Identification of immune-associated lncRNAs as a prognostic marker for lung adenocarcinoma
Introduction
Lung cancer is one of the most prevalent malignancy with approximately 2.1 million new cases and 1.59 million deaths worldwide in 2018 (1). Lung adenocarcinoma (LUAD) is the most common pathological subtype of non-small cell lung cancer (NSCLC) and amounts to 40% of all lung cancers across the world (1,2). Previous studies have reported that despite the development of targeted molecular therapies and treatment strategies, the 5-year survival rates in LUAD patients remain low, especially in advanced patients (3-5). Cancer immunotherapy shows great promise and immune-checkpoint inhibitors for PD-L1, CTLA-4, and TIM-3 have demonstrated effectiveness in treating advanced lung cancer (6-8). However, the selection criteria for sensitive populations suitable for immunotherapy remains undefined (7,9). A growing number of studies have recognized that the tumour microenvironment (TME) plays a critical role in LUAD and the fraction of stromal cells in the TME may influence the patients’ prognosis (10). Recent studies have indicated that a high immune score is associated with improved survival in LUAD, which suggests that the immune microenvironment may be a prognostic factor (11).
LncRNAs have a length of over 200 nucleotides without coding functions and studies have demonstrated an array of biological functions (12,13). An increasing number of abnormal biological behaviours in tumours have been linked to lncRNAs including the unusual activity of the genetic material and changes in the TME, which are involved in processes promoting tumour progression such as immune escape, DNA damage, metabolic disorders, epithelial-mesenchymal transition, cell stemness, and chemical resistance (14). Previous studies have also investigated the regulation of tumour immunity by lncRNAs (13,15,16). For example, lncRNA LNMAT1 affects lymphatic metastasis of bladder cancer by inducing CCL2 in the TME via the recruitment of tumour-associated macrophages (17). In addition, Lnc-SNHG1 promotes immune escape of breast cancer by increasing the differentiation of regulatory T cells (18). The prognostic value of lncRNAs in NSCLC have also received an increasing amount of interest. For instance, a risk signature composed of 7 lncRNAs has been proposed by Lin et al. to predict overall survival (OS) for early-stage NSCLC patients (19) and Miao et al. showed similar findings for 8 lncRNAs in elderly NSCLC patients (20). However, the prognostic value of immune-associated lncRNAs has not been widely studied.
In this study, we obtained 12 immune-associated and prognostic lncRNAs according to the co-expression network and univariate Cox proportional hazards regression analysis. Then we constructed a risk signature of 7 immune-associated lncRNAs that clustered LUAD samples into low- or high-risk based on this signature and we validated the signature’s prognostic and clinical value. Unlike previous studies, we analysed the immune status using 29 immune-associated gene sets, which represented diverse immune cell types, functions, and pathways based on previous literature (21-23), and employed the ESTIMATE algorithm to verify consistency of the results. Furthermore, we analysed the characteristics of genomic alterations including somatic mutations and copy number variations in multiple risk groups. Finally, we applied the risk model to pancreatic ductal adenocarcinoma (PDAC) samples to explore whether the model is valid for other tumours.
We present the following article in accordance with the MDAR checklist (available at http://dx.doi.org/10.21037/tcr-20-2827).
Methods
Data acquisition and processing
Gene expression profiles and clinicopathological features of LUAD and PDAC patients were downloaded from The Cancer Genome Atlas (TCGA, https://portal.gdc.cancer.gov/) with standardised gene expression in fragments per kilobase million. The following exclusion criteria were applied to ensure data integrity: (I) patients with survival time less than 30 days and (II) missing information on survival status, TNM, AJCC stage, age, and gender. We included a total of 499 LUAD patients and 170 PDAC patients in this study. The 499 LUAD patients were randomly assigned to a training set (N=351) or a validation set (N=148) in a 7:3 ratio (Table S1). The gene transfer format was downloaded from GENCODE (https://www.gencodegenes.org/) to convert the Entrez IDs to gene IDs (24,25) and the lncRNA profiles were extracted from mRNA expression data. We obtained 332 immune‐related genes categorised as immune system process (M13664) or immune response (M19817) through the molecular signatures database (http://www.broadinstitute.org/gsea/msigdb/index.jsp) (21,26). The expression of immune-related genes was further correlated with lncRNA expressions based on Pearson’s correlation analysis.
Risk score construction and survival analysis
Univariate and multivariate Cox regression and least absolute shrinkage and selection operator (LASSO) regression analysis (27) were performed on the immune-associated lncRNAs based on lncRNAs expressions and survival data from patients in the training group. The risk scores were obtained according to the following algorithm as described previously (20,28).
Where Expi is the normalised expression level of each of the immune-associated lncRNAs and Coei is the weighted regression coefficient of each item (20). LUAD patients were subsequently divided into high- and low-risk group based on the cut-off value of the median risk score of the training group. Kaplan-Meier survival analysis and receiver operating characteristic (ROC) curve was used to differentiate the survival time between low- and high-risk LUAD patients.
Evaluation of immune status and somatic mutations status
We used 29 immune signatures in the single-sample gene-set enrichment analysis (ssGSEA) to analyse the immune status of the high- and low-risk samples as described previously (23). ESTIMATE algorithm was performed to evaluate the immune cell infiltration level (immune score), stromal content (stromal score), and tumour purity (29). Expression patterns in the different groups were investigated by principal component analysis (PCA) and gene set enrichment analysis (GSEA) was performed to study the different biological processes and pathways enriched in the high- or low-risk groups (http://www.broadinstitute.org/gsea/index.jsp). All gene somatic mutations of LUAD and PDAC samples were also downloaded from TCGA. We then analysed the variant classification and type, classification summary, single nucleotide variation (SNV) class, variants per sample, and top ten mutated genes.
Statistical analysis
R version 4.0.1 and SPSS Statistics version 23.0 were used for all statistical analyses. To ensure equal clinical baseline of the training and validation set, we applied the Chi-squared test, Fisher’s exact test, or Student’s t-test. Wilcoxon-Mann-Whitney or Kruskal-Wallis tests were used to compare different groups depending on the number of comparisons. All reported P values were two-tailed and P<0.05 was considered statistically significant.
The study was conducted in accordance with the Declaration of Helsinki (as revised in 2013).
Results
Model construction of 7 immune-associated lncRNAs in the training group
The expression of 332 immune-associated genes in LUAD samples are shown in https://cdn.amegroups.cn/static/public/TCR-20-2827-1.xlsx. A total of 788 immune-associated lncRNAs were identified by the Pearson’s correlation analysis with |R| >0.5 and P<0.001 (https://cdn.amegroups.cn/static/public/TCR-20-2827-2.xlsx). Univariate Cox proportional hazards regression analysis was then performed on these 788 lncRNAs in the training set, resulting in 12 lncRNAs with a P value of <0.01 (https://cdn.amegroups.cn/static/public/TCR-20-2827-3.xlsx). We further applied LASSO for the 12 lncRNAs, of which the following 9 lncRNAs significantly correlated with the survival of LUAD patients: AP000695.2, AC026355.2, LINC01843, ITGB1-DT, DPYD-AS1, LINC01150, AL590226.1, AC091185.1, and AC005034.3 (Figure 1A). Multivariate Cox regression was performed and the coefficients were obtained to construct a risk score composed of 7 immune-associated lncRNAs with the following formula.
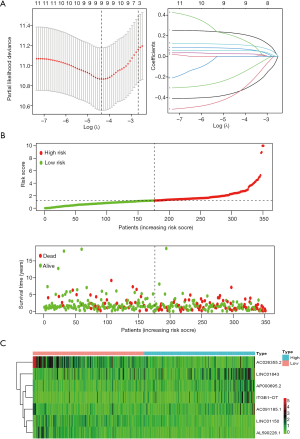
Risk score =0.251 × AP000695.2 - 0.334 × AC026355.2 + 0.085 × LINC01843 + 0.123 × ITGB1-DT - 0.476 × LINC01150 - 0.583 × AL590226.1 + 0.524 × AC091185.1 (Table 1). According to the median risk score, the training set was divided into the high- or low-risk group. The relationship between risk score and cancer-related mortality showed that the mortality rate in the low-risk group was significantly lower than in the high-risk group (Figure 1B). Moreover, a heatmap of the expression level of the 7 immune-associated lncRNAs revealed that AP000695.2, LINC01843, ITGB1-DT, and AC091185.1 may play a harmful role in LUAD whereas AC026355.2, LINC01150, and AL590226.1 may exert protective effects (Figure 1B,C).
Table 1
lncRNA_symbol | Ensemble ID | Coefficient | Univariate Cox regression analysis | |||
---|---|---|---|---|---|---|
HR | 95% CI lower | 95% CI higher | P value | |||
AP000695.2 | ENSG00000233818 | 0.251 | 1.411 | 1.153 | 1.726 | <0.01 |
AC026355.2 | ENSG00000236385 | −0.334 | 0.771 | 0.647 | 0.918 | <0.01 |
LINC01843 | ENSG00000251169 | 0.085 | 1.111 | 1.042 | 1.184 | <0.01 |
ITGB1-DT | ENSG00000229656 | 0.123 | 1.194 | 1.075 | 1.326 | <0.01 |
LINC01150 | ENSG00000229671 | −0.476 | 0.527 | 0.328 | 0.848 | <0.01 |
AL590226.1 | ENSG00000228401 | −0.583 | 0.397 | 0.217 | 0.727 | <0.01 |
AC091185.1 | ENSG00000253476 | 0.524 | 1.343 | 1.09 | 1.655 | <0.01 |
Validation of the prognostic value of immune-associated lncRNA signature
Further examination of the validation and combination set performed with the same algorithm, risk-score formula, and cut-off value showed similar results as expected and confirmed the findings outlined above (Figure 2A,B). We next explored the relationship between the lncRNA risk signature and the OS of LUAD patients. Both the training and validation groups showed that patients in the low-risk group exhibited longer OS than the high-risk group (Figure 2C). Moreover, the lncRNA risk signature showed excellent AUC values (>0.65 at 1, 3, and 5 years) in the ROC analysis in all cohorts (Figure 2D and Figure S1), indicating effective prediction of survival by our lncRNA risk signature.
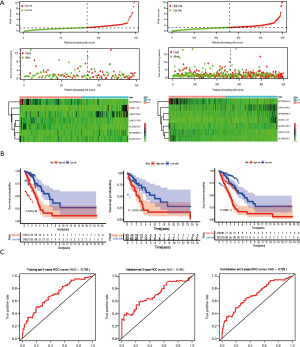
Clinical correlation and independent risk factor analysis
We subsequently explored the underlying mechanisms by comparing the correlation between the lncRNA risk signature and clinical pathological characteristics. We observed that TNM stage, gender, and AJCC stage were significantly associated with the expression of the 7 lncRNAs in the combination set (Figure 3A and Figure S2), which was particularly remarkable in AP000695.2, ITGB1-DT, LINC01150, and AL590226.1. ITGB1-DT expression was positively correlated with T stage (P<0.01), N stage (P<0.05), and AJCC stage (P<0.01) and its expression level was higher in males than in females (P<0.01). Interestingly, the expression of the four lncRNAs significantly associated with OS independently (Figure 3B). Kaplan-Meier analysis was performed after risk stratification by AJCC stage, gender, age, and TNM stage (Figure 3C and Figure S2). Patients in the low-risk group showed improved OS compared with patients with high-risk for stage I/II (P<0.001), stage III/IV (P=0.02799), age ≤65 (P<0.001), age >65 (P<0.001), male (P<0.001), and female (P<0.001). Multivariate Cox analysis was performed to define the independent risk factors in the training, validation, and combination sets. The results suggested that in the combination set, AJCC stage and the lncRNA risk signature were independent prognostic factors that were significantly associated with OS. However, only the lncRNA risk signature was an independent prognostic factor in the training and validation set (Table 2). These data further support that the 7 immune-associated lncRNAs may be an independent predictor for the prognosis of LUAD patients.
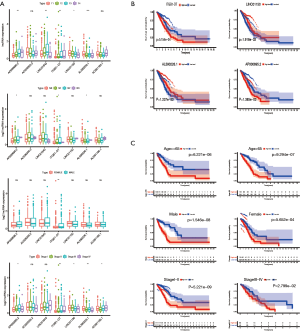
Table 2
Variable | Training set | Validation set | Combination set | |||||
---|---|---|---|---|---|---|---|---|
HR (95% CI) | P value | HR (95% CI) | P value | HR (95% CI) | P value | |||
Age, years | ||||||||
≤65 | 1 | 1 | 1 | |||||
>65 | 1.39 (0.93–2.09) | 0.11 | 0.64 (0.34–1.21) | 0.17 | 1.16 (0.84–1.61) | 0.37 | ||
Gender | ||||||||
Female | 1 | 1 | 1 | |||||
Male | 1.04 (0.67–1.57) | 1.00 (0.54–1.85) | 0.99 | 1.00 (0.72–1.40) | 0.98 | |||
T stage | ||||||||
T1 | 1 | 0.86 | 1 | 1 | ||||
T2 | 1.10 (0.65–1.84) | 0.73 | 0.90 (0.45–1.82) | 0.77 | 1.04 (0.69–1.55) | 0.86 | ||
T3-T4 | 1.43 (0.69–3.01) | 0.34 | 1.68 (0.51–5.51) | 0.4 | 1.41 (0.78–2.56) | 0.26 | ||
N stage | ||||||||
N0 | 1 | 1 | 1 | |||||
N1 | 1.04 (0.49–2.19) | 0.92 | 1.91 (0.51–7.16) | 0.34 | 1.19 (0.66–2.15) | 0.56 | ||
N2-N3 | 0.76 (0.22–2.55) | 0.65 | 1.31 (0.23–7.54) | 0.76 | 0.89 (0.34–2.32) | 0.81 | ||
NX | 1.37 (0.18–10.24) | 0.76 | 12.049 (1.25–116.04) | 0.03 | 2.20 (0.52–9.16) | 0.28 | ||
M stage | ||||||||
M0 | 1 | 1 | 1 | |||||
M1 | 0.76 (0.23–2.51) | 0.65 | 0.52 (0.06–4.47) | 0.55 | 0.79 (0.30–2.07) | 0.64 | ||
MX | 0.90 (0.56–1.44) | 0.67 | 1.23 (0.60–2.57) | 0.57 | 0.93 (0.63–1.37) | 0.71 | ||
AJCC stage | ||||||||
Stage I | 1 | 1 | 1 | |||||
Stage II | 1.71 (0.78–3.76) | 0.18 | 2.66 (0.64–11.02) | 0.18 | 2.01 (1.07–3.79) | 0.03 | ||
Stage III-IV | 3.32 (0.89–12.42) | 0.07 | 4.95 (0.64–38.41) | 0.13 | 3.33 (1.17–9.49) | 0.02 | ||
Risk score | ||||||||
Low risk | 1 | 1 | 1 | |||||
High risk | 2.61 (1.67–4.08) | <0.001 | 3.48 (1.86–6.51) | <0.001 | 2.69 (1.89–3.81) | <0.001 |
Low risk score was associated with highly active immune status
To investigate the immune status in the different risk groups, 29 immune-associated gene sets were analysed in the combination set. ssGSEA was performed to visualise the enrichment levels, functions, or pathways of immune cells in LUAD patients. We found that the low-risk group showed a separate cluster from the high-risk group with a higher ssGSEA score (Figure 4A). Similar results were obtained when the low- or high-risk group was scored by the ESTIMATE algorithm. We observed that the immune and stromal scores were significantly elevated in the low-risk group compared with the high-risk group (P<0.001, Figure 4B). In contrast, tumour purity was significantly lowered in the low-risk group (P<0.001, Figure 4C). We used PCA to evaluate the different distribution patterns in the immune-associated gene expression set and the whole genome expression set between low- and high-risk LUAD patients. The status of the patients in the low- and high-risk group was well distinguished in the immune-associated gene expression set. While the separation was not as clear when using the whole genome expression set, the two groups were still distinguishable (Figure 4D). These results showed that the 7 immune-associated lncRNAs may represent the immune status of the patient, in which the low-risk group harboured the highest number of immune and stromal cells in the TME whereas the high-risk group showed the highest number of tumour cells.
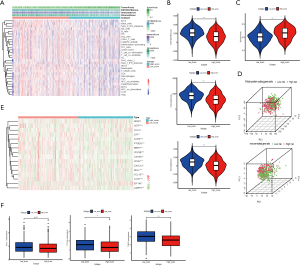
Recently, tertiary lymphatic structures (TLSs) have gained scientific interest as a pathologic marker for immunotherapy in a variety of tumours (30-32). To investigate whether the risk score was related to TLSs in the LUAD immune micro-environment, we compared the expression level of the TLS signatures (CD79B, CD1D, CCR6, LAT, SKAP1, CETP, EIF1AY, RBP5, and PTGDS) (30) and TLS-hallmark genes (CCL19, CCL21, CXCL13, CCR7, CXCR5, SELL, and LAMP3) (33) between the low- and high-risk group (Figure 4E). These data suggested that TLSs may be more active in the low-risk group and may explain why patients in the low-risk group had a higher immune status.
In addition, we compared the expression level of PD-L1, CTLA-4, and TIM-3 in the two groups, which may be associated with a patient’s immunotherapeutic responsiveness. We found higher PD-L1, CTLA-4, and TIM-3 expression levels in the low-risk group compared with the high-risk group (P<0.1, P<0.01, and P<0.001, respectively, Figure 4F). This indicated that patients in the low-risk group may respond better to immunotherapy than those in the high-risk group.
Association of cancer-related pathways and tumour mutation burden (TMB) with immune-associated lncRNA signature
We employed GSEA to discover the biological processes and pathways in the low- and high-risk groups in the combination set. As expected, the low-risk group showed significant enrichment in the gene sets related to the immune system in LUAD such as “IMMUNE_RESPONSE” (P=0.02, NSE =−1.94), “IMMUNE_SYSTEM_PROCESS” (P=0.004, NSE =−1.95), “KEGG_CYTOKINE_CYTOKINE_RECEPTOR_INTERACTION” (P=0.039, NSE =−1.68), and “GO_CYTOKINE_ RECEPTOR_ ACTIVITY” (P=0.002, NSE =−1.99). These data further underlined that a low-risk score may represent a higher immune status in LUAD. The high-risk group had the following significantly enriched gene sets: “GO_MITOTIC_NUCLEAR_DIVISION” (P<0.001, NSE =2.52), “GO_DNA_REPLICATION” (P<0.001, NSE =2.49), “GO_REGULATION_OF_SIGNAL_TRANSDUCTION_BY_P53_CLASS_MEDIATOR” (P<0.001, NSE =2.43), “KEGG_CELL_CYCLE” (P<0.001, NSE =2.32), and “KEGG_P53_SIGNALING_PATHWAY” (P=0.002, NSE =1.98, Figure 5A), which have been reported to determine cancer cell division and proliferation. We observed unusually active signalling in tumour suppressor protein p53 (TP53). TP53 is the most commonly mutated gene in more than 50% of all human cancers including NSCLC (34). Next, we identified the somatic mutations in patients with LUAD and investigated the relationship between the risk score and the TMB. Figure 5B illustrates the variant classification, variant type, and SNV class and Figure 5C shows the variants per sample, variant classification summary, and top ten mutated genes in LUAD patients. TTN (41%), MUC16 (40%), RYR2 (34%), CSMD3 (34%), TP53 (47%), LRP1B (29%), USH2A (27%), ZFHX4 (27%), XIRP2 (22%), and KRAS (25%) were the top 10 mutant genes in LUAD, which were comparable to previous reports (34,35). TP53 was the most frequently mutated gene in LUAD and the main types of mutations were missense mutations (Figure 5C,D). TMB was also significantly higher in the high-risk group (P<0.001, Figure 5E), which may explain why patients in the high-risk group had reduced OS compared with low-risk patients.
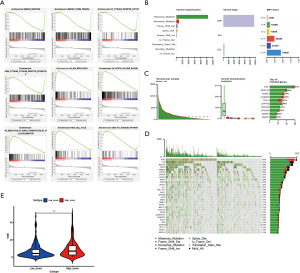
Prognostic value of immune-associated lncRNA risk signature may be applicable in other tumours
In the GSEA analysis outlined above, we found that “KEGG_PANCREATIC_ CANCER” (P=0.01, NSE =1.77) was also significantly enriched in the high-risk group (Figure 6A). Thus, we examined whether the prognostic value of the 7 immune-associated lncRNAs is also applicable in PDAC. We downloaded the expression of the 7 lncRNAs in PDAC samples from TCGA and established survival curves for the low- and high-risk groups. PDAC patients with low-risk scores had a significantly longer OS than high-risk patients (P<0.001, Figure 6B). ssGSEA and ESTIMATE analyses revealed that low- and high-risk groups were segregated (Figure 6C). The low-risk group showing higher ssGSEA score and proportion of immune and stromal cells, which was consistent with our results in LUAD samples (P<0.001, Figure 6D). However, unlike in LUAD patients, the mutation variants per sample in PDAC were lower (33.5/Mb vs. 140/Mb) (Figure 6E), although similar results were obtained when comparing the TMB between the low- and high-risk groups (P<0.001, Figure 6F). These data indicated that the 7 immune-associated lncRNAs may be able to predict patient survival in other tumours as well.
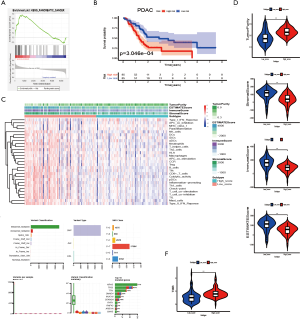
Discussion
NSCLC is one of the most common causes of cancer morbidity and mortality globally, in which LUAD accounts for a large proportion (1,2). Despite advances in treatment methods in recent years, the prognosis of patients remains poor (3-5). Immunotherapy has been regarded as the most promising treatment since its introduction to the clinic. However, determining the eligibility of a patient is still an unsolved issue (6-8). One of the causes is the lack of effective biomarkers or risk models to guide physicians (7,8). In the recent years, lncRNA has attracted a significant amount of attention due to its wide range of biological effects in carcinogenesis and tumour progression (13,15,16). The role of lncRNA in immune regulation has also been reported. For instance, LncNKILA enhances T cell sensitivity by NF-κB-induced apoptosis and knockdown lncNKILA effectively attenuates tumour-specific cytotoxic T cells and improves patient survival in lung cancer (36). Therefore, immune-associated lncRNAs can be used to distinguish different immune statuses and predict a patient’s prognostic risk. In this study, we constructed a novel model to predict prognosis and survival using immune-related lncRNA.
A total of 788 immune-associated lncRNAs were derived from the expression of 332 immune-related genes in LUAD samples from TCGA based on Pearson’s correlation analysis. Univariate and multivariate Cox regression and LASSO regression analysis was performed to construct an immune-associated lncRNA signature consisting of 7 lncRNAs, which was able to determine the prognosis of samples in the training, validation, and combination group. 1-, 3-, and 5-year ROC analyses also demonstrated the feasibility of our prognostic lncRNA risk signature. Through stratified analysis, we further found that the immune-associated lncRNA risk signature may be used to distinguish the survival outcomes of patients with different clinical variables. Moreover, multivariate Cox analysis revealed that the lncRNA risk signature was an independent prognostic factor. These 7 immune-associated lncRNAs were first reported in lung cancer and AP000695.2, ITGB1-DT, LINC01150, and AL590226.1 appeared to be closely related to clinical characteristics and independently predicted survival, although further investigations are required. These data revealed that immune-associated lncRNAs may be good prognostic indicators.
To investigate the TME in LUAD, ssGSEA and ESTIMATE analyses were performed to evaluate the immune and stromal status as well as tumour purity. We observed elevated immune activity in the low-risk group compared with the high-risk group, resulting in a significantly higher immune and stromal score while the high-risk group had higher tumour purity, suggesting that innate and adaptive immunity in the TME of low-risk LUAD patients were more aggressive. These findings were confirmed in subsequent GSEA analysis. Combining these results with clinical parameters, we postulate that the expression of immune checkpoint genes is a necessary indicator for screening patients before immunotherapy and that TLS is the most promising biomarker for guiding immunotherapy (28-31). We examined the differences in the expression of these genes including PD-L1, CTLA4, TIM3, and TLS signature and TLS hallmark genes (6-8) in the low- and high-risk groups. The expression of most of these genes were higher in the low-risk group, suggesting that the low-risk group may better respond to immunotherapy. The data above revealed that according to the risk model of the 7 immune-associated lncRNAs, a higher proportion of immune cells and stromal cells were present in the TME. ssGSEA and ESTIMATE analyses indicated a stronger immune-related response, which was reflected in the high expression of signals for immune-related pathways and TLS-related genes in tissues of low-risk patients, indicating that low-risk patients with LUAD may be more responsive to immunotherapy.
Somatic mutations have been confirmed to be a key aspect of carcinogenesis and cancer development in previous studies (37-39). Gao et al. demonstrated that the expression of some lncRNAs is affected by somatic mutations and were commonly downregulated while carrying low mutation frequencies and non-silent mutations in most cancer types, thus influencing the molecular pathogenesis (38). In our study, we found that the high-risk group was more likely to harbour enriched pathways related to cancer cell division and proliferation. In addition, the signalling pathway of the most commonly mutated gene TP53 was also significantly related to the high-risk group. Consequently, we also found that TMB was significantly higher in the high-risk group. We speculate that the immune-related lncRNA risk signature can identify patients with a highly active immune status and low TMB in LUAD and such patients have a better prognosis than other patients.
Extrapolation of the immune-related lncRNA risk signature from LUAD to other cancers may further confirm the role of immune-associated lncRNAs in tumour progression and prognosis. In this study, we investigated the applicability of our lncRNA risk signature model in PDAC samples and observed similar consistency as in LUAC samples in predicting prognosis, immune status, and TMB status in low- and high-risk groups. Our study is in agreement with the work of Ruess et al. (40), who collectively refer to some NSCLC and PDAC as mutant KRAS-driven tumours. In our study, KRAS was in the top ten mutated genes in both LUAD and PDAC samples and accounted for 25% and 77%, respectively. Further exploration of the relationship between KRAS and immune lncRNA is required. Taken together, we demonstrated that our immune-related lncRNA risk signature may be of prognostic value in certain types of tumour patients based on its ability to assess the immune status and TMB. Further studies are required to define this special type of tumours.
In conclusion, we constructed an immune-related lncRNA signature composed of 7 lncRNAs that indicated significant differences in immune microenvironment and somatic mutations in LUAD patients. This may play an important role in predicting the prognosis of patients and providing information related to immunotherapy effectiveness. We provided a new perspective on the role of lncRNA in the development and the molecular mechanism of immunity in LUAD. Through the preliminary exploration of the immune-related lncRNA risk signature in PDAC, we suggest the extension of the use of this risk signature to pancreatic cancer in the future. There are certain limitations in this study. Firstly, the results of the study were based on TCGA database, which lacked detailed information on resection extent, subtypes of LUAD, radiotherapy, and chemotherapy. Secondly, the 7 immune-associated lncRNAs have not been reported before and the mechanisms through which the prognostic immune-associated lncRNAs modulate the progression of LUAD require further investigation.
Acknowledgments
Funding: This work was supported by
Footnote
Reporting Checklist: The authors have completed the MDAR checklist. Available at http://dx.doi.org/10.21037/tcr-20-2827
Peer Review File: Available at http://dx.doi.org/10.21037/tcr-20-2827
Conflicts of Interest: All authors have completed the ICMJE uniform disclosure form (available at http://dx.doi.org/10.21037/tcr-20-2827). The authors have no conflicts of interest to declare.
Ethical Statement: The authors are accountable for all aspects of the work in ensuring that questions related to the accuracy or integrity of any part of the work are appropriately investigated and resolved. The study was conducted in accordance with the Declaration of Helsinki (as revised in 2013).
Open Access Statement: This is an Open Access article distributed in accordance with the Creative Commons Attribution-NonCommercial-NoDerivs 4.0 International License (CC BY-NC-ND 4.0), which permits the non-commercial replication and distribution of the article with the strict proviso that no changes or edits are made and the original work is properly cited (including links to both the formal publication through the relevant DOI and the license). See: https://creativecommons.org/licenses/by-nc-nd/4.0/.
References
- Bray F, Ferlay J, Soerjomataram I, et al. Global cancer statistics 2018: GLOBOCAN estimates of incidence and mortality worldwide for 36 cancers in 185 countries. CA Cancer J Clin 2018;68:394-424. [Crossref] [PubMed]
- Siegel RL, Miller KD, Jemal A. Cancer statistics, 2019. CA Cancer J Clin 2019;69:7-34. [Crossref] [PubMed]
- Nilssen Y, Strand TE, Fjellbirkeland L, et al. Lung cancer survival in Norway, 1997-2011: from nihilism to optimism. Eur Respir J 2016;47:275-87. [Crossref] [PubMed]
- Arbour KC, Riely GJ. Systemic Therapy for Locally Advanced and Metastatic Non-Small Cell Lung Cancer: A Review. JAMA 2019;322:764-74. [Crossref] [PubMed]
- Ettinger DS, Akerley W, Borghaei H, et al. Non-small cell lung cancer, version 2.2013. J Natl Compr Canc Netw 2013;11:645-53; quiz 53. [Crossref] [PubMed]
- Patel SP, Kurzrock R. PD-L1 Expression as a Predictive Biomarker in Cancer Immunotherapy. Mol Cancer Ther 2015;14:847-56. [Crossref] [PubMed]
- Sharma P, Allison JP. The future of immune checkpoint therapy. Science 2015;348:56-61. [Crossref] [PubMed]
- Acharya N, Sabatos-Peyton C, Anderson AC. Tim-3 finds its place in the cancer immunotherapy landscape. J Immunother Cancer 2020;8:e000911. [Crossref] [PubMed]
- Sharma P, Hu-Lieskovan S, Wargo JA, et al. Primary, Adaptive, and Acquired Resistance to Cancer Immunotherapy. Cell 2017;168:707-23. [Crossref] [PubMed]
- Lee SS, Cheah YK. The Interplay between MicroRNAs and Cellular Components of Tumour Microenvironment (TME) on Non-Small-Cell Lung Cancer (NSCLC) Progression. J Immunol Res 2019;2019:3046379. [Crossref] [PubMed]
- Öjlert ÅK, Halvorsen AR, Nebdal D, et al. The immune microenvironment in non-small cell lung cancer is predictive of prognosis after surgery. Mol Oncol 2019;13:1166-79. [Crossref] [PubMed]
- Mercer TR, Dinger ME, Mattick JS. Long non-coding RNAs: insights into functions. Nat Rev Genet 2009;10:155-9. [Crossref] [PubMed]
- Rinn JL, Chang HY. Genome regulation by long noncoding RNAs. Annu Rev Biochem 2012;81:145-66. [Crossref] [PubMed]
- Jiang MC, Ni JJ, Cui WY, et al. Emerging roles of lncRNA in cancer and therapeutic opportunities. Am J Cancer Res 2019;9:1354-66. [PubMed]
- Yu WD, Wang H, He QF, et al. Long noncoding RNAs in cancer-immunity cycle. J Cell Physiol 2018;233:6518-23. [Crossref] [PubMed]
- Hanahan D, Coussens LM. Accessories to the crime: functions of cells recruited to the tumor microenvironment. Cancer Cell 2012;21:309-22. [Crossref] [PubMed]
- Chen C, He W, Huang J, et al. LNMAT1 promotes lymphatic metastasis of bladder cancer via CCL2 dependent macrophage recruitment. Nat Commun 2018;9:3826. [Crossref] [PubMed]
- Pei X, Wang X, Li H. LncRNA SNHG1 regulates the differentiation of Treg cells and affects the immune escape of breast cancer via regulating miR-448/IDO. Int J Biol Macromol 2018;118:24-30. [Crossref] [PubMed]
- Lin T, Fu Y, Zhang X, et al. A seven-long noncoding RNA signature predicts overall survival for patients with early stage non-small cell lung cancer. Aging (Albany NY) 2018;10:2356-66. [Crossref] [PubMed]
- Miao R, Ge C, Zhang X, et al. Combined eight-long noncoding RNA signature: a new risk score predicting prognosis in elderly non-small cell lung cancer patients. Aging (Albany NY) 2019;11:467-79. [Crossref] [PubMed]
- Barbie DA, Tamayo P, Boehm JS, et al. Systematic RNA interference reveals that oncogenic KRAS-driven cancers require TBK1. Nature 2009;462:108-12. [Crossref] [PubMed]
- Hänzelmann S, Castelo R, Guinney J. GSVA: gene set variation analysis for microarray and RNA-seq data. BMC Bioinformatics 2013;14:7. [Crossref] [PubMed]
- He Y, Jiang Z, Chen C, et al. Classification of triple-negative breast cancers based on Immunogenomic profiling. J Exp Clin Cancer Res 2018;37:327. [Crossref] [PubMed]
- Lossos IS, Czerwinski DK, Alizadeh AA, et al. Prediction of survival in diffuse large-B-cell lymphoma based on the expression of six genes. N Engl J Med 2004;350:1828-37. [Crossref] [PubMed]
- Wei C, Liang Q, Li X, et al. Bioinformatics profiling utilized a nine immune-related long noncoding RNA signature as a prognostic target for pancreatic cancer. J Cell Biochem 2019;120:14916-27. [Crossref] [PubMed]
- Wang W, Zhao Z, Yang F, et al. An immune-related lncRNA signature for patients with anaplastic gliomas. J Neurooncol. 2018;136:263-71. [Crossref] [PubMed]
- Friedman J, Hastie T, Tibshirani R. Regularization Paths for Generalized Linear Models via Coordinate Descent. J Stat Softw 2010;33:1-22. [Crossref] [PubMed]
- Tu Z, He D, Deng X, et al. An eight-long non-coding RNA signature as a candidate prognostic biomarker for lung cancer. Oncol Rep 2016;36:215-22. [Crossref] [PubMed]
- Yoshihara K, Shahmoradgoli M, Martinez E, et al. Inferring tumour purity and stromal and immune cell admixture from expression data. Nat Commun 2013;4:2612. [Crossref] [PubMed]
- Cabrita R, Lauss M, Sanna A, et al. Tertiary lymphoid structures improve immunotherapy and survival in melanoma. Nature 2020;577:561-5. [Crossref] [PubMed]
- Dieu-Nosjean MC, Antoine M, Danel C, et al. Long-term survival for patients with non-small-cell lung cancer with intratumoral lymphoid structures. J Clin Oncol 2008;26:4410-7. [Crossref] [PubMed]
- Sautès-Fridman C, Petitprez F, Calderaro J, et al. Tertiary lymphoid structures in the era of cancer immunotherapy. Nat Rev Cancer 2019;19:307-25. [Crossref] [PubMed]
- Dieu-Nosjean MC, Goc J, Giraldo NA, et al. Tertiary lymphoid structures in cancer and beyond. Trends Immunol 2014;35:571-80. [Crossref] [PubMed]
- Xu F, Lin H, He P, et al. A TP53-associated gene signature for prediction of prognosis and therapeutic responses in lung squamous cell carcinoma. Oncoimmunology 2020;9:1731943. [Crossref] [PubMed]
- Li J, Zhang C, Zhang C, et al. Construction of immune-related and prognostic lncRNA clusters and identification of their immune and genomic alterations characteristics in lung adenocarcinoma samples. Aging (Albany NY) 2020;12:9868-81. [Crossref] [PubMed]
- Huang D, Chen J, Yang L, et al. NKILA lncRNA promotes tumor immune evasion by sensitizing T cells to activa-tion-induced cell death. Nat Immunol 2018;19:1112-25. [Crossref] [PubMed]
- Watson IR, Takahashi K, Futreal PA, et al. Emerging patterns of somatic mutations in cancer. Nat Rev Genet 2013;14:703-18. [Crossref] [PubMed]
- Gao Y, Li X, Zhi H, et al. Comprehensive Characterization of Somatic Mutations Impacting lncRNA Expression for Pan-Cancer. Mol Ther Nucleic Acids 2019;18:66-79. [Crossref] [PubMed]
- Kandoth C, McLellan MD, Vandin F, et al. Mutational landscape and significance across 12 major cancer types. Nature 2013;502:333-9. [Crossref] [PubMed]
- Ruess DA, Heynen GJ, Ciecielski KJ, et al. Mutant KRAS-driven cancers depend on PTPN11/SHP2 phosphatase. Nat Med 2018;24:954-60. [Crossref] [PubMed]