Identification of key genes and pathways at the downstream of S100PBP in pancreatic cancer cells by integrated bioinformatical analysis
Introduction
Pancreatic cancer is a highly malignant tumor and the seventh leading cause of cancer-related mortality worldwide (1). Due to the difficulty in detecting aggressive biologic properties and early signs of pancreatic cancer, most patients are at a locally advanced or metastatic stage when they are first diagnosed; consequently, the 5-year survival rate stands at less than 7% (2,3). Despite the effort that has been invested into their development, treatment modalities are still insufficient. Therefore, it is important to investigate the key molecules in pancreatic cancer progression and to identify potential biomarkers for early diagnosis and therapeutic strategies.
S100PBP, located at 1p34.3, encodes a protein that is a binding partner of S100P and shares no sequence or structural similarity with any other protein. S100PBP is a widely expressed protein detected in normal brain, breast, spleen, and lung tissue, whereas its expression is decreased in pancreatic cancer (4). Studies have reported that S100PBP can inhibit the adhesion of pancreatic cancer cells through the S100PBP/CTSZ/RGD αvβ5 pathway (5).
In recent years, high-throughput platforms, such as microarrays, have been widely used in the analysis of gene expression and genetic alteration in tumorigenesis (6). Bioinformatics methods for processing and analyzing a great amount of data generated by microarray technology have been used to explore key genes and potential molecular mechanisms for the diagnosis and treatment of pancreatic cancer. Given the function of S100PBP in pancreatic cancer, the microarray datasets GSE35196 (S100PBP knockdown) and GSE35198 (S100PBP overexpression) in the present study were downloaded from the Gene Expression Omnibus (GEO) database, and the differentially expressed genes (DEGs) were analyzed. Functional enrichment and protein-protein interaction (PPI) network analyses were also applied in order to identify the key pathways that the DEGs are involved in. To further explore the impact of node genes on pancreatic cancer, overall survival (OS) analysis and gene expression analysis were also employed.
We present the following study in accordance with the MDAR checklist (available at http://dx.doi.org/10.21037/tcr-20-2531).
Methods
Microarray data
The GEO (http://www.ncbi.nlm.nih.gov/geo) is a public storage library from which microarray, next-generation sequencing, and other high-throughput data are freely available. The SuperSeries GSE35199, which comprises GSE35196 and GSE35198, was based on the GPL570 platform and downloaded from the GEO database. GSE35196 includes array data on S100PBP knockdown for 2 control samples and 3 S100PBP siRNA transfection samples. GSE35198 includes array data on S100PBP overexpression for 3 control samples and 3 samples with S100PBP overexpression.
Collection and inclusion criteria of studies
We searched the GEO database using the following keywords: “pancreatic cancer” (study keyword), “S100PBP” (study keyword), “Homo sapiens” (organism), and “series” (entry type). The search returned 3 items. The inclusion criteria for studies were as follows: (I) samples needed to be in 2 groups, including the control group and the experimental group; (II) the sample count needed to be appropriate; (III) S100PBP expression in the experimental group needed to be either knockdown or overexpression; and (IV) sufficient information was needed to perform the analysis. Accordingly, SuperSeries GSE35199, which is composed of GSE35196 and GSE35198, was used for the analysis.
Data processing
A large amount of high-throughput functional genomic data are stored in the GEO database for free research and are normalized using various methods or tools. Limma package was used to identify the different expression of genes by linear modelling. P<0.05 and fold change >1.5, or <0.67 were set as the cut-off criteria. R software package, a language tool for statistical calculations and drawing that provides a wide variety of statistical and graphical techniques, was used to obtain the heatmaps of DEGs, and P<0.05 was set as the cut-off criterion.
Functional and pathway enrichment analysis
The Database for Annotation, Visualization and Integrated Discovery (DAVID, http://www.david.niaid.nih.gov) is a database that is used for annotation, visualization, and integrated discovery (7). FunRich is a software tool that is mainly used for gene and protein functional enrichment and interaction network analyses. Gene Ontology (GO) enrichment analysis of identified DEGs was annotated and visualized using DAVID and FunRich, with P<0.05 set as the cut-off criterion. Kyoto Encyclopedia of Genes and Genomes (KEGG, http://www.genome.jp/kegg/pathway.html) is an integrated database resource used for the biologic interpretation of genome sequences and other high-throughput data (8). The KEGG Orthology-Based Annotation System (KOBAS, http://kobas.cbi.pku.edu.cn/annotate.php) is a web server for gene/protein functional annotation and functional gene set enrichment. In the present study, we conducted KOBAS and KEGG pathway enrichment analyses.
PPI network construction and analysis of modules
The Search Tool for the Retrieval of Interacting Genes (STRING, http://string-db.org/) database is an online tool that collects and integrates information on all functional interactions between expressed proteins through consolidating known and predicted protein–protein association data for organisms (9). A minimum required interaction score of >0.15 was set as the cut-off criterion. Cytoscape is a popular bioinformatics package that visualizes the biologic network and integrates data (10). DEGs were mapped to STRING to evaluate the PPI information and were visualized using Cytoscape.
Survival analysis of DEGs
To analyze the prognosis of identified DEGs, the expression datasets were downloaded from The Cancer Genome Atlas (https://tcga-data.nci.nih.gov/tcga). The OS analysis was carried out using R software package, and results were determined by Kaplan-Meier curves, on which following the P value was shown. Gene Expression Profiling Interactive Analysis (GEPIA, http://gepia.cancer-pku.cn/) is a database that can comparatively analyze gene expression in tumor and normal tissues (11). We displayed the expression of key genes of pancreatic cancer tissues and adjacent tissues, using P<0.05 as the critical criterion.
Statistical analysis
Survival analysis of the key genes was performed by the Survival package in the R software. Survival plots were showed by the Kaplan-Meier method, and the significance was estimated by the log-rank test. P value <0.05 was considered as statistically significant.
Ethical statement
The study was conducted in accordance with the Declaration of Helsinki (as revised in 2013). All information from GEO is available and free for public, so the agreement of the medical ethics committee board was not necessary.
Results
Identification of DEGs
From GSE35196 and GSE35198, 606 DEGs (316 upregulated and 290 downregulated) and 961 DEGs (451 upregulated and 510 downregulated) were identified, respectively. Then, we took the intersection of the downregulated DEGs from GSE35196 and the upregulated DEGs from GSE35198, and found 11 upregulated genes (Figure 1A and Table 1). A further 23 downregulated genes were found by taking the intersection of the upregulated DEGs from GSE35196 and the downregulated DEGs from GSE35198 (Figure 1B). In total, 34 DEGs (11 upregulated and 23 downregulated genes) were screened out. By using R package, heatmaps of the DEGs were generated (Figure 1C,D).
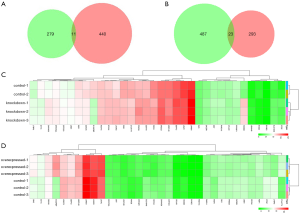
Table 1
DEGs | Genes name |
---|---|
Up-regulated | S100PBP, DDX3X, STYX, PPFIBP1, LIN7C, PSPH, C1orf174, RHOB, GNS, MRPL30, RSF1 |
Down-regulated | HOXB8, RNF213, DST, CFLAR, USB1, SYNE2, LAMA5, GSTA4, SFXN4, LSM14B, SPATS2L, LTBP2, DICER1, GTF3C3, LMNB1, PRKRA, CTSZ, SLC16A6, GLS, SEPT2, RAPH1, XRCC5, ZMYND8 |
Functional and pathway enrichment analysis
To obtain further insight into the function of the identified DEGs, functional enrichment analysis was performed using DAVID and FunRich. The GO enrichment analysis showed that in the biologic process-associated category, DEGs were significantly enriched in the production of siRNA involved in RNA interference, the production of miRNAs involved in gene silencing by miRNA, and pre-miRNA processing. Cell component analysis showed the DEGs to be enriched in the nucleus, nuclear envelope, and focal adhesion. For molecular function, DEGs were revealed to be enriched in ATPase activity (Figure 2 and Table 2). Furthermore, the KEGG pathway analysis, using KOBAS, showed that the DEGs were significantly enriched in apoptosis, non-homologous end-joining (NHEJ), and miRNAs in cancer, with P<0.05 as the cut-off criterion (Figure 3A and Table 3).
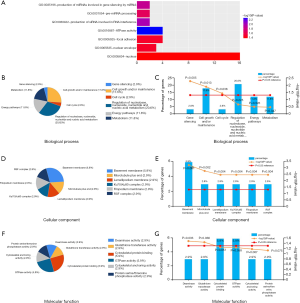
Table 2
Category | Term | Count | % | P value |
---|---|---|---|---|
GOTERM_MF_DIRECT | GO:0016887~ATPase activity | 4 | 11.76470588 | 0.005383803 |
GOTERM_BP_DIRECT | GO:0030422~production of siRNA involved in RNA interference | 2 | 5.882352941 | 0.013735725 |
GOTERM_BP_DIRECT | GO:0035196~production of miRNAs involved in gene silencing by miRNA | 2 | 5.882352941 | 0.022227855 |
GOTERM_BP_DIRECT | GO:0031054~pre-miRNA processing | 2 | 5.882352941 | 0.022227855 |
GOTERM_CC_DIRECT | GO:0005925~focal adhesion | 4 | 11.76470588 | 0.033246716 |
GOTERM_CC_DIRECT | GO:0005635~nuclear envelope | 3 | 8.823529412 | 0.033471497 |
GOTERM_CC_DIRECT | GO:0005634~nucleus | 16 | 47.05882353 | 0.040230878 |
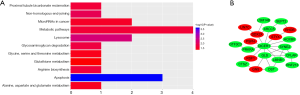
Table 3
Pathway ID | Term | Count | P value |
---|---|---|---|
hsa04210 | Apoptosis | 3 | 0.000250568 |
hsa04142 | Lysosome | 2 | 0.005141728 |
hsa03450 | Non-homologous end-joining | 1 | 0.011897331 |
hsa00531 | Glycosaminoglycan degradation | 1 | 0.016954027 |
hsa00220 | Arginine biosynthesis | 1 | 0.018634003 |
hsa04964 | Proximal tubule bicarbonate reclamation | 1 | 0.020311193 |
hsa01100 | Metabolic pathways | 4 | 0.021089253 |
hsa05206 | MicroRNAs in cancer | 2 | 0.027283703 |
hsa00250 | Alanine, aspartate and glutamate metabolism | 1 | 0.030316064 |
hsa00260 | Glycine, serine and threonine metabolism | 1 | 0.034455428 |
hsa00480 | Glutathione metabolism | 1 | 0.04432005 |
PPI network construction
In the PPI network of identified DEGs, 21 nodes and 30 edges were mapped, including 7 upregulated genes and 14 downregulated genes. Some of the genes isolated relatively at the edge were removed; therefore, not all of the 34 genes are shown in the network. Red nodes represent the upregulated genes and the green nodes represent the downregulated genes (Figure 3B).
Overall survival (OS) analysis
All 21 genes in the PPI network, including LIN7C, LSM14B, SEPT2, RHOB, HOXB8, SYNE2, CFNE2, RNF213, LMNB1, DST, GNS, CTSZ, RSF1, PRKRA, GTF3C3, PSPH, DDX3X, DICER1, GLS, LMNB1, and STYX, were analyzed using the survival package in R, and the OS of these genes for patients with pancreatic cancer is shown in Figure 4. Of the upregulated genes, DDX3X, STYX, and RSF1 were significantly associated with prognosis. Of the downregulated genes, LMNB1, PRKRA, SEPT2, and XRCC5 were associated with OS. A low expression of these 4 downregulated genes was associated with better survival, which indicates that S100PBP may downregulate these genes to inhibit the invasion and migration of pancreatic cancer cells. We also analyzed the expression of these 7 genes in pancreatic cancer by using the GEPIA database. The results showed that the expression levels of LMNB1 and XRCC5 were higher in pancreatic cancer tissues than in normal tissues (P<0.05) (Figure 5).
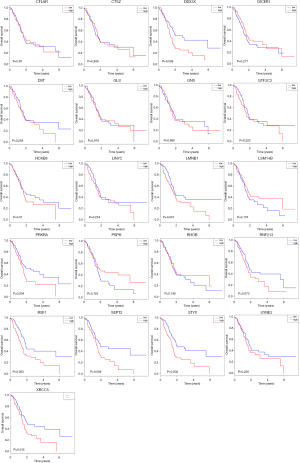
Discussion
As one of the most highly malignant tumors, pancreatic cancer is the fourth leading cause of cancer death, with a 5-year survival rate of only 7% (12,13). Despite a large number of related studies, due to a lack of understanding of the molecular mechanisms of the carcinogenesis of pancreatic cancer, there is still insufficient information on its early diagnosis and treatment. Therefore, further research into the etiological factors and mechanisms of pancreatic cancer progression should be performed to improve the survival rate of patients. The rapid development of microarray technology has enabled the general genetic alterations of disease progression to be determined, which may make it easier to identify the target genes for the diagnosis, therapy, and prognosis of diseases.
In our previous study to identify the potential function of S100PBP in pancreatic cancer, original pancreatic cancer cells and pancreatic cancer cells with S100PBP knockdown or overexpression were compared through analysis of the microarray datasets GSE35196 and GSE35198; a total of 34 DEGs were screened out, consisting of 11 upregulated genes and 23 downregulated genes. Through functional and pathway enrichment analyses, these genes were found to be enriched in the production of siRNA involved in RNA interference, the production of miRNAs involved in gene silencing by miRNA, and pre-miRNA processing. An OS analysis of 21 node genes in the PPI network (LIN7C, LSM14B, SEPT2, XRCC5, DDX3X, STYX, RHOB, GTF3C3, PSPH, DICER1, HOXB8, PRKRA, SYNE2, GLS, RSF1, CFLAR, LMNB1, CTSZ, GNS, DST, and RNF123) was also performed. We paid particular attention to LMNB1, PRKRA, SEPT2, and XRCC5, as they are highly expressed in pancreatic cancer and are associated with a poor prognosis.
LMNB1 is a component of the nuclear lamina, which encodes 1 of the 2 B-type lamin proteins. Lamins function in nuclear stability, transcription, DNA replication, and genome repair, together with lamin-binding proteins (14). LMNB1 regulates the proliferation and senescence of human diploid cells via a reactive oxygen species signaling pathway (15). The function of LMNB1 in cancer development and progression is still unclear. Some researchers have claimed that the overexpression of LMNB1 has been found in prostate, liver, and pancreatic cancers, whereas downregulation has been observed in gastric, colon, and lung cancers (14,16,17). Li et al. found that LMNB1 positively regulates the proliferation, invasion, and tumorigenicity of pancreatic cancer cells (18). In colon cancer, the overexpression of LMNB1 induces a small part of apoptosis by mitotic catastrophe, strengthens cell-cell junctions, and limits the migration of cancer cells by regulating β-catenin, lamin A/C, actin, and tubulin (19).
PRKRA is a protein activator of interferon-induced protein kinase PKR. PRKRA activates PKR to function as a negative regulator of growth in cellular apoptosis (20). In colon cancer, PKR activated by PRKRA plays a tumor suppressor function via the p53 signaling pathway (21). However, PKR also increases cell growth through the p38 mitogen-activated protein kinase and nuclear factor-κB pathways (22,23). PRKRA expression is higher in skin cancer and colorectal cancer than in healthy tissue (24,25). Furthermore, the PRKRA-PKR signaling pathway inhibits p53 turnover via a sumoylation-dependent mechanism, with the promotion of p53 phosphorylation and translational activation resulting in cell cycle arrest, which is related to tumor progression (26).
SEPT2 is a septin with GTPase activity and is consistently upregulated in most cancer types, such as brain, breast, cervical, gastric, head, and liver cancers (27). In hepatocellular carcinoma, SEPT2 accelerates cell growth by upregulating matrix metalloproteinases (MMP)-2 and -9 (28). In biliary tract cancer, SEPT2 is negatively regulated by miR-140-5p to accelerate cell proliferation and invasion (29). In a previous study, SEPT2 was found to promote breast cancer cell migration and invasion via activation of the MEK/extracellular signal-regulated kinase pathway (30).
XRCC5, an 80-kD subunit of the Ku heterodimer protein, binds to XRCC6 to form a dimer, which functions together with the IV-XRCC4 complex in the repair of DNA double-strand break by NHEJ. Many reports have found that NHEJ deficiencies increase genomic instability and tumorigenesis (31-36). XRCC5 expression is significantly higher in lung adenocarcinoma and esophageal squamous cell carcinoma than in corresponding healthy tissues (37,38). In hepatocellular carcinoma, XRCC5 inhibits tumor growth by inducing S-phase arrest through a p53-dependent pathway (39). However, XRCC5 can promote colon cancer growth through the XRCC5/p300/cyclooxygenase-2 signaling pathway (40).
In summary, we identified DEGs by performing a comprehensive bioinformatics analysis to identify multiple key genes downstream of S100PBP in pancreatic cancer. LMNB1, PRKRA, SEPT2, and XRCC5 were screened out and might play an important role in the development of pancreatic cancer. However, the specific functions of these genes and their mechanisms in pancreatic cancer require further analysis, and performing targeted experiments will be the aim of our future investigations. The expression levels of the 4 key genes in pancreatic cancer tissues are all higher than those in normal tissues, which might indicate that the people with higher expression levels of LMNB1, PRKRA, SEPT2 or XRCC5 are more likely to have pancreatic cancer if examined early. Meanwhile, we found the high expression of these 4 genes is related to poor survival, and this suggest that the expression levels of LMNB1, PRKRA, SEPT2 or XRCC5 may have a potential predictive role in the prognosis of pancreatic cancer patients. Our results might be promising for clinical transformation, which could provide new insight into the diagnosis and treatment of pancreatic cancer patients.
Conclusions
LMNB1, PRKRA, SEPT2, and XRCC5 might be the downstream key genes for the S100PBP gene in the promotion of the occurrence and development of pancreatic cancer.
Acknowledgements
Funding: None.
Footnote
Reporting Checklist: The authors have completed the MDAR checklist. Available at http://dx.doi.org/10.21037/tcr-20-2531
Conflicts of Interest: All authors have completed the ICMJE uniform disclosure form (available at http://dx.doi.org/10.21037/tcr-20-2531). The authors have no conflicts of interest to declare.
Ethical Statement: The authors are accountable for all aspects of the work in ensuring that questions related to the accuracy or integrity of any part of the work are appropriately investigated and resolved. The study was conducted in accordance with the Declaration of Helsinki (as revised in 2013). All information from GEO is available and free for public, so the agreement of the medical ethics committee board was not necessary.
Open Access Statement: This is an Open Access article distributed in accordance with the Creative Commons Attribution-NonCommercial-NoDerivs 4.0 International License (CC BY-NC-ND 4.0), which permits the non-commercial replication and distribution of the article with the strict proviso that no changes or edits are made and the original work is properly cited (including links to both the formal publication through the relevant DOI and the license). See: https://creativecommons.org/licenses/by-nc-nd/4.0/.
References
- Ferlay J, Soerjomataram I, Dikshit R, et al. Cancer incidence and mortality worldwide: sources, methods and major patterns in GLOBOCAN 2012. Int J Cancer 2015;136:E359-86. [Crossref] [PubMed]
- Neesse A, Algul H, Tuveson DA, et al. Stromal biology and therapy in pancreatic cancer: a changing paradigm. Gut 2015;64:1476-84. [Crossref] [PubMed]
- Waddell N, Pajic M, Patch AM, et al. Whole genomes redefine the mutational landscape of pancreatic cancer. Nature 2015;518:495-501. [Crossref] [PubMed]
- Dowen SE, Crnogorac-Jurcevic T, Gangeswaran R, et al. Expression of S100P and its novel binding partner S100PBPR in early pancreatic cancer. Am J Pathol 2005;166:81-92. [Crossref] [PubMed]
- Lines KE, Chelala C, Dmitrovic B, et al. S100P-binding protein, S100PBP, mediates adhesion through regulation of cathepsin Z in pancreatic cancer cells. Am J Pathol 2012;180:1485-94. [Crossref] [PubMed]
- Andreozzi M, Quintavalle C, Benz D, et al. HMGA1 Expression in Human Hepatocellular Carcinoma Correlates with Poor Prognosis and Promotes Tumor Growth and Migration in in vitro Models. Neoplasia 2016;18:724-31. [Crossref] [PubMed]
- Dennis G Jr, Sherman BT, Hosack DA, et al. DAVID: Database for Annotation, Visualization, and Integrated Dis-covery. Genome Biol 2003;4:3. [Crossref] [PubMed]
- Kanehisa M, Sato Y, Kawashima M, et al. KEGG as a reference resource for gene and protein annotation. Nucleic Acids Res 2016;44:D457-62. [Crossref] [PubMed]
- Szklarczyk D, Morris JH, Cook H, et al. The STRING database in 2017: quality-controlled protein-protein association networks, made broadly accessible. Nucleic Acids Res 2017;45:D362-8. [Crossref] [PubMed]
- Smoot ME, Ono K, Ruscheinski J, et al. Cytoscape 2.8: new features for data integration and network visualization. Bioinformatics 2011;27:431-2. [Crossref] [PubMed]
- Tang Z, Li C, Kang B, et al. GEPIA: a web server for cancer and normal gene expression profiling and interactive analyses. Nucleic Acids Res 2017;45:W98-W102. [Crossref] [PubMed]
- Siegel RL, Miller KD, Jemal A. Cancer statistics, 2015. CA Cancer J Clin 2015;65:5-29. [Crossref] [PubMed]
- Loosen SH, Neumann UP, Trautwein C, et al. Current and future biomarkers for pancreatic adenocarcinoma. Tumour Biol 2017;39:1010428317692231. [Crossref] [PubMed]
- Sakthivel KM, Sehgal P. A Novel Role of Lamins from Genetic Disease to Cancer Biomarkers. Oncol Rev 2016;10:309. [Crossref] [PubMed]
- Shimi T, Butin-Israeli V, Adam SA, et al. The role of nuclear lamin B1 in cell proliferation and senescence. Genes Dev 2011;25:2579-93. [Crossref] [PubMed]
- Butin-Israeli V, Adam SA, Goldman AE, et al. Nuclear lamin functions and disease. Trends Genet 2012;28:464-71. [Crossref] [PubMed]
- Irianto J, Pfeifer CR, Ivanovska IL, et al. Nuclear lamins in cancer. Cell Mol Bioeng 2016;9:258-67. [Crossref] [PubMed]
- Li L, Du Y, Kong X, et al. Lamin B1 is a novel therapeutic target of betulinic acid in pancreatic cancer. Clin Cancer Res 2013;19:4651-61. [Crossref] [PubMed]
- Izdebska M, Gagat M, Grzanka A. Overexpression of lamin B1 induces mitotic catastrophe in colon cancer LoVo cells and is associated with worse clinical outcomes. Int J Oncol 2018;52:89-102. [PubMed]
- Bennett RL, Blalock WL, Abtahi DM, et al. RAX, the PKR activator, sensitizes cells to inflammatory cytokines, serum withdrawal, chemotherapy, and viral infection. Blood 2006;108:821-9. [Crossref] [PubMed]
- Yoon CH, Lee ES, Lim DS, et al. PKR, a p53 target gene, plays a crucial role in the tumor-suppressor function of p53. Proc Natl Acad Sci U S A 2009;106:7852-7. [Crossref] [PubMed]
- Goh KC, deVeer MJ, Williams BR. The protein kinase PKR is required for p38 MAPK activation and the innate immune response to bacterial endotoxin. EMBO J 2000;19:4292-7. [Crossref] [PubMed]
- Deb A, Haque SJ, Mogensen T, et al. RNA-dependent protein kinase PKR is required for activation of NF-kappa B by IFN-gamma in a STAT1-independent pathway. J Immunol 2001;166:6170-80. [Crossref] [PubMed]
- Mullany LE, Herrick JS, Wolff RK, et al. Single nucleotide polymorphisms within MicroRNAs, MicroRNA targets, and MicroRNA biogenesis genes and their impact on colorectal cancer survival. Genes Chromosomes Cancer 2017;56:285-95. [Crossref] [PubMed]
- Sand M, Skrygan M, Georgas D, et al. Expression levels of the microRNA maturing microprocessor complex component DGCR8 and the RNA-induced silencing complex (RISC) components argonaute-1, argonaute-2, PACT, TARBP1, and TARBP2 in epithelial skin cancer. Mol Carcinog 2012;51:916-22. [Crossref] [PubMed]
- Bennett RL, Pan Y, Christian J, et al. The RAX/PACT-PKR stress response pathway promotes p53 sumoylation and activation, leading to G(1) arrest. Cell Cycle 2012;11:407-17. [Crossref] [PubMed]
- Liu M, Shen S, Chen F, et al. Linking the septin expression with carcinogenesis. Mol Biol Rep 2010;37:3601-8. [Crossref] [PubMed]
- Cao LQ, Shao ZL, Liang HH, et al. Activation of peroxisome proliferator-activated receptor-gamma (PPARgamma) inhibits hepatoma cell growth via downregulation of SEPT2 expression. Cancer Lett 2015;359:127-35. [Crossref] [PubMed]
- Yu J, Zhang W, Tang H, et al. Septin 2 accelerates the progression of biliary tract cancer and is negatively regulated by mir-140-5p. Gene 2016;589:20-6. [Crossref] [PubMed]
- Zhang N, Liu L, Fan N, et al. The requirement of SEPT2 and SEPT7 for migration and invasion in human breast cancer via MEK/ERK activation. Oncotarget 2016;7:61587-600. [Crossref] [PubMed]
- Gilley D, Tanaka H, Hande MP, et al. DNA-PKcs is critical for telomere capping. Proc Natl Acad Sci U S A 2001;98:15084-8. [Crossref] [PubMed]
- Goytisolo FA, Samper E, Edmonson S, et al. The absence of the dna-dependent protein kinase catalytic subunit in mice results in anaphase bridges and in increased telomeric fusions with normal telomere length and G-strand overhang. Mol Cell Biol 2001;21:3642-51. [Crossref] [PubMed]
- Difilippantonio MJ, Zhu J, Chen HT, et al. DNA repair protein Ku80 suppresses chromosomal aberrations and malignant transformation. Nature 2000;404:510-4. [Crossref] [PubMed]
- Ferguson DO, Alt FW. DNA double strand break repair and chromosomal translocation: lessons from animal models. Oncogene 2001;20:5572-9. [Crossref] [PubMed]
- Gao Y, Ferguson DO, Xie W, et al. Interplay of p53 and DNA-repair protein XRCC4 in tumorigenesis, genomic stability and development. Nature 2000;404:897-900. [Crossref] [PubMed]
- Zhu C, Mills KD, Ferguson DO, et al. Unrepaired DNA breaks in p53-deficient cells lead to oncogenic gene am-plification subsequent to translocations. Cell 2002;109:811-21. [Crossref] [PubMed]
- Wang S, Wang Z, Liu X, et al. Overexpression of Ku80 suggests poor prognosis of locally advanced esophageal squamous cell carcinoma patients. World J Surg 2015;39:1773-81. [Crossref] [PubMed]
- Ma Q, Li P, Xu M, et al. Ku80 is highly expressed in lung adenocarcinoma and promotes cisplatin resistance. J Exp Clin Cancer Res 2012;31:99. [Crossref] [PubMed]
- Wei S, Xiong M, Zhan DQ, et al. Ku80 functions as a tumor suppressor in hepatocellular carcinoma by inducing S-phase arrest through a p53-dependent pathway. Carcinogenesis 2012;33:538-47. [Crossref] [PubMed]
- Zhang Z, Zheng F, Yu Z, et al. XRCC5 cooperates with p300 to promote cyclooxygenase-2 expression and tumor growth in colon cancers. PLoS One 2017;12:e0186900. [Crossref] [PubMed]