New prognostic models for extranodal natural killer T-cell lymphoma, nasal-type using Cox regression and machine learning
Introduction
Extranodal natural killer T-cell lymphoma, nasal-type (ENKTL) is recognized as a distinct subtype of lymphoid neoplasms by the World Health Organization (WHO) (1). It has the characteristics of having a low global morbidity rate and a poor prognosis (2-4). L-asparaginase has been determined to be effective in inhibiting the proliferation of natural killer cells since patients with ENKTL respond poorly to conventional CHOP (cyclophosphamide, doxorubicin, vincristine, and prednisone) and CHOP-like regimens because of drug resistance (5-8). New chemotherapies incorporating L-asparaginase and its analogues were gradually introduced into clinical practice, with an increasing number of patients deriving benefit from these newer non-anthracycline–based treatments.
However, many studies (9-11) have shown that prognostic models based on conventional CHOP or CHOP-like chemotherapies, including the International prognostic index (IPI) (12) and Korean prognostic index (KPI) (13), were found no longer applicable under this new mode of treatment. As a result, a new prognostic model prognostic index of natural killer lymphoma (PINK) (14) was proposed in 2016. PINK incorporates four risk factors: age over 60 years, non-nasal type disease, stage III or IV disease in the Ann Arbor staging system and involvement of distant lymph nodes. It classifies patients into three groups: group 1 (risk factors ≤1), group 2 (risk factors =2), and group 3 (risk factors =3). These risks groups are significantly associated with different 3-year overall survival (OS). PINK has thus been validated for clinical use (15) and has been recommended by the latest National Comprehensive Cancer Network (NCCN) guideline (V.1, 2020); however, it classifies patients into just 3 groups. As a result, only a rough assessment of risk can be provided to a given patient: low risk, intermediate risk, or high risk. This does not provide an individualized or precise assessment of risk. Therefore, we have conducted this retrospective research to develop improved models. We present the following article in accordance with the TRIPOD reporting checklist (available at: http://dx.doi.org/10.21037/tcr-20-3017).
Methods
Participants
The study recruited 262 patients with ENKTL initially treated in the Peking University Third Hospital and the First Affiliated Hospital of Zhengzhou University from 2010 to 2020. Individuals were included in the study if they met the following criteria: (I) diagnosed as ENKTL according to histological and immunophenotypic features (including AE1/AE3, CD3, CD7, CD20, CD43, CD56, TIA-1, GranzymeB, EBER and Ki-67) on the basis of the WHO classification (16); (II) staged according to an endoscopic and imaging examination, including computed tomography (CT), magnetic resonance imaging (MRI) and/or ultrasound; (III) treated with non-anthracycline-based regimens. Patients were excluded if (I) they suffered from a previous malignancy or second primary tumor; (II) if they were not initially treated or their pretreatment information could not be accessed. Of the 262 patients, 5 suffered from previous malignancy or second primary tumor, 7 were not initially treated, or their pretreatment information could not be accessed. Finally, 250 patients were eligible for inclusion in our study. The study was conducted in accordance with the Declaration of Helsinki (as revised in 2013). This study was approved by our institutional review board (IRB00006761-M2020191). As our study was retrospective in nature with no personally identifiable information, the need for informed consent was waived.
Evaluation, definition and treatment
The data of participants’ medical history and physical examinations were gathered. Data from the endoscopic examination of upper aerodigestive tract; imaging examination (CT/enhanced CT/PET-CT, MR/enhanced MR and ultrasound) of involved regions and of the chest, abdomen, pelvis, limbs, and other regions; and bone marrow aspiration were collected. Each participant was staged using the Ann Arbor staging system and stratified using the PINK model. They were also staged by the newest staging system, the Chinese Southwest Oncology Group and Asia Lymphoma Study Group ENKTL (CA) system.
The Ann Arbor staging system was originally developed for Hodgkin’s lymphoma (17), However, this system was reported to be less accurate in assessing aggressive non-Hodgkin’s lymphoma due to disease spread patterns differing from those of non-Hodgkin’s lymphoma (18). ENKTL is particularly disparate, as it primarily occurs in the upper aerodigestive tract (19,20). A new staging system: the CA staging system, was first proposed in 2014, and in 2019 underwent prospective study and validation both in China and internationally. It has demonstrated superior evaluative ability for ENKTL compared to the Ann Arbor system (21), and thus patients were also staged by the CA staging system in the present study.
The CA staging system is consist of 4 stages: stage I, lesions located in nasal cavity or nasopharynx without local invasiveness or involvement of lymph node; stage II, lesions located in nasal cavity or nasopharynx with local invasiveness but without involvement of lymph node or lesions primarily located outside upper aerodigestive tract ; stage III, lesions combined with involvement of regional lymph node; stage IV, disseminated lesions or involvement of non-regional lymph nodes or lymph nodes involved on both sides of the diaphragm (21).
To facilitate machine learning, all involved regions are defined according to their anatomy, which included the nasal cavity, sinus, nasopharynx, oropharynx or tonsil, larynx, palate, oral cavity and tongue, parapharyngeal space or parotid gland, orbital cavity, skin of face, skin and soft tissue of body, gastrointestinal tract, lung, pericardium, spleen, liver, kidney or adrenal gland, testis, uterus or ovary, bone marrow and central nervous system; The involved lymph nodes are also defined according to the classification and delineation of systemic lymph nodes, which included the head, cervical, supraclavicular, axillary, mediastinal, abdominal, retroperitoneal, pelvic, and limb lymph nodes. Primary site could be classified as upper aerodigestive tract or extra upper aerodigestive tract.
All participants received non-anthracycline-based treatment according to their risk-based stratification. Comprehensive treatment included surgery, radiotherapy, chemotherapy, targeted therapy, stem cell transplantation, and maintenance therapy. Surgery treatment referred to resection of the lesion during the biopsy, and follow-up examinations returned negative results, usually followed by radiotherapy or chemotherapy. Radiotherapy was performed using the standard scheme of a radical dose of 50–52 Gy/25–26 Gy. Chemotherapy was performed using non-anthracycline-based regimens, mainly incorporating P-GEMOX (gemcitabine, pegaspargase and oxaliplatin), DDGP (dexamethasone, cisplatin, gemcitabine and pegaspargase) and Modified-SMILE (dexamethasone, methotrexate, ifosfamide, pegaspargase, Mesna and etoposide), which are the preferred regimens of the NCCN guideline (v.1, 2020).
It should be noted that 7 patients received conventional CHOP or CHOP-like regimens. However, 1 of them changed to conventional therapy because the expected clinical effects were not achieved after administration of non-anthracycline-based treatment, 2 received as consolidation therapy after being relieved, and 4 received it as pretreatment before transplantation. Since none of them were initially or mainly treated with conventional therapies, they were still included in the study. Targeted therapy was used when disease progressed, and mainly consisted of programmed cell death protein 1 (PD-1) receptor inhibitors and chidamide. Transplantation referred to autologous stem cell transplantation after complete remission (CR) or unconfirmed complete remission (CRu). Maintenance therapy was mainly used for patients in terminal stage, and included steroids, thalidomide, and other forms for palliative treatment.
The construction and validation of the statistical model nomogram
Fifteen variables associated with OS that were reported in previous studies were selected including sex, age, Ann Arbor stage, CA stage, lymph node involvement, the interval from primary symptoms to diagnosis, primary site, Eastern Cooperative Oncology Group (ECOG) score, B symptoms, PINK model stratification, and lactate dehydrogenase (LDH) level, hemoglobin, platelet, and monocyte and prognostic nutritional index [PNI, defined as albumin g/L + 5 × lymphocyte count (×109/L)]. We separated continuous variables into low and high groups either using the well-known cutoff (age) or on the basis of the usual cutoff value for the normal range (such as LDH and hemoglobin) or the cutoffs of which prognostic value had been validated in previous studies (platelet, monocyte, PNI) (22,23). Univariate analysis was then performed using the Kaplan–Meier method. Apart from gender and intervals from primary symptoms to diagnosis, the other thirteen variables were significant for OS and underwent multivariate analysis by Cox regression method. Five variables, including age, CA stage, ECOG, B symptoms, and LDH score, were found to be statistically significant and were finally selected to construct the statistical model nomogram. For a better evaluation of this method of constructing a model, we used 5-fold cross validation. All patients were stratified randomly divided and divided into 5 groups according to CA stage; in turn, each group served as the validation cohort with the other 4 serving as the training cohort, and the final model was constructed on all 250 patients’ data (Figure 1). Discrimination and calibration powers were evaluated by concordance index (C-index) and calibration curve, respectively, which as is were recommended for the evaluation of survival regression analysis.
The construction and validation of the machine learning model
As CA stage consists of the involved regions, lymph nodes, and the primary site, to facilitate machine learning, these 3 were analyzed instead of the CA stage itself. Age, ECOG score, B symptoms, and LDH score were also included in the machine learning model. LightGBM, recognized as an excellent gradient boosting decision tree (GBDT) algorithm in machine learning, was used for data analysis. A 5-fold cross-validation for the machine learning model was used for the same groups used by statistical model nomogram. For 3-year OS prediction, patients were first labeled as live or dead according to whether their survival time was over 3 years or not, and were then included in the analysis. AUC and binary errors were used to evaluate the discrimination and calibration powers due to binary classification nature of the LightGBM algorithm.
Statistical analysis
The OS rate was defined as the period from the initial diagnosis to ENKTL-related death or the last follow-up. Survival curves were depicted by the life table method, and univariate analysis was conducted with the Kaplan-Meier method. Multivariate analysis was conducted with Cox regression method by entering selection process, and the nomogram was constructed using multivariate Cox proportional hazards model method. Two missed values from PNI and intervals between primary symptoms to diagnosis were not included in univariate analysis and multivariate analysis. C-indices were calculated by calculating the area under the receiver operating characteristic (ROC) curve. Calibration curves comprised the predicted probability of OS curve and actual OS curve, and were plotted by bootstrap resampling (1,000 resamples for the training cohort and 500 for the validation cohort). AUC was defined as true positives (TP): TP + false positives (FP) and binary error was defined as FP + FN/all samples. Statistical analyses were performed in SPSS 20.0 (RRID:SCR_002865). The C-index, nomogram, and calibration curve were developed in R language using RStudio (RRID:SCR_000432). Machine learning was performed using Python in PyCharm 2020.1.2 (RRID:SCR_018221). Graphs were processed in GraphPad Prism 8 (RRID:SCR_002798) and Adobe Illustrator CC 2014 (RRID:SCR_010279). A P value <0.05 was considered statistically significant.
Results
Participant characteristics
The clinical characteristics of 250 participants are shown in Table 1. The involved regions and lymph nodes are outlined in Table 2. Out of all 250 patients analyzed, 195 (78%) patients received whole-body PET-CT imaging before initial treatment. In 55 patients who did not receive PET-CT, 38 were in early stage (Ann Arbor stage I or II) and 17 were in advanced stage (Ann Arbor stage III or IV). The mean follow-up time for survival patients was 32 months, and the 1- and 3-year OS were 80.9% and 69.6%, respectively (Figure 2). In the univariate analysis, the following 13 variables were associated with OS: age, Ann Arbor stage, CA stage, lymph node involvement, primary site, ECOG score, B symptoms, PINK, LDH level, hemoglobin, platelet, monocyte, and PNI. In the multivariate analysis, 5 variables were significant: age, CA stage, ECOG score, B symptoms, and LDH level (Table 3). The grouping by CA stage for cross-validation is shown in Table 4.
Table 1
Characteristics | n (%) |
---|---|
Gender | |
Male | 182 (72.8) |
Female | 68 (27.2) |
Age (years) | |
<60 | 209 (83.6) |
≥60 | 41 (16.4) |
Ann arbor stage | |
I | 67 (26.8) |
II | 101 (40.4) |
III | 20 (8.0) |
IV | 62 (24.8) |
CA stage | |
I | 72 (28.8) |
II | 53 (21.2) |
III | 44 (17.6) |
IV | 81 (32.4) |
Lymph node involvement | |
Yes | 95 (38.0) |
No | 155 (62.0) |
Interval from primary symptoms to diagnosis (months) | |
<12 | 214 (85.6) |
≥12 | 34 (13.6) |
Unknown | 2(0.8) |
Primary site | |
UADT | 196 (78.4) |
Extra-UADT | 54 (21.6) |
ECOG score | |
0 | 59 (23.6) |
1 | 141 (56.4) |
2 | 42 (16.8) |
≥3 | 8 (3.2) |
B symptoms | |
Yes | 133 (53.2) |
No | 117 (46.8) |
PINK | |
Group1 | 133 (53.2) |
Group2 | 53 (21.2) |
Group3 | 64 (25.6) |
LDH level (U/L) | |
<245 | 143 (57.2) |
≥245 | 107 (42.8) |
Hemoglobin (g/L) | |
<120 | 86 (34.4) |
≥120 | 164 (65.6) |
Platelet (109/L) | |
<200 | 119 (47.6) |
≥200 | 131 (52.4) |
Monocyte (109/L) | |
<0.5 | 115 (46.0) |
≥0.5 | 135 (54.0) |
PNI | |
<48 | 169 (67.6) |
≥48 | 79 (31.6) |
Unknown | 2(0.8) |
Treatment | |
Surgery | 14 (5.6) |
Radiotherapy | 113 (45.2) |
Chemotherapy | |
P-GEMOX | 27 (10.8) |
DDGP | 158 (63.2) |
Modified-SMILE | 39 (15.6) |
Others | 24 (9.6) |
CHOP or CHOP-like regimens | 7 (2.8) |
Targeted therapy | 17 (6.8) |
Transplantation | 4 (1.6) |
Maintenance therapy | 7 (2.8) |
CA, the Chinese Southwest Oncology Group and Asia Lymphoma Study Group ENKTL system; ECOG, Eastern Cooperative Oncology Group; PINK, prognostic index of natural killer lymphoma; LDH, lactate dehydrogenase concentrations; PNI, prognostic nutritional index; P-GEMOX, gemcitabine, pegaspargase and oxaliplatin; DDGP, dexamethasone, cisplatin, gemcitabine and pegaspargase; Modified-SMILE, dexamethasone, methotrexate, ifosfamide, pegaspargase, mesna and etoposide; CHOP, cyclophosphamide, doxorubicin, vincristine, and prednisone.
Table 2
n (%) | |
---|---|
Regions | |
None | 3 (1.2) |
Nasal cavity | 173 (69.2) |
Sinus | 40 (16.0) |
Nasopharynx | 82 (32.8) |
Oropharynx or tonsil | 38 (15.2) |
Larynx | 12 (4.8) |
Palate | 19 (7.6) |
Oral cavity and tongue | 7 (2.8) |
Parapharyngeal space or parotid gland | 26 (10.4) |
Orbital cavity | 11 (4.4) |
Skin of face | 23(9.2) |
Skin and soft tissue of body | 39 (15.6) |
Gastrointestinal tract | 28 (11.2) |
Lung | 22 (8.8) |
Pericardium | 1 (0.4) |
Spleen | 13 (5.2) |
Liver | 6 (2.4) |
Kidney or adrenal gland | 12 (4.8) |
Testis | 9 (3.6) |
Uterus or ovary | 7 (2.8) |
Bone marrow | 7 (2.8) |
Central nervous system | 5 (2.0) |
Lymph nodes | |
None | 156 (62.4) |
Head | 7 (2.8) |
Neck | 76 (30.4) |
Supraclavicular | 7 (2.8) |
Axilla | 19 (7.6) |
Mediastinum | 16 (6.4) |
Abdominal cavity | 16 (6.4) |
Retroperitoneal | 16 (6.4) |
Pelvic cavity | 30(12.0) |
Limb | 2 (0.8) |
Involved regions and lymph nodes are determined by CT/enhanced CT/PET-CT, MR/enhanced MR and ultrasound, 195/250 (78%) received whole-body PET-CT. In 55 patients who did not received PET-CT, 38 were in early stage (Ann Arbor stage I or II), and 17 were in advanced stage (Ann Arbor stage III or IV).
Table 3
Characteristics | Univariate analysis | Multivariable analysis | ||
---|---|---|---|---|
P value | HR (95% CI) | P value | ||
Gender (male vs. female) | 0.598 | – | – | |
Ann Arbor Stage (I, II, III, IV) | <0.001 | – | 0.983 | |
Lymph node involvement (yes vs. no) | <0.001 | – | 0.624 | |
Interval from primary symptoms to diagnosis (<12 vs. ≥12 months; n=248) | 0.962 | – | – | |
Primary site (UADT vs. Extra-UADT) | <0.001 | – | 0.595 | |
PINK (Group1, Group2, Group3) | <0.001 | – | 0.477 | |
Hemoglobin (<120 vs. ≥120 g/L) | <0.001 | – | 0.1 | |
Platelet (<200 vs. ≥200, 109/L) | 0.018 | – | 0.819 | |
Monocyte (<0.5 vs. ≥0.5, 109/L) | 0.008 | – | 0.498 | |
PNI (<48 vs. ≥48; n=248) | 0.001 | – | 0.355 | |
Age (<60 vs. ≥60, years) | 0.039 | 2.011 (1.043–3.878) | 0.037 | |
CA Stage (I, II, III, IV) | <0.001 | 2.341 (1.199–4.569) | 0.013 | |
ECOG score (0,1,2 vs. ≥3) | <0.001 | 2.4 (1.607–3.583) | <0.001 | |
B symptoms (Yes vs. No) | 0.007 | 0.325 (0.161–0.657) | 0.002 | |
LDH level (<245 vs. ≥245 U/L) | <0.001 | 3.746 (1.070–3.746) | 0.03 |
Two missed values in Interval from primary symptoms to diagnosis and PNI were not included in univariate analysis. Fifteen variables in 250 patients first received univariate analysis and 13 statistically significant ones received multivariable analysis. In multivariable analysis, 5 variables were statistically significant. UADT, upper aerodigestive tract; PINK, prognostic index of natural killer lymphoma; PNI, prognostic nutritional index; CA, the Chinese Southwest Oncology Group and Asia Lymphoma Study Group ENKTL system; ECOG, Eastern Cooperative Oncology Group; LDH, lactate dehydrogenase concentrations.
Table 4
CA stage | Group1 | Group2 | Group3 | Group4 | Group5 | Total |
---|---|---|---|---|---|---|
I | ||||||
Censored | 14 | 14 | 14 | 14 | 13 | 69 |
Not censored | 1 | 1 | 1 | 0 | 0 | 3 |
II | ||||||
Censored | 9 | 9 | 9 | 9 | 10 | 46 |
Not censored | 0 | 1 | 2 | 2 | 2 | 7 |
III | ||||||
Censored | 6 | 7 | 7 | 7 | 7 | 34 |
Not censored | 2 | 1 | 2 | 2 | 3 | 10 |
IV | ||||||
Censored | 7 | 7 | 7 | 7 | 8 | 36 |
Not censored | 11 | 10 | 8 | 9 | 7 | 45 |
Total | 50 | 50 | 50 | 50 | 50 | 250 |
According to the Ann Arbor staging system, the distribution for stages I-IV was 67 (26.8%), 101 (40.4%), 20 (8.0%) and 62 (24.8%), respectively. Stage III had the lowest proportion (8.0%), and its survival curve overlapped with that of stage IV, implying poor discrimination (Figure 3A). These results were in line with previous studies (19-26). In contrast, the distribution of the CA staging system for stages I–IV was 72 (28.8%), 53 (21.2%), 44 (17.6%), 81 (32.4%), respectively, which is more balanced, and there was less overlap between the survival curves (Figure 3B). The survival curves of the PINK model also showed good discrimination (Figure 3C).
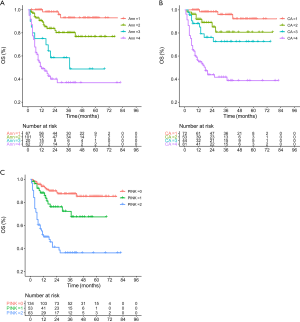
New models and comparison with the PINK model
The statistical model nomogram and machine learning model predicting the 3-year OS are illustrated in Figures 4,5.
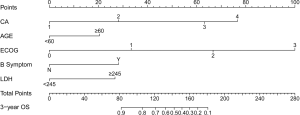
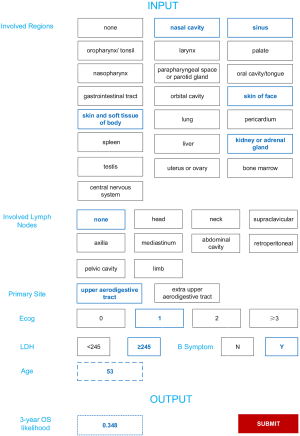
To use the statistical model nomogram, for an individual patient, the value of each variable is located on the corresponding variable axis, and a line drawn upwards to the Points axis to determines the point received for each variable. The sum of 3 points is located on the Total Points axis, and the lines drawn downwards to the 3-year OS axis determine the likelihood of 3-year OS.
To use machine learning model, a patient’s 3-year OS likelihood can be output by checking the involved regions and lymph nodes, primary site, ECOG, LDH, and entering age.
For discrimination power, C-indices for the statistical model nomogram were 0.840, 0.846, 0.855, 0.859, and 0.853 in training cohort, and 0.875, 0.872, 0.819, 0.815, and 0.834 in the validation cohort, with mean C-indices of 0.851±0.008 and 0.843±0.029, respectively. Accordingly, the C-indices for PINK were 0.734, 0.751, 0.783, 0.750, and 0.770 in the training cohort, and 0.862, 0.783, 0.652, 0.779, and 0.710 in the validation cohort, with mean C-indices 0.758±0.019 and 0.757±0.080, respectively. In addition, the 3-year AUCs for machine learning model were 0.929, 0.919, 0.904, 0.923, and 0.926 in the training cohort, and 0.911, 0.841, 0.821, 0.874, and 0.878 in the validation cohort, with mean AUCs 0.902±0.010 and 0.865±0.035.
To demonstrate the calibration power, calibration curves of 5 rounds cross-validation for statistical model nomogram are plotted in Figure 6; they show a good correlation between the predicted outcomes and the reality. Finally, binary errors in the machine learning model were 0.167, 0.175, 0.194, 0.148, and 0.162 in the training cohort, and 0.179, 0.250, 0.235, 0.222, and 0.120 in the validation cohort, with mean binary errors of 0.169±0.017 and 0.201±0.053, indicating good calibration power.
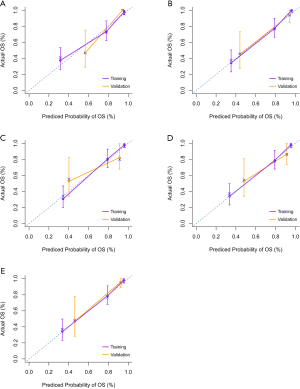
Discussion
At present, risk-adapted treatment is widely accepted, and thus improving the risk stratification of patients is a rational goal. In the past, the IPI, KPI, and other models were used (24,27); however, they were either not specially designed for ENKTL or based on conventional anthracycline-based chemotherapy. The treatment for ENKTL has changed from anthracycline-based treatment to non-anthracycline-based treatment, but the current NCCN guidelines do not recommend these models. PINK is applicable in the era of non-anthracycline–based treatment; however, patients cannot be assessed individually. Our study ultimately identified age, CA stage, ECOG, B symptoms, and LDH as significant factors in constructing statistical model nomograms, and replaced the CA stage with the involved regions, lymph nodes, and primary site in a machine learning model.
We found that our models' discriminatory power was superior to that of PINK, and this is perhaps due to two mains reasons. First, we used the CA staging system instead of the Ann Arbor system adopted by PINK. The CA staging system is specially developed for ENKTL, has been proven to be superior in stratifying ENKTL patients, and incorporates more clinical information. In PINK, there are 4 parameters, age, stage III or IV disease in Ann Arbor staging system, non-nasal type disease, and involvement of distant lymph nodes; however, the CA staging system itself contains non-nasal type disease and involvement of lymph nodes, so we use fewer variables while analyzing more information. Second, compared with the PINK statistical grouping method, less information is lost during modeling when using the Cox regression method. As for machine learning algorithm, lightGBM, unlike traditional statistical method, which assume a linear combination of risk factors, lightGBM is essentially a decision tree of machine learning that uses more details and manages more complex relationships.
Apart from improving discrimination power, our models’ variables are common and relevant to clinical practice, enhancing their practical utility.
First, age is statistically significant and included because older adults generally have more complications and are presumed to be less tolerance to chemotherapy than young patients (28,29), which makes inadequate treatment a common problem. Furthermore, gene aberrations are more likely to accumulate in older patients leading to more aggressive tumors (30,31).
The CA staging system has advantages over the Ann Arbor staging system. For unbalanced stratification in stage III and overlapping survival curves between stage III and IV in the Ann Arbor staging system, PINK incorporates stage III and IV as a single parameter. The CA staging system has a more balanced stratification across the 4 stages, and it is statistically significant in multivariate analysis, while the Ann Arbor staging system is not. The CA staging system is specially designed for ENKTL, and there is a high probability it will replace the Ann Arbor staging system in the future; our model is thus intended to better assist clinicians in this regard. We also discarded the staging system in the machine learning model, which allows it to analyze more clinical information and be more adaptable.
We found that the ECOG score in our models demonstrated greater weight than in previous models; in particular, when the ECOG score exceeded 3, it had more weight than stage IV of the CA staging system. There are two plausible explanations for this. First, non-anthracycline-based treatments are considered more intense than conventional CHOP or CHOP-like regimens, and thus patients with a poor performance status could be more vulnerable to treatment-related side effects (14). Second, with the effect of non-anthracycline-based treatments exceeding conventional therapies, advanced stage patients who are tolerant to newer regimens generally benefit more than before, making a poor performance status the most dangerous risk factor.
Last, unlike the 3 variables mentioned above, which mainly reflect the patient’s ability to tolerate treatment and invasive potential of the tumor (32), B symptoms and elevated LDH can indicate the burden and replication capacity, and thus correlate with a worse OS (33,34); therefore, these 2 variables could further assist in identifying those at a higher risk.
Although our models reached acceptable levels of discrimination and calibration in the cross-validation, they still have not undergone further validation from other centers’ data. Furthermore, although the 3 parameters we adopted could easily be applied to clinical practice and enhance practical utility, other risk factors, such as Epstein–Barr (EB) virus DNA, could not be assessed. This is mainly due to the different definition of high titer and the measurement procedures in our and other centers, which make the wider application of these factors challenging (14,35). Although the CA staging system has undergone prospective studies and has proven to be more suitable for ENKTL, the power to discriminate between CA stage II and III was not statistically significant in our data. This may be because regional lymph nodes lie in the radiation field and radiotherapy improves the response rate in stage III, or simply because there was bias arising from the limited data of our study. We assume it might because regional lymph nodes are in the radiation field and radiotherapy hence improve the response rate in stage III, or it is just due to bias because we are not able to include many centers’ data. As the machine learning model did not include a staging system, it does not have the same issues; however, machine leaning is usually used for the analysis of a vast amount of multidimensional data, and, due to low incidence of ENKTL, the amount of data in our research was limited.
Conclusions
In conclusion, we developed two prognostic models that could provide an individual estimation of risk for patients with ENKLT in the era of non-anthracycline-based treatment. The models were preliminarily validated to have good discriminatory power; however, this still needs to be verified by prospective study.
Acknowledgments
We thank Xiaoxiao Wang and Nan Li (Clinical epidemiology research center of Peking University Third Hospital) for their suggestions on statistical analysis and Zhiwei Zhang (Qidian Fuliu Technology Co., Ltd, Beijing) for his technical assistance.
Funding: This work was supported by
Footnote
Reporting Checklist: The authors have completed the TRIPOD reporting checklist. Available at http://dx.doi.org/10.21037/tcr-20-3017
Data Sharing Statement: Available at http://dx.doi.org/10.21037/ tcr-20-3017
Peer Review File: Available at http://dx.doi.org/10.21037/ tcr-20-3017
Conflicts of Interest: All authors have completed the ICMJE uniform disclosure form (available at http://dx.doi.org/10.21037/tcr-20-3017). The authors have no conflicts of interest to declare.
Ethical Statement: The authors are accountable for all aspects of the work in ensuring that questions related to the accuracy or integrity of any part of the work are appropriately investigated and resolved. The study was conducted in accordance with the Declaration of Helsinki (as revised in 2013). This study was approved by our institutional review board (IRB00006761-M2020191). As our study was retrospective in nature with no personally identifiable information, the need for informed consent was waived.
Open Access Statement: This is an Open Access article distributed in accordance with the Creative Commons Attribution-NonCommercial-NoDerivs 4.0 International License (CC BY-NC-ND 4.0), which permits the non-commercial replication and distribution of the article with the strict proviso that no changes or edits are made and the original work is properly cited (including links to both the formal publication through the relevant DOI and the license). See: https://creativecommons.org/licenses/by-nc-nd/4.0/.
References
- Swerdlow SH, Campo E, Pileri SA, et al. The 2016 revision of the World Health Organization classification of lymphoid neoplasms. Blood 2016;127:2375-90. [Crossref] [PubMed]
- Schuler A, Smith E, Lowe L, et al. Extranodal natural killer/T-cell lymphoma, nasal type: A rare but critical diagnosis. JAAD Case Rep 2017;3:225-7. [Crossref] [PubMed]
- Au WY, Weisenburger DD, Intragumtornchai T, et al. Clinical differences between nasal and extranasal natural killer/T-cell lymphoma: a study of 136 cases from the International Peripheral T-Cell Lymphoma Project. Blood 2009;113:3931-7. [Crossref] [PubMed]
- Vose J, Armitage J, Weisenburger D, et al. International peripheral T-cell and natural killer/T-cell lymphoma study: pathology findings and clinical outcomes. J Clin Oncol 2008;26:4124-30. [Crossref] [PubMed]
- Yamaguchi M, Kita K, Miwa H, et al. Frequent expression of P-glycoprotein/MDR1 by nasal T-cell lymphoma cells. Cancer 1995;76:2351-6. [Crossref] [PubMed]
- Kim WS, Song SY, Ahn YC, et al. CHOP followed by involved field radiation: is it optimal for localized nasal natural killer/T-cell lymphoma? Ann Oncol 2001;12:349-52. [Crossref] [PubMed]
- Lee SH, Ahn YC, Kim WS, et al. The effect of pre-irradiation dose intense CHOP on anthracyline resistance in lo-calized nasal NK/T-cell lymphoma. Haematologica 2006;91:427-8. [PubMed]
- Scherf U, Ross DT, Waltham M, et al. A gene expression database for the molecular pharmacology of cancer. Nat Genet 2000;24:236-44. [Crossref] [PubMed]
- Wang L, Xia ZJ, Lu Y, et al. A modified international prognostic index including pretreatment hemoglobin level for early stage extranodal natural killer/T cell lymphoma. Leuk Lymphoma 2015;56:3038-44. [Crossref] [PubMed]
- Cai Q, Luo X, Zhang G, et al. New prognostic model for extranodal natural killer/T cell lymphoma, nasal type. Ann Hematol 2014;93:1541-9. [Crossref] [PubMed]
- Huang JJ, Zhu YJ, Xia Y, et al. A novel prognostic model for extranodal natural killer/T-cell lymphoma. Med Oncol 2012;29:2183-90. [Crossref] [PubMed]
- International Non-Hodgkin's Lymphoma Prognostic Factors P.. A predictive model for aggressive non-Hodgkin's lymphoma. N Engl J Med 1993;329:987-94. [Crossref] [PubMed]
- Lee J, Suh C, Park YH, et al. Extranodal natural killer T-cell lymphoma, nasal-type: a prognostic model from a retrospective multicenter study. J Clin Oncol 2006;24:612-8. [Crossref] [PubMed]
- Kim SJ, Yoon DH, Jaccard A, et al. A prognostic index for natural killer cell lymphoma after non-anthracycline-based treatment: a multicentre, retrospective analysis. Lancet Oncol 2016;17:389-400. [Crossref] [PubMed]
- Shustov AR. Extranodal natural killer T-cell lymphoma-new profiling, old tricks. Lancet Oncol 2016;17:271-3. [Crossref] [PubMed]
- Chan JK. Extranodal NK/T-cell lymphoma, nasal type. WHO classification of tumours of haematopoietic and lymphoid tissues 2008:285-8.
- Carbone PP, Kaplan HS, Musshoff K, et al. Report of the Committee on Hodgkin's Disease Staging Classification. Cancer Res 1971;31:1860-1. [PubMed]
- Rosenberg SA. Validity of the Ann Arbor staging classification for the non-Hodgkin's lymphomas. Cancer Treat Rep 1977;61:1023-7. [PubMed]
- Hong H, Du X, Zhang M, et al. A Proposal for a New Staging System of Extranodal Natural Killer T-cell Lymphoma, Nasal-Type: a Multicenter Study of Chinese Southwest Oncology Group (CSWOG). Meeting of the American-society-of-hematology. 2014.
- Yamaguchi M, Oguchi M, Suzuki R. Extranodal NK/T-cell lymphoma: Updates in biology and management strategies. Best Pract Res Clin Haematol 2018;31:315-21. [Crossref] [PubMed]
- Hong H, Li Y, Lim ST, et al. A proposal for a new staging system for extranodal natural killer T-cell lymphoma: a multicenter study from China and Asia Lymphoma Study Group. Leukemia 2020;34:2243-8. [Crossref] [PubMed]
- Huang JJ, Li YJ, Xia Y, et al. Prognostic significance of peripheral monocyte count in patients with extranodal natural killer/T-cell lymphoma. BMC Cancer 2013;13:222. [Crossref] [PubMed]
- Yao N, Hou Q, Zhang S, et al. Prognostic Nutritional Index, Another Prognostic Factor for Extranodal Natural Killer/T Cell Lymphoma, Nasal Type. Front Oncol 2020;10:877. [Crossref] [PubMed]
- Yang Y, Zhang YJ, Zhu Y, et al. Prognostic nomogram for overall survival in previously untreated patients with extranodal NK/T-cell lymphoma, nasal-type: a multicenter study. Leukemia 2015;29:1571-7. [Crossref] [PubMed]
- Kim TM, Lee SY, Jeon YK, et al. Clinical heterogeneity of extranodal NK/T-cell lymphoma, nasal type: a national survey of the Korean Cancer Study Group. Ann Oncol 2008;19:1477-84. [Crossref] [PubMed]
- Kwong YL, Pang AW, Leung AY, et al. Quantification of circulating Epstein-Barr virus DNA in NK/T-cell lymphoma treated with the SMILE protocol: diagnostic and prognostic significance. Leukemia 2014;28:865-70. [Crossref] [PubMed]
- Hong H, Huang H, Fang X, et al. A prognostic index for nasal-type early-stage extranodal natural killer/T-cell lymphoma: A multicenter study. Am J Hematol 2019;94:E122-4. [Crossref] [PubMed]
- Kim SM, Park S, Oh DR, et al. Extra-nodal natural killer/T cell lymphoma in elderly patients: the impact of aging on clinical outcomes and treatment tolerability. Ann Hematol 2016;95:581-91. [Crossref] [PubMed]
- Saygin C, Jia X, Hill B, et al. Impact of comorbidities on outcomes of elderly patients with diffuse large B-cell lymphoma. Am J Hematol 2017;92:989-96. [Crossref] [PubMed]
- Mareschal S, Lanic H, Ruminy P, et al. The proportion of activated B-cell like subtype among de novo diffuse large B-cell lymphoma increases with age. Haematologica 2011;96:1888-90. [Crossref] [PubMed]
- Thunberg U, Amini RM, Linderoth J, et al. BCL2 expression in de novo diffuse large B-cell lymphoma partly reflects normal differences in age distribution. Br J Haematol 2009;146:683-4. [Crossref] [PubMed]
- Chen SY, Yang Y, Qi SN, et al. Validation of nomogram-revised risk index and comparison with other models for extranodal nasal-type NK/T-cell lymphoma in the modern chemotherapy era: indication for prognostication and clinical decision-making. Leukemia 2021;35:130-42. [Crossref] [PubMed]
- Liu ZL, Bi XW, Zhang XW, et al. Characteristics, Prognostic Factors, and Survival of Patients with NK/T-Cell Lym-phoma of Non-upper Aerodigestive Tract: A 17-Year Single-Center Experience. Cancer Res Treat 2019;51:1557-67. [Crossref] [PubMed]
- Na II, Kang HJ, Park YH, et al. Prognostic factors for classifying extranodal NK/T cell lymphoma, nasal type, as lymphoid neoplasia. Eur J Haematol 2007;79:1-7. [Crossref] [PubMed]
- Preiksaitis JK, Pang XL, Fox JD, et al. Interlaboratory comparison of epsteinbarr virus viral load assays. Am J Transplant 2009;9:269-79. [Crossref] [PubMed]