Development and validation of a prognostic nomogram for predicting the overall survival of myxofibrosarcoma patients: a large population-based study
Introduction
Myxofibrosarcoma (MFS) represents 5% of soft tissue sarcomas (1-3), and soft tissue sarcomas account for less than 1% of all malignancies (4-5). MFS was first described in 1977, and it was originally considered myxoid degeneration of malignant fibrous tissue cell tumors (6-9). It is usually found in elderly individuals, with a median age at diagnosis of 64–75 years, and mainly occurs in the extremities, especially the lower extremities (1-4). MFS has a higher local recurrence (LR) rate and relatively better outcomes than other soft tissue sarcomas; the 5-year overall survival (OS) rate is 60–80%, and the LR rate exceeds 25% (1,4,6,10). However, the optimal therapeutic strategy for MFS remains unclear (11). Surgery followed by adjuvant radiotherapy is considered the standard therapeutic strategy in clinical practice (1,3,4,12). However, the sensitivity of sarcomas to radiotherapy and the effect of radiotherapy on local incision remains controversial (1,4,6,7,10). Moreover, anthracyclines and ifosfamide-based chemotherapy, as well as novel targeted therapies, are rarely administered, so their roles in the treatment of MFS are uncertain (1,2,4,13-15).
Clinicians should consider comprehensive factors when treating an individual patient, which is crucial to each patient’s outcome. However, prognostic predictions and clinical decisions depend mainly on the American Joint Committee on Cancer (AJCC) staging system, which has been considered an effective method for evaluating the risk of malignant tumors for 40 years (16). However, this system has some limitations, such as not taking into account the anatomical site, pathological type, or patient characteristics (8). Although some studies have explored prognostic factors of MFS, such as molecular markers, tumor size, tumor grade, surgical margin, and LR (6,11,17-21), the sample sizes were small, generally fewer than 100 patients (1-3). Therefore, the findings were not consistent among the different studies. For example, Sanfilippo et al. confirmed that tumor size, tumor grade, and resection margins were statistically significant predictors of survival in MFS patients (1). However, Mühlhofer et al. found that tumor grade and metastasis were significant prognostic factors of survival, whereas negative surgical margins were not a significant prognostic factor (6).
The nomogram, a prognostic tool that is tailored to an individual patient, is a novel effective tool for clinicians to estimate patient outcomes. Demographic data, clinical data, and histopathological data are integrated to construct a model to specifically predict more individualized and accurate outcomes. Relevant studies have validated the utility of nomograms in predicting prognosis in cancer patients (22-24). Nomograms can be used to solve some of the problems in the AJCC staging system or other prognostic methods. However, to our knowledge, there is still no effective prognostic nomogram model for MFS patients based on a large population and comprehensive factors. This study aimed to develop and validate a large population-based, clinically relevant nomogram to estimate the survival of MFS patients as a useful clinical tool. We present the following article in accordance with the TRIPOD reporting checklist (available at http://dx.doi.org/10.21037/tcr-20-2588).
Methods
Data source
The Surveillance, Epidemiology, and End Results (SEER) database is a population-based cancer reporting system of the National Cancer Institute that is publicly available for researchers. The SEER data, covering approximately 28% of the total population of the United States, have become increasingly utilized in the study of cancer epidemiology and outcomes over the past three decades (25-27). We downloaded SEER Datasets and Software (RRID:SCR_003293) version #8.3.6 (http://seer.cancer.gov/seerstat/) and acquired the data from Identity-SEER 18Regs Custom Data (with additional treatment fields), Nov 2017 Sub (1973-2015 varying). To construct and validate the novel nomogram, all patients were randomly allocated to the training or validation cohorts (seed =15). The study was conducted in accordance with the Declaration of Helsinki (as revised in 2013). Institutional review board approval was not required in the current study because the SEER research data are publicly available. Moreover, we registered and received access to the SEER database (accession No. 16375-Nov2018).
Data selection
This was a retrospective study. We included data from patients with MFS (ICD-O-3 code: 8811/3) according to the 2013 World Health Organization Classification of Soft Tissue Tumors (Fourth Edition) who were diagnosed between 2004 and 2015 from 18 registry centers in the SEER database. MFS diagnoses were confirmed by histology or cytology examinations, and data from 2,234 patients were obtained. Patients with missing crucial information, such as unknown survival status, unknown survival time, or unknown treatment, and age at diagnosis <18 were excluded. Finally, data from 1,270 patients were included after filtration (inclusion and exclusion criteria are shown in Figure 1). The variables of interest were identified, including age at diagnosis, sex, race, marital status, socioeconomic status (SES), tumor site, tumor size, tumor extension, AJCC stage, grade, total number of in situ or malignant tumors, surgical status, radiotherapy, and chemotherapy. The outcomes assessed in this study included the 3- and 5-year OS rates. OS was calculated from the date of first diagnosis of MFS to death or loss to follow-up. The data of patients who were lost to follow-up or who were still alive at the last follow-up were censored. The SES variable was divided into three levels using previously reported cutoff points: <10% (low), ≥10% and <20% (moderate), and ≥20% (high) (23). A tumor was considered to be in the soft tissues of the extremities if it was in the soft tissue of the upper limbs, shoulders, lower limbs, or hips and in the soft tissues of other sites if it was in soft tissues of the head, face, neck, thorax, abdomen, pelvis, trunk, etc. All other tumors at different sites, including the bones, peritoneum, retroperitoneum, heart, and nerves, were classified into one group. Tumor size was categorized as ≤50 or >50 mm (recorded as the largest dimension or diameter of the tumor). Tumors were labeled as confined, localized, or metastatic (extension to adjacent connective tissue or distant organ) according to the tumor extension. Tumor grade was categorized into grade I (well-differentiated), grade II (moderately differentiated), grade III (poorly differentiated), and grade IV (undifferentiated). Patient data were randomly divided into a training cohort and a validation cohort at a ratio of 7:3, with the training cohort having 889 patients and the validation cohort having 381 patients.
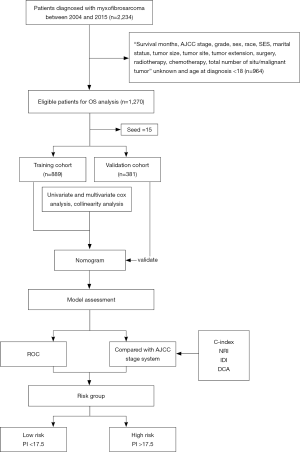
Statistical analysis
The univariate analysis was performed using the Kaplan-Meier method and univariate Cox regression analysis. Survival curves were compared using the log-rank test. Variables identified as significant on univariate analysis were entered into the multivariate Cox proportional hazards regression models to identify the independent prognostic factors of MFS. Cox regression model was used to handle missing data and build a nomogram with the variables identified in the multivariate analysis. The variance inflation factor (VIF) was used to assess multicollinearity among the covariates. Variables with VIF >5 were considered to have multicollinearity and were not included in the final model. A nomogram was constructed to predict the 3- and 5-year OS, and its efficacy was compared with that of the AJCC staging system, 6th edition. The discriminative ability and predictive accuracy of the nomogram were evaluated by receiver operating characteristic (ROC) curve analysis, the concordance index (C-index), integrated discrimination improvement (IDI), and net reclassification improvement (NRI). Calibration curves described the agreement between the predicted probabilities of the nomogram and the observed outcomes. The clinical net benefits of the nomogram were determined by decision curve analysis (DCA). Further, a prognostic index (PI) score was calculated using the nomogram for each patient in the training and validation cohorts, and the patients were divided into a high-risk group and low-risk group based on the PI score. Finally, survival analysis was used to estimate the ability of the nomogram to distinguish patients from different risk groups.
The nomogram was constructed using R software version 3.1.0 (https://www.r-project.org). Survival curves were drawn using GraphPad Prism 8 software (GraphPad Software, Inc., La Jolla, CA, USA). All statistical analyses were performed using SPSS, version 26 (IBM, Armonk, NY, USA), and a two-tailed P<0.05 was considered statistically significant.
Results
Demographic data
Data from 1,270 patients were included, including 889 in the training cohort and 381 in the validation cohort. In the entire cohort, the median age at diagnosis was 63 years, and the age range was 18–98 years. The median follow-up period was 41 months (range, 1–143 months), the 3- and 5-year OS rates were 80.7% and 74.3%, respectively, and the 3- and 5-year disease-specific survival rates were 90.2% and 87.3%, respectively.
In the entire cohort, the median tumor size was 59 mm (range, 2–996 mm). Men (54.3% in the training cohort and 53.8% in the validation cohort), whites (83.7% and 83.5%, respectively), married patients (60.0% and 57.5%, respectively), and patients with moderate SES (53.7% and 53.8%, respectively) were most common in both cohorts. In terms of tumor characteristics, tumors in the soft tissue of the extremities (71.3% in the training cohort and 74.8% in the validation cohort), tumors >50 mm (56.2% and 53.3%, respectively), confined tumors (52.1% and 53.0%, respectively), grade II tumors (36.1% and 40.4%, respectively), and AJCC stage I tumors (51.2% and 52.2%) were predominant in both cohorts. In terms of treatment, surgery (97.9% in the training cohort and 96.1% in the validation cohort) was the most common treatment, whereas few patients underwent chemotherapy (8.8% and 9.8%, respectively). In the entire cohort, approximately the same proportions of patients did (53.9%) and did not (46.1%) receive radiotherapy (Table 1).
Table 1
Variable | Total (n=1,270) | Training (n=889) | Validation (n=381) |
---|---|---|---|
Age at diagnosis, median [range] | 63 [18–98] | 63 [18–98] | 62 [18–97] |
Sex, n (%) | |||
Male | 688 (54.2) | 483 (54.3) | 205 (53.8) |
Female | 582 (45.8) | 406 (45.7) | 176 (46.2) |
Race, n (%) | |||
White | 1062 (83.6) | 744 (83.7) | 318 (83.5) |
Black | 98 (7.7) | 62 (6.9) | 36 (9.4) |
A/PI | 103 (8.1) | 78 (8.7) | 25 (6.6) |
AI/AN | 7 (0.6) | 5 (0.7) | 2 (0.5) |
Site, n (%) | |||
STE | 919 (72.4) | 634 (71.3) | 285 (74.8) |
STOS | 319 (25.1) | 237 (26.7) | 82 (21.5) |
Other | 32 (2.5) | 18 (2.0) | 14 (3.7) |
Grade, n (%) | |||
I | 199 (15.7) | 145 (16.3) | 54 (14.2) |
II | 475 (37.4) | 321 (36.1) | 154 (40.4) |
III | 213 (16.8) | 142 (16.0) | 71 (18.6) |
IV | 383 (30.1) | 281 (31.6) | 102 (26.8) |
AJCC stage, n (%) | |||
I | 654 (51.5) | 455 (51.2) | 199 (52.2) |
II | 319 (25.1) | 221 (24.9) | 98 (25.7) |
III | 246 (19.4) | 180 (20.2) | 66 (17.4) |
IV | 51 (4.0) | 33 (3.7) | 18 (4.7) |
Surgery, n (%) | |||
No | 34 (2.7) | 19 (2.1) | 15 (3.9) |
Yes | 1,236 (97.3) | 870 (97.9) | 366 (96.1) |
Radiotherapy, n (%) | |||
No/unknown | 585 (46.1) | 401 (45.1) | 184 (48.3) |
Yes | 685 (53.9) | 488 (54.9) | 197 (51.7) |
Chemotherapy, n (%) | |||
No/unknown | 1,157 (91.1) | 811 (91.2) | 346 (90.8) |
Yes | 113 (8.9) | 78 (8.8) | 35 (9.2) |
Tumor size, n (%) | |||
≤50 mm | 567 (44.6) | 389 (43.8) | 178 (46.7) |
>50 mm | 703 (55.4) | 500 (56.2) | 203 (53.3) |
Extension, n (%) | |||
Confined | 665 (52.4) | 463 (52.1) | 202 (53.0) |
Localized | 336 (26.4) | 236 (26.5) | 100 (26.2) |
Metastasis | 269 (21.2) | 190 (21.4) | 79 (20.8) |
Total number of in situ/malignant tumors, n (%) | |||
≤3 | 1,249 (98.3) | 875 (98.4) | 374 (98.2) |
>3 | 21 (1.7) | 14 (1.6) | 7 (1.8) |
Marital status, n (%) | |||
Single | 216 (17.0) | 148 (16.6) | 68 (17.8) |
Married | 752 (59.2) | 533 (60.0) | 219 (57.5) |
Other | 302 (23.8) | 208 (23.4) | 94 (24.7) |
SES, n (%) | |||
Low | 505 (39.8) | 360 (40.5) | 145 (38.1) |
Moderate | 682 (53.7) | 477 (53.7) | 205 (53.8) |
High | 83 (6.5) | 52 (5.8) | 31 (8.1) |
SES, socioeconomic status; A/PI, Asian or Pacific Islander; STE, soft tissue of extremities; STOS, soft tissue of other sites; AI/AN, American Indian/Alaska Native; AJCC stage, American Joint Committee on Cancer stage.
Kaplan-Meier survival analysis
The OS curve was calculated using the Kaplan-Meier method in the training cohort. Most of the variables were significantly correlated with OS (P<0.05), except sex, race, radiotherapy, and SES (P>0.05) (Figure 2).

Univariate Cox regression analysis
In the univariate regression analysis, ten variables (age at diagnosis, marital status, tumor size, tumor site, tumor extension, tumor grade, AJCC stage, surgery status, chemotherapy, and total number of in situ or malignant tumors) were significantly associated with OS (P<0.05), whereas sex, race, SES, and radiotherapy were not significantly associated with OS (P>0.05) (Table 2).
Table 2
Variables | Univariate analysis | ||
---|---|---|---|
HR | 95% CI | P | |
Age at diagnosis | 1.053 | 1.043–1.063 | <0.001 |
Sex | |||
Male | Reference | ||
Female | 0.895 | 0.682–1.174 | 0.422 |
Race | |||
White | Reference | ||
Black | 0.835 | 0.476–1.467 | 0.531 |
A/PI | 1.172 | 0.730–1.882 | 0.512 |
AI/AN | 1.983 | 0.492–7.999 | 0.336 |
Site | |||
STE | Reference | ||
STOS | 0.975 | 0.717–1.324 | 0.869 |
Other | 2.376 | 1.212–4.659 | 0.012 |
Grade | |||
I | Reference | ||
II | 1.294 | 0.784–2.137 | 0.314 |
III | 2.861 | 1.718–4.765 | <0.001 |
IV | 2.618 | 1.623–4.223 | <0.001 |
AJCC stage | |||
I | Reference | ||
II | 1.811 | 1.276–2.569 | <0.001 |
III | 2.783 | 1.976–3.919 | <0.001 |
IV | 8.849 | 5.513–14.204 | <0.001 |
Surgery | |||
No | Reference | ||
Yes | 0.106 | 0.063–0.181 | <0.001 |
Radiotherapy | |||
No/unknown | Reference | ||
Yes | 0.825 | 0.630–1.080 | 0.162 |
Chemotherapy | |||
No/unknown | Reference | ||
Yes | 1.760 | 1.189–2.604 | <0.01 |
Tumor size (mm) | |||
≤50 | Reference | ||
>50 | 1.561 | 1.177–2.071 | <0.01 |
Extension | |||
Confined | Reference | ||
Localized | 1.738 | 1.253–2.412 | <0.001 |
Metastasis | 2.097 | 1.516–2.900 | <0.001 |
Total situ | |||
≤3 | Reference | ||
>3 | 3.748 | 1.985-7.079 | <0.001 |
Marital status | |||
Single | Reference | ||
Married | 1.207 | 0.777–1.876 | 0.403 |
Other | 2.480 | 1.567–3.926 | <0.001 |
SES | |||
Low | Reference | ||
Moderate | 1.168 | 0.879–1.552 | 0.285 |
High | 1.135 | 0.618–2.085 | 0.683 |
SES, socioeconomic status; A/PI, Asian or Pacific Islander; STE, soft tissue of extremities; STOS, soft tissue of other sites; AI/AN, American Indian/Alaska Native; Total situ, total number of in situ/malignant tumors; AJCC stage, American Joint Committee on Cancer stage.
Multivariate Cox regression analysis
Based on the results obtained from the Kaplan-Meier survival analysis, univariate Cox regression analysis and clinical reasoning, we included eleven variables in the multivariate analysis: age at diagnosis, AJCC stage, marital status, tumor grade, tumor size, tumor extension, tumor site, surgery, chemotherapy, radiotherapy, and the total number of in situ or malignant tumors. Multivariate analysis revealed that age at diagnosis, AJCC stage, tumor size, tumor extension, tumor site, surgery, chemotherapy, radiotherapy, and the total number of in situ or malignant tumors were the independent prognostic factors for MFS. Collinearity analysis showed that the VIFs among all the variables were <5 (Table S1).
Nomogram construction and validation
Multivariate Cox proportional hazards regression model indicated the risk of death was significantly increased for patients with older age, AJCC stage II–IV, chemotherapy, tumor size >50 mm, >3 in situ or malignant tumors, localized tumors, metastasized tumors, and primary tumors located in the bones, peritoneum, retroperitoneum, heart, and nerves (P<0.05). In contrast, the risk of death for patients who underwent surgery and radiotherapy was decreased significantly (P<0.05) (Figure 3, Table S2).
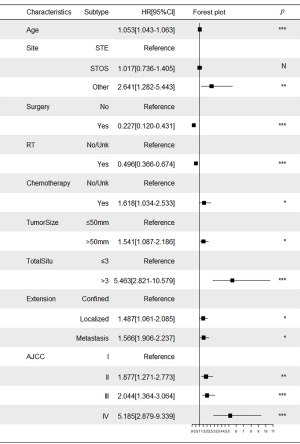
Finally, a novel nomogram was constructed to predict the 3- and 5-year OS using age, AJCC stage, chemotherapy, surgical status, radiotherapy, tumor size, total number of in situ or malignant tumors, tumor site, and tumor extension (Figure 4). Age at diagnosis, which had the highest effect, was converted into 100 points, and then the points assigned to the remaining variables were calculated to measure their effect size. The points were then added, and that sum corresponded to the predicted probability of 3- and 5-year OS. The predicted probability ranged from 0.1 to 0.9.
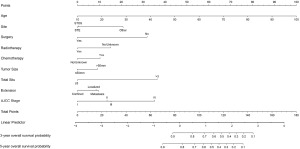
ROC curve analysis was performed to test the accuracy of the nomogram (Figure 5). The areas under the curve (AUCs) for 3- and 5-year OS in the training cohort were 0.769 and 0.759, respectively. Likewise, the validation cohort had relatively high AUCs of 0.793 and 0.752 for 3- and 5-year OS, respectively. The C-indexes of the nomogram were greater than those of the sixth edition of the AJCC staging system (Table 3). The calibration curves had an acceptable level of agreement between the predicted survival probabilities of the nomogram and the observed survivals (Figure 6).
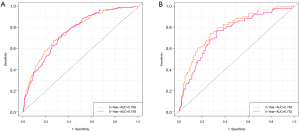
Table 3
Model | Training cohort | Validation cohort | |||
---|---|---|---|---|---|
C-index | 95% CI | C-index | 95% CI | ||
Nomogram | 0.806 | 0.804–0.808 | 0.783 | 0.778–0.788 | |
AJCC staging system | 0.669 | 0.666–0.672 | 0.674 | 0.668–0.680 |
C-CI, influence interval; index, concordance index; AJCC, American Joint Committee on Cancer.
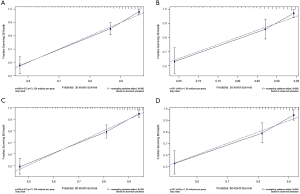
The NRI values for 3- and 5-year OS are shown in Table 4. The IDI values for 3- and 5-year OS were 0.157 (P<0.001) and 0.180 (P<0.001) in the training cohort, respectively, and 0.111 (P<0.001) and 0.134 (P<0.001) in the validation cohort, respectively. The efficacy of the new model was superior to that of the AJCC staging system.
Table 4
Cohort | 3-year | 5-year | |||
---|---|---|---|---|---|
NRI | 95% CI | NRI | 95% CI | ||
Training cohort | 0.741 | 0.580–0.923 | 0.777 | 0.626–0.958 | |
Validation cohort | 0.610 | 0.278–0.889 | 0.679 | 0.314–0.939 |
CI, influence interval; NRI, net reclassification improvement.
The DCA graphs showed a significant net benefit of the nomogram for almost all threshold probabilities in both the training and validation cohorts compared to the AJCC staging system (Figure 7).
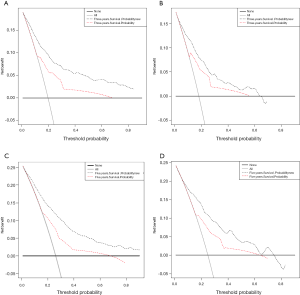
Kaplan-Meier curves showed that there were significant differences in survival between the high-risk group (PI >17.5) and the low-risk group (PI <17.5) (P<0.001) (Figure 8).
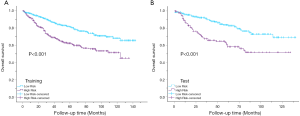
Discussion
Traditionally, treatment selection and outcome assessment for patients with malignant soft tissue sarcomas has relied on the TNM staging system to a great extent. Indeed, the TNM staging system has been widely used to stage malignant tumors for over 40 years. This is an anatomic staging method, which has the advantages of simple parameters, easy operation, and high universality. However, a clear disadvantage of this method is that it ignores some critical factors, such as age, tumor location, histological subtype, and treatment. Even though the latest edition of the AJCC staging system improved the tumor staging method and added new parameters to predict patient prognosis, the inherent defects of the staging system still exist (22). A nomogram is a novel effective tool for clinicians to estimate patient outcomes. In this study, we constructed a specific nomogram for MFS patients using the SEER database. Our nomogram included AJCC stage and other factors to predict the survival outcome. The C-indexes of the nomogram were superior to those of the AJCC staging system, 6th edition in both the training and validation cohorts. Furthermore, the DCA graph showed a significant net benefit of the nomogram. Finally, there were significant differences in survival among different risk groups (P<0.001), which proved that the nomogram could identify patients in different risk groups.
There are few relevant studies on prognosis prediction in MFS patients, and in the available studies, the sample size is usually limited to dozens to hundreds (28,29). The SEER database is a large database that collects information on cancer patients from 18 centers that cover 28% of the population of the United States, which could improve the generalizability of our training cohort and validation cohort to some extent. However, concerns still exist. In the current study, we conducted a large population-based study using the SEER database (n=1,270). The median age at diagnosis was 63 years, which was slightly lower than the previously reported range of 64–72 years (1,30,31). The median follow-up period was 41 months, which was lower than what has been observed in previous studies (51–53 months) (1,4). The 3- and 5-year OS rates were 80.7% and 74.3%, respectively, and the 3- and 5-year disease-specific survival rates were 90.2% and 87.3%, respectively, which suggested relatively good outcomes. In terms of tumor characteristics, the median size was 59 mm, which is smaller than the previously reported 60–95 mm (1,4,10). Tumors were most common in the extremities, especially in the lower extremities, which is consistent with the results of previous studies (1,6,10).
In the present study, we first identified the factors affecting the survival of patients with MFS. Age at diagnosis, AJCC stage, tumor size, tumor extension, tumor site, surgery status, chemotherapy, radiotherapy, and the total number of in situ or malignant tumors were the nine independent factors affecting survival. We found that age at diagnosis had the greatest effect on survival: the older the patient at diagnosis, the worse the outcome. Previous studies have shown a significant increase in LR in patients older than 65 years (2), which may explain why age affects survival. Similarly, survival outcomes were worse in patients with tumors >5 cm. It has been reported that the prognosis of patients with tumors >7.5 cm is significantly poorer than those with smaller tumors (3). We did not identify tumor grade as an independent prognostic factor for OS in multivariate Cox analysis, which differs from the results of the study by Sambri et al. (16,30,31). Considering that there may be collinearity between AJCC stage and tumor grade, which can lead to deviation in the conclusion, we performed collinearity analysis. All of the factors had a VIF <5, suggesting that there was no collinearity between AJCC stage and grade.
Although there is currently no standard treatment for MFS (10), surgery was the most common treatment in this study (97.3%). However, it can be difficult for surgeons to achieve negative margins in some areas, and adjuvant radiotherapy is particularly critical (31-34). Boughzala-Bennadji et al. confirmed that a combination of R0 resection (absence of tumor within 1 mm from the inked surface) and adjuvant radiation therapy provided the best local control (4). In our study, univariate Cox regression analysis suggested that radiotherapy was not significantly associated with OS (P=0.162). However, considering the clinical significance and the P value, we decided to include it in the multivariate analysis. The results showed that radiotherapy was indeed an independent factor beneficial to survival (P<0.001, VIF <5). In addition, multivariate Cox regression analysis showed that chemotherapy was an independent poor prognostic factor (hazard ratio =1.618, P<0.035, and VIF <5). However, we cannot arbitrarily assume that chemotherapy increased the risk of death because we cannot state whether patients who received chemotherapy represented a high-risk population. Therefore, further randomized controlled trials or propensity score matching studies should examine this question. Moreover, the SEER database unfortunately does not include the details of radiotherapy and chemotherapy regimens, which leads to the limited applicability of our conclusions.
There were some limitations to our study. First, the information contained in the database is incomplete. In particular, SEER has highly limited data regarding chemotherapy and radiation therapy, such as the dose of radiotherapy and chemotherapeutic drugs, and only includes those used as front-line therapy. In addition to LR, a distinctive feature of MFS is that it has a high rate of LR, and recurrent sarcoma tends to be of higher grade. Therefore, research on recurrence as a risk factor or as an outcome is of great significance (30,35,36). Second, we excluded many patients because of the strict inclusion and exclusion criteria, resulting in a 43% reduction in sample size, which to some extent limits the validity of the conclusions that can be drawn. Third, there is variability in the predictions when a nomogram is used in the clinic (24). This specific nomogram applies only to patient populations similar to the cohort we studied.
Conclusions
In conclusion, age at diagnosis, tumor size, tumor site, tumor extension, AJCC stage, surgery, chemotherapy, radiotherapy and the total number of in situ or malignant tumors were pertinent factors affecting OS in patients with MFS. A novel nomogram that included tumor characteristics and treatment methods was constructed to evaluate survival outcomes for MFS patients. Its predictive efficacy was markedly superior to the traditional AJCC staging system.
Acknowledgments
The authors sincerely appreciate the staffs who have made efforts in the SEER program. We would like to thank Editage (www.editage.cn) for English language editing.
Funding: None.
Footnote
Reporting Checklist: The authors have completed the TRIPOD reporting checklist Available at http://dx.doi.org/10.21037/tcr-20-2588
Peer Review File: Available at http://dx.doi.org/10.21037/tcr-20-2588
Conflicts of Interest: All authors have completed the ICMJE uniform disclosure form (available at http://dx.doi.org/10.21037/tcr-20-2588). The authors have no conflicts of interest to declare.
Ethical Statement: The authors are accountable for all aspects of the work in ensuring that questions related to the accuracy or integrity of any part of the work are appropriately investigated and resolved. The study was conducted in accordance with the Declaration of Helsinki (as revised in 2013). Institutional review board approval was not required in the current study because the SEER research data is publicly available. Moreover, we registered and got access to the SEER database (accession No. 16375-Nov2018).
Open Access Statement: This is an Open Access article distributed in accordance with the Creative Commons Attribution-NonCommercial-NoDerivs 4.0 International License (CC BY-NC-ND 4.0), which permits the non-commercial replication and distribution of the article with the strict proviso that no changes or edits are made and the original work is properly cited (including links to both the formal publication through the relevant DOI and the license). See: https://creativecommons.org/licenses/by-nc-nd/4.0/.
References
- Sanfilippo R, Miceli R, Grosso F, et al. Myxofibrosarcoma: prognostic factors and survival in a series of patients treated at a single institution. Ann Surg Oncol 2011;18:720-5. [Crossref] [PubMed]
- Odei B, Rwigema JC, Eilber FR, et al. Predictors of Local Recurrence in Patients With Myxofibrosarcoma. Am J Clin Oncol 2018;41:827-31. [Crossref] [PubMed]
- Haglund KE, Raut CP, Nascimento AF, et al. Recurrence patterns and survival for patients with intermediate- and high-grade myxofibrosarcoma. Int J Radiat Oncol Biol Phys 2012;82:361-7. [Crossref] [PubMed]
- Boughzala-Bennadji R, Stoeckle E, Le Pechoux C, et al. Localized Myxofibrosarcomas: Roles of Surgical Margins and Adjuvant Radiation Therapy. Int J Radiat Oncol Biol Phys 2018;102:399-406. [Crossref] [PubMed]
- Wibmer C, Leithner A, Zielonke N, et al. Increasing incidence rates of soft tissue sarcomas? A population-based epidemiologic study and literature review. Ann Oncol 2010;21:1106-11. [Crossref] [PubMed]
- Mühlhofer HM, Lenze U, Gersing A, et al. Prognostic Factors and Outcomes for Patients With Myxofibrosarcoma: A 13-Year Retrospective Evaluation. Anticancer Res 2019;39:2985-92. [Crossref] [PubMed]
- Iwata S, Yonemoto T, Araki A, et al. Impact of infiltrative growth on the outcome of patients with undifferentiated pleomorphic sarcoma and myxofibrosarcoma. J Surg Oncol 2014;110:707-11. [Crossref] [PubMed]
- Sbaraglia M, Dei Tos AP. The pathology of soft tissue sarcomas. Radiol Med 2019;124:266-81. [Crossref] [PubMed]
- Roland CL, Wang WL, Lazar AJ, et al. Myxofibrosarcoma. Surg Oncol Clin N Am 2016;25:775-88. [Crossref] [PubMed]
- Look Hong NJ, Hornicek FJ, Raskin KA, et al. Prognostic factors and outcomes of patients with myxofibrosarcoma. Ann Surg Oncol 2013;20:80-6. [Crossref] [PubMed]
- Hu Q, Zhou S, Hu X, et al. Systematic screening identifies a 2-gene signature as a high-potential prognostic marker of undifferentiated pleomorphic sarcoma/myxofibrosarcoma. J Cell Mol Med 2020;24:1010-21. [Crossref] [PubMed]
- Kiyuna T, Tome Y, Murakami T, et al. A combination of irinotecan/cisplatinum and irinotecan/temozolomide or tumor-targeting Salmonella typhimurium A1-R arrest doxorubicin- and temozolomide-resistant myxofibrosarcoma in a PDOX mouse model. Biochem Biophys Res Commun 2018;505:733-9. [Crossref] [PubMed]
- Miserocchi G, De Vita A, Mercatali L, et al. Characterization and Drug Sensitivity of a New High-Grade Myxofi-brosarcoma Cell Line. Cells 2018;7:186. [Crossref] [PubMed]
- Stefano S, Giovanni S. The PTEN Tumor Suppressor Gene in Soft Tissue Sarcoma. Cancers (Basel) 2019;11:1169. [Crossref] [PubMed]
- Tsukahara T, Watanabe K, Murata K, et al. Peptide vaccinations elicited strong immune responses that were re-boosted by anti-PD1 therapy in a patient with myxofibrosarcoma. Cancer Immunol Immunother 2020;69:189-97. [Crossref] [PubMed]
- Tsuchie H, Kaya M, Nagasawa H, et al. Distant metastasis in patients with myxofibrosarcoma. Ups J Med Sci 2017;122:190-3. [Crossref] [PubMed]
- Song B, Lee K, Lee C, et al. Prognostic significance of microscopic tumor extension in local recurrence of myxofibrosarcoma and undifferentiated pleomorphic sarcoma. Pathology International 2018;68:509-16. [Crossref] [PubMed]
- Sambri A, Bianchi G, Righi A, et al. Surgical margins do not affect prognosis in high grade myxofibrosarcoma. Eur J Surg Oncol 2016;42:1042-8. [Crossref] [PubMed]
- Manoso MW, Pratt J, Healey JH, et al. Infiltrative MRI pattern and incomplete initial surgery compromise local control of myxofibrosarcoma. Clin Orthop Relat Res 2006;89-94. [Crossref] [PubMed]
- Sambri A, De Paolis M, Spinnato P, et al. The Biology of Myxofibrosarcoma: State of the Art and Future Perspectives. Oncol Res Treat 2020;43:314-22. [Crossref] [PubMed]
- Ogura K, Hosoda F, Arai Y, et al. Integrated genetic and epigenetic analysis of myxofibrosarcoma. Nat Commun 2018;9:2765. [Crossref] [PubMed]
- Callegaro D, Miceli R, Mariani L, et al. Soft tissue sarcoma nomograms and their incorporation into practice. Cancer 2017;123:2802-20. [Crossref] [PubMed]
- Kattan MW, Leung D, Brennan MF, et al. Postoperative nomogram for 12-year sarcoma-specific death. J Clin Oncol 2002;20:791-6. [Crossref] [PubMed]
- Iasonos A, Schrag D, Raj GV, et al. Panageas KS: How to build and interpret a nomogram for cancer prognosis. J Clin Oncol 2008;26:1364-70. [Crossref] [PubMed]
- Doll KM, Rademaker A, Sosa JA, et al. Practical Guide to Surgical Data Sets: Surveillance, Epidemiology, and End Results (SEER) Database. JAMA Surg 2018;153:588-9. [Crossref] [PubMed]
- Yu JB, Gross CP, Wilson LD, et al. NCI SEER public-use data: applications and limitations in oncology research. Oncology 2009;23:288-95. [PubMed]
- Zhang J, Pan Z, Zhao F, et al. Development and validation of a nomogram containing the prognostic determinants of chondrosarcoma based on the Surveillance, Epidemiology, and End Results database. Int J Clin Oncol 2019;24:1459-67. [Crossref] [PubMed]
- Stefanovski PD, Bidoli E, De Paoli A, et al. Prognostic factors in soft tissue sarcomas: a study of 395 patients. Eur J Surg Oncol 2002;28:153-64. [Crossref] [PubMed]
- Penel N, Coindre JM, Giraud A, et al. Presentation and outcome of frequent and rare sarcoma histologic subtypes: A study of 10,262 patients with localized visceral/soft tissue sarcoma managed in reference centers. Cancer 2018;124:1179-87. [Crossref] [PubMed]
- Sambri A, Zucchini R, Giannini C, et al. Systemic Inflammation Is Associated with Oncological Outcome in Patients with High-Grade Myxofibrosarcoma of the Extremities: A Retrospective Analysis. Oncology Research and Treatment 2020;43:531-8. [Crossref] [PubMed]
- Sambri A, Tuzzato G, Spinnato P, et al. Grading in Myxofibrosarcoma of the Extremities Can Predict Survival and Local Control. Oncol Res Treat 2020;43:189-95. [Crossref] [PubMed]
- Sambri A, Spinnato P, Bazzocchi A, et al. Does pre-operative MRI predict the risk of local recurrence in primary myxofibrosarcoma of the extremities? Asia Pac J Clin Oncol 2019;15:e181-e186. [Crossref] [PubMed]
- Fujiwara T, Stevenson J, Parry M, et al. What is an adequate margin for infiltrative soft-tissue sarcomas? Eur J Surg Oncol 2020;46:277-81. [Crossref] [PubMed]
- Ghazala CG, Agni NR, Ragbir M, et al. Myxofibrosarcoma of the extremity and trunk: a multidisciplinary approach leads to good local rates of LOCAL control. Bone Joint J 2016;98-B:1682-8. [Crossref] [PubMed]
- Spinnato P, Clinca R, Vara G, et al. MRI Features as Prognostic Factors in Myxofibrosarcoma: Proposal of MRI Grading System. Acad Radiol 2020; Epub ahead of print. [Crossref] [PubMed]
- Spinnato P, Sambri A, Fujiwara T, et al. Myxofibrosarcoma: clinical and prognostic value of MRI features. Curr Med Imaging 2020. [Epub ahead of print]. doi:
10.2174/1573405616999200729152135 .