Identifying the potential regulators of neutrophils recruitment in hepatocellular carcinoma using bioinformatics method
Introduction
Currently, hepatocellular carcinoma (HCC) is the most frequent liver malignancy worldwide, and the morbidity and mortality rates are high (1). Within 5 years after curative resection, metastasis and recurrence of HCC are up to 70% (2). The tumor immune microenvironment plays a crucial role in initiation, progression, metastasis, and recurrence of HCC. As major components in the tumor immune microenvironment, neutrophils have been increasingly recognized as important factors in cancer behavior and progression (3). Recent evidence has demonstrated that neutrophils contribute to the metastatic process of tumor cells via cell-to-cell interactions (4). Evidence suggests that the neutrophil-to-lymphocyte ratio can predict the prognosis of HCC (5). Therefore, a deep understanding of the mechanisms leading to the interaction between neutrophils and cancer cells will provide better strategies to orchestrate the immune system to eradicate cancers.
Recently, a number of studies have focused on the roles of neutrophils in the tumor microenvironment. Neutrophils have been confirmed to induce the stem cell characteristics in HCC cells by secreting BMP2 and TGFB2 (6). Xiao et al. (7) reported that neurotensin increases the neutrophil count of the local HCC microenvironment by inducing the expression of IL-8. Haider et al. (8) concluded that the CXCL5-dependent attraction of neutrophils depends on TGF-β and Axl signaling. Despite substantial progress in research involving the roles of neutrophils in the tumor microenvironment, the precise molecular mechanisms underlying neutrophil recruitment are only partially understood.
In this study we showed that the transcription factor, EHF, and its target, FGD6, had significantly different levels of expression in patients with high- and low-level neutrophil counts. The complete nomenclature of transcription factor EHF is larger E26 transformation-specific (ETS) homologous factor. Increasing evidence over recent years has revealed that EHF has a critical function in tumorigenesis. It has been demonstrated that EHF activates the TGF-β signaling pathway in colorectal carcinoma cells (9). Increased expression of EHF leads to poor survival in gastric cancer by activating HER family signaling (10). Liu et al. (11) reported that the level of EHF expression has a close relationship with the efficacy of anti-PD-1 therapy in pancreatic ductal adenocarcinoma. Our outcome showed that the expression level of FGD6 had significant correlation with the expression level of RIC8B and SIPA1L3. Previous evidence demonstrates that FGD6 can interact with CDC42 which contributes to cell adhesion, cell polarity, and membrane recycling during bone degradation (12). Taken together, our data suggest that the transcription factor, EHF, influenced recruitment of neutrophils by mediating the transcription of FGD6. EHF-FGD6-CDC42 plays an important role in neutrophil recruitment.
We present the following article in accordance with the MDAR reporting checklist (available at http://dx.doi.org/10.21037/tcr-20-2714).
Methods
HCC data sets downloading and preprocessing
RNA-seq data and clinical data of HCC patient samples were extracted from the National Cancer Institute Genomic Data Commons (https://gdc.cancer.gov/about-data/publications/pancanatlas). We performed log2 normalization for RNA-seq data. The study was conducted in accordance with the Declaration of Helsinki (as revised in 2013).
Assess and analyze the infiltration of immune cells in HCC
Infiltrations of immune cells in HCC were assessed using the CIBERSORT algorithm (13) with default parameters. Next, we carried out Kaplan-Meier survival analysis to screen the immune cells that were significantly correlated with patient survival. Patients were divided into high and low neutrophil groups based on the median neutrophil count. Kaplan-Meier survival analysis was implemented for the neutrophil fraction using the survival package in R (14). The P values for the Kaplan-Meier survival analysis were adjusted using the false discovery rate (FDR) method. We screened the different expression genes (DEGs) in the two groups utilizing the limma package in R (15) [filter criteria: fold change (FC) ≥1.5; adjusted P value <0.01]. The P values were further adjusted using the Benjamini-Hochberg (BH) multiple testing correction method.
Construct prognosis prediction model using least absolute shrinkage and selection operator (LASSO) regression
In this step, we constructed a prognostic predictive module using LASSO regression. We screened survival-related genes using univariate Cox proportional risk regression model in survival R package (14). P<0.001 was selected as the threshold. We screened the most powerful prognostic markers from the survival-related genes using LASSO regression in the glmnet R package (16). The workflow of the prognostic predictive module was as follows: (I) patients were randomly divided into training (70% patients) and test sets (30% patients); (II) the training set was trained on the prognostic model and the performance of the model on the test set was assessed; (III) and the concordance index (c index) of the prognostic model in the training and test sets were calculated utilizing the Hmisc R package (17). We used a univariate Cox proportional risk regression model for age, gender, stage, stage T, stage N, stage M, cigarette smoking, and subtypes. The survival-related clinical features were selected for multivariate Cox proportional risk regression for risk scores and abundance of immune cells.
Identifying transcription factor and the targets
The detailed information of human transcription factor was downloaded from Ensembl (18). We screened all transcription factors from DEGs based on information about transcription factors downloaded from Ensembl. Next, we selected the transcription factor with the maximum logFC for further analysis. The ChIP-seq outcome of transcription factor was downloaded from Cistrome Data Browser (19). Yan et al. (20) contributed to the ChIP-seq outcome. We selected the genes which were at the intersection of the ChIP-seq outcome and DEGs as the target of transcription factor for further analysis. We uploaded genes we selected to DAVID (21) and assessed the gene ontology (GO) functions and Kyoto Encyclopedia of Genes and Genomes (KEGG) pathways. Next, we calculated the correlation between the transcription factor and the differentially-expressed targets. The target that had the greatest correlation was selected for further analysis.
Function analysis of transcription factor targets
We selected the target that had the greatest correlation with the transcription factor. Then, we submitted the target to DAVID and obtained the GO functions and KEGG pathways. The GSVA package in R (22) was used to calculate the GO function and KEGG pathway scores in all patients. The limma package in R to screen GO functions and KEGG pathways were shown to be different between the high and low neutrophil group (filter criteria: log2FC ≥0.1; adjusted P value <0.01). To further evaluate the possible mechanisms underlying the target with the greatest correlation, we calculated the correlation between the target with the greatest correlation and the screened genes with different GO functions and KEGG pathways. The functions and pathways of significantly correlated genes were annotated by DAVID.
Statistical analysis
Wilcoxon test was utilized to compare continuous variables. Correlations were calculated with Spearman correlation. All the tests were two-sided, and P<0.05 was regarded as indicating significance, unless otherwise stated. Survival analysis was performed using the Kaplan-Meier method, and the survival of the clusters was compared using the log-rank test. The FDR correction was utilized in multiple tests to decrease false positive rates. All of the analyses were performed with R software (version 3.4.2, http://www.R-project.org).
Results
HCC data sets downloading and pre-processing
RNA-seq data and clinical data of 323 HCC patients were extracted from the National Cancer Institute Genomic Data Commons (https://gdc.cancer.gov/about-data/publications/pancanatlas). We performed log2 normalization for RNA-seq data.
Assessment and analysis of immune cell infiltration in HCC
Utilizing the CIBERSORT algorithm, we assessed infiltration of 22 immune cells in HCC (Figure 1A). Next, we divided patients into high and low immune cell groups based on the median of each immune cell count. We carried out Kaplan-Meier survival analysis for 22 immune cells. The P values of Kaplan-Meier survival analysis were adjusted using the FDR method (Table 1). Our outcome showed that neutrophil infiltration was significantly associated with patient survival (Figure 1B). Next, we screened DEGs between the high (n=147) and low neutrophil groups (n=206) using the limma R package. A total of 736 DEGs were screened between the high and low neutrophil groups (Figure 2). Five hundred sixty-three DEGs had significantly high expression, whereas 173 DEGs were downregulated in the high neutrophil group.
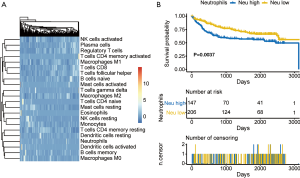
Table 1
Immune cells | FDR |
---|---|
T cells (CD4 memory activated) | 0.988706859 |
T cells (CD4 naïve) | 0.952450144 |
NK cells (resting) | 0.952450144 |
T cells (follicular helper) | 0.94049182 |
Eosinophils | 0.915125939 |
Dendritic cells (resting) | 0.915125939 |
T cells (CD8) | 0.915125939 |
B cells (memory) | 0.748294157 |
Mast cells (activated) | 0.748294157 |
Macrophages (M1) | 0.748294157 |
Mast cells (resting) | 0.664405339 |
Plasma cells | 0.576581743 |
Dendritic cells (activated) | 0.576581743 |
T cells regulatory (Tregs) | 0.576581743 |
T cells (gamma delta) | 0.576581743 |
Monocytes | 0.525440109 |
NK cells (activated) | 0.525440109 |
T cells (CD4 memory resting) | 0.12998342 |
B cells (naïve) | 0.12998342 |
Macrophages (M2) | 0.042122642 |
Macrophages (M0) | 0.042122642 |
Neutrophils | 0.042122642 |
P values were adjusted using FDR method. FDR, false discovery rate.
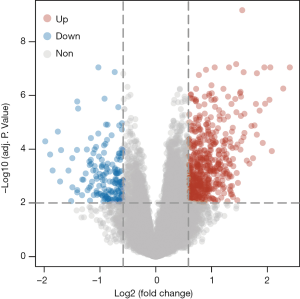
Construction of a prognosis predictive model using LASSO regression
A total of 323 patients were selected to fit the prognosis predictive model. We screened 1,616 survival-related genes using a univariate Cox proportional risk regression model with a P<0.001 threshold. Next, we performed LASSO regression to screen survival-related markers. A total of three survival-related markers were screened. The risk score for each patient was calculated by combining the expression of the prognosis-related markers with the corresponding LASSO coefficients. The formula of the risk score is listed as follows:
To assess the performance of our model, we plotted cross-validated time-dependent receiver operating characteristic (ROC) curves. The 12-month area under the curve (AUC) was 0.823 (Figure 3A). The 36- and 60-month AUCs were both >0.79. The AUCs indicated that our model had good performance in prognosis prediction both in the short- and long-term. We calculated the optimal cut-off value according to the optimum sensitivity and specificity of the 5-year survival ROC curve. Patients with a risk score ≥−0.027 were assigned to the high-risk group, and the remaining patients comprised the low-risk group. The survival of patients in the low-risk group was significantly better than patients in the high-risk group (Figure 3B). The number of patients in high risk group was less than the number of patients in low risk group (Figure 4A). The high-risk group had more deaths than the low-risk group (Figure 4B). As presented in Figure 4C, RAP1GAP and SLC39A1 in patients in the high-risk group exhibited high expression levels while ZNF23 showed the opposite tendency. Finally, we carried out multivariate Cox regression analysis and the outcome revealed that our prognosis predictive model independently predicted prognosis (Table 2).
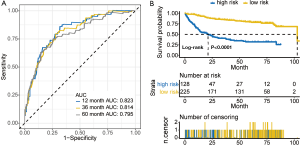
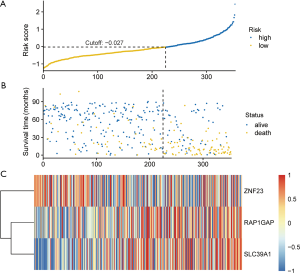
Table 2
Variables | Univariate | Multivariate | |||
---|---|---|---|---|---|
HR (95% CI) | P value | HR (95% CI) | P value | ||
Age (years) | 1.01 (1.00–1.03) | 0.1270 | Not included | ||
Gender | |||||
Male | Ref. | Ref. | |||
Female | 1.48 (1.00–2.19) | 0.0496 | 1.38 (0.92–2.06) | 0.1167 | |
Stage | |||||
Stage 1 | Ref. | Ref. | |||
Stage 2 | 1.27 (0.77–2.09) | 0.3540 | 0.88 (0.53–1.46) | 0.6170 | |
Stage 3 | 2.55 (1.64–3.97) | 2.98×10−5 | 1.78 (1.13–2.81) | 0.0124 | |
Stage 4 | 4.49 (1.38–14.61) | 0.0124 | 3.45 (1.04–11.43) | 0.0426 | |
Risk score | 4.38 (3.25–5.90) | 3.17×10−22 | 4.24 (3.11–5.77) | 5.46×10−20 |
HRs and P values of risk score and the covariates in the univariate and multivariate Cox proportional hazards model. Risk score can predict prognosis independently. HCC, hepatocellular carcinoma; HR, hazard ratio; CI, confidence interval.
Identifying the transcription factor and the targets
The information of human transcription factor was downloaded from Ensembl. Through comparison, we identified 18 transcription factors in DEGs (Figure 5A). Transcription factor EHF with maximum logFC (logFC =1.1717) was selected for further analysis (Figure 5B). We downloaded the ChIP-seq outcome of EHF from Cistrome Data Browser. Based on the ChIP-seq outcome, we screened 702 targets of transcription factor EHF in DEGs. Among these targets, the expression level of FGD6 was most correlated with transcription factor EHF (Figure 5C). Next, we submitted the targets of EHF to DAVID and carried out pathways and GO function analysis. The outcome revealed that the targets of EHF mainly enriched in GO:0016021 integral component of membrane, GO:0006954 inflammatory response, GO:0005887 integral component of plasma membrane, GO:0005886 plasma membrane and GO:0005615 extracellular space (Figure 6).
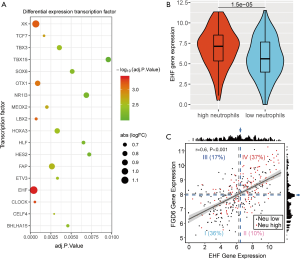
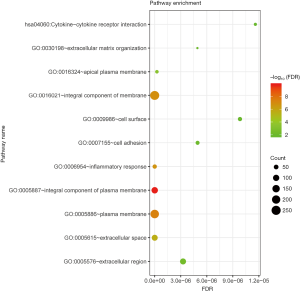
Function analysis of transcription factor targets
We submitted FGD6 to DAVID and obtained 16 GO function terms and zero KEGG pathways. GSVA and limma analysis demonstrated that the high and low neutrophil groups had significant differences in 2 GO terms [GO:0043547 (positive regulation of GTPase activity) and GO:0007010 (cytoskeleton organization); Figure 7]. To further evaluate the possible mechanisms underlying FGD6 in GO:0043547 and GO:0007010, we calculated the correlation between FGD6 and genes in the two GO terms. The outcome showed that the RIC8B and SIPA1L3 genes had the greatest correlation (Figure 8). The KEGG pathways analysis utilizing DAVID showed that SIPA1L3 was involved in the hsa04015: Rap1 signaling pathway.
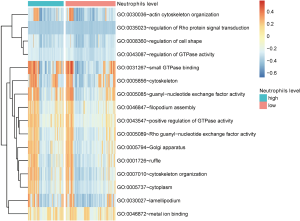
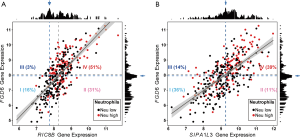
Discussion
Our findings demonstrated that the neutrophil count was significantly correlated with patient survival. The transcription factor, EHF, and its target, FGD6, had significantly different levels of expression between patients with high and low neutrophil counts. GO:0043547 exerts positive regulation of GTPase activity and GO:0007010 facilitates cytoskeleton organization; FGD6 participates in both functions and there were significant differences in patients with high and low neutrophil counts. Correlation analysis showed that FGD6 had the greatest correlation with RIC8B and SIPA1L3 in the positive regulation of GTPase activity and cytoskeleton organization. SIPA1L3 is a member of the hsa04015: Rap1 signaling pathway.
EHF is a member of the highly diverse ETS superfamily. Accumulation evidence over recent years has demonstrated that EHF has a great effect on tumorigenesis and the tumor microarray environment. Studies have revealed that EHF inhibits tumor invasion, metastasis, and the mesenchymal phenotype (23-25). Liu et al. (11) reported that deficiency of EHF induces T reg cell and myeloid derived suppressor cell (MDSC) accumulation and causes a decrease in CD8+ T cell infiltration. Wang et al. (9) found that EHF promotes colorectal carcinoma progression via activation pf TGF-β1 transcription and canonical TGF-β signaling. Liu et al. (11) revealed that overexpression of EHF can significantly lead to better response to anti-PD-1 therapy. High expression of EHF promotes oral squamous cell carcinoma metastasis, cancer stemness, and drug resistance (26). Taken together, EHF has emerged as a promising target in cancer research; however, the contributions of EHF to neutrophils in HCC are not fully understood. We found that the level of EHF expression was different between patients with high and low neutrophil counts. Our finding suggested that EHF plays an important role in neutrophil recruitment.
To understand the mechanism underlying EHF, we screened its downstream target, FGD6, based on the outcome of ChIP-seq. FGD6 is a member of the faciogenital dysplasia family. FGD6 includes six Rho guanine nucleotide exchange factors (GEFs) that activate Rho proteins (12). FGD6, which is highly homologous to FGD1, has two pleckstrin homology (PH) domains and one Dbl homology (DH) domain. Previous studies have shown that mutations in the FGD1 gene are linked to the inherited disease, faciogenital dysplasia, and Aarskog-Scott syndrome (27,28). Recent evidence demonstrated that FGD6 plays a crucial role in cell polarity and membrane recycling by regulating the assembly of different actin-based protein networks and activating CDC42 at different locations (12). Our data showed that FGD6 is involved in GO:0043547 positive regulation of GTPase activity and GO:0007010 cytoskeleton organization. In addition, the level of FGD6 expression was highly correlated with the level of RIC8B and SIPA1L3 expression in GO:0043547 positive regulation of GTPase activity and GO:0007010 cytoskeleton organization. This finding indicated that FGD6, RIC8B, and SIPA1L3 may work together in the positive regulation of GTPase activity and cytoskeleton organization.
SIPA1L3, which has N-terminal RapGAP (a Rap-GTPase activating domain), PDZ, and C-terminal leucine zipper domains, is a member of the signal-induced proliferation-associated (SIPA) proteins. A previous study revealed that loss of SIPA1L3 interferes with cell polarity and cytoskeletal organization (29). Previous evidence showed that RIC8B is GEFs for the α subunits of heterotrimeric guanine nucleotide-binding proteins (30). In addition, it has been suggested that RIC8B contributes to the steady state of G protein α subunits (31). Some studies have showed that overexpression of RIC8B enhances activation of adenylyl cyclase (AC) via Gs and Golf (32,33). We discovered that the level of FGD6 expression had a high correlation with the level of RIC8B and SIPA1L3 expression. Therefore, it is essential to explore the relationship and potential mechanisms underlying FGD6, RIC8B, and SIPA1L3.
Our data showed that SIPA1L3 participates in the has04015: Rap1 signaling pathway. Accumulating evidence demonstrates that the Rap1 signaling pathway contributes a major role in the endothelial barrier regulation, vasculogenesis, and angiogenesis (34,35). A recent study involving the Rap1 signaling pathway has focused on integrin-mediated cell adhesion (36). Based on the diagram of the has04015: Rap1 signaling pathway on the KEGG website (https://www.genome.jp/kegg-bin/show_pathway?map=hsa04015&show_description=show) (Figure 9), we found that the G protein-coupled receptors (GPCRs) signaling pathway activates the Rap1 signaling pathway via EPAC. EPAC, which includes GEFs, can exchange bound GDP of Rap1 for free GTP. In contrast, SIPA1L3, which contains the RapGAP domain, can activate the Rap-GTPase inhibiting Rap1 signaling pathway. Active Rap1 can activate CDC42 via Src and FRG. CDC42 will activate the process of cell adhesion, migration, and polarity. Collectively, these processes can be characterized in three sections: (I) the process of GPCRs regulating Rap1; (II) the process of regulation between Rap1-GDP and Rap1-GTP; and (III) the regulation of CDC42 in Rap1 downstream. We showed that FGD6, RIC8B, and SIPA1L3 are crucial regulatory factors in these three sections. RIC8B enhances activation of AC via Gs. This process will trigger EPAC to promote Rap1-GDP conversion into Rap1-GTP, which will activate the Rap1 signaling pathway. SIPA1L3, which has an N-terminal RapGAP domain, promotes the transformation of Rap1-GTP to Rap1-GDP. This process inhibits the Rap1 signaling pathway. FGD6, which is regulated by the transcription factor, EHF, can activate CDC42. This process will positively regulate the Rap1 signaling pathway and contribute to cell adhesion, migration, and polarity.
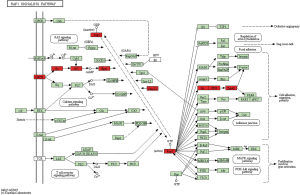
We inferred that the transcription factor, EHF, mediates GO:0043547 positive regulation of GTPase activity, GO:0007010 cytoskeleton organization, and the has04015: Rap1 signaling pathway by regulating the transcription of FGD6. These processes will mediate local adhesion. Increasing local adhesion may contribute to the recruitment and persistence of neutrophils in tumor lesions. Also, tumor cells might be protected and transported by the surrounding neutrophils following cell-cell interactions (3). As the key step in tumor metastasis, increasing adhesion will raise the ability of metastasis and colonization of tumor cells. This feature can contribute to the attachment of tumor cells to matrix collagen fibers of vascular endothelial cells and secondary lesions (37). These reasons suggest that patients with a high neutrophil count had worse survival. Increased EHF upregulates FGD6. Increased FGD6 and RIC8B or decreased SIPA1L3 will contribute to activation of the Rap1 signaling pathway. Activation of the Rap1 signaling pathway will increase cell adhesion, which is advantageous to neutrophil recruitment and metastasis, and colonization of tumor cells.
Conclusions
These findings demonstrated that transcription factor EHF can influence recruitment of neutrophils by mediating the transcription of FGD6. Further investigations are needed to shed new light on EHF and its target FGD6 which may contribute to immunotherapy in HCC.
Acknowledgments
We thank all of the subjects enrolled in this study.
Funding: None.
Footnote
Reporting Checklist: The authors have completed the MDAR reporting checklist. Available at http://dx.doi.org/10.21037/tcr-20-2714
Conflicts of Interest: All authors have completed the ICMJE uniform disclosure form (available at http://dx.doi.org/10.21037/tcr-20-2714). The authors have no conflicts of interest to declare.
Ethical Statement: The authors are accountable for all aspects of the work in ensuring that questions related to the accuracy or integrity of any part of the work are appropriately investigated and resolved. The study was conducted in accordance with the Declaration of Helsinki (as revised in 2013).
Open Access Statement: This is an Open Access article distributed in accordance with the Creative Commons Attribution-NonCommercial-NoDerivs 4.0 International License (CC BY-NC-ND 4.0), which permits the non-commercial replication and distribution of the article with the strict proviso that no changes or edits are made and the original work is properly cited (including links to both the formal publication through the relevant DOI and the license). See: https://creativecommons.org/licenses/by-nc-nd/4.0/.
References
- Bray F, Ferlay J, Soerjomataram I, et al. Global cancer statistics 2018: GLOBOCAN estimates of incidence and mortality worldwide for 36 cancers in 185 countries. CA Cancer J Clin 2018;68:394-424. [Crossref] [PubMed]
- Llovet JM, Zucman-Rossi J, Pikarsky E, et al. Hepatocellular carcinoma. Nat Rev Dis Primers 2016;2:16018. [Crossref] [PubMed]
- Mollinedo F. Neutrophil Degranulation, Plasticity, and Cancer Metastasis. Trends Immunol 2019;40:228-42. [Crossref] [PubMed]
- Zhang W, Gu J, Chen J, et al. Interaction with neutrophils promotes gastric cancer cell migration and invasion by inducing epithelial-mesenchymal transition. Oncol Rep 2017;38:2959-66. [Crossref] [PubMed]
- Hong YM, Cho M, Yoon KT, et al. Neutrophil-lymphocyte ratio predicts the therapeutic benefit of neoadjuvant transarterial chemoembolization in patients with resectable hepatocellular carcinoma. Eur J Gastroenterol Hepatol 2020;32:1186-91. [Crossref] [PubMed]
- Zhou SL, Yin D, Hu ZQ, et al. A Positive Feedback Loop Between Cancer Stem-Like Cells and Tumor-Associated Neutrophils Controls Hepatocellular Carcinoma Progression. Hepatology 2019;70:1214-30. [Crossref] [PubMed]
- Xiao P, Long X, Zhang L, et al. Neurotensin/IL-8 pathway orchestrates local inflammatory response and tumor invasion by inducing M2 polarization of Tumor-Associated macrophages and epithelial-mesenchymal transition of hepatocellular carcinoma cells. Oncoimmunology 2018;7:e1440166. [Crossref] [PubMed]
- Haider C, Hnat J, Wagner R, et al. Transforming Growth Factor-beta and Axl Induce CXCL5 and Neutrophil Re-cruitment in Hepatocellular Carcinoma. Hepatology 2019;69:222-36. [Crossref] [PubMed]
- Wang L, Ai M, Nie M, et al. EHF promotes colorectal carcinoma progression by activating TGF-β1 transcription and canonical TGF-β signaling. Cancer Sci 2020;111:2310-24. [Crossref] [PubMed]
- Shi J, Qu Y, Li X, et al. Increased expression of EHF via gene amplification contributes to the activation of HER family signaling and associates with poor survival in gastric cancer. Cell Death Dis 2016;7:e2442. [Crossref] [PubMed]
- Liu J, Jiang W, Zhao K, et al. Tumoral EHF predicts the efficacy of anti-PD1 therapy in pancreatic ductal adenocarcinoma. J Exp Med 2019;216:656-73. [Crossref] [PubMed]
- Steenblock C, Heckel T, Czupalla C, et al. The Cdc42 guanine nucleotide exchange factor FGD6 coordinates cell polarity and endosomal membrane recycling in osteoclasts. J Biol Chem 2014;289:18347-59. [Crossref] [PubMed]
- Newman AM, Liu CL, Green MR, et al. Robust enumeration of cell subsets from tissue expression profiles. Nat Methods 2015;12:453-7. [Crossref] [PubMed]
- Therneau TM, Grambsch PM. Modeling Survival Data: Extending the Cox Model. New York: Springer-Verlag, 2013.
- Ritchie ME, Phipson B, Wu D, et al. limma powers differential expression analyses for RNA-sequencing and microarray studies. Nucleic Acids Res 2015;43:e47. [Crossref] [PubMed]
- Simon N, Friedman J, Hastie T, et al. Regularization Paths for Cox's Proportional Hazards Model via Coordinate Descent. J Stat Softw 2011;39:1-13. [Crossref] [PubMed]
- Harrell FE Jr, with contributions from Charles Dupont and many others. (2020). Hmisc: Harrell Miscellaneous. R package version 4.4-0. Available online: https://CRAN.R-project.org/package=Hmisc
- Zerbino DR, Johnson N, Juetteman T, et al. Ensembl regulation resources. Database (Oxford) 2016;2016:bav119. [Crossref] [PubMed]
- Zheng R, Wan C, Mei S, et al. Cistrome Data Browser: expanded datasets and new tools for gene regulatory analysis. Nucleic Acids Res 2019;47:D729-35. [Crossref] [PubMed]
- Yan J, Enge M, Whitington T, et al. Transcription factor binding in human cells occurs in dense clusters formed around cohesin anchor sites. Cell 2013;154:801-13. [Crossref] [PubMed]
- Huang da W. Sherman BT, Lempicki RA. Systematic and integrative analysis of large gene lists using DAVID bioinformatics resources. Nat Protoc 2009;4:44-57. [Crossref] [PubMed]
- Hänzelmann S, Castelo R, Guinney J. GSVA: gene set variation analysis for microarray and RNA-Seq data. BMC Bioinformatics 2013;14:7. [Crossref] [PubMed]
- Albino D, Civenni G, Dallavalle C, et al. Activation of the Lin28/let-7 Axis by Loss of ESE3/EHF Promotes a Tumorigenic and Stem-like Phenotype in Prostate Cancer. Cancer Res 2016;76:3629-43. [Crossref] [PubMed]
- Albino D, Civenni G, Rossi S, et al. The ETS factor ESE3/EHF represses IL-6 preventing STAT3 activation and expansion of the prostate cancer stem-like compartment. Oncotarget 2016;7:76756-68. [Crossref] [PubMed]
- Zhao T, Jiang W, Wang X, et al. ESE3 Inhibits Pancreatic Cancer Metastasis by Upregulating E-Cadherin. Cancer Res 2017;77:874-85. [Crossref] [PubMed]
- Huang WC, Jang TH, Tung SL, et al. A novel miR-365-3p/EHF/keratin 16 axis promotes oral squamous cell carcinoma metastasis, cancer stemness and drug resistance via enhancing β5-integrin/c-met signaling pathway. J Exp Clin Cancer Res 2019;38:89. [Crossref] [PubMed]
- Estrada L, Caron E, Gorski JL. Fgd1, the Cdc42 guanine nucleotide exchange factor responsible for faciogenital dysplasia, is localized to the subcortical actin cytoskeleton and Golgi membrane. Hum Mol Genet 2001;10:485-95. [Crossref] [PubMed]
- Orrico A, Galli L, Cavaliere ML, et al. Phenotypic and molecular characterisation of the Aarskog-Scott syndrome: a survey of the clinical variability in light of FGD1 mutation analysis in 46 patients. Eur J Hum Genet 2004;12:16-23. [Crossref] [PubMed]
- Greenlees R, Mihelec M, Yousoof S, et al. Mutations in SIPA1L3 cause eye defects through disruption of cell polarity and cytoskeleton organization. Hum Mol Genet 2015;24:5789-804. [Crossref] [PubMed]
- Chan P, Gabay M, Wright FA, et al. Ric-8B is a GTP-dependent G protein alphas guanine nucleotide exchange factor. J Biol Chem 2011;286:19932-42. [Crossref] [PubMed]
- Gabay M, Pinter ME, Wright FA, et al. Ric-8 proteins are molecular chaperones that direct nascent G protein alpha subunit membrane association. Sci Signal 2011;4:ra79. [Crossref] [PubMed]
- Kerr DS, Von Dannecker LE, Davalos M, et al. Ric-8B interacts with G alpha olf and G gamma 13 and co-localizes with G alpha olf, G beta 1 and G gamma 13 in the cilia of olfactory sensory neurons. Mol Cell Neurosci 2008;38:341-8. [Crossref] [PubMed]
- Von Dannecker LE, Mercadante AF, Malnic B. Ric-8B, an olfactory putative GTP exchange factor, amplifies signal transduction through the olfactory-specific G-protein Galphaolf. J Neurosci 2005;25:3793-800. [Crossref] [PubMed]
- Chrzanowska-Wodnicka M, White GC 2nd, Quilliam LA, et al. Small GTPase Rap1 Is Essential for Mouse Development and Formation of Functional Vasculature. PLoS One 2015;10:e0145689. [Crossref] [PubMed]
- Chrzanowska-Wodnicka M, Kraus AE, Gale D, et al. Defective angiogenesis, endothelial migration, proliferation, and MAPK signaling in Rap1b-deficient mice. Blood 2008;111:2647-56. [Crossref] [PubMed]
- Bos JL, de Bruyn K, Enserink J, et al. The role of Rap1 in integrin-mediated cell adhesion. Biochem Soc Trans 2003;31:83-6. [Crossref] [PubMed]
- Benoliel AM, Pirro N, Marin V, et al. Correlation between invasiveness of colorectal tumor cells and adhesive potential under flow. Anticancer Res 2003;23:4891-6. [PubMed]