Application of CT radiomics features to predict the EGFR mutation status and therapeutic sensitivity to TKIs of advanced lung adenocarcinoma
Introduction
Lung cancer accounts for approximately 13% of newly diagnosed cancers worldwide and is the leading cause of cancer-related deaths (1). Approximately 85% of all lung cancers are non-small cell lung cancer, and lung adenocarcinoma is the main tissue subtype, accounting for more than 50% of all non-small cell lung cancers (2). More than 70% of patients with non-small cell lung cancer have locally advanced or distantly metastatic disease at the time of diagnosis (3); however, platinum-based combination chemotherapy has increased the median survival time of these patients. Despite this treatment, the prognosis is still very poor, and the efficacy of the current first-line chemotherapy is only approximately 30% (4). Epidermal growth factor receptor (EGFR) is a transmembrane receptor tyrosine kinase that activates the EGFR signaling pathway to promote cell proliferation, invasion, metastasis, and neovascularization and inhibit apoptosis. EGFR mutations are mainly located in exons 18-21; the exon 19 deletion mutation (del E746-A750) and the exon 21 point mutation (L858R) are sensitive mutations, accounting for 85% of all mutations. The effective rate of treatment with tyrosine kinase inhibitors (TKIs) in patients with EGFR-sensitive mutations is 70%. Relevant reports have shown that TKI treatment can be extended to 9 to 13 months as a first-line treatment (5-7). EGFR-TKIs are the main treatment for advanced unresectable lung adenocarcinoma, so EGFR-sensitive mutations are the main predictors of the efficacy of EGFR-TKIs (8,9). IPASS studies have shown that 20-30% of patients with EGFR-sensitive mutations are primarily resistant to EGFR-TKIs. Primary resistance in patients with EGFR-sensitive mutations is defined as disease progression within 3 months of targeted drug use. Therefore, knowledge of the EGFR mutation status of lung adenocarcinoma and the patient's sensitivity to drugs is necessary before choosing a treatment plan. Traditionally, the method for determining EGFR mutation status is pathological examination after tissue biopsy. Because of the risks associated with tissue biopsy, it is often difficult to perform multiple biopsies to obtain sufficient tissue specimens and local tissues due to tumor heterogeneity. Such samples do not represent the tumor as a whole; thus, this method has some limitations in the clinic (10-12). Studies such as PIONEER have shown that women, nonsmokers, those with adenocarcinoma, and Asian patients are the dominant populations in whom TKIs can be used, but there are no reliable clinical features to accurately predict EGFR mutation status. Therefore, we need noninvasive early predictors to determine which patients are most likely to benefit from TKI treatment.
With the advancement of image acquisition technology, standardized processing and image analysis technology, more imaging information can be objectively extracted, accurately quantified and further transformed into deeply extensible feature data through various algorithms, and these image feature data can be analyzed in depth. Image features can reveal specific information that is closely related to disease occurrence, development and prognosis and can be used to guide disease treatment and predict disease outcome. This process and the emerging field are called radiomics (13). Radiomics assumes that microscopic changes in gene or protein patterns can be expressed by macroscopic imaging features and that macroscopic image features are closely related to microscopic changes in genes, proteins, and molecules (14). Aerts et al. (15) found that 440 image features were extracted from CT images of 1,019 patients with lung cancer and head and neck cancer. The results showed that a large number of imaging features were significantly associated with patient prognosis, while images and genomes. The association analysis showed that radiomics features can capture the heterogeneity within the tumor and are related to the pattern of gene expression, and some features are common in lung cancer and head and neck tumors. This study used radiomics to analyze and predict the genetic phenotype and sensitivity to TKI treatment and provided a reference for the accurate treatment of lung adenocarcinoma. We present the following article in accordance with the STARD reporting checklist (available at http://dx.doi.org/10.21037/tcr-20-1216).
Methods
The study was conducted in accordance with the Declaration of Helsinki (as revised in 2013). The study was approved by the Institutional Review Board of Shandong Tumor Hospital and Institute (No. 201705021) and individual consent for this retrospective analysis was waived.
The study subjects were patients who were diagnosed with stage IIIB or stage IV lung adenocarcinoma confirmed by pathology at Jining No. 1 People’s Hospital from 2014.10.01 to 2017.12.31. These patients had no previous surgery, radiotherapy or chemotherapy. Because the study was approved by the hospital ethics committee for retrospective studies, there was no need for informed consent.
The inclusion criteria
Pathologically confirmed lung adenocarcinoma and EGFR detection (16-19), all using the Amplification Refractory Mutation System (ARMS); plain and enhanced CT scans performed before treatment; oral TKI treatment; clinical stage IIIB-IV disease with lesions that could be measured; and CT evaluation performed within 3 months after treatment (using the RECIST 1.11.1 criteria, complete or partial remission was considered treatment-sensitive, and stable disease or progression was considered primary resistance).
The exclusion criteria
radiotherapy, chemotherapy or concurrent chemoradiotherapy before targeted therapy; undetermined clinical stage; CT not performed before and after treatment; or clinical stage other than stage IIIB or IV.
Chest CT examination method
A United States GE Lightspeed VCT 64-slice spiral CT scanner with the following scanning parameters was used: tube voltage of 120 kVp, automatic tube current, 0.6-mm collimator, reconstruction layer thickness of 3 mm, 3-mm layer spacing, and matrix of 512×512. An enhanced scan was performed with contrast agent (Ultravist 370 mg/mL; Schering, Berlin, Germany) injected with a double-barrel high-pressure syringe using a syringe pump (Ulrich medical, Ulm, Germany) in the cubital vein at an injection rate of 2.3–3.0 mL/sec with a maximum dose of 100 ml, using a syringe pump. The arterial phase lasted from 25–30 s after the contrast agent was injected, and the venous phase was initiated 60 seconds later. The images from the plain scan and arterial and venous phase scans were exported in Digital Imaging and Communications in Medicine (DICOM) format.
Image segmentation and feature extraction
In this study, automatic delineation and manual modification were used for target area delineation. First, MIM Maestro 6.7.6 (US MIM Software) software was used to delineated the gross tumor volume (GTV) in the lung window according to the threshold. Then the automatically delineated GTV was modified by a radiologist with more than 5 years of experience. A clinician with more than 10 years of experience also examined the area of interest. Any questionable areas were evaluated until an agreement was reached after deliberation. Feature extraction is a key step in radiomics, and it is important to extract the best predictive features. Image feature extraction 3D Slicer 4.7.0 software was used to extract 715 features from pretreatment CT scans, including 13 shape features, 78 grayscale features and 624 wavelet transform features.
Statistical analysis and modeling
The LASSO algorithm and 10-fold cross-validation method were used for modeling and analysis. These analyses were performed with the “glmnet” software package with R software. P<0.05 was considered statistically significant (Figures S1,S2).
Results
Patients with EGFR mutation status and TKI treatment sensitivity Among 253 patients, 167 patients had EGFR-sensitive mutations (89 patients with exon19 deletions and 78 patients with exon 21 L858R point mutations), and 86 patients had no EGFR-negative mutations. Among the 167 patients with EGFR mutations, 84 patients were sensitive to TKIs, and 83 had primary resistance to TKIs (Tables 1,2).
Table 1
Feature | EGFR+ (n=167) | EGFR− (n=86) | P value |
---|---|---|---|
Gender | |||
Male | 53 | 45 | 0.001 |
Female | 114 | 41 | |
Age | 64 [37–79] | 60 [40–77] | 0.62 |
Stage | |||
IIIB | 41 | 20 | 0.82 |
IV | 126 | 46 | |
Smoking status | |||
Yes | 50 | 55 | <0.001 |
No | 117 | 31 |
EGFR+, epidermal growth factor receptor sensitive mutation; EGFR−, epidermal growth factor receptor have no mutation.
Table 2
Feature | TKI sensitive (n=84) | TKI resistant (n=83) | P value |
---|---|---|---|
Gender | |||
Male | 23 | 30 | 0.225 |
Female | 61 | 53 | |
Age | 56 [37–67] | 66 [40–79] | 0.51 |
Stage | |||
IIIB | 20 | 21 | 0.621 |
IV | 64 | 62 | |
Smoking status | |||
Yes | 40 | 49 | 0.469 |
No | 44 | 34 |
TKI sensitive, tyrosine kinase inhibitors sensitive; TKI resistant, tyrosine kinase inhibitors resistant.
The correlation analysis between EGFR mutation status and imaging feature characteristics is shown in Table 3. Based on the model established by the imaging phenotypic characteristics of the plain scans, the AUC value of EGFR mutation status (Figure 1) was 0.6713, and the sensitivity and specificity were 70.3% and 89.3%, respectively. Based on the model established by the imaging features obtained during the arterial phase, the AUC value of EGFR mutation status was predicted to be 0.8194, and the sensitivity and specificity were 76.6% and 81.8%, respectively. The established model predicted an AUC value for mutational state of 0.8464, and the sensitivity and specificity were 70.3% and 83.3%, respectively. The AUC value was highest during the venous phase. Three-phase CT imaging features: The EGFR mutation group had several imaging characteristics with predictive potential including 5 plain scan image features (1 neighborhood grayscale difference matrix feature and 4 wavelet transform features; AUC value: 0.614–0.663; P<0.05), 18 arterial phase image features (2 first-order features, 1 grayscale matrix feature, and 15 wavelet transform features; AUC value: 0.609–0.696; P<0.05), and 23 venous phase features (1 first-order feature, 1 shape feature, 1 neighborhood grayscale difference matrix feature, and 20 wavelet transform features; AUC value: 0.603–0.700; P<0.05).
Table 3
Model | Data | Sensitivity (%) | Specificity (%) | Positive predictive value (%) | Negative predictive value (%) | Accuracy (%) | AUC |
---|---|---|---|---|---|---|---|
Plain scan | Training cohort | 70.3 (45/64) | 75.7 (50/66) | 73.7 (45/61) | 72.5 (50/69) | 73.1 (95/130) | 0.6713 |
Validation cohort | 75.0 (15/20) | 65.0 (13/20) | 68.2 (15/22) | 72.2 (13/18) | 70.0 (28/40) | 0.7625 | |
Total | 71.4 (60/84) | 73.3 (63/86) | 72.3 (60/83) | 72.4 (63/87) | 72.4 (123/170) | ||
Arterial phase | Training cohort | 76.6 (49/64) | 78.7 (52/66) | 77.8 (49/63) | 77.6 (52/67) | 77.7 (101/130) | 0.8194 |
Validation cohort | 80.0 (16/20) | 80.0 (16/20) | 80.0 (16/20) | 80.0 (16/20) | 80.0 (32/40) | 0.8065 | |
Total | 77.3 (65/84) | 79.1 (68/86) | 78.3 (65/83) | 78.1 (68/87) | 78.2 (133/170) | ||
Venous phase | Training cohort | 70.3 (45/64) | 83.3 (55/66) | 80.4 (45/56) | 74.3 (55/74) | 76.9 (100/130) | 0.8464 |
Validation cohort | 80.0 (16/20) | 80.0 (16/20) | 80.0 (16/20) | 80.0 (16/20) | 80.0 (32/40) | 0.8075 | |
Total | 72.6 (61/84) | 82.6 (71/86) | 80.3 (61/76) | 75.5 (71/94) | 77.6 (132/170) |
AUC, the area under the curve value.
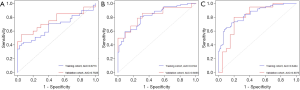
The correlation between TKI treatment sensitivity and imaging phenotypes is shown in Table 4. Based on the model established by the imaging phenotypic characteristics obtained during the plain scan period, the AUC value of TKI sensitivity AUC (Figure 2) was 0.7268, and the sensitivity and specificity were 61% and 82.5%, respectively. Based on the model established by the imaging features obtained during the arterial phase, the AUC value of TKI sensitivity was estimated to be 0.7793, and the sensitivity and specificity were 62.5% and 69.8%, respectively. Based on the imaging phenotype of image features obtained during the venous phase, the established model predicted an AUC value of TKI sensitivity of 0.9104, and the sensitivity and specificity were 76.6% and 88.9%, respectively. The AUC value was the highest during the venous phase. Three-phase CT imaging features: The TKI treatment-sensitive group had several imaging characteristics with predictive potential including 3 plain scan image features (3 wavelet transform features; AUC value: 0.619–0.688; P<0.05), 7 arterial phase image features (1 grayscale difference matrix feature and 6 wavelet transform features; AUC value: 0.606–0.686; P<0.05) and 22 venous phase image features (2 first-order features, 1 neighborhood grayscale difference matrix feature, and 19 wavelet transform features; AUC value: 0.616–0.703; P <0.05).
Table 4
Model | Data | Sensitivity (%) | Specificity (%) | Positive predictive value (%) | Negative predictive value (%) | Accuracy (%) | AUC |
---|---|---|---|---|---|---|---|
Plain scan | Training cohort | 61 (39/64) | 69.8 (44/63) | 67.2 (39/58) | 63.8 (44/69) | 65.4 (83/127) | 0.7268 |
Validation cohort | 70.0 (14/20) | 65.0 (13/20) | 66.7 (14/21) | 68.4 (13/19) | 67.5 (27/40) | 0.73 | |
Total | 63.1 (53/84) | 68.7 (57/83) | 67.1 (53/79) | 64.8 (57/88) | 65.9 (110/167) | ||
Arterial phase | Training cohort | 68.8 (44/64) | 71.4 (45/63) | 71.0 (44/62) | 67.2 (45/67) | 70.1 (89/127) | 0.7793 |
Validation cohort | 65 (13/20) | 70.0 (14/20) | 68.4 (13/19) | 66.7 (14/21) | 77.5 (31/40) | 0.8325 | |
Total | 67.9 (57/84) | 71.1 (59/83) | 70.4 (57/81) | 67.0 (59/88) | 71.9 (120/167) | ||
Venous phase | Training cohort | 76.6 (49/64) | 88.9 (56/63) | 87.5 (49/56) | 78.9 (56/71) | 82.7 (105/127) | 0.9104 |
Validation cohort | 65 (13/20) | 90.0 (18/20) | 86.7 (13/15) | 72.0 (18/25) | 77.5 (31/40) | 0.895 | |
Total | 73.8 (62/84) | 89.2 (74/83) | 87.3 (62/71) | 77.1 (74/96) | 81.4 (136/167) |
AUC, the area under the curve value.
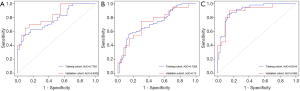
There was no significant correlation among the morphologic features of interphase imaging and the EGFR mutation status and sensitivity to TKI therapy.
Discussion
During the process of lung cancer diagnosis and treatment, the genetic characteristics of tumors are analyzed at the gene, molecular and protein levels by applying various techniques of genomics and proteomics to guide individualized clinical treatment. Compared with proteomics and genomics, radiomics has the following advantages: (I) Radiomics does not require biopsy; thus, it is a noninvasive test. (II) Radiomics features reflect of the entire tumor and are not limited to measurable tissue samples. (III) Radiomics can be applied during the whole process of diagnosis, treatment and prognostication of diseases as a real-time and reusable data analysis method.
This study aimed to investigate the potential of different phases of CT to predict EGFR mutation status and TKI treatment sensitivity. We extracted 715 radiomics features to quantify tumor phenotype differences. In the EGFR mutation prediction group, the results showed that there were 5, 18 and 23 features of EGFR (+) and EGFR (−) extracted from the plain scan and arterial and venous phases, respectively, and the AUC values were 0.6713, 0.8194, and 0.8464, respectively. Rios Velazquez et al. (20) performed a comprehensive analysis of 352 patients with lung adenocarcinoma who underwent EGFR gene mutation detection and CT and found that imaging histology can effectively distinguish between EGFR (+) and EGFR (−) with an AUC =0.69. The sensitivity was 78.0%, the specificity was 53.0%, and the accuracy was 60%. Our study showed that the sensitivity was 70.3%, the specificity was 89.3%, and the accuracy was 80.0%. These results were slightly better than those of Rios Velazquez et al.
Treatment with EGFR-TKIs prolongs progression-free survival in patients with EGFR-sensitive mutations. However, patients with primary resistance to treatment for EGFR-sensitive mutations cannot benefit from treatment of TKIs. The mechanism of primary resistance in patients with EGFR-sensitive mutations is still unclear. There is no definitive way to further screen for TKI sensitivity to benefit patients. This study attempts to solve this problem by applying the CT radiomics. Coroller et al. (21) extracted 15 imaging histological features from CT images of 127 non-small-cell lung cancer patients before neoadjuvant radiotherapy and chemotherapy and found that 8 imaging histological features could predict treatment response (AUC >0.6, P<0.05). In this study, 715 features were used for analysis, including 3 extracted from the plain scan, and 7 extracted during the arterial phase. Twenty-two features were extracted from the venous phase were able to distinguish TKI-sensitive populations. The AUC values from plain scans and the arterial and venous phases were 0.7268, 0.7793, and 0.9104, respectively. These findings indicate that there is a significant difference in the tumor tissue of patients who are sensitive to TKI treatment and that in patients with primary drug resistance to TKIs. This potential difference can be distinguished by the imaging histology obtained by CT.
Studies have shown that the heterogeneity of lung cancer may be related to genetic subtype mutations or to changes in related growth and angiogenic factors and in the tumor microenvironment (16). The intratumoral density provided by CT images can indirectly provide relevant information for tumor heterogeneity analysis from an overall level. In previous studies, radiomics features were generally extracted from a single phase. Although both plain and enhanced scan images reflect density information, plain scans mainly reflect differences in tissue components, cell density, necrosis, hemorrhage, and cystic changes. The density in secondary scans is heterogeneous, and enhanced scans can also reflect the heterogeneity of the blood supply of the tumor. The radiomics method can quantify these differences.
To further clarify the predictive performance of each phase in radiomics, this study used multiphase CT images as the research object and obtained imaging features from each different time phase to predict EGFR mutation status and TKI sensitivity. Therapeutic sensitivity has a high diagnostic value. The AUC value of the imaging phenotype was the largest in the venous phase for the following reasons: the capillary cells of normal lung tissue are continuous with each other, the cells are tightly connected, and the basement membrane is intact; thus, macromolecular contrast agent cannot leak into the intercellular space. Tumor blood vessels have different characteristics from normal blood vessels: the microvessels in the tumor are immature, the basement membrane is incomplete, and the permeability of the microvessels in lung cancer is increased, which facilitates the leakage of macromolecular contrast agent into the microvessels and the intercellular space. Therefore, intravenous injection of a contrast agent easily penetrates into the surrounding tissues, and the tumor heterogeneity is more obvious with the high background of the leaked contrast agent (17-19,22). The AUC values of EGFR mutations predicted in phase III of this study and those of the imaging phenotypes of the populations sensitive to TKI treatment were also the highest during the venous phase after enhancement; these were higher than values obtained during the plain scan and arterial phase.
The angiographic features of EGFR mutation status and TKI treatment sensitivity were predicted by different time phases. The AUC values of the features after wavelet transform were the highest. The EGFR mutation status group had the following results predicted: During the plain scan period, the neighborhood gray difference matrix was detected after the wavelet transform. The AUC value of intensity was 0.663, the AUC value of the first-order skewness feature obtained during the arterial phase was 0.696 after wavelet transform, and the AUC value of the grayscale zone matrix uniformity feature obtained during the venous phase was 0.7 after wavelet transform. The TKI treatment sensitivity group had the following results predicted: the AUC value of the neighborhood grayscale difference matrix contrast feature after wavelet transform was 0.688, and the neighborhood grayscale difference matrix intensity feature obtained during the arterial phase had an AUC value of 0.686. The venous phase was wavelet transformed. The gray level cooccurrence matrix feature had an AUC value of 0.703. Aerts et al. (15) used wavelets to analyze the stability of image features and obtained the four most stable results. Two of them were wavelet features. The results of the wavelet transform feature ensured the repeatability and stability of the feature analysis.
All the patients included in this study had advanced lung adenocarcinoma (stage IIIB-IV), which limits the use of this method in patients with early stage disease. However, there is potential value in differentiating patients who will benefit from EGFR-TKI sensitivity, and in subsequent studies, we will include early lung cancer patients to increase the sample size. This study was a retrospective analysis. In clinical trials, prospective collection of independent cohorts is used as a reference standard to verify a definitive statistical association or biological marker. Further prospective studies will be conducted to further verify the effectiveness of the prediction model.
In conclusion, CT imaging features can predict the EGFR mutation status and screen a sensitive population for TKI treatment, especially features obtained during the venous phase.
Acknowledgments
Funding:
Footnote
Reporting Checklist: The authors have completed the STARD reporting checklist. Available at http://dx.doi.org/10.21037/tcr-20-1216
Data Sharing Statement: Available at http://dx.doi.org/10.21037/tcr-20-1216
Conflicts of Interest: All authors have completed the ICMJE uniform disclosure form (available at http://dx.doi.org/10.21037/tcr-20-1216). The authors have no conflicts of interest to declare.
Ethical Statement: The authors are accountable for all aspects of the work in ensuring that questions related to the accuracy or integrity of any part of the work are appropriately investigated and resolved. The study was conducted in accordance with the Declaration of Helsinki (as revised in 2013). The study was approved by the Institutional Review Board of Shandong Tumor Hospital and Institute (No. 201705021) and individual consent for this retrospective analysis was waived. Medical record review was performed in accordance with Institutional Ethics Review Board guidelines.
Open Access Statement: This is an Open Access article distributed in accordance with the Creative Commons Attribution-NonCommercial-NoDerivs 4.0 International License (CC BY-NC-ND 4.0), which permits the non-commercial replication and distribution of the article with the strict proviso that no changes or edits are made and the original work is properly cited (including links to both the formal publication through the relevant DOI and the license). See: https://creativecommons.org/licenses/by-nc-nd/4.0/.
References
- Halpenny DF, Riely GJ, Hayes S, et al. Are there imaging characteristics associated with lung adenocarcinomas harboring ALK rearrangements Lung Cancer 2014;86:190-4. [Crossref] [PubMed]
- Ganeshan B, Panayiotou E, Burnand K, et al. Tumour heterogeneity in non small-cell lung carcinoma assessed by CT texture analysis: a potential marker of survival. Eur Radiol 2012;22:796-802. [Crossref] [PubMed]
- Bittner N, Ostoros G, Géczi L. New treatment options for lung adenocarcinoma-in view of molecular background. Pathol Oncol Res 2014;20:11-25. [Crossref] [PubMed]
- Paez JG, Janne PA, Lee JC, et al. EGFR mutations in lung cancer: correlation with clinical response to gefitinib therapy. Science 2004;304:1497-500. [Crossref] [PubMed]
- Novello S. Epidermal growth factor receptor tyrosine kinase inhibitors as adjuvant therapy in completely resected non-small-cell lung cancer. J Clin Oncol 2015;33:3985-6. [Crossref] [PubMed]
- Soria JC, Wu YL, Nakagawa K, et al. Gefitinib plus chemotherapy versus placebo plus chemotherapy in EGFR -mutation-positive non-small-cell lung cancer after progression on first-line gefitinib (IMPRESS): a phase 3 randomised trial. Lancet Oncol 2015;16:990-8. [Crossref] [PubMed]
- Sequist LV, Martins RG, Spigel D, et al. First-line gefitinib in patients with advanced non-small-cell lung cancer harboring somatic EGFR mutations. J Clin Oncol 2008;26:2442-9. [Crossref] [PubMed]
- Lynch TJ, Bell DW, Sordella R, et al. Activating mutations in the epidermal growth factor receptor underlying responsiveness of non-small-cell lung cancer to gefitinib. N Engl J Med 2004;350:2129-39. [Crossref] [PubMed]
- Maemondo M, Inoue A, Kobayashi K, et al. Gefitinib or chemotherapy for non-small-cell lung cancer with mutated EGFR. N Engl J Med 2010;362:2380-8. [Crossref] [PubMed]
- Lee JK, Shin J, Kim S, et al. Primary resistance to epidermal growth factor receptor (EGFR) tyrosine kinase inhibitors (TKIs) in patients with non-small-cell lung cancer harboring TKI-sensitive EGFR mutations: an exploratory study. Ann Oncol 2013;24:2080-7. [Crossref] [PubMed]
- Bedard PL, Hansen AR, Ratain MJ, et al. Tumour heterogeneity in the clinic. Nature 2013;501:355-64. [Crossref] [PubMed]
- Swanton C. Intratumor heterogeneity: evolution through space and time. Cancer Res 2012;72:4875-82. [Crossref] [PubMed]
- Taniguchi K, Okami J, Kodama K, et al. Intratumor heterogeneity of epidermal growth factor receptor mutations in lung cancer and its correlation to the response to gefitinib. Cancer Sci 2008;99:929-35. [Crossref] [PubMed]
- Lambin P, Rios-Velazque Z, Leijenaa R, et al. Radiomics: extracting more information from medical images using advanced feature analysis. Eur J Cancer 2012;48:441-6. [Crossref] [PubMed]
- Aerts HJ, Velazquez ER, Leijenaar RT, et al. Decoding tumour phenotype by noninvasive imaging using a quantitative radiomics approach. Nat Commun 2014;5:4006. [Crossref] [PubMed]
- Gerlinger M, Rowan AJ, Horswell S, et al. Intratumor heterogeneity and branched evolution revealed by multiregion sequencing. N Engl J Med 2012;366:883-92. [Crossref] [PubMed]
- Miles KA. Functional computed tomography in oncology. Eur J Cancer 2002;38:2079-84. [Crossref] [PubMed]
- Purdie TG, Henderson E, Lee TY. Functional CT imaging of angiogenesis in rabbit VX2 soft-tissue tumour. Phys Med Biol 2001;46:3161-75. [Crossref] [PubMed]
- Konerding MA, Fait E, Gaumann A. 3D microvascular architecture of pre -cancerous lesions and invasive carcimomas of the colon. Br J Cancer 2001;84:1354-62. [Crossref] [PubMed]
- Rios Velazquez E, Parmar C, Liu Y, et al. Somatic mutations drive distinct imaging phenotypes in lung cancer. Cancer Res 2017;77:3922-30. [Crossref] [PubMed]
- Coroller TP, Agrawal V, Narayan V, et al. Radiomic phenotype features predict pathological response in non-small cell lung cancer. Radiother Oncol 2016;119:480-6. [Crossref] [PubMed]
- Miles KA. Tumour angiogenesis and its relation to contrast enhancement on computed tomography: a review. Eur J Radiol 1999;30:198-205. [Crossref] [PubMed]