Novel long noncoding RNA (lncRNA) panel as biomarkers for prognosis in lung squamous cell carcinoma via competitive endogenous RNA (ceRNA) network analysis
Introduction
Lung cancer is always associated with aberrantly expressed transcriptomes. Previous work on the human transcriptome found that only 2% of the whole genome encodes protein, while as much as over 75% of the whole genome is transcribed (1). Existing studies discovered the functions of some non-coding transcripts (ncRNAs), such as transfer RNAs (tRNAs), small nucleolar RNAs (snoRNAs), circular RNA (circRNA), and microRNAs (miRNAs) (2). Based on the coding sequence length, ncRNAs could be divided into short ncRNAs and long ncRNAs (lncRNAs). miRNAs are short single-stranded RNAs which are 20–24 nucleotides long. They could be grouped into different families based on their functions. Through interacting with the miRNA response element (MRE), miRNAs could influence the expression of the target gene (3).
Generally, lncRNAs are over 200 nucleotides in length, which are considered as a large family of ncRNAs. Based on their functions, lncRNAs could be generally divided into two subgroups including functional lncRNAs and non-functional lncRNAs. Although functional lncRNAs are considered to have involved in many biological processes, the details of cellular mechanisms are not fully understood. The functions of lncRNAs in tumor progression have been a hot topic in recent years (4). CeRNA theories are based on one core finding: lncRNAs and mRNAs with similar sequences that have MRE elements could competitively bind with miRNAs to form a complex and generated a regulatory network, which is commonly called miRNA-based ceRNA networks (ceRNETs) (5). Recently, an increasing amount of evidence indicated that a large number of lncRNAs had been proved to be involved in ceRNET and proved to be closely related to the diagnosis and prognosis of cancer. For instance, by systematically analyzing the ceRNETs in different cancer progression, many researchers reported that the aberrant expression of lncRNAs had become the hallmarks of cancer (6,7). Since over two thirds of the entire human genome was transcriptional, the studies on prediction of potential ceRNETs could contribute significantly to the human transcriptome study (8,9).
Non-small cell lung cancer (NSCLC) is a heterogeneous disease that is typically classified into two broad subtypes, adenocarcinoma (AD) and squamous cell carcinoma (SCC), by using standard pathology methods (10). The mortality rate associated with lung cancer remains high in the recent years, and despite the presence of several potentially targetable mutations in lung AD, many lung tumors do not carry a known driver mutation that can be targeted with existing therapies, especially for SCC patients (11), till now there are few methods in accurate prediction of prognosis for SCC patients. Thus, detecting lung SCC at early stage could improve cure outcome and prolong overall survival (OS). Lung SCC always comes with aberrant gene expression that leads to some histopathological features. This complicates the clinical prediction and treatment of patients. The ceRNET improves our insight into the progression of lung SCC, hence provides novel genetic features which could be used as biomarker panel for potential prognostic value (11,12).
Early study on ceRNET could be traced back to the research of breast cancer (13). The finding suggested that HOTAIR could be used as a good indicator for predicting the metastasis status of primary breast cancer and the OS (13). Later on, many other studies extended the work of HOTAIR by employing multiple lncRNAs to evaluate the prognosis of patient, which notably improved the test’s sensitivity and specificity. In a recent study, Shin et al. constructed an mRNA mediated lncRNA signature which could be a candidate of biomarker to predict cancer recurrence among triple-negative breast cancer patients (14). Another recent study showed that a novel lncRNA named LINC00336 may have prognosis value in lung cancer (15). Given using multiple lncRNAs as biomarker could improve the prediction accuracy, Zhou et al. developed a computational approach to establish a lung-cancer-specific ceRNET. By using lung SCC high-throughput sequencing data, Zhou et al. designed a hub RNAs system that consists of multiple RNAs (16). According to their work, biomarker-panel based on multiple differentially expressed RNAs (DERNAs) can effectively distinguishes patients’ metastatic status in multiple independent datasets.
To summarize, it has been widely demonstrated that lncRNAs have great potential in the prediction of lung SCC progression. Nevertheless, many unknown details are yet to be explored in the area of new biomarkers in lung SCC prognosis. In this study, RNA expression profiles of SCC were obtained from The Cancer Genome Atlas (TCGA) database, then we constructed a ceRNET by analyzing three DERNAs in lung SCC—lncRNA, miRNA and mRNA. Through data mining and analysis, we further detected several SCC associated lncRNAs and its possible roles in the prediction of prognosis of SCC patients, furthermore, we investigated its potential application in clinical and scientific significance. We present the following article in accordance with the MDAR reporting checklist (available at http://dx.doi.org/10.21037/tcr-20-2410).
Methods
Declaration of Helsinki
The study was conducted in accordance with the Declaration of Helsinki (as revised in 2013).
RNA-seq data procession and R packages
Lung SCC RNA seq data from TCGA database (https://portal.gdc.cancer.gov/) were retrieved with the software R, including 504 patients’ clinical data, mRNA sequencing data of 450 tumor and 51 normal samples, miRNA sequencing data of 501 tumor and 49 normal samples, lncRNA sequencing data of 342 tumor and 45 normal samples. mRNA and miRNA data were downloaded with RTCGAToolBox. LncRNA data was downloaded in GDC Data Portal. mRNA and lncRNA expression unit was FPKM-UQ, miRNA expression unit was raw count. R packages used in this study included the following: RTCGAToolbox, ggbiplot, plyr, ggplot2, scales, ggbiplot, grid, limma, RColorBrewer, gplots, edgeR, hash, survival, survminer, GenomicDataCommons, magrittr, R.utils, rtracklayer, corrplot, Hmisc and pROC.
All databases were free and publicly available. The sample data included RNAseq data (mRNA and lncRNA) and the miRNA data. We declare that this study was fully abided by the published guidelines provided by TCGA.
Analysis of differentially expressed transcripts
We divided the seq data of samples into two groups, and then filtered out the unchanged mRNA data, lncRNA data and miRNA data. We used the limma and Edge R packages in R to process the raw data and generated expression data as Anders et al. described (17). Corrected P values and false discovery rate (FDR) were used to analyze the statistical profiles. The log2 fold change of expression data was set ≥1.5 and the FDR corrected P value was set <0.01 as the statistical significance threshold. The lncRNA transcripts were annotated by R-based GENCODE package, and after that all the unannotated transcripts were discarded.
ceRNET model generation and gene enrichment profile
In detail, first used miRWalk to screen miRNAs that match the target gene in the database (these database-supported miRNAs are likely to play a regulatory role in the network), and then screened lncRNAs with more than 0.3 correlation with these miRNAs. In detail, all correlation analyses were based on the Pearson’s correlation analysis, and the correlation coefficient must meet the correlation test P<0.05. LncRNAs with a certain correlation with miRNAs are more likely to play a regulatory role in the network for unsupervised clustering and subsequent modeling and model training. The miRNA + mRNA bindings were analyzed with miRWalk software (18) (http://mirwalk.umm.uni-heidelberg.de/). RNA bindings which were experimentally validated were firstly selected to build our prediction model. In order to improve the model’s reliability, we then filtered the miRNA-mRNA interactions to discard the ones that cannot significantly differentiate tumor from normal tissues. The software Cytoscape 3.6.1 was used to visualize our prediction model. Moreover, the Pearson’s correlation was utilized to assess the expression profiles between transcripts. Gene Ontology (GO) and Kyoto Encyclopedia of Genes and Genomes (KEGG) were analyzed by R-based Cluster Profiler package to analyze target differentially expressed genes (DEGs) with P<0.05 and enrichment score higher than 1.5 (19). All the results were finally clustered by the Enrichment Map plugin in Cytoscape 3.6.1 software.
In addition, a schematic view of the whole process of building the ceRNET and the final prediction model is shown in Figure S1.
Survival analysis
R package survival and survminer and Kaplan-Meier method were used for survival analysis according to previously described (20). We constructed a multivariable Cox regression model, plugging in all the prognostic lncRNA biomarkers and risk score information. The model takes the following form:
Where Exp refers to the expression level of prognostic RNAs; N refers to the number of prognostic RNAs; and Wi denotes the estimated regression coefficient of RNA in multivariate Cox regression model. We divided patients into high- and low-risk group according to the median value of their risk score. Kaplan-Meier survival analysis was used to evaluate the significance of OS between high- and low-risk groups.
We then conducted the two-sided log-rank test within the R-package “survival” to analyze the statistical significance of our model estimates. In addition, multivariate Cox regression analysis and data stratification analysis were performed to evaluate whether other clinical features, such as age and tumor stages will affect the model prediction. We also used the “Daim” package within the software R to perform receiver operating characteristic (ROC) curve analysis to assess the model’s sensitivity and specificity (21). Furthermore, we calculated the value of the area under the curve (AUC) via the ROC curve for validation of data.
Statistical analysis
For the mRNA and lncRNA analysis, the limma package of the R software was selected for differential expressed RNA analysis, while the miRNA analysis utilizes the edgeR package instead. Student’s t-test method was then selected for statistical significance analysis. When constructing the ceRNET, the correlations between miRNA, lncRNA and mRNA were tested, and only the characteristic pair of P<0.05 were considered as having a potential correlation. After the hub lncRNAs (hublncRNAs) were selected, all samples that had been sequenced for mRNA were used to construct a Cox regression model, including univariate and multivariate analysis for evaluating risk factors. All survival analysis used the Kaplan-Meier algorithm method and P<0.05 was considered to be statistically significant. The construction of the Cox regression model and the survival analysis were performed using the survival and survminer packages in R.
Results
Transcriptome and lncRNA profiles
All the TCGA samples included in our analysis were clinically diagnosed as lung SCC. Detailed clinical information was displayed in the online table (available at https://cdn.amegroups.cn/static/public/10.21037tcr-20-2410-1.docx). The transcriptome data of 504 lung SCC tissues and 51 normal tissues were further grouped into two categories. Log2 fold change of transcripts >1.5 with corrected P value of transcripts <0.01 were used as filtering criteria. Finally, 192 and 1,966 up- or down-regulated genes were found. The heatmap with the top 1,000 DEGs was generated by using R-based Heatmap package (Figure 1A). Median was used to cut the expression profiles. The principal component analysis (PCA) outcome was shown in Figure 1B. All the DERNAs and the expression fold changes were presented in the online table (available at https://cdn.amegroups.cn/static/public/10.21037tcr-20-2410-2.docx).
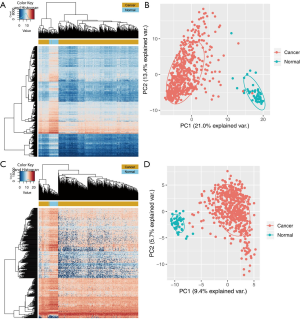
For lncRNA profiles, we set corrected P value <0.01 and log2 fold change >1.5 as the cut-off points. As shown in Figure 1C [DEGs were shown in the online table (available at https://cdn.amegroups.cn/static/public/10.21037tcr-20-2410-3.docx)], there were 25 aberrantly upregulated lncRNAs and 335 aberrantly downregulated lncRNAs in lung SCC tissues. The PCA analyzed data results were presented in Figure 1D. As suggested by the results, those 360 differentially expressed lncRNAs (DElncRNAs) were annotated in the tumorigenesis of lung SCC. The TCGA samples were further grouped into several categories according to their pathological tumor-node-metastasis (TNM) stages as well as with or without pathological stage. Then, we analyzed whether these DElncRNAs were connected with tumor progression. Our findings indicated that 82 DElncRNAs were highly correlated with the progression of lung SCC (https://cdn.amegroups.cn/static/public/10.21037tcr-20-2410-4.docx).
MiRNA profiles
For the purpose of generating a lncRNA/miRNA/mRNA ceRNET, we further investigated the miRNA expression profiles among 342 tumor tissues and 51 normal tissues. We managed to identify 368 differently expressed miRNAs (DEmiRNAs), including 56 upregulated miRNAs and 312 downregulated miRNAs (https://cdn.amegroups.cn/static/public/10.21037tcr-20-2410-5.docx). The heatmap with clustering upregulated and downregulated genes was analyzed in R platform (Figure 2A). The PCA analysis data was shown in Figure 2B. We also performed the survival analysis with the help of Kaplan-Meier curve to explore the correlation between DEmiRNAs and OS of patients. We discovered statistically significant correlation among three miRNAs, namely hsa-miR-2278, hsa-miR-3176 and hsa-miR-3654, and the patients’ OS (P<0.05). To be more specific, all miRNAs were positively correlated with patients’ OS (Figure 2C,D,E).
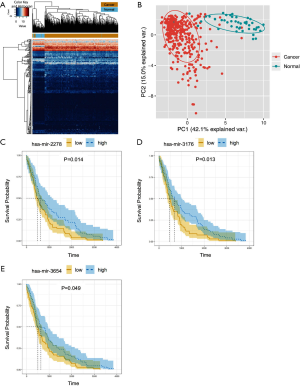
Construction of ceRNET in lung SCC
Another key objective of this work is to identify those lncRNAs that are of great importance in lung SCC under the guidance of ceRNA theory. To achieve this target, we constructed a lncRNA/miRNA/mRNA ceRNETs model, which helped us identify three DEmiRNAs that involved in a ceRNET. In order to double check whether our finding is reliable, we further did unsupervised test to optimize the network, finally we found three miRNAs that are the most important cores of the ceRNET (Figure 3A), named hsa-miR-2278, hsa-miR-3176 and hsa-miR-3654. For instance, AL360219 and AC092071 could interact with miR-3654-mediated FGFBP3, TP73, UBE2J1 and ZNF225; AL590226, AL090044 and LINC01352 could interact with miR-2278-mediated DKK2, EDA, TMEM218 and MYD88; AC010976 could interact with miR-3176-mediated IL19, ALOX15B, ATPAF1 and MMP19. In the following step, we put the expression profiles of DElncRNAs and DERNAs involved in the network into a linear regression model. Our regression analysis showed a strong positive correlation among the expression levels of ceRNAs (Figure 3B). The above results suggest that DElncRNAs could indirectly interact with functional mRNAs in lung SCC and the ceRNA based model we built was successful.
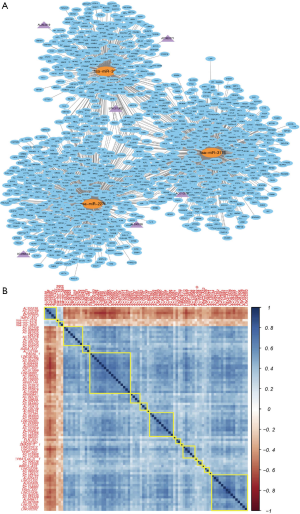
Screening and verification of pivotal hub nodes in ceRNET
In an effort to single out those potentially pivotal DElncRNAs that have strong predictive power of tumorigenesis, we performed topological analysis to identify the key hublncRNAs in the generation of progression-related ceRNET model. In order to reduce falsely reported positives, we only included top 5% correlated lncRNA-miRNA pairs with correlation coefficient greater than 95% and Pearson’s correlation coefficient higher than 0.3 into our analytical framework (Figure 4A,B,C). We managed to identify six lncRNAs that could be potential hublncRNAs candidates, and they are AL360219, AC092071, AL590226, AL090044, LINC01352 and AC010976. Moreover, we found that three of the above six lncRNAs, namely AL590226, AL090044 and LINC01352 could interact with miR-2278. We also discovered that AL360219 and AC092071 could interact with miR-3654. Another finding is that one lncRNA named AC010976 could interact with miR-3176 (https://cdn.amegroups.cn/static/public/10.21037tcr-20-2410-5.docx).
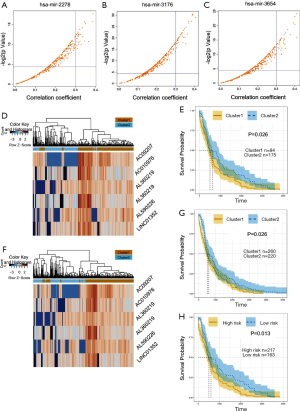
Taking our above findings as given, we took a further step to test prognostic significance of those six hublncRNAs in a group of lung SCC for training of the biomarker panel. To start with, we adopted an unsupervised hierarchical clustering strategy to identify the expression patterns of these six hublncRNAs in 359 lung SCC patients. Guided by first bifurcation of the clustering dendrogram, we divided the 359 patients into two subgroups named Cluster1 and Cluster2. Number of patients in Cluster 1 and Cluster 2 are 84 and 175 respectively (as is shown in Figure 4D). Then we conducted the survival analysis, and found that the OS of the two patient subgroups are significantly different from each other (median OS, 618 vs. 503.5 days) (log-rank test P=0.026; Figure 4E).
Next, we tested the prognostic significance of these six hublncRNAs in a larger group of lung SCC patients. Similar to the procedure in the previous step, we used an unsupervised hierarchical clustering strategy to identify the expression patterns of these six hublncRNAs in 480 lung SCC patients. Again, with the guidance of first bifurcation of the clustering dendrogram, the 480 patients were divided into two subgroups, Cluster1 and Cluster 2, containing 260 and 220 patients respectively (Figure 4F). The same survival analysis was performed, and this time we also found a statistically significant difference in OS between these two patient subgroups (median OS, 604 vs. 516 days) (log-rank test P=0.026 (Figure 4G), the above results confirmed that those six hublncRNAs we identified at the beginning of this section have statistical predictive power to the progression of lung SCC.
Using the survival data (OS) as the dependent variable, we managed to estimate the coefficients W associated with each RNAs expression in the cox model we discussed before. After incorporating the coefficient estimates, we now can construct a six hublncRNAs-based model to compute the patients’ risk score. The model takes the following form.
Risk score = (0.0531823169723279 × expression value of AC092071) + (0.0144310221664348 × expression value of AC010976) + (0.0032589130067618 × expression value of LINC01352) + (0.0135413904152731 × expression value of AL360219) + (0.00550930675039574 × expression value of AL590226) + (0.0267536304160777 × expression value of AC090044) (Intercept =−0.50777324831789).
Finally, we tested the risk model with all available patients in the lung SCC samples (n=480) to see whether our identification of RNAs could be useful in predicting lung SCC patients’ survival. We first calculated each patient’s risk score based on the above model. Then we divided them into high-risk group (n=217) and low-risk group (n=163), using the median risk score as the cut-off point. We found that OS of the high-risk group was significantly shorter than then low-risk group (506 vs. 604 days median, log-rank P=0.012, Figure 4H). Hazard ratios (HRs) were calculated with univariate and multivariate analysis. For example, multivariate cox analysis [P=0.0022; 95% confidence interval (CI): 0.36–0.8, HR and other data was shown in Table 1].
Table 1
Variables | Univariate analysis | Multivariate analysis | |||||
---|---|---|---|---|---|---|---|
HR | 95% CI for HR | P value | HR | 95% CI for HR | P value | ||
Age | 1.00 | 0.94–1.10 | 0.99 | 1.00 | 0.96–1.10 | 0.5992 | |
T | |||||||
T1 | 0.63 | 0.40–1.00 | 0.048 | 0.37 | 0.16–0.84 | 0.0181 | |
T2 | 1.50 | 0.91–2.40 | 0.89 | 0.56 | 0.26–1.20 | 0.1236 | |
T3 | 1.70 | 0.83–3.60 | 0.11 | 0.80 | 0.35–1.80 | 0.5948 | |
T4 | 1.20 | 0.79–1.90 | 0.14 | – | – | – | |
Risk_score | 0.57 | 0.39–0.85 | 0.0053 | 0.54 | 0.36–0.80 | 0.0022 |
TCGA, The Cancer Genome Atlas; CI, confidence interval; HR, hazard ratio; lncRNA, long noncoding RNA.
Clinical prognostic significance of lncRNA signature
The patient status, risk profiles, and hublncRNA profiles of 480 samples were summarized in Figure 5A,B,C,D. Six hublncRNAs including AL360219, AC092071, AL590226, AL090044, LINC01352 and AC010976 were finally validated as risky lncRNAs. Patients with high-risk scores tend to feature risky hublncRNAs expression, and those with a low-risk score tend to possess protective hublncRNAs expressions (Figure 5A), survival time of the patients was shown in Figure 5B, expression profiles of the six hublncRNAs were shown in Figure 5C. ROC curve analysis is conducted to test the sensitivity and specificity of our model (Figure 5D). According to our analysis, the AUC value of the six-hublncRNA panel reached 0.578, confirming its strong predictive power.
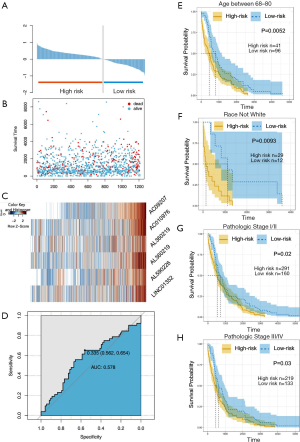
To further test whether our model’s performance will be affected by the inclusion of other clinical factors, we conducted stratification analysis based on patients’ age, race and their clinical stage.
We found that the lncRNAs signature could serve as a potential prognostic factor in group age between 68–80 as well as patient’s race which was not white. If we combined all the lncRNAs together as a biomarker panel, the signature can accurately distinguish high-risk group patients from low-risk group patients (Figure 5E,F). Considering the multivariate analysis in Table 1, the results confirmed that our model’s predictive prognostic power is affected by patients’ age and race.
Similarly, in the clinical stage-based stratification analysis, we first divided the patients into two groups featuring stages I–II cancer and stages III–IV cancer. Again, our model’s prediction worked in both groups. Within the stages I–II group, our model predicted high- risk group had a shorter survival time than the low-risk group (log-rank test P=0.02, Figure 5G). The differences of OS between the high-risk group and the low-risk group were also significant in patients of stages III–IV (log-rank test P=0.03 Figure 5H). Therefore, the lncRNAs signature we designed could be a useful prognostic indicator of lung SCC.
Discussion
In cancer research, the ceRNA model is a cutting-edge regulation mechanism that was developed on the basis of competitive binding of miRNA. There has been increasing amount of evidence suggesting that those miRNAs and their competitive endogenous targets can form a complicated ceRNAs network (22). As discovered by many recent studies, miRNAs and lncRNAs are closely related in the ceRNAs model (23).
Owing to the great improvement of cancer databases, such as TCGA (NIH, USA), this study managed to develop a lung-cancer specific ceRNA model that incorporating multiple RNA related patient profiles. Our ceRNA model provided additional insight for future studies on the tumorigenesis of lung SCC.
By reviewing the latest studies, lncRNAs are the currently the preferred diagnostic and prognostic biomarkers over mRNAs or miRNAs. This is mainly due to its unique linkages with tumorigenesis. Some well-researched lncRNAs, such as HOTAIR in breast cancer, MALAT1 in lung cancer (13,24), have already been applied in molecular therapy as a usage of translational medicine.
As 95% of human genes are noncoding, there are countless lncRNAs waiting to be discovered. Our study identified read-specific lncRNAs on the intersecting expression between lung cancer tissues and adjacent normal tissues from different stages, including 82 DElncRNAs, and we found that six hublncRNAs (i.e., AL360219, AC092071, AL590226, AL090044, LINC01352 and AC010976) were strongly correlated with the progression of lung SCC. The six hublncRNAs are also proved to have significant predictive power of lung SCC patients’ OS. Among the six hublncRNAs discussed in our study, previous work only suggested the LINC01352 was proved to be a potential biomarker in endometrial cancer (25). The other five lncRNAs are not covered by any existing literature regarding their connection with any types of cancer yet. Hence, we are the first to report these six lncRNAs as predictors in lung SCC. Apart from that, the model we developed could be useful in future targeted therapy of lung SCC.
In this study, we found two lncRNAs AL360219 and AC092071 could interact with miR-3654. MiR-3654 was a miRNA which was recently reported to be overexpressed in HPV-induced warts and functioned as a potentially oncogene in cancers such as cervical cancer and ovarian cancer (26). Three lncRNAs, AL590226, AL090044 and LINC01352 could interact with miR-2278. In this study, we found that miR-2278 showed a potential tumor promoting feature (Figure 2C), while in a recent study, miR-2278 was reported to be a potential tumor suppressor (27). One lncRNA, AC010976 could interact with miR-3176. As far as we could trace, there are no studies regarding miR-3176 in cancer or any other areas. Most lncRNAs that interact with other RNAs may play pivotal roles in cellular processes, thus further study still needs to be done for a deeper understanding of their correlation with clinical features and cellular mechanisms.
Unlike for lung ADs, for squamous cell lung cancers, no molecular targeted therapies have yet been found or developed, because targetable dysregulated transcriptomes are scarce in this tumor type (11). Hence, taking lncRNAs and ceRNET as a new start is supposed to be necessary and promising for the benefit of patients. Furthermore, as shown in Figure S1 and Figure 4D,E,F,G,H, we used unsupervised test to optimize the ceRNET, we expect to generate a more trustable biomarker panel, which could combine multiple lncRNAs into a powerful biomarker panel and classify the samples directly, which made the results more reliable comparing with similar studies in ceRNET (28-30).
Our study proved that the six lncRNAs together could be a powerful panel in the prediction of the prognosis of lung SCC. Nevertheless, we still are looking forward to carrying out more experiments in order to unveil more about the members in our biomarker panel, and to form a deep understanding of lung SCC.
Conclusions
Our study indicates that a panel consists of those six lncRNAs together could be an effective prognosis biomarker of lung SCC, which could potentially benefit the patients in the future.
Acknowledgments
All R packages used in this study can be downloaded from the R official website (http://r-pkgs.had.co.nz/).
Funding: This work was supported by
Footnote
Reporting Checklist: The authors have completed the MDAR checklist. Available at http://dx.doi.org/10.21037/tcr-20-2410
Conflicts of Interest: All authors have completed the ICMJE uniform disclosure form (available at http://dx.doi.org/10.21037/tcr-20-2410). The authors have no conflicts of interest to declare.
Ethical Statement: The authors are accountable for all aspects of the work in ensuring that questions related to the accuracy or integrity of any part of the work are appropriately investigated and resolved. The study was conducted in accordance with the Declaration of Helsinki (as revised in 2013). All original data and analysis results are available under reasonable request to the corresponding author.
Open Access Statement: This is an Open Access article distributed in accordance with the Creative Commons Attribution-NonCommercial-NoDerivs 4.0 International License (CC BY-NC-ND 4.0), which permits the non-commercial replication and distribution of the article with the strict proviso that no changes or edits are made and the original work is properly cited (including links to both the formal publication through the relevant DOI and the license). See: https://creativecommons.org/licenses/by-nc-nd/4.0/.
References
- Djebali S, Davis CA, Merkel A, et al. Landscape of transcription in human cells. Nature 2012;489:101-8. [Crossref] [PubMed]
- ENCODE Project Consortium. An integrated encyclopedia of DNA elements in the human genome. Nature 2012;489:57-74. [Crossref] [PubMed]
- Bartel DP. MicroRNAs: genomics, biogenesis, mechanism, and function. Cell 2004;116:281-97. [Crossref] [PubMed]
- Bhan A, Soleimani M, Mandal SS. Long Noncoding RNA and Cancer: A New Paradigm. Cancer Res 2017;77:3965-81. [Crossref] [PubMed]
- Abdollahzadeh R, Daraei A, Mansoori Y, et al. Competing endogenous RNA (ceRNA) cross talk and language in ceRNA regulatory networks: A new look at hallmarks of breast cancer. J Cell Physiol 2019;234:10080-100. [Crossref] [PubMed]
- Do D, Bozdag S. Cancerin: A computational pipeline to infer cancer-associated ceRNA interaction networks. PLoS Comput Biol 2018;14:e1006318. [Crossref] [PubMed]
- Zheng L, Zhang Y, Fu Y, et al. Long non-coding RNA MALAT1 regulates BLCAP mRNA expression through binding to miR-339-5p and promotes poor prognosis in breast cancer. Biosci Rep 2019;39:BSR20181284. [Crossref] [PubMed]
- Karreth FA, Pandolfi PP. ceRNA cross-talk in cancer: when ce-bling rivalries go awry. Cancer Discov 2013;3:1113-21. [Crossref] [PubMed]
- Calloni R, Bonatto D. Characteristics of the competition among RNAs for the binding of shared miRNAs. Eur J Cell Biol 2019;98:94-102. [Crossref] [PubMed]
- Faruki H, Mayhew GM, Serody JS, et al. Lung Adenocarcinoma and Squamous Cell Carcinoma Gene Expression Subtypes Demonstrate Significant Differences in Tumor Immune Landscape. J Thorac Oncol 2017;12:943-53. [Crossref] [PubMed]
- Hashemi-Sadraei N, Hanna N. Targeting FGFR in Squamous Cell Carcinoma of the Lung. Target Oncol 2017;12:741-55. [Crossref] [PubMed]
- Shima H, Kida K, Adachi S, et al. Lnc RNA H19 is associated with poor prognosis in breast cancer patients and promotes cancer stemness. Breast Cancer Res Treat 2018;170:507-16. [Crossref] [PubMed]
- Sorensen KP, Thomassen M, Tan Q, et al. Long non-coding RNA HOTAIR is an independent prognostic marker of metastasis in estrogen receptor-positive primary breast cancer. Breast Cancer Res Treat 2013;142:529-36. [Crossref] [PubMed]
- Shin VY, Chen J, Cheuk IW, et al. Long non-coding RNA NEAT1 confers oncogenic role in triple-negative breast cancer through modulating chemoresistance and cancer stemness. Cell Death Dis 2019;10:270. [Crossref] [PubMed]
- Wang M, Mao C, Ouyang L, et al. Long noncoding RNA LINC00336 inhibits ferroptosis in lung cancer by functioning as a competing endogenous RNA. Cell Death Differ 2019;26:2329-43. [Crossref] [PubMed]
- Zhou X, Liu J, Wang W. Construction and investigation of breast-cancer-specific ceRNA network based on the mRNA and miRNA expression data. IET Syst Biol 2014;8:96-103. [Crossref] [PubMed]
- Anders S, Huber W. Differential expression analysis for sequence count data. Genome Biol 2010;11:R106. [Crossref] [PubMed]
- Sticht C, De La Torre C, Parveen A, et al. miRWalk: An online resource for prediction of microRNA binding sites. PLoS One 2018;13:e0206239. [Crossref] [PubMed]
- Merico D, Isserlin R, Stueker O, et al. Enrichment map: a network-based method for gene-set enrichment visualization and interpretation. PLoS One 2010;5:e13984. [Crossref] [PubMed]
- Li M, Spakowicz D, Burkart J, et al. Change in neutrophil to lymphocyte ratio during immunotherapy treatment is a non-linear predictor of patient outcomes in advanced cancers. J Cancer Res Clin Oncol 2019;145:2541-6. [Crossref] [PubMed]
- Fontoura CA, Castellani G, Mombach JC. The R implementation of the CRAN package PATHChange, a tool to study genetic pathway alterations in transcriptomic data. Comput Biol Med 2016;78:76-80. [Crossref] [PubMed]
- Salmena L, Poliseno L, Tay Y, et al. A ceRNA hypothesis: the Rosetta Stone of a hidden RNA language? Cell 2011;146:353-8. [Crossref] [PubMed]
- Qi X, Zhang DH, Wu N, et al. ceRNA in cancer: possible functions and clinical implications. J Med Genet 2015;52:710-8. [Crossref] [PubMed]
- Jen J, Tang YA, Lu YH, et al. Oct4 transcriptionally regulates the expression of long non-coding RNAs NEAT1 and MALAT1 to promote lung cancer progression. Mol Cancer 2017;16:104. [Crossref] [PubMed]
- Tang H, Wu Z, Zhang Y, et al. Identification and Function Analysis of a Five-Long Noncoding RNA Prognostic Signature for Endometrial Cancer Patients. DNA Cell Biol 2019;38:1480-98. [Crossref] [PubMed]
- Al-Eitan LN, Alghamdi MA, Tarkhan AH, et al. Gene Expression Profiling of MicroRNAs in HPV-Induced Warts and Normal Skin. Biomolecules 2019;9:757. [Crossref] [PubMed]
- Kaymaz BT, Gunel NS, Ceyhan M, et al. Revealing genome-wide mRNA and microRNA expression patterns in leukemic cells highlighted "hsa-miR-2278" as a tumor suppressor for regain of chemotherapeutic imatinib response due to targeting STAT5A. Tumour Biol 2015;36:7915-27. [Crossref] [PubMed]
- Gao C, Li H, Zhuang J, et al. The construction and analysis of ceRNA networks in invasive breast cancer: a study based on The Cancer Genome Atlas. Cancer Manag Res 2019;11:1-11. [Crossref] [PubMed]
- Long J, Bai Y, Yang X, et al. Construction and comprehensive analysis of a ceRNA network to reveal potential prognostic biomarkers for hepatocellular carcinoma. Cancer Cell Int 2019;19:90. [Crossref] [PubMed]
- Zhang Z, Wang S, Ji D, et al. Construction of a ceRNA network reveals potential lncRNA biomarkers in rectal adenocarcinoma. Oncol Rep 2018;39:2101-13. [Crossref] [PubMed]