Non-SMC condensin I complex subunit H (NCAPH), a regulator of cell cycle, predicts poor prognosis in lung adenocarcinoma patients: a study mainly based on TCGA and GEO database
Introduction
Lung cancer, the leading cause of cancer-related mortality, is a major disease that threatens human health (1-4). As far as the progression in surgery, radiotherapy and chemotherapy, the 5-year survival rate of lung cancer is only 15% (5). However, the adoption of immunotherapies and targeted therapies have increased the 5-year survival rate obviously (range from 15% to 50%) (6,7). Lung adenocarcinoma (LUAD) is the main sub-type of lung cancer, and in recent years the incidence of LUAD has increased distinctly. So, it’s obliged to find new therapeutic targets for LUAD.
Condensin, a multiprotein complex, serves as a regulator of chromosome-wide gene. During mitosis and meiosis, It plays an important role in the process of chromosome assembly and segregation, regulating the cell cycle (8). There are two kinds of condensin complex: condensin I and condensin II. Condensing I complex is composed of structural maintenance of chromosomes (SMC) proteins and three non-SMC subunits, including non-SMC condensin I complex subunit H (NCAPH), subunit I (NCAPG) and subunit D2 (NCAPD2) (9). NCAPH is up-regulated in many kinds of tumor tissue, such as prostate cancer, pancreatic cancer and hepatocellular carcinoma, and it often predicts poor prognosis (10-12), regulating the cell cycle, migration and invasion. These studies proved that NCAPH could be an important therapeutic target for cancer. But in colon cancer, NCAPH is positively correlated with prognosis (13). Till now, the exact role of NCAPH in LUAD and its possible upstream regulation mechanism remain unclearly.
In order to answer this question, The Cancer Genome Atlas (TCGA) database and Gene Expression Omnibus (GEO) database were analyzed with online tools. We demonstrate that NCAPH is up-regulated in LUAD compared with adjacent normal tissues. What’s more, the survival analysis revealed that LUAD patients with high level of NCAPH had worse survival time. Potential mechanism and its upstream microRNAs (miRNAs) were also analyzed by online tools, such as cBioPortal, GEPIA, UALCAN and TargetScan. In conclusion, our study offers a new potential therapeutic target for the treatment of LUAD. We present the following article in accordance with the TRIPOD reporting checklist (available at http://dx.doi.org/10.21037/tcr-20-2217).
Methods
Analysis of TCGA and GEO database
TCGA database (https://www.cancer.gov/tcga), a landmark cancer genomics program, was created by the National Cancer Institute and the National Human Genome Research Institute in 2006. It includes genomic, epigenomic, transcriptomic, and proteomic data of 33 cancer types, as well as the matched normal samples. In order to view the expression profile of NCAPH in pan-cancer, we use a web-based database-GEPIA (http://gepia.cancer-pku.cn). This database could analyze the RNA sequencing expression data from the TCGA and the GTEx projects (14). For LUAD patients, the relationship between clinicopathological parameters and NCAPH expression was explored by UALCAN (http://ualcan.path.uab.edu/index.html) (15), which is an interactive and user-friendly web resource mainly based on TCGA data. The enrolled clinicopathological features included gender, age, tumor stage, lymph node metastasis status and smoking history.
GEO database (https://www.ncbi.nlm.nih.gov/geo/) was furtherly mined for demonstrate the deferent expression level of NCAPH mRNA between the LUAD tissue and normal lung tissue. GEO database is a good helper for providing users with array- and sequence-based data. In this study, the RNA-seq data of GSE19188 (16), GSE7670 (17) and GSE10072 (18) were extracted and analyzed by GEO2R online tool. The study was conducted in accordance with the Declaration of Helsinki (as revised in 2013).
Human Protein Atlas (HPA) database analysis
Expression level of NCAPH mRNA and protein in human normal organs were retrieved by HPA database (https://www.proteinatlas.org) (19). This database contains immunohistochemistry (IHC) staining pictures of 20 LUAD tissues and 6 normal lung tissues. According to the IHC staining intensity, the picture was scored from 0 to 3 (0, negative; 1, weak staining; 2, moderate staining; 3, strong staining). Based on the percentage of stained cells, the picture was scored from 0 to 3 (0, none; 1, <25%; 2, 25–75%; 3, >75%). The IHC score for every picture was calculated by multiplying the staining intensity score with the staining extent score, so the IHC score ranges from 0 to 12 for each sample.
The Kaplan Meier plotter database analysis
The Kaplan Meier plotter (https://kmplot.com/analysis/)includes gene chip and RNA-seq data of 20 different cancer types. The system data mainly comes from GEO and TCGA database, which can make a meta-analysis based discovery and validation of survival biomarkers (20). By using the Kaplan Meier plotter database, we examined the relationship between NCAPH mRNA expression and the overall survival (OS) time of 720 LUAD patients. All the patients were divided into two groups (high vs. low) by median expression value of NCAPH mRNA. Then the web-based tool can calculate the log-rank P value, hazard ratio (HR), and 95% confidence interval (CI) directly. OS time, FP (first progression time), and PPS (post progression survival time) were used to estimate the prognosis of LUAD.
GO and KEGG pathway analysis of co-expression genes of NCAPH
Firstly, we explored the co-expressed genes of NCAPH in LUAD patients using cBioPortal database (http://www.cbioportal.org/). With the help of cBioPortal database, we can download the co-expressed genes of NCAPH in LUAD patients based on TCGA data (21). In order to obtain the accurate co-expressed genes, we used two other databases: GEPIA and UALCAN. Then we intersected the results obtained from these three databases through a website tool-VENNY (https://bioinfogp.cnb.csic.es/tools/venny/).
Secondly, for the aim to understand the potential biological process and pathway, the terminal results of co-expressed genes were furtherly processed with gene ontology (GO) and Kyoto Encyclopedia of Genes and Genomes (KEGG) pathway analysis. The Database for Annotation, Visualization and Integrated Discovery (DAVID) (https://david.ncifcrf.gov) was accepted for these analyses (22). We visualized the GO and KEGG analysis results using R/Bio conductor (version 3.26.5).
PPI network construction and hub-gene analysis
STRING (version 11.0) is a web-based tool (https://string-db.org) for exploring the known and predicted protein-protein interactions (PPI) (23). We inputted the co-expressed genes of NCAPH in LUAD patients into the STRING database, then it could analyze the PPI network automatically. In order to get the hub-genes in the PPI network, the cytoHubba app of Cytoscape software (version 3.6.1) was utilized. The top nine genes ranked by degree were calculated and visualized (24).
Prediction of potential miRNAs that regulate NCAPH
TargetScan (version 7.2, http://www.targetscan.org/vert_72/) predicts potential miRNAs for mRNA by searching for the seed region of miRNA that matches to human 3' UTRs (25). OncomiR database (http://www.oncomir.org/) is an open-source platform for pan-cancer miRNA dysregulation in cancer (26). MiRNAs and its target genes are negatively correlated. So, we searched OncomiR database for down-regulated miRNAs in LUAD tissues. To further narrow down the range of target genes, miRNAs positively related with survival time were also analyzed using OncomiR database. Then, we got 9 common miRNAs of the three data sets. ENCORI (http://starbase.sysu.edu.cn/) was an online tool for analyzing TCGA RNA-Seq data of many kinds of cancers including LUAD (27). Then, we used ENCORI database to assess the expression correlation of NCAPH mRNA and these 9 miRNAs.
The analysis of potential chemicals that down-regulate NCAPH
CTD (http://ctdbase.org/) is a publicly available database that can be used to analyze the chemical-gene interactions (28). We searched chemicals that could down-regulate NCAPH mRNA or protein. Chemicals with at least 2 references were treated as potential drugs. The structure of potential chemicals was furtherly downloaded by using PubChem database (https://pubchem.ncbi.nlm.nih.gov/).
Statistical analysis
Statistics analysis were carried out using GraphPad Prism 7, and the results are presented as the mean ± SD. Differences between two groups was evaluated by Student’s t-test (unpaired, two-tailed). One-way ANOVA was used for the data subject to normal distribution. P<0.05 was considered significant.
Results
Expression levels of NCAPH in human normal and cancer tissues
In order to clarify the expression level of NCAPH mRNA in normal tissues, the HPA database was used. The results proved that the level of NCAPH mRNA was very low in many normal tissues, such as lung, cerebral cortex, adipose tissue, cervix, and uterine, etc. (Figure 1A). However, in LUAD as well as many other types of cancers, the NCAPH mRNA expression level increased significantly in comparison with normal tissues (Figure 1B).
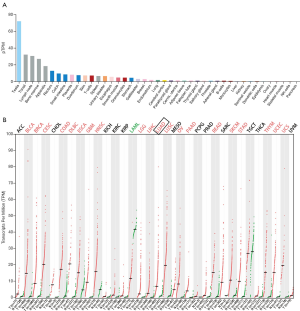
Elevated NCAPH mRNA in LUAD based on TCGA and GEO
ULCAN, the online analysis tool of TCGA, showed that NCAPH mRNA expression level was higher in LUAD (Figure 2A). Then we investigated the relationship between the NCAPH mRNA in LUAD and the clinicopathological parameters. LUAD patients from stage I to stage IV got a higher level of NCAPH mRNA compared with normal lung tissues (Figure 2B). The results showed that patients with all kinds of subtype such as nodal metastasis status (Figure 2C), gender (Figure 2D), age (Figure 2E), and smoking habits (Figure 2F) got higher NCAPH mRNA levels compared with normal lung tissues. We also explored the expression of NCAPH mRNA in GEO database. GSE19188, GSE7670 and GSE10072 were enrolled in our study. NCAPH mRNA levels were relatively higher in LUAD patients in the three datasets (Figure 2G,H,I).
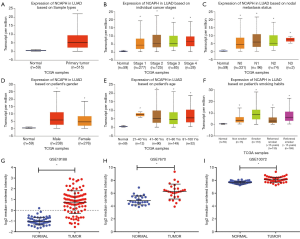
Increased expression of NCAPH protein in LUAD
Proteins of cells are the fundamental of biological function. In order to study the expression of NCAPH protein, we check the HPA database. There are two kinds of antibody for NCAPH: HPA002647 and HPA003008. Each antibody staining group concluded 3 IHC images of normal lung tissue and 10 IHC images of LUAD tissue. As shown in Figure 3, the IHC images of LUAD got a higher IHC score compared with normal tissues. This result demonstrated that the expression level of NCAPH protein was increased in LUAD tissues in comparison with normal lung tissues.
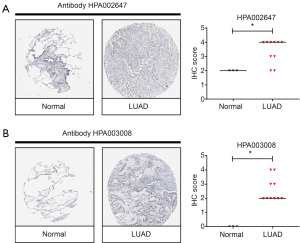
The prognostic role of NCAPH in LUAD patients
The prognostic role of NCAPH In LUAD patients was mined by using the Kaplan Meier plotter database. The probe name of NCAPH was 212949_at. As shown in Figure 4, increased level of NCAPH indicated a significantly worse prognosis in the aspects of OS time (Figure 4A) and FP time (Figure 4B). But, no significant difference was seen between low and high NCAPH expression patients in PPS time (Figure 4C).
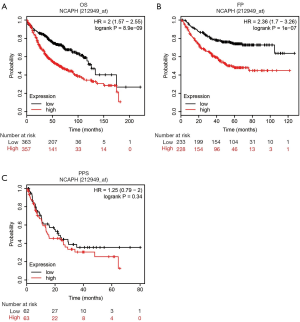
GO functional, KEGG pathway enrichment and PPI network analysis of co-expressed genes of NCAPH
As shown in Figure 5A, we analyzed the co-expressed genes of NCAPH using three databases: UALCAN, cBioPortal and GEPIA. The number of co-expressed genes in the three databases were 3,182, 13,480 and 200 separately. One hundred and seventy-five co-expressed genes were in commonly shared. In order to understand the function of these genes, GO functional analysis (cellular component, molecular function and biological process) and KEGG pathway enrichment analysis were performed by using DAVID database (Table 1).

Table 1
Category | Term | Gene count | P value |
---|---|---|---|
GOTERM_CC_FAT | GO:0005694—chromosome | 57 | 5.72E-46 |
GOTERM_CC_FAT | GO:0044427—chromosomal part | 50 | 1.16E-40 |
GOTERM_CC_FAT | GO:0043232—intracellular non-membrane-bounded organelle | 99 | 2.27E-40 |
GOTERM_CC_FAT | GO:0043228—non-membrane-bounded organelle | 99 | 2.27E-40 |
GOTERM_CC_FAT | GO:0005819—spindle | 37 | 2.65E-40 |
GOTERM_CC_FAT | GO:0015630—microtubule cytoskeleton | 55 | 3.55E-39 |
GOTERM_CC_FAT | GO:0000793—condensed chromosome | 33 | 5.77E-36 |
GOTERM_CC_FAT | GO:0000775—chromosome, centromeric region | 31 | 2.39E-33 |
GOTERM_CC_FAT | GO:0044430—cytoskeletal part | 56 | 7.13E-28 |
GOTERM_CC_FAT | GO:0000779—condensed chromosome, centromeric region | 21 | 1.35E-24 |
GOTERM_MF_FAT | GO:0005524—ATP binding | 56 | 4.19E-20 |
GOTERM_MF_FAT | GO:0032559—adenyl ribonucleotide binding | 56 | 7.84E-20 |
GOTERM_MF_FAT | GO:0030554—adenyl nucleotide binding | 56 | 8.73E-19 |
GOTERM_MF_FAT | GO:0001883—purine nucleoside binding | 56 | 1.75E-18 |
GOTERM_MF_FAT | GO:0001882—nucleoside binding | 56 | 2.39E-18 |
GOTERM_MF_FAT | GO:0032555—purine ribonucleotide binding | 57 | 1.69E-16 |
GOTERM_MF_FAT | GO:0032553—ribonucleotide binding | 57 | 1.69E-16 |
GOTERM_MF_FAT | GO:0017076—purine nucleotide binding | 57 | 1.53E-15 |
GOTERM_MF_FAT | GO:0000166—nucleotide binding | 58 | 3.63E-13 |
GOTERM_MF_FAT | GO:0003777—microtubule motor activity | 12 | 1.96E-10 |
GOTERM_BP_FAT | GO:0007049—cell cycle | 103 | 1.90E-88 |
GOTERM_BP_FAT | GO:0022403—cell cycle phase | 77 | 8.97E-74 |
GOTERM_BP_FAT | GO:0000279—M phase | 72 | 9.33E-74 |
GOTERM_BP_FAT | GO:0022402—cell cycle process | 83 | 1.35E-71 |
GOTERM_BP_FAT | GO:0000278—mitotic cell cycle | 69 | 3.24E-65 |
GOTERM_BP_FAT | GO:0000280—nuclear division | 58 | 3.88E-63 |
GOTERM_BP_FAT | GO:0007067—mitosis | 58 | 3.88E-63 |
GOTERM_BP_FAT | GO:0000087—M phase of mitotic cell cycle | 58 | 1.23E-62 |
GOTERM_BP_FAT | GO:0048285—organelle fission | 58 | 5.02E-62 |
GOTERM_BP_FAT | GO:0051301—cell division | 55 | 9.61E-51 |
KEGG_PATHWAY | hsa04110: cell cycle | 26 | 1.41E-26 |
KEGG_PATHWAY | hsa03030: DNA replication | 12 | 5.00E-14 |
KEGG_PATHWAY | hsa04114: oocyte meiosis | 13 | 1.44E-09 |
KEGG_PATHWAY | hsa04914: progesterone-mediated oocyte maturation | 11 | 2.12E-08 |
KEGG_PATHWAY | hsa03430: mismatch repair | 7 | 1.19E-07 |
KEGG_PATHWAY | hsa04115: p53 signaling pathway | 7 | 8.78E-05 |
KEGG_PATHWAY | hsa03440: homologous recombination | 5 | 2.22E-04 |
KEGG_PATHWAY | hsa03420: nucleotide excision repair | 4 | 0.012179 |
KEGG_PATHWAY | hsa03410: base excision repair | 3 | 0.056228 |
KEGG_PATHWAY | hsa00240: pyrimidine metabolism | 4 | 0.08622 |
The top ten GO terms were enriched shown in Figure 5B (cellular component), Figure 5C (molecular function) and Figure 5D (biological process). The mainly enriched items for cellular component were chromosome, chromosomal part, intracellular non-membrane-bounded organelle, non-membrane-bounded organelle and spindle. As far as molecular function items, ATP binding, adenyl nucleotide binding, purine nucleoside binding and nucleoside binding were significantly enriched. The co-expressed genes mainly participated the biological processes of cell cycle, cell cycle phase, M phase, cell cycle process, and mitotic cell cycle. The mainly enriched KEGG pathway items were cell cycle, DNA replication, oocyte meiosis, progesterone-mediated oocyte maturation, and mismatch repair (Figure 5E).
The PPI network analysis was performed by using STRING database and Cytoscape software, containing 175 nodes and 7,840 edges. To find out the key role genes in the network, the cytoHubba app based on Cytoscape software was utilized. Nodes’ degree were calculated and ranked by degree from high to low. The top 9 genes were CDK1, BUB1, BUB1B, CCNB1, CCNA2, KIF11, TOP2A, CDC45, CDC20 (Figure 5F).
Validation of the prognosis role of co-expression hub genes
We searched the GEPIA database to furtherly validate the prognosis role of co-expression hub genes. As shown in Figure 6, The top 9 genes, CDK1, BUB1, BUB1B, CCNB1, CCNA2, KIF11, TOP2A, CDC45, and CDC20, acted as onco-gene role in LUAD. Patients with high level of these gene were proved to have worse prognosis compared with that with low level.
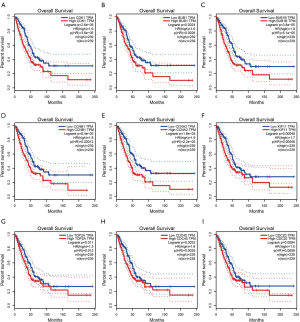
Prediction of miRNAs that regulate NCAPH
To predict miRNAs that regulate NCAPH, TargetScan database was used to find the potential miRNAs. The results contained 1,075 miRNAs with conserved sites or poorly conserved sites. After searching OncomiR database, we found 123 miRNAs with significantly increased expression level in LUAD. Moreover, there were 66 miRNAs that had negative correlated relationship with the prognosis in LUAD based on Oncomir. After we drawn the Venn picture of the three miRNA lists, 9 miRNA were found in common: miR-1976, miR-1468-5p, miR-195-3p, miR-490-3p, miR-133b, miR-497-5p, miR-195-5p, miR-125a-5p, miR-500a-3p (Figure 7A). Then, we explored the expression relationship between NCAPH mRNA and each miRNA of the 9 miRNAs. miR-1976, miR-1468-5p, miR-195-3p, miR-490-3p, miR-133b, miR-497-5p, miR-195-5p, and miR-125a-5p had negative correlation with NCAPH mRNA (r<0, P<0.05, Figure 7B,C,D,E,F,G,H,I) except miR-500a-3p (r>0, P<0.05, Figure 7J). The prognostic values of 8 potential miRNAs in LUAD were shown in Table 2 based on Oncomir database.
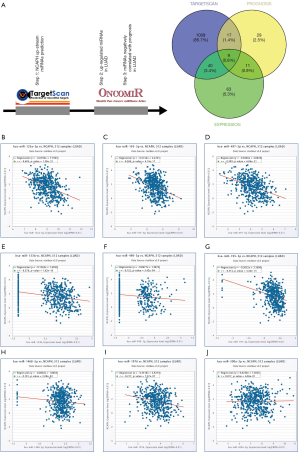
Table 2
miRNA name | Log rank P value | Deceased Log2 mean expression | Living Log2 mean expression | T-test P value |
---|---|---|---|---|
hsa-miR-1976 | 2.46E-02 | 3.69 | 3.95 | 5.15E-04 |
hsa-miR-1468-5p | 1.16E-03 | 1.86 | 2.37 | 3.51E-04 |
hsa-miR-195-3p | 4.97E-03 | 1.6 | 1.87 | 2.37E-04 |
hsa-miR-490-3p | 1.23E-02 | 0.1 | 0.6 | 5.47E-02 |
hsa-miR-133b | 9.99E-03 | 0.51 | 0.7 | 1.75E-01 |
hsa-miR-497-5p | 1.02E-02 | 4.23 | 4.51 | 7.35E-04 |
hsa-miR-195-5p | 3.48E-02 | 5.19 | 5.28 | 2.98E-01 |
hsa-miR-125a-5p | 2.35E-02 | 9.09 | 9.27 | 1.48E-02 |
Potential chemicals that down-regulate NCAPH
In order to find out chemicals that suppress the NCAPH, we used the CTD database. As a result, 5 chemicals (cyclosporin A, bisphenol A, methyl methanesulfonate, doxorubicin, valproic acid) were identified to decrease the expression of NCAPH mRNA with ≥2 references. The structure was furtherly downloaded by using PubChem database (Figure 8).
Discussion
The knowledge about the prognostic role of NCAPH in LUAD is very poor. In this study, we aimed to demonstrate that NCAPH expression level was elevated in LUAD and predicted poor prognosis. Systematic analysis of the NCAPH expression level in LUAD patients was performed by using TCGA, GEO, and HPA databases from two aspects: mRNA level and protein level (29-31). The co-expression genes enriched analysis suggested that NCAPH may play an important role in cell cycle in LUAD.
Similarly, in prostate cancer, pancreatic cancer and hepatocellular carcinoma, NCAPH is up-regulated and often predicts poor prognosis (10-12). With the help of co-expression network analysis, 9 top enriched co-expressed genes (CDK1, BUB1, BUB1B, CCNB1, CCNA2, KIF11, TOP2A, CDC45, CDC20) were distinguished and considered to play important role in cell cycle. Previous studies showed that CDK1 and BUB1 were negatively related with LUAD prognosis (32). CCNB1, CCNA2 and BUB1B were regulators in cell cycle and proliferation (33-35). KIF11 could control bipolar spindle formation and chromosomal stability (36). TOP2A was considered to be a bad prognosis factor in LUAD (37). These previous studies proved the reliability of our conclusions. We argued that NCAPH probably promoted cell proliferation through cell cycle pathway in LUAD. However, elevated expression of NCAPH meant longer OS time in patients with colon cancer (13). The contradiction may be due to the biological differences of variant tumors.
Why was the NCAPH expression level elevated in LUAD. In order to answer this question, we analyzed the miRNAs in LUAD, which could potentially participate in post-transcriptional regulation of many kinds of proteins. Previous study showed that miRNAs could sponge 3' UTR of its target mRNA (38). We identified 8 aberrantly down-regulated miRNAs that could potentially inhibit the expression of NCAPH. The correlation analysis of miRNAs and NCAPH mRNA was carried out based on ENCORI, which contained TCGA RNA-Seq data of LUAD. So, the results were more convincing. Among these 8 miRNAs, miR-1976, miR-490-3p, miR-133b, miR-497-5p, miR-195-5p, miR-125a-5p were previously reported as tumor inhibitors in non-small cell lung cancer (39-44). These results are consistent with our findings. Hence, we assume that NCAPH might be down-regulated by these 8 miRNAs.
New therapeutic target means a lot to the conquer of cancer. Considering the role of NCAPH as a potential therapeutic target, the CTD database was utilized for finding the chemicals which could down-regulate the NCAPH expression level. Five kinds of chemicals were identified based on previous studies in the database. The problem that whether these chemicals could inhibit the LUAD would be answered with our next experiments.
Despite the big data analysis was used in this study, there are still some limitations: Firstly, lack of the validation in clinical LUAD samples. Secondly, the expression levels of proteins are regulated by many factors, such as promoter methylation, transcription factor and ubiquitination (45,46). These factors were not analyzed in our study. Thirdly, the onco-gene role of NCAPH was not testified invitro and in vivo. These shortcomings will be solved in the next experiment.
Conclusions
In conclusion, we propose that NCAPH expression in LUAD is a poor prognostic indicator. It may play an important role in cell cycle. In the near future, it may be a potential therapeutic target of LUAD.
Acknowledgments
Funding: We thank financial support from
Footnote
Reporting Checklist: The authors have completed the TRIPOD reporting checklist. Available at http://dx.doi.org/10.21037/tcr-20-2217
Conflicts of Interest: All authors have completed the ICMJE uniform disclosure form (available at http://dx.doi.org/10.21037/tcr-20-2217). The authors have no conflicts of interest to declare.
Ethical Statement: The authors are accountable for all aspects of the work in ensuring that questions related to the accuracy or integrity of any part of the work are appropriately investigated and resolved. The study was conducted in accordance with the Declaration of Helsinki (as revised in 2013).
Open Access Statement: This is an Open Access article distributed in accordance with the Creative Commons Attribution-NonCommercial-NoDerivs 4.0 International License (CC BY-NC-ND 4.0), which permits the non-commercial replication and distribution of the article with the strict proviso that no changes or edits are made and the original work is properly cited (including links to both the formal publication through the relevant DOI and the license). See: https://creativecommons.org/licenses/by-nc-nd/4.0/.
References
- Torre LA, Siegel RL, Jemal A. Lung Cancer Statistics. Adv Exp Med Biol 2016;893:1-19. [Crossref] [PubMed]
- Park CK, Kim SJ. Trends and Updated Statistics of Lung Cancer in Korea. Tuberc Respir Dis (Seoul) 2019;82:175-7. [Crossref] [PubMed]
- Feng RM, Zong YN, Cao SM, et al. Current cancer situation in China: good or bad news from the 2018 Global Cancer Statistics? Cancer Commun (Lond) 2019;39:22. [Crossref] [PubMed]
- Chen W, Zheng R, Baade PD, et al. Cancer statistics in China, 2015. CA Cancer J Clin 2016;66:115-32. [Crossref] [PubMed]
- Strano S, Lupo A, Lococo F, et al. Prognostic significance of vascular and lymphatic emboli in resected pulmonary adenocarcinoma. Ann Thorac Surg 2013;95:1204-10. [Crossref] [PubMed]
- Reck M, Rodriguez-Abreu D, Robinson AG, et al. Updated Analysis of KEYNOTE-024: Pembrolizumab Versus Platinum-Based Chemotherapy for Advanced Non-Small-Cell Lung Cancer With PD-L1 Tumor Proportion Score of 50% or Greater. J Clin Oncol 2019;37:537-46. [Crossref] [PubMed]
- Ramalingam SS, Vansteenkiste J, Planchard D, et al. Overall Survival with Osimertinib in Untreated, EGFR-Mutated Advanced NSCLC. N Engl J Med 2020;382:41-50. [Crossref] [PubMed]
- Hirano T. Condensins: universal organizers of chromosomes with diverse functions. Genes Dev 2012;26:1659-78. [Crossref] [PubMed]
- Schleiffer A, Kaitna S, Maurer-Stroh S, et al. Kleisins: A superfamily of bacterial and eukaryotic SMC protein partners. Mol Cell 2003;11:571-5. [Crossref] [PubMed]
- Cui F, Hu J, Xu Z, et al. Overexpression of NCAPH is upregulated and predicts a poor prognosis in prostate cancer. Oncol Lett 2019;17:5768-76. [Crossref] [PubMed]
- Kim JH, Youn Y, Kim KT, et al. Non-SMC condensin I complex subunit H mediates mature chromosome condensation and DNA damage in pancreatic cancer cells. Sci Rep 2019;9:17889. [Crossref] [PubMed]
- Sun C, Huang S, Wang H, et al. Non-SMC condensin I complex subunit H enhances proliferation, migration, and invasion of hepatocellular carcinoma. Mol Carcinog 2019;58:2266-75. [Crossref] [PubMed]
- Yin L, Jiang LP, Shen QS, et al. NCAPH plays important roles in human colon cancer. Cell Death Dis 2017;8:e2680. [Crossref] [PubMed]
- Tang Z, Li C, Kang B, et al. GEPIA: a web server for cancer and normal gene expression profiling and interactive analyses. Nucleic Acids Res 2017;45:W98-W102. [Crossref] [PubMed]
- Chandrashekar DS, Bashel B, Balasubramanya SAH, et al. UALCAN: A Portal for Facilitating Tumor Subgroup Gene Expression and Survival Analyses. Neoplasia 2017;19:649-58. [Crossref] [PubMed]
- Hou J, Aerts J, den Hamer B, et al. Gene expression-based classification of non-small cell lung carcinomas and survival prediction. PLoS One 2010;5:e10312. [Crossref] [PubMed]
- Su LJ, Chang C, Wu Y, et al. Selection of DDX5 as a novel internal control for Q-RT-PCR from microarray data using a block bootstrap re-sampling scheme. Bmc Genomics 2007;8:140. [Crossref] [PubMed]
- Landi MT, Dracheva T, Rotunno M, et al. Gene expression signature of cigarette smoking and its role in lung adenocarcinoma development and survival. PLoS One 2008;3:e1651. [Crossref] [PubMed]
- Pontén F, Schwenk JM, Asplund A, et al. The Human Protein Atlas as a proteomic resource for biomarker discovery. J Intern Med 2011;270:428-46. [Crossref] [PubMed]
- Nagy Á, Lánczky A, Menyhárt O, et al. Validation of miRNA prognostic power in hepatocellular carcinoma using expression data of independent datasets. Sci Rep 2018;8:9227. [Crossref] [PubMed]
- Cerami E, Gao J, Dogrusoz U, et al. The cBio cancer genomics portal: an open platform for exploring multidimensional cancer genomics data. Cancer Discov 2012;2:401-4. [Crossref] [PubMed]
- Huang da W. Sherman BT, Lempicki RA. Systematic and integrative analysis of large gene lists using DAVID bioinformatics resources. Nat Protoc 2009;4:44-57. [Crossref] [PubMed]
- Szklarczyk D, Gable A, Lyon D, et al. STRING v11: protein-protein association networks with increased coverage, supporting functional discovery in genome-wide experimental datasets. Nucleic Acids Res 2019;47:D607-13. [Crossref] [PubMed]
- Chin CH, Chen S, Wu H, et al. cytoHubba: identifying hub objects and sub-networks from complex interactome. BMC Syst Biol 2014;8:S11. [Crossref] [PubMed]
- Agarwal V, Bell G, Nam J, et al. Predicting effective microRNA target sites in mammalian mRNAs. Elife 2015;4:e05005. [Crossref] [PubMed]
- Wong NW, Chen Y, Chen S, et al. OncomiR: an online resource for exploring pan-cancer microRNA dysregulation. Bioinformatics 2018;34:713-5. [Crossref] [PubMed]
- Li JH, Liu S, Zhou H, et al. starBase v2.0: decoding miRNA-ceRNA, miRNA-ncRNA and protein-RNA interaction networks from large-scale CLIP-Seq data. Nucleic Acids Res 2014;42:D92-7. [Crossref] [PubMed]
- Davis AP, Grondin C, Johnson R, et al. The Comparative Toxicogenomics Database: update 2019. Nucleic Acids Res 2019;47:D948-54. [Crossref] [PubMed]
- Hu J, Zhou L, Song Z, et al. The identification of new biomarkers for bladder cancer: A study based on TCGA and GEO datasets. J Cell Physiol 2019; [Epub ahead of print]. [Crossref] [PubMed]
- Wang Y, Chen L, Wang G, et al. Fifteen hub genes associated with progression and prognosis of clear cell renal cell carcinoma identified by coexpression analysis. J Cell Physiol 2019;234:10225-37. [Crossref] [PubMed]
- Wang J, Wang Y, Kong F, et al. Identification of a six-gene prognostic signature for oral squamous cell carcinoma. J Cell Physiol 2020;235:3056-68. [Crossref] [PubMed]
- Zhang L, He M, Zhu W, et al. Identification of a panel of mitotic spindle-related genes as a signature predicting survival in lung adenocarcinoma. J Cell Physiol 2020;235:4361-75. [Crossref] [PubMed]
- Li J, Qian W, Sun Q. Cyclins regulating oocyte meiotic cell cycle progression. Biol Reprod 2019;101:878-81. [Crossref] [PubMed]
- Zhang S, Tischer T, Barford D. Cyclin A2 degradation during the spindle assembly checkpoint requires multiple binding modes to the APC/C. Nat Commun 2019;10:3863. [Crossref] [PubMed]
- Tian JH, Mu L, Wang M, et al. BUB1B Promotes Proliferation of Prostate Cancer via Transcriptional Regulation of MELK. Anticancer Agents Med Chem 2020;20:1140-6. [Crossref] [PubMed]
- Venuto S, Monteonofrio L, Cozzolino F, et al. TRIM8 interacts with KIF11 and KIFC1 and controls bipolar spindle formation and chromosomal stability. Cancer Lett 2020;473:98-106. [Crossref] [PubMed]
- Guo W, Sun S, Guo L, et al. Elevated TOP2A and UBE2C expressions correlate with poor prognosis in patients with surgically resected lung adenocarcinoma: a study based on immunohistochemical analysis and bioinformatics. J Cancer Res Clin Oncol 2020;146:821-41. [Crossref] [PubMed]
- Thomson DW, Dinger M. Endogenous microRNA sponges: evidence and controversy. Nat Rev Genet 2016;17:272-83. [Crossref] [PubMed]
- Zheng H, Wu J, Shi J, et al. miR-125a-5p upregulation suppresses the proliferation and induces the cell apoptosis of lung adenocarcinoma by targeting NEDD9. Oncol Rep 2017;38:1790-6. [Crossref] [PubMed]
- Chen G, Hu J, Huang Z, et al. MicroRNA-1976 functions as a tumor suppressor and serves as a prognostic indicator in non-small cell lung cancer by directly targeting PLCE1. Biochem Biophys Res Commun 2016;473:1144-51. [Crossref] [PubMed]
- Gu H, Yang T, Fu S, et al. MicroRNA-490-3p inhibits proliferation of A549 lung cancer cells by targeting CCND1. Biochem Biophys Res Commun 2014;444:104-8. [Crossref] [PubMed]
- Li G, Wang K, Wang J, et al. miR-497-5p inhibits tumor cell growth and invasion by targeting SOX5 in non-small-cell lung cancer. J Cell Biochem 2019;120:10587-95. [Crossref] [PubMed]
- Luo J, Pan J, Jin Y, et al. MiR-195-5p Inhibits Proliferation and Induces Apoptosis of Non-Small Cell Lung Cancer Cells by Targeting CEP55. Onco Targets Ther 2019;12:11465-74. [Crossref] [PubMed]
- Zhen Y, Liu J, Huang Y, et al. miR-133b Inhibits Cell Growth, Migration, and Invasion by Targeting MMP9 in Non-Small Cell Lung Cancer. Oncol Res 2017;25:1109-16. [Crossref] [PubMed]
- Li Y, Sun Q, Jiang M, et al. KLF9 suppresses gastric cancer cell invasion and metastasis through transcriptional inhibition of MMP28. FASEB J 2019;33:7915-28. [Crossref] [PubMed]
- Liu L, Yu L, Zeng C, et al. E3 Ubiquitin Ligase HRD1 Promotes Lung Tumorigenesis by Promoting Sirtuin 2 Ubiquitination and Degradation. Mol Cell Biol 2020;40:e00257-19. [Crossref] [PubMed]