Identification of potential microRNAs in glioblastoma using bioinformatic analysis and prognostic evaluation
Introduction
Glioblastoma (GB) is the most common and invasive brain malignancy (1), accounting for 45.2% of malignant primary and central nervous system tumors (2) and about 4% of cancer deaths worldwide. Despite combinatorial treatment of surgery, chemotherapy, and radiation therapy, the prognosis of patients with GB is still poor, with a median survival rate of only 12–15 months (3). The occurrence and development of GB are complex and reproducible diagnoses are poor, demonstrating an inability to accurately predict sensitivity or resistance to chemotherapy schemes. It is therefore necessary to understand the relevant molecular mechanisms underlying GB to improve early diagnosis, prognosis, and treatment prediction for better outcomes.
MicroRNAs (miRNAs) are small endogenous single-stranded, non-coding RNAs approximately 21–25 nucleotides in length. MiRNAs regulate gene expression by binding to complementary mRNA sequences (4) in a variety of biological processes (BPs) including cell proliferation, apoptosis, cell cycle, differentiation, and DNA repair (5). Luo et al. demonstrated that several miRNAs act as oncogenes or tumor suppressors, as they regulate many pathways such as cell proliferation, apoptosis resistance, autophagy, invasion and metastasis, angiogenesis, and drug resistance in tumors (6). In this study, we detected differentially expressed miRNAs (DE-miRNAs) in GB tissues compared to normal tissues by analyzing the GSE103228 dataset downloaded from the Gene Expression Omnibus (GEO) database. We further analyzed these DE-miRNAs using Geno Ontology (GO) and Kyoto Encyclopedia of Genes and Genomes (KEGG). Potential target genes of these DE-miRNAs were predicted using the miRTarBase, and the protein-protein interaction (PPI) network was constructed using the STRING database. Hub genes were identified using Cytoscape software and the expression levels and prognostic roles of these hub genes were further validated using the University of Alabama Cancer (UALCAN) database (7). Two miRNAs—miR-218-5p and miR-148a-3p—in GB were detected and evaluated using the UALCAN and Oncolnc databases, among which miR-148a-3p was determined to be an important prognostic predictor of outcomes in GB patients.
We present the following article in accordance with the MDAR checklist (available at http://dx.doi.org/10.21037/tcr-20-2487).
Methods
MiRNA microarray
The microarray dataset GSE103228 was downloaded from the National Center for Biotechnology Information GEO database (http://www.ncbi.nlm.nih.gov/geo). This dataset contained five GB tissues (GSM2758534–GSM2758538) and five normal tissues (GSM2758539–GSM2758543). All the tissues were from Chinese. The study was conducted in accordance with the Declaration of Helsinki (as revised in 2013).
Screening for DE-miRNAs
DE-miRNAs between GB tissues and normal tissues were analyzed using the GEO2R analytic tool in the GEO database. A P value <0.05 and |fold change (FC)| >2 (|log2FC| >1)were set as the thresholds for detecting DE-miRNAs.
Predicting target genes of DE-miRNAs
The miRTarBase (http://mirtarbase.mbc.nctu.edu.tw/php/index.php), which is an experimentally validated miRNA-target interactions database, was used to predict targets of the top three most down-regulated and up-regulated DE-miRNAs (8).
GO annotation and KEGG pathway enrichment analyses
The database for annotation, visualization, and integrated discovery (DAVID, http://david-d.ncifcrf.gov/) was used to perform GO annotation and KEGG pathway enrichment analyses for the predicted target genes of six DE-miRNAs (9).
Construction and analysis of the PPI network and miRNA-target network
The PPI network was established using the STRING database (http://string-db.org) (10) to access the interactive relationship of the top three most down-regulated and up-regulated DE-miRNAs. The Cytoscape software (version 3.6.1) was used to analyze the degree of connectivity. According to degree, 20 hub genes were identified in the PPT network (11) that were used to develop miRNA-hub gene networks using the Cytoscape software.
Validation of target gene expression using the UALCAN database
The UALCAN database (http://ualcan.path.uab.edu/), which is a portal for facilitating tumor subgroup gene expression and survival analyses, provides easy access to publicly available cancer transcriptome data including the Cancer Genome Atlas (TCGA) (7). Expression levels of the predicted target genes of DE-miRNAs were further validated using the UALCAN database. The normal and tumor groups were statistically compared using the log-rank test with a P value <0.05 in the database.
Survival analysis using the Oncolnc database
The Oncolnc database (http://www.oncolnc.org/) was used to investigate the prognostic values of miR-218-5p and miR-148a-3p in GB. MiR-218-5p and miR-148a-3p were loaded into the Oncolnc system to generate Kaplan-Meier survival plots using the log-rank test. A P value <0.05 was considered statistically significant.
Statistical analysis
The results are presented as mean ± standard deviation (SD) and differences between two groups were determined using a two-tailed Student’s t-test. A two-tailed P value <0.05 was considered statistically significant.
Results
Identification of DE-miRNAs and their targets in GB
In total, 49 significant DE-miRNAs were identified (Figure 1), among which 30 DE-miRNAs were down-regulated and 19 were up-regulated. The top 10 most up-regulated and down-regulated DE-miRNAs are listed in Table 1. The volcano plot of the 49 DE-miRNAs is shown in Figure 1.
Table 1
Down-regulated | Up-regulated | |||||
---|---|---|---|---|---|---|
miRNA-ID | P value | LogFC | miRNA-ID | P value | LogFC | |
hsa-miR-873-5p | 0.0000191 | –5.261868 | hsa-miR-148a-3p | 0.0126427 | 6.297996 | |
hsa-miR-218-5p | 0.0273504 | –4.64753 | hsa-miR-590-5p | 0.0226093 | 5.749235 | |
hsa-miR-144-5p | 0.0364835 | –4.499103 | hsa-miR-455-3p | 0.0099771 | 5.476172 | |
hsa-miR-136-5p | 0.0447164 | –4.454104 | hsa-miR-301b | 0.0102319 | 4.950535 | |
hsa-miR-29c-5p | 0.014377 | –4.253382 | hsa-miR-20a-3p | 0.0080751 | 4.752412 | |
hsa-miR-129-5p | 0.0002705 | –3.765884 | hsa-miR-494 | 0.0152322 | 4.458626 | |
hsa-miR-3200-3p | 0.0067668 | –3.740519 | hsa-miR-4692 | 0.0156984 | 3.978466 | |
hsa-miR-128 | 0.0019142 | –3.642832 | hsa-miR-21-3p | 0.0005109 | 3.903087 | |
hsa-miR-544a | 0.0152057 | –3.565528 | hsa-miR-3529-3p | 0.0262743 | 3.264283 | |
hsa-miR-19b-2-5p | 0.0318371 | –3.414159 | hsa-miR-5681a | 0.0137035 | 3.209292 |
miRNAs, microRNAs; FC, fold change.
A total of 2,181 potential target genes were predicted from the first three most prominent miRNAs using miRTarBase, among which 1,118 genes were down-regulated and 1,063 genes were up-regulated. Three miRNAs (has-miR-873-5p, has-miR-218-5p and has-miR-144-5p) were the most significantly down-regulated, whereas has-miR-148a-3p, has-miR-590-5p and has-miR-455-3p were the most significantly up-regulated.
GO annotation and KEGG pathway analyses
The target genes were categorized into BP, cellular component (CC), and molecular function (MF). The top 20 GO terms of the target genes predicted by the top three most down-regulated DE-miRNAs are shown in Figure S1. For the BP category, the candidate target genes were significantly enriched in regulation of transcription and regulation of macromolecule metabolic process. For the CC category, the candidate target genes were enriched in non-membrane-bounded organelle, and intracellular non-membrane-bounded organelle. For the MF category, the target genes were significantly enriched in nucleotide binding, purine ribonucleotide binding, ribonucleotide binding, and purine nucleotide binding.
As we can see, the target genes were enriched in several pathways, including pathways in cancer, regulation of actin cytoskeleton, and focal adhesion.
As shown in Figure S2, the target genes of the top three most upregulated DE-miRNAs in the BP category were significantly enriched in regulation of transcription and negative regulation of macromolecule metabolic process. The target genes in the CC category were significantly enriched in membrane-enclosed lumen, organelle lumen, and intracellular organelle lumen. The target genes in the MF category were significantly enriched in DNA binding and nucleotide binding. KEGG pathway analysis showed that the target genes were significantly enriched in pathways in cancer, cell cycle, and endocytosis.
PPI network and miRNA-target network
The candidate target genes of the three most down-regulated and up-regulated DE-miRNAs were mapped into the STRING database to construct the PPI network and identify hub genes using the Cytoscape software. As shown in Table 2, the top 20 hub nodes were determined according to degree. For the target genes of the three most down-regulated DE-miRNAs, the top 20 hub genes were ACTB, CDK6, DICER1, CCNE1, CDKN1B, CCND3, BCL2L1, ATM, CREBBP, BIRC5, CDH2, ABL1, CCNF, BUB1, CCNE2, ACTN1, CD44, AGO1, AXIN1, and AGO2, among which ACTB showed the highest node degree (degree =29). For the target genes of the three most up-regulated DE-miRNAs, the top 20 hub genes were MYC, STAT3, HSP90AA1, CDKN1A, HSPA4, SMAD3, DICER1, FOXO3, CYCS, SMAD2, CDKN1B, CCNA2, BCL2L11, DNMT1, KDM6B, AGO2, AGO1, AURKB, YWHAB and HSP90B1, among which MYC showed the highest node degree (degree =58).
Table 2
Up-regulated | Down-regulated | |||
---|---|---|---|---|
Gene | Degree | Gene | Degree | |
MYC | 58 | ACTB | 29 | |
STAT3 | 47 | CDK6 | 26 | |
HSP90AA1 | 43 | DICER1 | 23 | |
CDKN1A | 32 | CCNE1 | 22 | |
HSPA4 | 28 | CDKN1B | 20 | |
SMAD3 | 28 | CCND3 | 19 | |
DICER1 | 27 | BCL2L1 | 19 | |
FOXO3 | 27 | ATM | 19 | |
CYCS | 25 | CREBBP | 19 | |
SMAD2 | 24 | BIRC5 | 18 | |
CDKN1B | 23 | CDH2 | 18 | |
CCNA2 | 23 | ABL1 | 17 | |
BCL2L11 | 23 | CCNF | 16 | |
DNMT1 | 22 | BUB1 | 15 | |
KDM6B | 22 | CCNE2 | 15 | |
AGO2 | 21 | ACTN1 | 15 | |
AGO1 | 20 | CD44 | 15 | |
AURKB | 20 | AGO1 | 14 | |
YWHAB | 20 | AXIN1 | 14 | |
HSP90B1 | 20 | AGO2 | 14 |
The interactive relationships between the top 20 hub genes according to degree are shown in Figure 2.
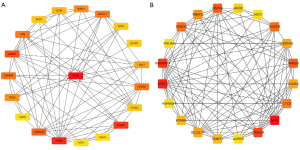
As shown in Figure 3A, miR-218-5p was the most important miRNA in the regulatory network of the three most down-regulated miRNAs, regulating 16 of the 20 hub genes. miR-148a-3p was the most important up-regulated miRNA genes in the regulatory networks of the three most upregulated miRNAs (Figure 3B), regulating 17 of the 20 hub genes. These results indicate the important regulatory roles of miR-218-5p and miR-148a-3p in the development of GB.
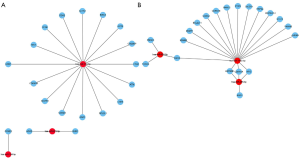
Expression levels of target genes in the UALCAN database
To further validate the association of miR-218-5p and miR-148a-3p with the development of GB, the miRNA levels of the target genes in the TCGA database were validated using the UALCAN database. As shown in Figure S3 five (MYC, STAT3, CCNA2, BCL2L11, and AURKB) of the 16 target genes corresponding to the up-regulated miR-148a-3p were significantly up-regulated, whereas two (HSP90AA1 and YWHAB) were significantly down-regulated. Because gene expression is modulated solely by negative regulation of miRNAs, we hypothesized that the two significantly down-regulated genes (HSP90AA1 and YWHAB) might be targets of miR-148a-3p. Four (ACTB, BIRC5, CDH2, and CDK6) of the 16 target genes corresponding to the down-regulated miR-218-5p were significantly up-regulated, suggesting that these genes might be targets of miR-218-5p, these results were shown in Figure S4.
Prognostic impacts of miR-218-5p and miR-148a-3p
The relationship between the expression levels of miR-218-5p and miR-148a-3p with the prognosis of patients with GB was validated using the Oncolnc database. As shown in Figure 4A, patients with higher levels of miR-148a-3p had poorer prognosis compared with those with lower levels (P<0.001). However, no correlation between the level of miR-218-5p and the survival of GB patients (P>0.05) was detected (Figure 4B). These findings suggest that miR-148a-3p was aberrantly expressed in GB and might serve as a prognostic biomarker.
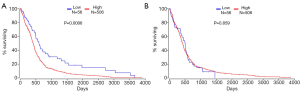
Discussion
GB is a high-grade glioma with high incidence and very poor prognosis (12). Standard treatment consists of maximal surgical resection, radiotherapy, and concomitant and adjuvant chemotherapy with temozolomide. Despite advances in cancer therapy, GB treatment remains difficult, in that no contemporary treatments are curative. MiRNAs have been demonstrated to play a very important role in the regulation and development of tumors, including breast cancer, lung cancer, and GB, by modulating tumor suppressor genes, oncogenes, and other downstream signaling molecules (13). In the present study, we identified 49 DE-miRNAs for GB and predicted 1,118 and 1,063 potential target genes from the top three most up-regulated and down-regulated DE-miRNAs, respectively. miR-218-5p and miR-148a-3p regulated most of the hub genes in the PPI network, and miR-148a-3p was significantly associated with prognostic outcomes of GB patients. The findings of the present study provide new insights for therapeutic treatments of GB.
MiRNAs are a group of small, endogenous non-coding RNAs with a variety of biological functions, including modulation of cell metabolism and cell survival (14). Godlewski et al. reported that miR-128 reduced glioma cell proliferation in vitro and glioma xenograft growth in vivo (15). MiR-21 is a recognized oncogene that modulates tumor cell proliferation, migration, invasion, and apoptosis by regulating the epidermal growth factor receptor (EGFR) and matrix metalloproteinases (MMP) pathway and inhibiting programmed cell death 4 (PDCD4) expression (16-18). MiR-34a is recognized as a master regulator of tumor suppression and inhibits the occurrence of cancer by targeting p53 and Bcl-2 (19,20). In this study, we identified 30 down-regulated miRNAs and 19 up-regulated miRNAs for GB. According to node degree, the top 20 hub genes of the three most up-regulated miRNAs and the three most down-regulated miRNAs were selected to construct a miRNA-gene network. Most of the hub genes in this network were potentially modulated by miR-218-5p and miR-148a-3p. Xu et al. revealed that inhibiting miR-218-5p altered cell growth and metastasis in cervical cancer (21). MiR-218-5p can regulate proliferation, apoptosis, and invasion of glioma cells by targeting LHFPL3 (22). To the best of our knowledge, we are the first to demonstrate that miR-218-5p might be involved in several pathways (ACTB, BIRC5, CDH2, and CDK6) affecting the occurrence of GB. Recent studies have suggested an association of miR-148a-3p with the proliferation and invasion of various types of cancer, including bladder cancer, breast cancer, esophageal cancer, and non-small-cell lung cancer (23-26). Our results demonstrate that miR-148a-3p was not only related to GB occurrence, but also potentially associated with overall survival (OS) of GB patients.
We demonstrated that aberrant expression of miR-218-5p and miR-148a-3p is crucial in the regulation of GB cells. miR-148a-3p could serve as a prognostic biomarker for GB and as a future therapeutic candidate for GB patients. Further study is needed to validate our findings and determine the potential for using miR-218-5p and miR-148a-3p as convenient diagnostic and prognostic markers for GB.
Acknowledgments
The authors would like to thank the staff for their technical assistance and assistance in data collection.
Funding: This work was supported by
Footnote
Reporting Checklist: The authors have completed the MDAR checklist. Available at http://dx.doi.org/10.21037/tcr-20-2487
Conflicts of Interest: All authors have completed the ICMJE uniform disclosure form (available at http://dx.doi.org/10.21037/tcr-20-2487). The authors have no conflicts of interest to declare.
Ethical Statement: The authors are accountable for all aspects of the work in ensuring that questions related to the accuracy or integrity of any part of the work are appropriately investigated and resolved. The study was conducted in accordance with the Declaration of Helsinki (as revised in 2013).
Open Access Statement: This is an Open Access article distributed in accordance with the Creative Commons Attribution-NonCommercial-NoDerivs 4.0 International License (CC BY-NC-ND 4.0), which permits the non-commercial replication and distribution of the article with the strict proviso that no changes or edits are made and the original work is properly cited (including links to both the formal publication through the relevant DOI and the license). See: https://creativecommons.org/licenses/by-nc-nd/4.0/.
References
- Thakkar JP, Dolecek TA, Horbinski C, et al. Epidemiologic and molecular prognostic review of glioblastoma. Cancer Epidemiol Biomarkers Prev 2014;23:1985-96. [Crossref] [PubMed]
- Ostrom QT, Gittleman H, Farah P, et al. CBTRUS statistical report: Primary brain and central nervous system tumors diagnosed in the United States in 2006-2010. Neuro Oncol 2013;15:ii1-56. [Crossref] [PubMed]
- Nicholas MK. Glioblastoma multiforme: evidence-based approach to therapy. Expert Rev Anticancer Ther 2007;7:S23-7. [Crossref] [PubMed]
- Bartel DP. MicroRNAs: target recognition and regulatory functions. Cell 2009;136:215-33. [Crossref] [PubMed]
- Shukla GC, Singh J, Barik S. MicroRNAs: processing, maturation, target recognition and regulatory functions. Mol Cell Pharmacol 2011;3:83-92. [PubMed]
- Luo JW, Wang X, Yang Y, et al. Role of micro-RNA (miRNA) in pathogenesis of glioblastoma. Eur Rev Med Pharmacol Sci 2015;19:1630-9. [PubMed]
- Chandrashekar DS, Bashel B, Balasubramanya SAH, et al. UALCAN: a portal for facilitating tumor subgroup gene expression and survival analyses. Neoplasia 2017;19:649-58. [Crossref] [PubMed]
- Chou CH, Shrestha S, Yang CD, et al. miRTarBase update 2018: a resource for experimentally validated microRNA-target interactions. Nucleic Acids Res 2018;46:D296-302. [Crossref] [PubMed]
- Huang DW, Sherman BT, Lempicki RA. Systematic and integrative analysis of large gene lists using DAVID bioinformatics resources. Nat Protoc 2009;4:44-57. [Crossref] [PubMed]
- Szklarczyk D, Franceschini A, Wyder S, et al. STRING v10: protein-protein interaction networks, integrated over the tree of life. Nucleic Acids Res 2015;43:D447-52. [Crossref] [PubMed]
- Shannon P, Markiel A, Ozier O, et al. Cytoscape: a software environment for integrated models of biomolecular interaction networks. Genome Res 2003;13:2498-504. [Crossref] [PubMed]
- Shea A, Harish V, Afzal Z, et al. MicroRNAs in glioblastoma multiforme pathogenesis and therapeutics. Cancer Med 2016;5:1917-46. [Crossref] [PubMed]
- Banelli B, Forlani A, Allemanni G, et al. MicroRNA in glioblastoma: an overview. Int J Genomics 2017;2017:7639084. [Crossref] [PubMed]
- Zhu B, Gong Y, Yan G, et al. Down-regulation of lncRNA MEG3 promotes hypoxia-induced human pulmonary artery smooth muscle cell proliferation and migration via repressing PTEN by sponging miR-21. Biochem Biophys Res Commun 2018;495:2125-32. [Crossref] [PubMed]
- Godlewski J, Nowicki MO, Bronisz A, et al. Targeting of the Bmi-1 oncogene/stem cell renewal factor by microRNA-128 inhibits glioma proliferation and self-renewal. Cancer Res 2008;68:9125-30. [Crossref] [PubMed]
- Gabriely G, Wurdinger T, Kesari S, et al. MicroRNA 21 promotes glioma invasion by targeting matrix metalloproteinase regulators. Mol Cell Biol 2008;28:5369-80. [Crossref] [PubMed]
- Gaur AB, Holbeck SL, Colburn NH, et al. Downregulation of Pdcd4 by mir-21 facilitates glioblastoma proliferation in vivo. Neuro Oncol 2011;13:580-90. [Crossref] [PubMed]
- Zhou X, Ren Y, Moore L, et al. Downregulation of miR-21 inhibits EGFR pathway and suppresses the growth of human glioblastoma cells independent of PTEN status. Lab Invest 2010;90:144-55. [Crossref] [PubMed]
- Gao H, Zhao H, Xiang W. Expression level of human miR-34a correlates with glioma grade and prognosis. J Neurooncol 2013;113:221-8. [Crossref] [PubMed]
- Luan S, Sun L, Huang F. MicroRNA-34a: a novel tumor suppressor in p53-mutant glioma cell line U251. Arch Med Res 2010;41:67-74. [Crossref] [PubMed]
- Xu Y, He Q, Lu Y, et al. MicroRNA-218-5p inhibits cell growth and metastasis in cervical cancer via LYN/NF-κB signaling pathway. Cancer Cell Int 2018;18:198. [Crossref] [PubMed]
- Li Z, Qian R, Zhang J, et al. MiR-218-5p targets LHFPL3 to regulate proliferation, migration, and epithelial-mesenchymal transitions of human glioma cells. Biosci Rep 2019;39:BSR20180879. [Crossref] [PubMed]
- Ashizawa M, Okayama H, Ishigame T, et al. microRNA-148a-3p regulates immunosuppression in DNA mismatch repair-deficient colorectal cancer by targeting PD-L1. Mol Cancer Res 2019;17:1403-13. [Crossref] [PubMed]
- Wang Y, Hu Y, Guo J, et al. miR-148a-3p suppresses the proliferation and invasion of esophageal cancer by targeting DNMT1. Genet Test Mol Biomarkers 2019;23:98-104. [Crossref] [PubMed]
- Xiang M, Yuan W, Zhang W, et al. Expression of miR-490-5p, miR-148a-3p and miR-608 in bladder cancer and their effects on the biological characteristics of bladder cancer cells. Oncol Lett 2019;17:4437-42. [Crossref] [PubMed]
- Xie Q, Yu Z, Lu Y, et al. microRNA-148a-3p inhibited the proliferation and epithelial-mesenchymal transition progression of non-small-cell lung cancer via modulating Ras/MAPK/Erk signaling. J Cell Physiol 2019;234:12786-99. [Crossref] [PubMed]