. We use UALCAN to investigate the expression of CENPF in different subgroups of LUAD.
Statistical analysis
We used GEO2R to analysis GEO data and identify DEGs, an online tool (https://www.ncbi.nlm.nih.gov/geo/geo2r/). The criteria of DEGs was adjust P value <0.05 and |logFC| >1. Survival analysis was performed using Kaplan-Meier survival analysis and a log-rank test. P<0.05 was considered to indicate a statistically significant difference.
Results
Identification of DEGs in LUAD
We screened 897, 1,239 and 646 DEGs from three datasets GSE43458, GSE32863 and GSE10072 respectively, with cut-off criterion of adjusting P value <0.05 and |logFC| >1. And 240 overlapping DEGs were obtained from the three profile datasets (Figure 1).
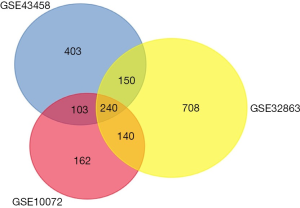
Functional annotation of DEGs
The functions and pathways enrichment of candidate DEGs were analyzed using Cytoscape software platform CluoGO APP. The DEGs were classified into biological process (BP) group, molecular function (MF) group and cellular component (CC) group (Figure 2). As shown in Figure 2, the BP group of DEGs mainly enriched in: kidney development, response to corticosteroid, regulation of epithelial cell apoptotic process, cardiac chamber morphogenesis, muscle organ development, negative regulation of proteolysis, negative regulation of peptidase activity, cellular response to transforming growth factor beta stimulus; the MF group of DEGs mainly enriched in: low density lipoprotein receptor activity, calcitonin family receptor activity, primary amine oxidase activity, metalloendopeptidase inhibitor activity, phospholipase A2 inhibitor activity, potassium channel inhibitor activity; the CC group of DEGs mainly enriched: in spindle pole, amylin receptor complex, fibrillar collagen trimer, external side of plasma membrane, basolateral plasma membrane, myosin Il complex, intrinsic component of external side of plasma membrane; the WikiPathway of DEGs mainly enriched: in matrix metalloproteinases, angiogenesis, gastric cancer network 1, spinal cord injury, IL1 and megakaryocytes in obesity.
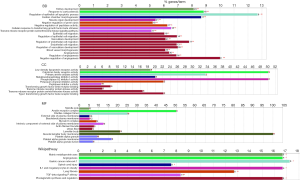
The construction of PPI network and identification of key candidate genes
All the overlapping 240 DEGs were filtered into the DEGs PPI network by the STRING online tools and Cytoscape software. There are 240 nodes and 749 edges in the network (Figure 3).
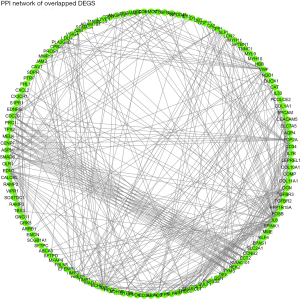
We have used two kinds of topological algorithms (including Degree and MCC) to calculate the top 25 nodes in the PPI-network (Figure 4A,B, Tables 1,2), and we have identified 20 shared nodes (IL6, MMP9, EDN1, VWF, TOP2A, CD34, COL1A1, SPP1, CDC20, KIAA0101, CDH5, CCNB2, NEK2, PRC1, TIMP3, TYMS, CENPF, TPX2) as the most significant candidate genes in both methods (Figure 4C, Table 3).
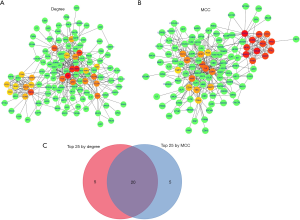
Table 1
Rank | Name | Score |
---|---|---|
1 | IL6 | 47 |
2 | MMP9 | 31 |
3 | EDN1 | 27 |
4 | VWF | 24 |
5 | TOP2A | 22 |
6 | CD34 | 21 |
7 | FOS | 20 |
8 | COL1A1 | 17 |
8 | SPP1 | 17 |
8 | DCN | 17 |
11 | TIMP1 | 16 |
11 | COL3A1 | 16 |
13 | CDC20 | 15 |
13 | CD36 | 15 |
15 | KIAA0101 | 14 |
15 | CDH5 | 14 |
17 | CCNB2 | 13 |
18 | NEK2 | 12 |
18 | PRC1 | 12 |
18 | TIMP3 | 12 |
18 | TYMS | 12 |
18 | A2M | 12 |
18 | CAV1 | 12 |
24 | CENPF | 11 |
24 | TPX2 | 11 |
Table 2
Rank | Name | Score |
---|---|---|
1 | TOP2A | 7257613 |
2 | CDC20 | 7257607 |
3 | KIAA0101 | 7257603 |
4 | CCNB2 | 7257602 |
5 | NEK2 | 7257601 |
5 | PRC1 | 7257601 |
7 | CENPF | 7257600 |
7 | TPX2 | 7257600 |
7 | ASPM | 7257600 |
7 | MELK | 7257600 |
11 | TYMS | 3628803 |
12 | ECT2 | 3628800 |
13 | IL6 | 2752 |
14 | MMP9 | 2633 |
15 | VWF | 2121 |
16 | EDN1 | 1500 |
17 | TIMP1 | 1375 |
18 | COL1A1 | 1054 |
19 | SPP1 | 954 |
20 | TEK | 868 |
21 | ANGPT1 | 864 |
22 | CD34 | 805 |
23 | CDH5 | 741 |
24 | FOS | 498 |
25 | TIMP3 | 319 |
Table 3
IL6 |
MMP9 |
EDN1 |
VWF |
TOP2A |
CD34 |
FOS |
COL1A1 |
SPP1 |
TIMP1 |
CDC20 |
KIAA0101 |
CDH5 |
CCNB2 |
NEK2 |
PRC1 |
TIMP3 |
TYMS |
CENPF |
TPX2 |
Verify the hub genes in the TCGA and the GTEx projects
We verified the differential expression of LUAD of these hub genes in the TCGA and the GTEx projects, using GEPIA, which contains 483 LUAD patients and 374 normal individuals. With the criteria of P value <0.001 and |logFC| >1, all these hub genes were differentially expressed except TIMP1 and FOS (Figure 5).
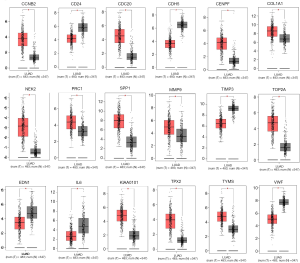
Verify the association between hub genes and prognosis of LUAD
We first verified the association between hub genes and prognosis of LUAD using GEPIA, which contains 483 LUAD patients’ survival data. The high expression of these ten genes, including: TPX2 (HR =1.6; log rank P=0.0013), CENPF (HR =1.5; log rank P=0.0098), TYMS (HR =1.7; log rank P=0.00052), PRC1 (HR =1.6; log rank P=0.0012), NEK2 (HR =1.7; log rank P=0.00031), CCNB2 (HR =1.7; log rank P=0.00079), KIAA0101 (HR =1.6; log rank P=0.0012), CDC20 (HR =1.5; log rank P=0.0094), TOP2A (HR =1.5; log rank P=0.011) and SPP1 (HR =1.4; log rank P=0.015), were confirmed to associate with the poor prognosis of LUAD in this analysis (Figure 6).
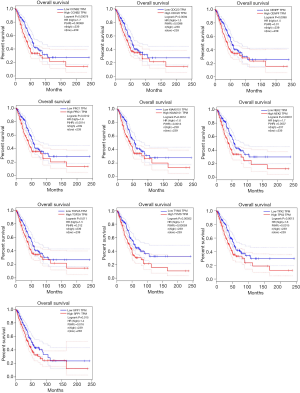
Then we further verified the association between the prognosis of lung cancer and hub genes by Kaplan-Meier analysis using online tool Kaplan-Meier Plotter, which contains 2,437 lung cancer patients with relapse-free and overall survival data. The high expression of these ten genes also have significant association with the poor prognosis of lung cancer in this analysis (Figure 7), including TPX2 [HR =2.62 (2.19−3.15); log rank P<1E−16], CENPF [HR =1.61 (1.42−1.84); log rank P=2E−13], TYMS [HR =1.86 (1.6−2.15); log rank P<1E−16], PRC1 [HR =2.31 (1.94−2.76); log rank P<1E−16], NEK2 [HR =2.09 (1.76−2.49); log rank P<1E−16], CCNB2 [HR =2.57 (2.14−3.08); log rank P<1E−16], KIAA0101 [HR =1.9 (1.63−2.22); log rank P=1.1E−16], CDC20 [HR =2.29 (1.93−2.73); log rank P<1E−16], TOP2A [HR =2.15 (1.81−2.56); log rank P<1E−16] and SPP1 [HR =1.33 (1.17−1.51); log rank P=1E−05].
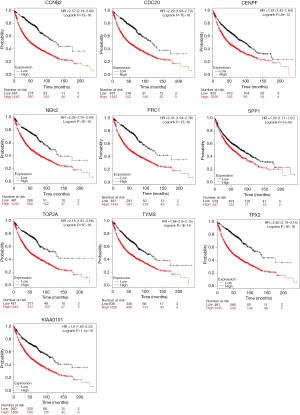
The genetic alterations of these hub genes in LUAD patients
The genetic alterations of these hub genes by cBioPortal in LUAD patients showed that CENPF had the highest rate of 4%, the rate of NEK2 was 2.2%, the rate of TPX2 was 1.5%, and the most commonly alteration type was missense mutation (Figure 8).
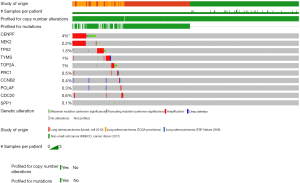
The expression of CENPF in different subgroups of LUAD patients
The expression of CENPF was significantly increased in all age, gender, race, smoking condition and cancer stage groups of LUAD patients (Figure 9). The P value of all subgroup vs. normal group was <0.05. But there was no significant difference in LUAD patients between different age groups, and also no significant difference between different gender, race and cancer stage groups. The P value between each subgroup was not <0.05.
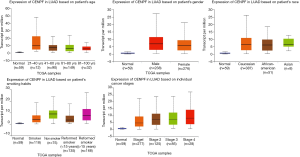
Discussion
In recent years, a large number of basic and clinical studies have been conducted to explore the underlying mechanisms and causes of LUAD development and progression, but the morbidity and mortality rates of LUAD are still high worldwide. The primary cause of this dilemma is that the development and progression of LUAD is a multifactor process, which is influenced by numerous genes. However, most studies only study a single cohort population or focus on a single gene, which may limit the accuracy and credibility of the results.
In this study, we integrated three LUAD profile datasets from NCBI-GEO public database to deeply analyze the data by bioinformatic methods, and identified 240 overlapped DEGs in the first step. And the results of GO analysis showed that the DEGs were involved in kidney development, response to corticosteroid, regulation of epithelial cell apoptotic process in BP; wiki-pathway enrichment annotation indicated that the DEGs were mainly enriched in matrix metalloproteinases, angiogenesis, gastric cancer network 1, spinal cord injury.
Bioinformatic analysis is considered as a powerful tool to explore novel diagnosis markers and therapeutic targets for various diseases, particularly for cancers, in recent years. A study in gastric cancer reveal that COL1A2, THBS2, COL1A1, ITGA5 and COL4A1 may be potential biomarkers and useful therapeutic targets for gastric cancer patients (17). Another integrated bioinformatic analysis study of prostate cancer has shown that the BZRAP1-AS1 may become a potential powerful biomarker of prostate cancer (18). Numerous similar studies of LUAD have been conducted as well. In LUAD patients, four transcription factors, including forkhead box D1, homeobox A5, E74-like ETS transcription factor 5 and Krüppel-like factor 5, had been identified to be associated with LUAD by integrated bioinformatic analysis. However, their studies selected candidate genes only using module method to calculate the degree of connectivity of the nodes in the PPI-network. In addition, their selected candidate genes were not validated in the TCGA and the GTEx projects, and the association between the candidate genes and the prognosis of LUAD had not been validated in the study.
In Our study, we integrated three GEO datasets, and in the PPI-network we calculate the top 25 genes by the MCC method and Degree method of CytoHubba plugin, and 20 genes was overlapped in both methods. Then we revalidated the results in the database of TCGA and the GTEx. As a result, we identified 18 common nodes, including IL6, MMP9, EDN1, VWF, TOP2A, CD34, COL1A1, SPP1, CDC20, KIAA0101, CDH5, CCNB2, NEK2, PRC1, TIMP3, TYMS, CENPF, TPX2, as the most significant candidate genes in both databases. Furthermore, we verified the association between these hub genes and the prognosis of LUAD by TCGA and the GTEx projects, which increased the reliability of our research. We found that ten hub genes, including TPX2, CENPF, TYMS, PRC1, NEK2, CCNB2, KIAA0101, CDC20, TOP2A and SPP1, associated with robust poor prognosis of LUAD.
TPX2 has the highest HR value and the third highest genetic alteration rate in this study. Numerous studies reported that TPX2 was a mitotic factor and important for organization of microtubule, formation of spindle (19-21). A recent study has reported that TPX2 plays a critical role as a coactivator of AURKA in the drug resistance of LUAD for carrying EGFR mutations (22). The thymidylate synthase (TYMS) plays an important role in the early stages of DNA biosynthesis and have been identified as an important chemotherapy target for several pathological type of cancers (23). TYMS is found to be associated with the effective treatment of pemetrexed in non-small cell lung cancer (NSCL) (24), and the polymorphisms of TYMS are contribute to the risk of NSCL in non-Hispanic whites (25). PRC1, which plays a crucial role in activating the Wnt/β-catenin pathway, is considered as a powerful prognostic biomarker and a promising therapeutic target for LUAD (26). In other studies, NEK2, CCNB2, KIAA0101, CDC20, SPP1 and TOP2A were also identified as prognostic factors to predict outcomes for patients with NSCLC or LUAD (27-31).
CENPF has been reported as a prognostic biomarker of prostate cancer for poor survival and metastasis (32), but rare studies have reported the association between CENPF and LUAD. Centromere protein F (CENPF) encodes a centromere-kinetochore protein, which is a component of the nuclear matrix during the G2 phase of interphase and plays an important role in the process of chromosome segregation during cell mitosis (33). Many studies have revealed that the overexpression of CENPF was associated with poor prognosis of several kinds of tumors, such as breast cancer, nasopharyngeal carcinoma, hepatocellular carcinoma and bladder cancer (34,35). But the carcinogenesis role, expression characteristic and potential target of CENPF in LUAD have not been adequately studied. In our study, CENPF has the highest genetic alteration rate in LUAD patients. The most commonly alteration type was missense mutation. The expression of CENPF was significantly increased in all age, gender, race, smoking condition and cancer stage groups of LUAD patients. The result suggested that CENPF may become a promising target for further study in LUAD.
In recent similar study, the mRNA level and protein level of CENPF was significantly increased in breast cancer and lung cancer, and the potential downstream signal pathway of CENPF were P53 pathway, PI3K/AKT/mTOR pathway, and mTORC1 pathway. CENPF played an important role in promoting bone metastasis in breast cancer through the PI3K-AKT-mTORC1 pathway (34). In our study, we found CENPF has the highest genetic alteration rate in LUAD, this manifest CENPF maybe a potential powerful biomarker or a therapy target of LUAD. Large sample clinical studies should be performed to further confirm the association between CENPF and LUAD in the future.
However, there are certain limitations in this study, such as that these findings were obtained from microarray data and online databases via bioinformatic methods. Therefore, more basic studies and clinic studies of large sample and multicenter are necessary to further confirm the results of this study in the future.
Conclusions
A total of ten genes including TPX2, CENPF, TYMS, PRC1, NEK2, CCNB2, KIAA0101, CDC20, TOP2A and SPP1 were identified as key candidate genes in LUAD, and CENPF may play a critical role in the carcinogenesis of LUAD. Our findings may provide a deeper understanding of the development and progression of LUAD.
Acknowledgments
Funding: This work was supported by grants from
Footnote
Reporting Checklist: The authors have completed the MDAR checklist. Available at http://dx.doi.org/10.21037/tcr-20-2110
Peer Review File: Available at http://dx.doi.org/10.21037/tcr-20-2110
Conflicts of Interest: All authors have completed the ICMJE uniform disclosure form (available at http://dx.doi.org/10.21037/tcr-20-2110). The authors have no conflicts of interest to declare.
Ethical Statement: The authors are accountable for all aspects of the work in ensuring that questions related to the accuracy or integrity of any part of the work are appropriately investigated and resolved. The study was conducted in accordance with the Declaration of Helsinki (as revised in 2013).
Open Access Statement: This is an Open Access article distributed in accordance with the Creative Commons Attribution-NonCommercial-NoDerivs 4.0 International License (CC BY-NC-ND 4.0), which permits the non-commercial replication and distribution of the article with the strict proviso that no changes or edits are made and the original work is properly cited (including links to both the formal publication through the relevant DOI and the license). See: https://creativecommons.org/licenses/by-nc-nd/4.0/.
References
- Torre LA, Bray F, Siegel RL, et al. Global cancer statistics, 2012. CA Cancer J Clin 2015;65:87-108. [Crossref] [PubMed]
- DeSantis CE, Siegel RL, Sauer AG, et al. Cancer statistics for African Americans, 2016: Progress and opportunities in reducing racial disparities. CA Cancer J Clin 2016;66:290-308. [Crossref] [PubMed]
- Tang Y, Zhang Z, Tang Y, et al. Identification of potential target genes in pancreatic ductal adenocarcinoma by bioinformatics analysis. Oncol Lett 2018;16:2453-61. [Crossref] [PubMed]
- Guo Y, Bao Y, Ma M, et al. Identification of Key Candidate Genes and Pathways in Colorectal Cancer by Integrated Bioinformatical Analysis. Int J Mol Sci 2017;18:722. [Crossref] [PubMed]
- Fu Q, Yang F, Zhao J, et al. Bioinformatical identification of key pathways and genes in human hepatocellular carcinoma after CSN5 depletion. Cell Signal 2018;49:79-86. [Crossref] [PubMed]
- Tang J, Kong D, Cui Q, et al. Prognostic Genes of Breast Cancer Identified by Gene Co-expression Network Analysis. Front Oncol 2018;8:374. [Crossref] [PubMed]
- Tang J, Lu M, Cui Q, et al. Overexpression of ASPM, CDC20, and TTK Confer a Poorer Prognosis in Breast Cancer Identified by Gene Co-expression Network Analysis. Front Oncol 2019;9:310. [Crossref] [PubMed]
- Lopez-Ayllon BD, Moncho-Amor V, Abarrategi A, et al. Cancer stem cells and cisplatin-resistant cells isolated from non-small-lung cancer cell lines constitute related cell populations. Cancer Med 2014;3:1099-111. [Crossref] [PubMed]
- Sato T, Kaneda A, Tsuji S, et al. PRC2 overexpression and PRC2-target gene repression relating to poorer prognosis in small cell lung cancer. Sci Rep 2013;3:1911. [Crossref] [PubMed]
- Kabbout M, Garcia MM, Fujimoto J, et al. ETS2 mediated tumor suppressive function and MET oncogene inhibition in human non-small cell lung cancer. Clin Cancer Res 2013;19:3383-95. [Crossref] [PubMed]
- Selamat SA, Chung BS, Girard L, et al. Genome-scale analysis of DNA methylation in lung adenocarcinoma and integration with mRNA expression. Genome Res 2012;22:1197-211. [Crossref] [PubMed]
- Landi MT, Dracheva T, Rotunno M, et al. Gene expression signature of cigarette smoking and its role in lung adenocarcinoma development and survival. PLoS One 2008;3:e1651. [Crossref] [PubMed]
- Tang Z, Li C, Kang B, et al. GEPIA: a web server for cancer and normal gene expression profiling and interactive analyses. Nucleic Acids Res 2017;45:W98-W102. [Crossref] [PubMed]
- Győrffy B, Surowiak P, Budczies J, et al. Online Survival Analysis Software to Assess the Prognostic Value of Biomarkers Using Transcriptomic Data in Non-Small-Cell Lung Cancer. PLoS One 2013;8:e82241. [Crossref] [PubMed]
- Smoot ME, Ono K, Ruscheinski J, et al. Cytoscape 2.8: new features for data integration and network visualization. Bioinformatics 2011;27:431-2. [Crossref] [PubMed]
- Szklarczyk D, Franceschini A, Wyder S, et al. STRING v10: protein-protein interaction networks, integrated over the tree of life. Nucleic Acids Res 2015;43:D447-52. [Crossref] [PubMed]
- Cao L, Chen Y, Zhang M, et al. Identification of hub genes and potential molecular mechanisms in gastric cancer by integrated bioinformatics analysis. PeerJ 2018;6:e5180. [Crossref] [PubMed]
- Tan J, Jin X, Wang K. Integrated Bioinformatics Analysis of Potential Biomarkers for Prostate Cancer. Pathol Oncol Res 2019;25:455-60. [Crossref] [PubMed]
- Li M, Gao K, Chu L, et al. The role of Aurora-A in cancer stem cells. Int J Biochem Cell Biol 2018;98:89-92. [Crossref] [PubMed]
- Neumayer G, Nguyen MD. TPX2 impacts acetylation of histone H4 at lysine 16: implications for DNA damage response. PLoS One 2014;9:e110994. [Crossref] [PubMed]
- Chu TL, Connell M, Zhou L, et al. Cell Cycle-dependent Tumor Engraftment and Migration are Enabled by Aurora A. Mol Cancer Res 2018;16:16-31. [Crossref] [PubMed]
- Shah KN, Bhatt R, Rotow J, et al. Aurora kinase A drives the evolution of resistance to third-generation EGFR inhibitors in lung cancer. Nat Med 2019;25:111-8. [Crossref] [PubMed]
- Peters GJ, Backus HH, Freemantle S, et al. Induction of thymidylate synthase as a 5-fluorouracil resistance mechanism. Biochim Biophys Acta 2002;1587:194-205. [Crossref] [PubMed]
- Yang M, Fan W, Pu X, et al. The role of thymidylate synthase in non-small-cell lung cancer treated with pemetrexed continuation maintenance therapy. J Chemother 2017;29:106-12. [Crossref] [PubMed]
- Cui LH, Yu Z, Zhang TT, et al. Influence of polymorphisms in MTHFR 677 C→T, TYMS 3R→2R and MTR 2756 A→G on NSCLC risk and response to platinum-based chemotherapy in advanced NSCLC. Pharmacogenomics 2011;12:797-808. [Crossref] [PubMed]
- Zhan P, Zhang B, Xi GM, et al. PRC1 contributes to tumorigenesis of lung adenocarcinoma in association with the Wnt/β-catenin signaling pathway. Mol Cancer 2017;16:108. [Crossref] [PubMed]
- Liu L, Shi M, Wang Z, et al. A molecular and staging model predicts survival in patients with resected non-small cell lung cancer. BMC Cancer 2018;18:966. [Crossref] [PubMed]
- Shi YX, Yin JY, Shen Y, et al. Genome-scale analysis identifies NEK2, DLGAP5 and ECT2 as promising diagnostic and prognostic biomarkers in human lung cancer. Sci Rep 2017;7:8072. [Crossref] [PubMed]
- Qian X, Song X, He Y, et al. CCNB2 overexpression is a poor prognostic biomarker in Chinese NSCLC patients. Biomed Pharmacother 2015;74:222-7. [Crossref] [PubMed]
- Kato T, Daigo Y, Aragaki M, et al. Overexpression of KIAA0101 predicts poor prognosis in primary lung cancer patients. Lung Cancer 2012;75:110-8. [Crossref] [PubMed]
- Kato T, Daigo Y, Aragaki M, et al. Overexpression of CDC20 predicts poor prognosis in primary non-small cell lung cancer patients. J Surg Oncol 2012;106:423-30. [Crossref] [PubMed]
- Aytes A, Antonina M, Celine L, et al. Cross-species regulatory network analysis identifies a synergistic interaction between FOXM1 and CENPF that drives prostate cancer malignancy. Cancer Cell 2014;25:638-51. [Crossref] [PubMed]
- Chen EB, Qin X, Peng K, et al. HnRNPR-CCNB1/CENPF axis contributes to gastric cancer proliferation and metastasis. Aging 2019;11:7473-91. [Crossref] [PubMed]
- Sun J, Huang J, Lan J, et al. Overexpression of CENPF correlates with poor prognosis and tumor bone metastasis in breast cancer. Cancer Cell Int 2019;19:264. [Crossref] [PubMed]
- Shi J, Zhang P, Liu L, et al. Weighted gene coexpression network analysis identifies a new biomarker of CENPF for prediction disease prognosis and progression in nonmuscle invasive bladder cancer. Mol Genet Genomic Med 2019;7:e982. [Crossref] [PubMed]