A comprehensive assessment of the prognostic role of cell adhesion molecules in acute myeloid leukemia
Introduction
Acute myeloid leukemia (AML) is the most common malignant type of leukemia in adults, is associated with clonal hematopoietic stem-cell disorders, and has shown a disparate response to therapy (1). Although the majority of patients with newly diagnosed AML experience complete morphologic remission following treatment with intensive induction chemotherapy, the outcome in older patients who are unable to receive intensive chemotherapy without unacceptable side effects remains dismal (1,2). Decisions about the choice of postremission therapy in patients with AML currently depend on the identification of a selected set of genetic markers at diagnosis and the detection of residual disease with multiparameter flow cytometry (3). Quantitative molecular evaluation during complete remission could further improve prognostication of outcomes in patients with AML (4). Emerging immunotherapies such as chimeric antigen receptor T cells have advanced the treatment of acute lymphoblastic leukemia (5); so far, most of the targets have been membrane proteins and members of cell adhesion molecule (CAM) sets (6).
The CAMs are specific proteins, which expressed on the cell surface (7). They have been reported to play a critical role in multiple biologic processes, including hemostasis, the immune response, inflammation, embryogenesis, and development of neuronal tissue. There are four main groups: the integrin family, immunoglobulin superfamily, selectins, and cadherins. Membrane proteins that mediate immune cell–cell interactions fall into different categories, namely those involved in antigen recognition, costimulation, and cellular adhesion. Adhesion plays an important role both in normal hematopoiesis and in AML (8). Blasts of AML express many of the CAMs identified on normal hematopoietic precursors. The differential expression of CAMs between normal hematopoietic cells and leukemic blasts has been documented as differently expressed, likely reflecting the heterogeneity of the disease (9). A variety of processes within the bone marrow (BM) are governed by CAMs, including migration, homing, and quiescence. The AML blasts home to BM, as the CAM-mediated interaction with the niche protects them from chemotherapeutic agents. On the contrary, they then detach from the niches and move from the BM into the peripheral blood to colonize other sites such as the spleen and liver, possibly in a process that is reminiscent of epithelial-to-mesenchymal-transition in metastatic solid cancers (10). The expression of CAMs has a prognostic impact and there are ongoing efforts to therapeutically target adhesion in the fight against leukemia (11).
In this study, we elucidate the transcriptional pattern of CAMs in a cohort of 173 patients with AML. All data collected from a precise published work and complete follow-up information were also available. We focused on the prognostic role of assembled genes and sole CAMs, and further sought the independent prognostic factors, which might play crucial roles in the development of AML and emerge as potential immunotherapy targets. We present the following article in accordance with the MADR reporting checklist (available at http://dx.doi.org/10.21037/tcr-20-3315).
Methods
Patients and RNA-seq
The normalized RNA sequencing data of 200 patients were obtained from a public database (cbioportal.org), and the corresponding clinical records were also collected (12). In the previously published study, patients underwent pathological diagnosis and detailed clinical features were recorded, including age, gender, and grade. All 200 patients were diagnosed with AML according to the histological records. Gene expressional values were shown as messenger (m)RNA z-score data and compared between each subject. Collectively, there were 27 cases missing related transcriptional information, and 173 eligible cases were enrolled in the final study. All public omics data sets used were generated by previous studies and ethical approval was granted prior to their use. The study was conducted in accordance with the Declaration of Helsinki (as revised in 2013).
Bioinformatics
Genes relevant to CAMs statistics annotated in the Kyoto Encyclopedia of Genes and Genomes (KEGG) database (kegg.jp/hsa04514) were enrolled in the current study (13). After excluding 8 genes lacking expressional information, the profiles of 141 genes involved in cell adhesion were assessed in AMLs. A cluster analysis of the genetic expression of integral gene sets was performed to distinguish samples based on gene expression profiles. Participants with similar gene expression patterns were identified from the entire population. The transcriptional levels were shown as mRNA z-scores and clustered using the hierarchical clustering algorithm via a Stanford program (14). The cluster heat map and pattern according to tumor stage were generated with the Java Treeview program (jtreeview.sourceforge.net) (15) and GraphPad Prism 8.0 (GraphPad Software, Inc., San Diego, CA, USA; Version 8).
Prognostic relevance analyses
The prognostic roles of the chromatin remodeling related genes were investigated by comparing the survivals of different groups. The overall survival (OS), progression-free survival (PFS), disease-free survival (DFS), and disease-specific survival (DSS) were accessed using a GraphPad Prism program (GraphPad Software, Inc., San Diego, CA, USA; Version 8). Comparisons of survival in different clusters revealed the relevance of gene expressional profiles and the prognosis. Additionally, an analysis of the difference in OS between the cohorts with low or high expression levels of individual genes was conducted using GraphPad Prism 8.0.
Statistical analysis
Survival curves of different groups were plotted and compared using the log-rank test in GraphPad Prism 8.0. Differences in gene expression levels between clusters were detected using analysis of variance (ANOVA). Correlations between variables were determined by regression analyses. All tests were performed with the statistical software SPSS 24.0 (IBM, Inc., Armonk, NY, USA). Statistical significance was detected when a P value was <0.05.
Results
The expressional profile of CAMs was significantly associated with AML survival
To investigate the specific AML subpopulation, patients were divided into different groups according to similar CAMs expressing patterns. Primarily, there were two clusters which showed different expression models (Figure 1A). Compared to the outcomes in cluster_2, cluster_1 showed a better OS with an inapparent difference (26.3 vs. 17.0 months, P=0.2522); however, cluster_1 had a significantly prolonged PFS (34.1 vs. 13.8 months, P=0.0379) (Figure 1B). We also detected detailed subgroups according to different expression of CAMs, and identified four subclusters in total (Figure 1A). Comparison of prognoses of those subclusters showed that subcluster_1 clearly displayed the best OS and PFS (Figure 1C, upper). Furthermore, we compared the survivals of patients in subcluster_1 with the others and detected a notably favorable OS (46.5 vs. 15.8 months) and PFS (undefined period vs. 13.9 months) in subcluster_1, and the differences were significant (P=0.0421 and P=0.0137, respectively).
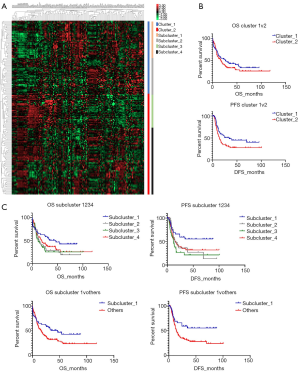
The expressions of human leukocyte antigen (HLA) were wildly varied in AML
After having identified specific AML cohorts with diverse prognoses, we assessed the differently expressed CAMs between each subgroup. There were 76 genes which showed discrepant expressional levels between cluster_1 and cluster_2, as shown in Figure 2A. Additionally, there were 67 genes showing discrepant expressional levels between subcluster_1 and other patients, as shown in Figure 2B. Comparison of the two differently expressed genes (DEGs) sets revealed 50 genes in the overlaps represented by HLAs. Multiple genes of HLAs members, such as HLA-DMB [DM beta chain] and HLA-DPA1 (DP alpha 1 chain) (Figure 2C), were significantly down-regulated in both cluster_1 and subcluster_1 (P<0.05). On the other side, genes like NTNG2, SPN, CNTN1, NRXN3, PVR and CLCN10 were significantly highly-regulated either in cluster_1 or subcluster_1 (P<0.05) (Table 1).
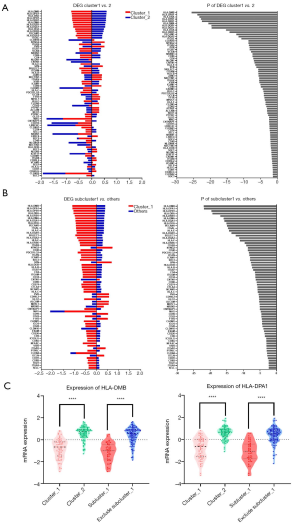
Table 1
Groups | OS (month) | P value | PFS (month) | P value |
---|---|---|---|---|
Primary | ||||
Cluster_1 | 26.3 | 0.2522 | 34.1 | 0.0379* |
Cluster_2 | 17.0 | 13.8 | ||
Subordinate | ||||
Cubcluster_1 | 46.5 | 0.0421* | Undefined | 0.0137* |
Others | 15.8 | 13.9 |
*P<0.05. OS, overall survival; PFS, progression free survival.
Expression of CAMs was correlated with AML prognosis
We arranged the AML participants in the order of survival status and assessed the prognosis-correlated genes from the CAMs. Finally, 21 genes were detected to be significantly associated with the OS of AML (r>0.15 or <−0.15, P<0.05) (Figure 3A). Among those genes, expressions of 10 genes were positively correlated to OS (e.g., SELE and NRCAM); expressions of 9 genes were negatively correlated to OS (e.g., L1CAM and PDCD1) (Tables 2,3). We performed multi-factor regression analysis in order to identify independently prognostic CAMs. In total, there were 5 genes, L1CAM, SDC1, NTNG1, CLDN14 and NRCAM, detected as independently correlated with OS. We compared the OS between the high expression subgroup and low expression subgroup regarding single genes. Among those genes, patients with up-regulated NRCAM showed a significantly prolonged OS comparing to the down-regulated cohort (27.0 vs. 11.8 months, P=0.0133) (Table 4, Figure 3B). Up-regulation of SDC1 also indicated a favorable prognosis in AML, but only with a slight significance (P=0.0531) (Table 4, Figure 3B). Moreover, we divided the entire participant cohort into two groups, a good prognosis group and poor prognosis group, according to their relevant OSs. There were 3 genes, CLDN14, ITGA4, and VCAM1, that were significantly correlated to the assignment to these groups (Table 4, Figure 3B). Among them, up-regulation of VCAM1 was notably correlated with favorable prognosis in AML (22.3 vs. 11.8 months, P=0.0449).
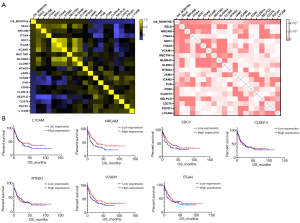
Table 2
Genes | Expression in subcluster_1 | Expression in subcluster_2 | P value |
---|---|---|---|
HLA-DMB | −1.09005 | 0.39482 | 1.17E-22 |
HLA-DPA1 | −1.08922 | 0.394519 | 1.29E-22 |
HLA-DPB1 | −1.04369 | 0.378035 | 2.24E-20 |
HLA-DRA | −1.03715 | 0.375663 | 4.52E-20 |
HLA-DRB1 | −0.98212 | 0.355725 | 1.12E-17 |
HLA-DMA | −0.95678 | 0.346553 | 1.15E-16 |
HLA-DQA1 | −0.95152 | 0.344645 | 1.84E-16 |
HLA-DOA | −0.93551 | 0.338843 | 7.45E-16 |
PECAM1 | −0.92947 | 0.336655 | 1.25E-15 |
ITGAL | −0.92201 | 0.33396 | 2.34E-15 |
HLA-E | −0.91268 | 0.330579 | 5.06E-15 |
SIGLEC1 | −0.95428 | 0.262643 | 4.97E-13 |
HLA-DQB1 | −0.89226 | 0.323181 | 2.61E-14 |
HLA-DQA2 | −0.87402 | 0.276047 | 5.84E-12 |
HLA-F | −0.81068 | 0.293637 | 9.86E-12 |
PDCD1LG2 | −0.84749 | 0.192393 | 5.04E-09 |
HLA-DRB5 | −0.75936 | 0.275041 | 2.61E-10 |
ITGB2 | −0.74179 | 0.268679 | 7.45E-10 |
CD40 | −0.72156 | 0.261351 | 2.38E-09 |
SDC3 | −0.74918 | 0.228917 | 1.1E-08 |
VCAN | −0.70289 | 0.254594 | 6.7E-09 |
ICAM1 | −0.69379 | 0.251296 | 1.09E-08 |
HLA-DOB | −0.67501 | 0.244497 | 2.93E-08 |
HLA-B | −0.66667 | 0.24147 | 4.48E-08 |
ITGB7 | −0.65125 | 0.235891 | 9.67E-08 |
CD274 | −0.68406 | 0.170463 | 1.34E-06 |
CD4 | −0.62595 | 0.226726 | 3.25E-07 |
ITGAM | −0.62033 | 0.224687 | 4.21E-07 |
CD34 | −0.61984 | 0.224508 | 4.31E-07 |
CTLA4 | −0.6669 | 0.176313 | 1.88E-06 |
CD86 | −0.60025 | 0.217407 | 1.05E-06 |
NCAM1 | −0.58578 | 0.212173 | 1.96E-06 |
MAG | −1.44184 | −0.64948 | 1.92E-05 |
HLA-C | −0.58124 | 0.210528 | 2.39E-06 |
GLG1 | −0.55807 | 0.202134 | 6.26E-06 |
CNTNAP1 | −0.54678 | 0.198043 | 9.84E-06 |
CADM1 | −0.56964 | 0.142971 | 4.61E-05 |
CD22 | −0.518 | 0.187615 | 2.98E-05 |
ICOS | −0.50532 | 0.183028 | 4.74E-05 |
CLDN4 | −0.92952 | −0.24651 | 0.001502 |
CD28 | −0.49706 | 0.180042 | 6.38E-05 |
ESAM | −0.46811 | 0.169554 | 0.000173 |
CD226 | −0.45431 | 0.164556 | 0.000273 |
NFASC | −0.61538 | 0.000243 | 0.000962 |
CD6 | −0.44748 | 0.162079 | 0.00034 |
ICOSLG | −0.41748 | 0.151217 | 0.000855 |
CD8A | −0.41444 | 0.150118 | 0.000935 |
VSIR | −0.41267 | 0.149471 | 0.000985 |
PTPRC | −0.40635 | 0.147178 | 0.001184 |
CDH1 | −0.43675 | 0.09549 | 0.002586 |
TIGIT | −0.39899 | 0.111005 | 0.004502 |
CD8B | −0.38757 | 0.113751 | 0.004476 |
ITGA9 | −0.35419 | 0.12829 | 0.004865 |
SELL | −0.35404 | 0.128235 | 0.004884 |
ITGAV | 0.411607 | −0.14909 | 0.001016 |
OCLN | 0.343257 | −0.301 | 0.001635 |
F11R | 0.510272 | −0.18482 | 3.96E-05 |
CD99 | 0.51868 | −0.18787 | 2.9E-05 |
MPZL1 | 0.549037 | −0.19886 | 9E-06 |
CLDN10 | 0.067996 | −0.68835 | 0.000118 |
ALCAM | 0.556498 | −0.20157 | 6.67E-06 |
CD99L2 | 0.561252 | −0.20329 | 5.5E-06 |
PVR | 0.567341 | −0.2055 | 4.28E-06 |
NRXN3 | 0.32957 | −0.48189 | 9.59E-06 |
CNTN1 | 0.14362 | −0.69853 | 5.62E-07 |
SPN | 0.681146 | −0.24672 | 2.13E-08 |
NTNG2 | 0.722824 | −0.2618 | 2.22E-09 |
Table 3
Genes | Expression in cluster_1 | Expression in cluster_2 | P value |
---|---|---|---|
HLA-DMB | −0.81386 | 0.594116 | 2.54E-26 |
HLA-DPA1 | −0.79287 | 0.578797 | 1.4E-24 |
HLA-DRA | −0.78532 | 0.573288 | 5.52E-24 |
HLA-DPB1 | −0.77898 | 0.568658 | 1.7E-23 |
HLA-DRB1 | −0.77563 | 0.566208 | 3.06E-23 |
HLA-DMA | −0.74841 | 0.546344 | 2.84E-21 |
HLA-DQB1 | −0.73524 | 0.536722 | 2.22E-20 |
HLA-DQA1 | −0.73474 | 0.536355 | 2.4E-20 |
HLA-DOA | −0.66189 | 0.483176 | 5.21E-16 |
HLA-DRB5 | −0.64205 | 0.46869 | 5.58E-15 |
HLA-DQA2 | −0.63298 | 0.410605 | 2.6E-12 |
PECAM1 | −0.59994 | 0.43795 | 5.62E-13 |
ITGAL | −0.56436 | 0.411991 | 1.85E-11 |
ITGB2 | −0.50369 | 0.367694 | 3.43E-09 |
ITGB7 | −0.49756 | 0.363226 | 5.54E-09 |
VCAN | −0.48534 | 0.354304 | 1.41E-08 |
CD86 | −0.47118 | 0.343958 | 4E-08 |
CD4 | −0.46371 | 0.338512 | 6.82E-08 |
HLA-E | −0.44415 | 0.324236 | 2.62E-07 |
SIGLEC1 | −0.49211 | 0.253827 | 2.05E-06 |
ITGAM | −0.41181 | 0.300617 | 2.07E-06 |
HLA-B | −0.41113 | 0.300124 | 2.15E-06 |
VSIR | −0.3992 | 0.291412 | 4.39E-06 |
ICAM1 | −0.3861 | 0.281859 | 9.32E-06 |
PDCD1LG2 | −0.45346 | 0.18552 | 9.3E-05 |
HLA-C | −0.34751 | 0.253686 | 7.28E-05 |
HLA-F | −0.31787 | 0.23205 | 0.000301 |
MAG | −1.14477 | −0.6524 | 0.003414 |
CLDN23 | −0.40655 | 0.076474 | 0.004711 |
PTPRC | −0.27473 | 0.200549 | 0.00188 |
GLG1 | −0.2595 | 0.189431 | 0.003372 |
CNTNAP1 | −0.25628 | 0.18708 | 0.0038 |
SDC2 | −0.51325 | −0.07472 | 0.008187 |
CD34 | −0.24505 | 0.17889 | 0.005699 |
SELL | −0.2397 | 0.174983 | 0.006873 |
CD40 | −0.23042 | 0.168201 | 0.00943 |
HLA-DOB | −0.22227 | 0.162263 | 0.012329 |
CADM1 | −0.25654 | 0.106812 | 0.022345 |
SDC3 | −0.2138 | 0.102174 | 0.047718 |
HLA-G | −0.18217 | 0.132977 | 0.040877 |
CLDN22 | −1.04872 | −0.89151 | 0.04586 |
CD40LG | 0.178337 | −0.13019 | 0.045359 |
CDH1 | 0.13824 | −0.18055 | 0.04523 |
SELP | 0.171721 | −0.15857 | 0.037128 |
CD99L2 | 0.194518 | −0.142 | 0.028863 |
ITGB8 | 0.179977 | −0.17933 | 0.022967 |
IGSF11 | −0.25645 | −0.62121 | 0.018708 |
VCAM1 | 0.043248 | −0.32297 | 0.030792 |
NLGN2 | 0.211922 | −0.15471 | 0.017124 |
SDC1 | 0.203079 | −0.17684 | 0.015446 |
LRRC4 | 0.235182 | −0.17168 | 0.008029 |
NECTIN3 | −0.05646 | −0.5135 | 0.010228 |
NRXN1 | −0.54641 | −1.01344 | 0.00734 |
LRRC4C | −0.64619 | −1.11556 | 0.004531 |
NLGN4X | −0.22064 | −0.69718 | 0.009706 |
NEO1 | 0.288804 | −0.21083 | 0.001065 |
CDH15 | −0.54425 | −1.05122 | 0.004088 |
ALCAM | 0.293719 | −0.21442 | 0.000867 |
PTPRF | 0.294229 | −0.21479 | 0.000849 |
CDH2 | −0.11512 | −0.66352 | 0.000543 |
MPZL1 | 0.327971 | −0.23942 | 0.000188 |
CLDN5 | 0.28767 | −0.28726 | 0.000306 |
F11R | 0.3331 | −0.24316 | 0.000148 |
CADM3 | −0.34015 | −0.94207 | 4.44E-05 |
CD80 | 0.380555 | −0.27781 | 1.27E-05 |
ITGAV | 0.400541 | −0.2924 | 4.06E-06 |
NECTIN1 | 0.40057 | −0.29241 | 4.05E-06 |
JAM3 | 0.407682 | −0.29761 | 2.65E-06 |
OCLN | 0.278019 | −0.42733 | 0.000103 |
SPN | 0.432433 | −0.31568 | 5.66E-07 |
NLGN1 | −0.12153 | −0.88699 | 1.08E-07 |
CNTN1 | −0.02019 | −0.80632 | 1.61E-07 |
PVR | 0.498486 | −0.3639 | 5.16E-09 |
NTNG2 | 0.507862 | −0.37073 | 2.46E-09 |
NRXN3 | 0.24736 | −0.64097 | 3.8E-08 |
CLDN10 | 0.10139 | −0.91694 | 2.37E-09 |
Table 4
Genes | Pearson correlation | P value |
---|---|---|
SELE | 0.235 | 0.006 |
NRCAM | 0.233 | 0.006 |
ITGA4 | 0.214 | 0.011 |
SDC1 | 0.208 | 0.014 |
ITGA8 | 0.195 | 0.019 |
VCAM1 | 0.194 | 0.020 |
NECTIN1 | 0.168 | 0.037 |
NLGN4X | 0.164 | 0.041 |
CLDN5 | 0.161 | 0.044 |
NTNG2 | 0.160 | 0.045 |
JAM3 | −0.157 | 0.048 |
ICAM2 | −0.161 | 0.044 |
PVR | −0.180 | 0.028 |
CD80 | −0.199 | 0.017 |
CLDN14 | −0.202 | 0.016 |
SELPLG | −0.204 | 0.015 |
CD276 | −0.207 | 0.014 |
PDCD1 | −0.230 | 0.007 |
L1CAM | −0.244 | 0.005 |
OS, overall survival; CAMs, cell adhesion molecules.
The CAMs was capable of predicting OS
According to the regression analysis, we identified genes that were independently correlated with OS and independently capable of distinguishing the participants into groups with good prognosis or poor prognosis (Table 5). To predict the AML OS, we determined the independent prognostic factors (CAMs) and constructed two OS prediction models. Both of the models had acceptable efficiency to predict the OS (AUC =0.78 and 0.77, respectively) (Figure 4). Statistic evaluation showed that the two models were significant in projecting OS of patients with AML (Table 6).
Table 5
Genes | Low expression | High expression | P value | HR | 95% CI of ratio |
---|---|---|---|---|---|
L1CAM | 18.1 | 18.5 | 0.4139 | ||
SDC1 | 15.8 | 25.8 | 0.0531 | ||
NTING1 | 24.1 | 16.4 | 0.6139 | ||
CLDN14 | 21.5 | 17.4 | 0.8518 | ||
NRCAM | 11.8 | 27.0 | 0.0133 | 1.599 | 1.103–2.318 |
VCAM1 | 11.8 | 22.3 | 0.0449 | 1.378 | 0.9464–2.005 |
ITGA4 | 13.6 | 24.6 | 0.2778 |
CI, confidence interval; HR, hazard ratio.
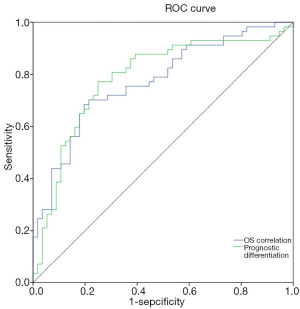
Table 6
Models | Variables | Coefficient | AUC | 95% CI of AUC | Sensitivity | Specificity | P value |
---|---|---|---|---|---|---|---|
OS correlation | CLDN14 | −0.39253 | 0.78 | 0.70–0.87 | 0.68 | 0.80 | 1.79E-07 |
ITGA4 | 0.673472 | ||||||
VCAM1 | 0.578583 | ||||||
Constant | −0.06208 | ||||||
Prognostic differentiation | L1CAM | −2.2895 | 0.77 | 0.69–0.86 | 0.77 | 0.75 | 6.4E-07 |
SDC1 | 3.821098 | ||||||
NTNG1 | −3.01709 | ||||||
CLDN14 | −2.63531 | ||||||
NRCAM | 2.149581 | ||||||
Constant | 10.66156 |
CI, confidence interval; AUC, area under the curve; OS, overall survival; AML, acute myeloid leukemia.
Discussion
In this study, we described the landscape of CAMs that are expressed in AML. Cell adhesion is a process through which cells interact with and attach to neighboring cells or matrix using specialized surface CAMs. Adhesion plays an important role in both normal hematopoiesis and AML. Many of the AMs identified on normal hematopoietic precursors are also expressed by AML blasts. Differential expression of AMs between normal hematopoietic cells and leukemic blasts has been documented as variable, likely reflecting the heterogeneity of the disease. Prognosis is affected by the expression of AMs and efforts continue to be made to therapeutically target adhesion in the fight against leukemia.
Different expression profiles revealed discrepant outcomes of AML. Both the primary cluster_1 (participants, n=73) and subcluster_1 (n=46) showed significantly prolonged OS and PFS compared to the other groups. All the differences showed statistical significance (P<0.05) except the discrepancy in OS between cluster_1 and cluster_2 (26.3 vs. 17.0 months), which could be attributed to the limited subject size. Evaluation of the differently expressed CAMs indicated that the down-regulation of HLA-relevant genes was associated with a favorable prognosis in AML. Most of the prognosis-related HLAs belonged to major histocompatibility complex (MHC) class II antigen. Among those HLAs, HLA-DMB was shown as a representative molecule and reported to play a critical role in the releasing of class II-associated invariant chain peptide (CLIP) from newly synthesized MHC class II molecules (16). A previous study showed that HLA-DM expression on myeloid leukemic blasts correlated with a poor outcome, which is concordant with our finding (17). Another expression differential of MHC class II genes, HLA-DPA1, was also significantly down-regulated in the favorable prognosis groups (Figure 2C). However, this result contrasts with a previous study, which showed that the HLA-DPA1 expression was specifically depressed in patients with relapse after transplantation or chemotherapy (18). We speculated that this discordance was caused by dynamic changes in the expression of HLAs in AML.
In the regression analysis for the detection of OS-correlated CAMs, neuronal cell adhesion molecule (NRCAM) was uncovered as an independent predictor and the expression was positively correlated with prolonged OS in AML. There is an involvement of NRCAM in the protein binding of heterotypic cell-cell adhesion, and NRCAM was reported to be inhibited by miR-29a and transcriptionally coactivated by Ski protein in AML (19). Previous studies have also shown that NRCAM is a potentially prognostic biomarker in solid tumors, such as glioma (20) and gastric cancer (21). The vascular cell adhesion molecule-1 (VCAM1) is involved in leukocyte-endothelial cell adhesion and interacts with integrin alpha-4/beta-1 (ITGA4/ITGB1) on leukocytes (22). In the current study, VCAM1 was notably and positively correlated with OS; moreover, up-regulation of VCAM1 was seen to independently predict a favorable prognosis in AML. Consistently, previous articles have reported that VCAM1 was more highly expressed on normal cells compared with leukemic bone marrow stromal cells. These findings suggest that the expression of NRCAM and VCAM1 are efficient predictive markers in AML.
Finally, based on the regression analysis, we constructed two prediction models for indicating the prognosis of AML. Both of the models showed a good efficiency and had AUCs of 0.78 and 0.77, respectively. The results suggested that the RNA-seq data of CAMs have the potential to predict OS. However, since the study is limited to a retrospective data set and has not been verified in the prospective subjects, determining its prognostic roles of CAMs requires further exploration. The specific mechanisms of CAMs to promote or suppress the AML also need to be deeply investigated.
Conclusions
In conclusion, we used data obtained from a public database to retrospectively analyze the expression profiles of CAMs and the prognostic roles of sole genes of CAMs in 173 patients with AML. According to gene expression values, specific subgroups with favorable prognoses and independent OS prediction genes were identified. Prospective clinical studies are required for further validation of these results.
Acknowledgments
Funding: None.
Footnote
Reporting Checklist: The authors have completed the MADR reporting checklist. Available at http://dx.doi.org/10.21037/tcr-20-3315
Conflicts of Interest: All authors have completed the ICMJE uniform disclosure form (available at http://dx.doi.org/10.21037/tcr-20-3315). The authors have no conflicts of interest to declare.
Ethical Statement: The authors are accountable for all aspects of the work in ensuring that questions related to the accuracy or integrity of any part of the work are appropriately investigated and resolved. All public omics data sets used were generated by previous studies and ethical approval was granted prior to their use. The study was conducted in accordance with the Declaration of Helsinki (as revised in 2013).
Open Access Statement: This is an Open Access article distributed in accordance with the Creative Commons Attribution-NonCommercial-NoDerivs 4.0 International License (CC BY-NC-ND 4.0), which permits the non-commercial replication and distribution of the article with the strict proviso that no changes or edits are made and the original work is properly cited (including links to both the formal publication through the relevant DOI and the license). See: https://creativecommons.org/licenses/by-nc-nd/4.0/.
References
- Döhner H, Weisdorf DJ, Bloomfield CD. Acute Myeloid Leukemia. N Engl J Med 2015;373:1136-52. [Crossref] [PubMed]
- Short NJ, Zhou S, Fu C, et al. Association of Measurable Residual Disease With Survival Outcomes in Patients With Acute Myeloid Leukemia: A Systematic Review and Meta-analysis. JAMA Oncol 2020;6:1890-9. [Crossref] [PubMed]
- Papaemmanuil E, Gerstung M, Bullinger L, et al. Genomic Classification and Prognosis in Acute Myeloid Leukemia. N Engl J Med 2016;374:2209-21. [Crossref] [PubMed]
- Xu M, Zhao XL, Zhu Y, et al. ND4 mutations are more prevalent in patients with acute myeloid leukemia of M2 morphology. Transl Cancer Res 2018;7:1064-71. [Crossref]
- Willier S, Rothämel P, Hastreiter M, et al. CLEC12A and CD33 co-expression as preferential target on pediatric AML for combinatorial immunotherapy. Blood 2020; [Epub ahead of print]. [Crossref] [PubMed]
- Kupsa T, Horacek JM, Jebavy L. The role of adhesion molecules in acute myeloid leukemia and (hemato)oncology: a systematic review. Biomed Pap Med Fac Univ Palacky Olomouc Czech Repub 2015;159:1-11. [Crossref] [PubMed]
- Gruszka AM, Valli D, Restelli C, et al. Adhesion Deregulation in Acute Myeloid Leukaemia. Cells 2019;8:66. [Crossref] [PubMed]
- Zhang H, Nakauchi Y, Köhnke T, et al. Integrated analysis of patient samples identifies biomarkers for venetoclax efficacy and combination strategies in acute myeloid leukemia. Nat Cancer 2020;1:826-39. [Crossref] [PubMed]
- Horiguchi H, Tsujimoto H, Shinomiya N, et al. A Potential Role of Adhesion Molecules on Lung Metastasis Enhanced by Local Inflammation. Anticancer Res 2020;40:6171-8. [Crossref] [PubMed]
- Oellerich T, Oellerich MF, Engelke M, et al. β2 integrin-derived signals induce cell survival and proliferation of AML blasts by activating a Syk/STAT signaling axis. Blood 2013;121:3889-99, S1-66.
- Bae MH, Oh SH, Park CJ, et al. VLA-4 and CXCR4 expression levels show contrasting prognostic impact (favorable and unfavorable, respectively) in acute myeloid leukemia. Ann Hematol 2015;94:1631-8. [Crossref] [PubMed]
- Gao J, Aksoy BA, Dogrusoz U, et al. Integrative analysis of complex cancer genomics and clinical profiles using the cBioPortal. Sci Signal 2013;6:pl1. [Crossref] [PubMed]
- Zhang Z, Yu H, Jiang S, et al. Evidence for Association of Cell Adhesion Molecules Pathway and NLGN1 Polymorphisms with Schizophrenia in Chinese Han Population. PLoS One 2015;10:e0144719. [Crossref] [PubMed]
- de Hoon MJ, Imoto S, Nolan J, et al. Open source clustering software. Bioinformatics 2004;20:1453-4. [Crossref] [PubMed]
- Saldanha AJ. Java Treeview--extensible visualization of microarray data. Bioinformatics 2004;20:3246-8. [Crossref] [PubMed]
- Weber DA, Evavold BD, Jensen PE. Enhanced dissociation of HLA-DR-bound peptides in the presence of HLA-DM. Science 1996;274:618-20. [Crossref] [PubMed]
- Chamuleau ME, Souwer Y, Van Ham SM, et al. Class II-associated invariant chain peptide expression on myeloid leukemic blasts predicts poor clinical outcome. Cancer Res 2004;64:5546-50. [Crossref] [PubMed]
- Christopher MJ, Petti AA, Rettig MP, et al. Immune Escape of Relapsed AML Cells after Allogeneic Transplantation. N Engl J Med 2018;379:2330-41. [Crossref] [PubMed]
- Teichler S, Illmer T, Roemhild J, et al. MicroRNA29a regulates the expression of the nuclear oncogene Ski. Blood 2011;118:1899-902. [Crossref] [PubMed]
- Hu G, Wang R, Wei B, et al. Prognostic Markers Identification in Glioma by Gene Expression Profile Analysis. J Comput Biol 2020;27:81-90. [Crossref] [PubMed]
- Liu JB, Jian T, Yue C, et al. Chemo-resistant Gastric Cancer Associated Gene Expression Signature: Bioinformatics Analysis Based on Gene Expression Omnibus. Anticancer Res 2019;39:1689-98. [Crossref] [PubMed]
- Becker PS, Kopecky KJ, Wilks AN, et al. Very late antigen-4 function of myeloblasts correlates with improved overall survival for patients with acute myeloid leukemia. Blood 2009;113:866-74. [Crossref] [PubMed]
(English Language Editor: J. Jones)