Combination of GC-MS based metabolomics analysis with mouse xenograft models reveals a panel of dysregulated circulating metabolites and potential therapeutic targets for colorectal cancer
Introduction
Colorectal cancer (CRC) is a common digestive tract cancer, which based on the 2018 global cancer statistics, ranks fourth in incidence rate, and third in mortality (1). Treatment of CRC usually involves surgery, chemotherapy, and radiotherapy. However, despite recent advances in these treatment strategies as well as the use of a multidisciplinary therapeutic approach, the prognosis for CRC remains poor. Poor prognosis is related to a lack of early symptoms, which means that diagnosis is often not confirmed until the patient reaches advanced stages of the disease. Indeed, the prognosis of CRC is closely related to the stage of the disease at the time of diagnosis. Thus, the development of an effective early detection approach for CRC is critical to prolong survival time and reduce mortality (2).
Traditionally, a fecal occult blood test has been widely used as a noninvasive screening tool for CRC detection (3). However, this method has shown a low sensitivity in the detection of adenomas and CRC due to false-positive results caused by diet and medicines (4). Invasive screening such as colonoscopy is more sensitive and effective, but its high cost and risk of complications limit its wide application in the early detection of CRC (5). Although, at present, carcinoembryonic antigen is still widely used as a serum biomarker for CRC, its low sensitivity (40.9–51.8%) means that it is mainly used to evaluate the therapeutic effects of treatments and to monitor the recurrence of tumors rather than being used as an early diagnostic marker of CRC. Such limited screening tools provide the rationale to develop a novel and effective diagnostic biomarker for CRC.
Changes in lipid metabolism are one of the hallmarks of many malignant tumors (6). Recent studies have described changes in the content and composition of fatty acids, polar lipids, lipoprotein, and triacylglycerol in the serum, tumor tissue, and adipose tissue of patients with CRC (6). Glycolysis is also often dysregulated in CRC, and overexpression of hexokinase 2 has been observed in CRC cases (7). Metabolomics is emerging as an important component of systems biology after genomics, transcriptomics, and proteomics (8). Metabolomics has the potential to identify biomarkers which may predict disease status, detect early-stage cancer, direct therapies and determine drug efficacy (8). Recent evidence suggests that CRC is a metabolic disease (9,10). Indeed, a multi-platform metabolomics system, used to predict biomarkers for chemotherapy efficacy of CRC, identified the serum level of octanoic acid as a useful biomarker (11). Furthermore, ultrahigh-performance liquid chromatography-mass spectrometry (UHPLC-MS) metabolomics and 16S microbiome analyses of 224 fecal samples from patients with advanced adenomas and CRC, identified cholesteryl esters and sphingolipids as potential early biomarkers in CRC. In addition, the actinobacterium, Adlercreutzia, was shown to be more abundant in advanced adenomas (12,13). Thus, the use of metabolic markers in combination with microbiome metagenomics may provide a novel strategy for the early detection of CRC.
In this study, we exploited a patient-derived xenograft (PDX) model to analyze the serum metabolites of CRC-bearing mice using gas chromatography-mass spectrometry (GC-MS) (14). Integrating these metabolomic results with publicly available gene expression data (GSE106582), we identified a unique metabolic signature in CRC that was associated with amino acid uptake and metabolism. Our study provides the foundation to examine amino acid metabolism as a potential early biomarker in CRC. We present the following article in accordance with the ARRIVE reporting checklist (available at http://dx.doi.org/10.21037/tcr-20-3406).
Methods
Patients
This study was conducted in accordance with the Declaration of Helsinki (as revised in 2013), and was approved by the ethical committee of Zhejiang Cancer Hospital (ethics approval number: IRB-2020-1). Informed consent was obtained from all the patients. Tumor samples were obtained from one patient undergoing surgical resection for adenocarcinoma of the rectum. Four tissue microarrays were collected from 97 CRC tissues of patients and 42 adjacent normal tissues.
Establishment of a PDX mouse model of CRC
Five-week-old male BALB/c nude mice (average body weight was 18±0.2 g) were purchased from Shanghai Slack Laboratory Animal Company (Shanghai, China). The mice were acclimatized for 1 week, and randomly assigned into control and PDX groups (10 mice in each group). Experiments were performed under a project license [SYXK(Zhe)2017-0012, No. 2019-02-010] granted by the Institutional Animal Care and Ethics Committee of Zhejiang Cancer Hospital, in compliance with national or institutional guidelines for the care and use of animals (15). All the animals were euthanized using isoflurane at the end of each experiment.
Fresh tumor samples were obtained from patients during the time of surgery at Zhejiang Cancer Hospital. The samples were immediately transferred into physiological saline and stored on ice. Tumors were sliced into 3×3×3 mm3 pieces and engrafted subcutaneously onto the flanks of BALB/c nude mice. Tumors were harvested for the PDX groups when they reached 300–500 mm3 (the tumor volumes were calculated as height × width2/2). The tumors were dissected and weighed (mean ± SD, Tables S1,S2) and the mice were euthanized using isoflurane. The presence of xenografts meant that the experimenter could not be blinded to whether the animal was in the control or PDX group. Blood was extracted retro-orbitally after isoflurane anesthesia. Samples were stored at room temperature for 30 min, then serum was extracted by centrifuging the samples for 10 min at 3,000 rpm, 4 °C. Serum samples were stored at –80 °C.
GC-MS-based metabolomic analysis
Frozen serum samples were thawed at room temperature, and GC/MS was carried out as described previously (16). The quality controls (QCs) were injected at regular intervals throughout the analytical run to provide a set of data from which repeatability could be assessed.
Statistics and metabolomic analysis
The converted data were imported into the MD-DIAL software for data processing (AnalysisBaseFileConverter software was used to convert the raw data). Metabolites were annotated through the LUG database (Untarget database of GC-MS from Lumingbio). Principal component analysis (PCA) and orthogonal partial least squares discriminant analysis (OPLS-DA) were performed using the SIMCA software package (14.0, Umea, Sweden) to visualize metabolic differences among experimental groups. Variable importance in the projection (VIP) ranks the overall contribution of each variable to the OPLS-DA model, and those variables with VIP >1 were considered relevant for group discrimination. The differential metabolites were selected based on the combination of a statistically significant threshold of VIP values obtained from the OPLS-DA model and p values from a two-tailed Student’s t-test on the normalized peak areas from different groups, where metabolites with VIP >1 and adjusted P value <0.05 were considered to be differential metabolites. The differential metabolites were analyzed by MetaboAnalyst 4.0 (https://www.metaboanalyst.ca/) (17) of the Joint Pathway Analysis section to examine the biologically meaningful metabolic patterns.
Bioinformatic analysis
Seventy-seven CRC and 117 mucosa tissue chip data (GSE106582) were used to screen the differentially expressed genes. Student’s test was used to identify the differences between the two groups.
Screening of differentially expressed genes was processed by R limma (v.3.6.2). Samples with an adjusted P value <0.05 and FC ≥1.2 or FC ≤0.83 were considered to be differentially expressed genes. The integration of microarray data and metabolites was performed by MetaboAnalyst 4.0 of the Joint Pathway Analysis section with Hypergeometric Test algorithms.
Immunohistochemistry
Slides were baked in an oven at 70 °C for 40 min, then deparaffinized in xylene for 10 min and hydrated with 100%, 100%, 95%, and 75% ethanol for 3 min each (v/v). Antigen retrieval was performed in citrate buffer by heating in a microwave for 10 min followed by drop cooling to 45 °C. After washing in water for 5 min, slides were placed in a 3% hydrogen peroxide solution for 10 min to inhibit endogenous peroxidase activity. Samples were washed with distilled water followed by 0.1% Tween (10 min), then incubated with rabbit anti-human SLC1A5 and SLC7A5 antibody (diluted 1:400, Cell Signaling Technology company; 1:500, Abcam company) overnight at 4 °C. Slides were incubated with a universal secondary antibody for 30 min each, followed by washing with 0.1% Tween. Reactions were developed using DAB and counterstained with hematoxylin for 2 min. After washing, samples were stained with blue promoting liquid for 2 min followed by incubation with 75%, 95%, 100%, and 100% ethanol for 3 min each, and xylene for 5 min twice, then sealed with neutral gum. Each of the tissues was scored. The immunohistochemical expression was assessed using light microscopy (magnification, ×100). The percentage of SLC1A5 and SLC7A5 positivity was scored from 0 to 4 as follows: 0 (positive range was <5%); 1 (positive range was 6–25%); 2 (positive range was 26–50%), 3 (positive range was 51–75%), and 4 (positive range was >75%). The staining intensity was scored according to a 4-point scale as follows: 0 (no staining); 1 (weak staining, light yellow); 2 (moderate staining, yellowish-brown); and 3 (strong staining, brown). Subsequently, SLC1A5 and SLC7A5 expression were calculated by multiplication of the percentage positivity score and staining intensity score, resulting in a final score ranging from 0 to 12.
Results
PDX mouse model maintains the characteristics of CRC
At the experimental endpoint, the weight of the mice and isolated tumors were assessed (Tables S1,S2). H&E staining showed that the histological characterization of the patient and PDX-derived tumors were all colorectal adenocarcinoma (Figure 1).
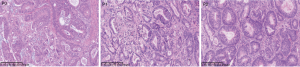
Differential expression of serum metabolites between the PDX and control groups
QC samples were clustered closely in the PCA score plot, indicating that the metabolomic analysis was stable and repeatable (Figure 2A). OPLS-DA was conducted to better understand intergroup differences (Figure 2B). The parameters of R2Y(cum) =0.995 and Q2(cum) =0.867 indicated that the classification was good for prediction. A permutation test (n=200) was used to validate the model and avoid overfitting. The R2 and Q2 were 0.901 and –0.462, respectively (Figure 2C). Differentially expressed metabolites were presented in a volcano plot. In total, 130 differentially expressed metabolites were found. The identified metabolites with the VIP of the first principal component of OPLS-DA exceeding 1.0, the adjusted P-value less than 0.05 and FC ≥1.2 or FC ≤0.83 were considered to be differential metabolites. In total, there were 67 differential metabolites between the PDX and control groups. Of these, 47 metabolites were significantly decreased in the PDX group (Figure 2D), including organic acids such as isocitric acid and enolpyruvate, and amino acids such as phenylalanine and D-aspartic acid. Non-endogenous metabolites were excluded from the study (Table 1).
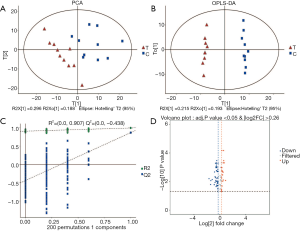
Table 1
No. | Name | VIP | Adj. P value | FC | Expressing trends |
---|---|---|---|---|---|
1 | Isocitric acid | 1.1020 | 0.0084 | 1.8511 | Upregulated |
2 | 2-hydroxypentanoic acid | 1.7756 | 0.0003 | 1.5165 | Upregulated |
3 | N-acetylornithine | 1.8574 | 0.0001 | 1.4909 | Upregulated |
4 | Glutamine | 1.3846 | 0.0095 | 1.3616 | Upregulated |
5 | Trehalose-6-phosphate | 1.4970 | 0.0034 | 1.3413 | Upregulated |
6 | Uridine 5’-monophosphate | 1.4394 | 0.0034 | 1.2815 | Upregulated |
7 | O-phosphoserine | 1.5299 | 0.0017 | 1.2539 | Upregulated |
8 | Glycine | 1.6753 | 0.0011 | 1.2482 | Upregulated |
9 | 5-methoxytryptamine | 1.8584 | 0.0001 | 1.2411 | Upregulated |
10 | Glycyl proline | 1.4859 | 0.0056 | 1.2134 | Upregulated |
11 | Indoxyl sulfate | 1.2116 | 0.0364 | 1.2025 | Upregulated |
12 | 2-monoolein | 1.4132 | 0.0104 | 0.8297 | Downregulated |
13 | Gamma-aminobutyric acid | 1.6109 | 0.0020 | 0.8290 | Downregulated |
14 | Guanosine | 1.2399 | 0.0304 | 0.8132 | Downregulated |
15 | Oxalic acid | 1.2676 | 0.0329 | 0.8116 | Downregulated |
16 | N-acetyl-d-mannosamine | 1.0815 | 0.0485 | 0.8053 | Downregulated |
17 | 3-hydroxybenzoic acid | 1.6178 | 0.0019 | 0.8043 | Downregulated |
18 | Saccharopine | 1.3948 | 0.0113 | 0.8034 | Downregulated |
19 | Erythritol | 1.6087 | 0.0019 | 0.7953 | Downregulated |
20 | Phenylalanine | 1.3433 | 0.0129 | 0.7925 | Downregulated |
21 | Aspartic acid | 1.4731 | 0.0121 | 0.7918 | Downregulated |
22 | Digalacturonic acid | 1.5938 | 0.0020 | 0.7878 | Downregulated |
23 | Homoserine | 1.4031 | 0.0093 | 0.7867 | Downregulated |
24 | 3-(3-hydroxyphenyl)-3-hydroxypropionic acid | 1.3003 | 0.0134 | 0.7588 | Downregulated |
25 | Uracil | 1.4230 | 0.0206 | 0.7520 | Downregulated |
26 | Enolpyruvate | 1.6525 | 0.0020 | 0.7467 | Downregulated |
27 | Thymine | 1.8096 | 0.0003 | 0.7190 | Downregulated |
28 | Udp-glucuronic acid | 1.7496 | 0.0009 | 0.7153 | Downregulated |
29 | N-carbamoylaspartate | 1.7602 | 0.0007 | 0.6940 | Downregulated |
30 | Glutaric acid | 1.2701 | 0.0225 | 0.6697 | Downregulated |
31 | Galactonic acid | 1.6475 | 0.0009 | 0.6581 | Downregulated |
32 | Gluconic acid | 1.8046 | 0.0005 | 0.6292 | Downregulated |
33 | Hexaric acid | 1.7207 | 0.0021 | 0.5690 | Downregulated |
34 | Tagatose | 1.4755 | 0.0165 | 0.5530 | Downregulated |
35 | Dodecanol | 1.6834 | 0.0073 | 0.4421 | Downregulated |
36 | 4a-carbinolamine tetrahydrobiopterin | 1.6895 | 0.0087 | 0.3818 | Downregulated |
Metabolite enrichment analysis
Of the 67 differential metabolites identified here, 36 had an FC ≥1.2 (11 metabolites) or FC ≤0.83 (25 metabolites) (Table 1; Figure 3A). These two groups of metabolites were selected for enrichment analysis to determine biologically meaningful metabolic patterns. Six metabolic pathways were identified, including (I) pyrimidine metabolism, (II) alanine, aspartate and glutamate metabolism, (III) arginine biosynthesis, (IV) aminoacyl-tRNA biosynthesis, (V) glyoxylate and dicarboxylate metabolism, and (VI) amino sugar and nucleotide sugar metabolism (Figure 3B).
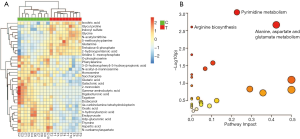
Integrative analysis of differentially expressed metabolites and genes
Microarray gene expression data (GSE106582) from 77 CRC and 117 mucosa tissues were used to screen the differentially expressed genes, and a total of 2,094 genes were selected (adjusted P value <0.05, FC ≥1.2 or FC ≤0.83). Then, we integrated the differentially expressed genes with the metabolites identified above using the Joint Pathway Analysis of MetaboAnalyst. We identified 74 enrichment pathways, 15 of which were significantly different (P<0.05) (Table 2). The enrichment pathways are as follows: (I) drug metabolism—other enzymes, (II) nitrogen metabolism, (III) glutathione metabolism, (IV) alanine, aspartate and glutamate metabolism, (V) glyoxylate and dicarboxylate metabolism, (VI) mucin type O-glycan biosynthesis, (VII) glycerolipid metabolism, (VIII) citric acid cycle (TCA cycle), (IX) phenylalanine, tyrosine and tryptophan biosynthesis, (X) purine metabolism, (XI) pentose and glucuronate interconversions, (XII) ascorbate and aldarate metabolism, (XIII) cysteine and methionine metabolism, (XIV) lysine degradation and (XV) phenylalanine metabolism (Figure 4).
Table 2
MetaboAnalyst pathway | Total | Hits | Raw P | Holm adjust | Impact |
---|---|---|---|---|---|
Drug metabolism—other enzymes | 70 | 18 | 0.0000 | 0.0033 | 0.3623 |
Nitrogen metabolism | 10 | 6 | 0.0001 | 0.0077 | 0.3333 |
Glutathione metabolism | 56 | 13 | 0.0014 | 0.1135 | 0.5091 |
Alanine, aspartate, and glutamate metabolism | 61 | 12 | 0.0088 | 0.7151 | 0.7000 |
Glyoxylate and dicarboxylate metabolism | 56 | 11 | 0.0121 | 0.9700 | 0.4727 |
Mucin type O-glycan biosynthesis | 22 | 6 | 0.0127 | 0.9996 | 0.6191 |
Glycerolipid metabolism | 35 | 8 | 0.0127 | 0.9996 | 0.6177 |
Citrate cycle (TCA cycle) | 42 | 9 | 0.0129 | 0.9996 | 1.0732 |
Phenylalanine, tyrosine and tryptophan biosynthesis | 11 | 4 | 0.0142 | 1.0000 | 2.0000 |
Purine metabolism | 166 | 24 | 0.0167 | 1.0000 | 0.7818 |
Pentose and glucuronate interconversions | 32 | 7 | 0.0245 | 1.0000 | 0.7742 |
Ascorbate and aldarate metabolism | 13 | 4 | 0.0266 | 1.0000 | 0.6667 |
Cysteine and methionine metabolism | 71 | 12 | 0.0282 | 1.0000 | 0.4857 |
Lysine degradation | 49 | 9 | 0.0336 | 1.0000 | 0.4375 |
Phenylalanine metabolism | 21 | 5 | 0.0393 | 1.0000 | 0.9500 |
Glycolysis or gluconeogenesis | 61 | 10 | 0.0518 | 1.0000 | 0.6000 |
Propanoate metabolism | 48 | 8 | 0.0718 | 1.0000 | 0.7021 |
Fructose and mannose metabolism | 40 | 7 | 0.0721 | 1.0000 | 0.4615 |
Sulfur metabolism | 18 | 4 | 0.0790 | 1.0000 | 0.3529 |
Arginine biosynthesis | 27 | 5 | 0.0993 | 1.0000 | 0.3077 |
beta-Alanine metabolism | 44 | 7 | 0.1084 | 1.0000 | 0.4651 |
Pyruvate metabolism | 45 | 7 | 0.1187 | 1.0000 | 0.7273 |
One carbon pool by folate | 31 | 5 | 0.1553 | 1.0000 | 0.9333 |
N-Glycan biosynthesis | 77 | 10 | 0.1734 | 1.0000 | 0.2763 |
Glycine, serine and threonine metabolism | 68 | 9 | 0.1758 | 1.0000 | 0.7164 |
Amino sugar and nucleotide sugar metabolism | 79 | 10 | 0.1942 | 1.0000 | 0.5897 |
Pyrimidine metabolism | 99 | 12 | 0.2047 | 1.0000 | 0.8163 |
Caffeine metabolism | 19 | 3 | 0.2570 | 1.0000 | 0.5556 |
Retinol metabolism | 47 | 6 | 0.2688 | 1.0000 | 0.3044 |
Pentose phosphate pathway | 47 | 6 | 0.2688 | 1.0000 | 0.9130 |
Butanoate metabolism | 29 | 4 | 0.2814 | 1.0000 | 0.4643 |
Ether lipid metabolism | 39 | 5 | 0.2945 | 1.0000 | 0.2632 |
Arginine and proline metabolism | 78 | 9 | 0.2979 | 1.0000 | 0.4416 |
Vitamin B6 metabolism | 21 | 3 | 0.3098 | 1.0000 | 1.1000 |
Neomycin, kanamycin and gentamicin biosynthesis | 4 | 1 | 0.3235 | 1.0000 | 0.6667 |
Folate biosynthesis | 61 | 7 | 0.3380 | 1.0000 | 0.2833 |
Histidine metabolism | 32 | 4 | 0.3470 | 1.0000 | 0.2258 |
Nicotinate and nicotinamide metabolism | 42 | 5 | 0.3517 | 1.0000 | 0.2683 |
Porphyrin and chlorophyll metabolism | 53 | 6 | 0.3708 | 1.0000 | 0.2500 |
Starch and sucrose metabolism | 43 | 5 | 0.3709 | 1.0000 | 0.4524 |
Tryptophan metabolism | 84 | 9 | 0.3795 | 1.0000 | 0.3615 |
Aminoacyl-tRNA biosynthesis | 74 | 8 | 0.3826 | 1.0000 | 0.1918 |
Valine, leucine and isoleucine degradation | 88 | 9 | 0.4350 | 1.0000 | 0.4483 |
Taurine and hypotaurine metabolism | 16 | 2 | 0.4468 | 1.0000 | 0.4000 |
Inositol phosphate metabolism | 69 | 7 | 0.4647 | 1.0000 | 0.3677 |
Galactose metabolism | 51 | 5 | 0.5217 | 1.0000 | 0.3600 |
Drug metabolism—cytochrome P450 | 98 | 9 | 0.5697 | 1.0000 | 0.1134 |
Riboflavin metabolism | 9 | 1 | 0.5854 | 1.0000 | 0.2500 |
Fatty acid degradation | 102 | 9 | 0.6198 | 1.0000 | 1.3663 |
D-Glutamine and D-glutamate metabolism | 10 | 1 | 0.6241 | 1.0000 | 0.1111 |
Arachidonic acid metabolism | 81 | 7 | 0.6404 | 1.0000 | 0.2750 |
Phosphatidylinositol signaling system | 74 | 6 | 0.7002 | 1.0000 | 0.4521 |
Glycerophospholipid metabolism | 86 | 7 | 0.7030 | 1.0000 | 0.3059 |
Fatty acid elongation | 75 | 6 | 0.7128 | 1.0000 | 0.6351 |
Thiamine metabolism | 14 | 1 | 0.7461 | 1.0000 | 0.1539 |
Steroid biosynthesis | 82 | 6 | 0.7903 | 1.0000 | 0.2222 |
Metabolism of xenobiotics by cytochrome P450 | 145 | 11 | 0.8090 | 1.0000 | 0.3264 |
Ubiquinone and other terpenoid-quinone biosynthesis | 17 | 1 | 0.8110 | 1.0000 | 0.2500 |
Glycosphingolipid biosynthesis—ganglio series | 47 | 3 | 0.8280 | 1.0000 | 0.4348 |
Glycosaminoglycan biosynthesis—chondroitin sulfate/ dermatan sulfate |
18 | 1 | 0.8287 | 1.0000 | 0.1177 |
Pantothenate and CoA biosynthesis | 34 | 2 | 0.8397 | 1.0000 | 0.0909 |
Terpenoid backbone biosynthesis | 36 | 2 | 0.8624 | 1.0000 | 0.1714 |
alpha-Linolenic acid metabolism | 22 | 1 | 0.8845 | 1.0000 | 0.2857 |
Primary bile acid biosynthesis | 92 | 5 | 0.9408 | 1.0000 | 0.4176 |
Mannose type O-glycan biosynthesis | 30 | 1 | 0.9476 | 1.0000 | 0.0690 |
Glycosphingolipid biosynthesis—globo and isoglobo series | 31 | 1 | 0.9525 | 1.0000 | 0.1333 |
Glycosylphosphatidylinositol (GPI)-anchor biosynthesis | 31 | 1 | 0.9525 | 1.0000 | 0.2000 |
Selenocompound metabolism | 35 | 1 | 0.9680 | 1.0000 | 0.0588 |
Various types of N-glycan biosynthesis | 36 | 1 | 0.9711 | 1.0000 | 0.2857 |
Glycosaminoglycan degradation | 44 | 1 | 0.9869 | 1.0000 | 0.1395 |
Tyrosine metabolism | 88 | 3 | 0.9915 | 1.0000 | 0.2299 |
Glycosphingolipid biosynthesis—lacto and neolacto series | 121 | 4 | 0.9975 | 1.0000 | 0.3333 |
Fatty acid biosynthesis | 129 | 2 | 1.0000 | 1.0000 | 0.0469 |
Steroid hormone biosynthesis | 199 | 5 | 1.0000 | 1.0000 | 0.2121 |
SLC1A5 and SLC7A5 are upregulated in CRC
Since amino acid metabolism was identified as a differential pathway, we next examined the expression patterns of the amino acid transporters, SLC1A5 and SLC7A5, in CRC. Tissue samples collected from 97 CRC patients and 42 adjacent normal tissues were stained with antibodies against SLC1A5 and SLC7A5. In the adjacent normal tissues, the cytoplasmic membrane staining score of SLC7A5 and SLC1A5 was 0~3+. SLC7A5 and SLC1A5 staining was observed in 31/95 and 85/94 CRC samples respectively (Table 3). Representative staining of control and CRC tissues is shown in Figure 5.
Table 3
Biomarkers | Adjacent normal tissues | Cancer tissue | |||||||
---|---|---|---|---|---|---|---|---|---|
0 | 1+ | 2+ | 3+ | 0 | 1+ | 2+ | 3+ | ||
SLC7A5 | 36/36 | 0/36 | 0/36 | 0/36 | 64/95 | 11/95 | 17/95 | 3/95 | |
SLC1A5 | 41/42 | 1/42 | 0/42 | 0/42 | 9/94 | 16/94 | 51/94 | 18/94 |
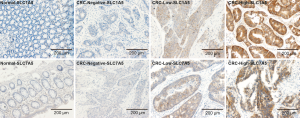
Discussion
In this study, GC-MS-based metabolomics analysis was used to determine specific metabolites in mice xenograft tumors in order to identify biomarkers for the early diagnosis and treatment of CRC. Significantly dysregulated serum metabolites included sugars, sugar alcohols, organic acids, amino acids, and fatty acids. Previously, Barberini et al. found that D-mannose and fructose were upregulated in the plasma of CRC patients compared with the control group, while methionine, valine, lysine, and proline were downregulated (18). Here, we observe similar trends in the amino acid levels in the serum of PDX-mice. In particular, we observed a consistent accumulation of isocitric acid, an upstream metabolite of the TCA cycle, consistent with Chen et al. (19). Our findings shown a reduction in mitochondrial metabolism, consistent with previous hypotheses suggesting that cancer is a type of mitochondrial metabolic disease (20). We also observed that many amino acids including phenylalanine and aspartic acid were downregulated in CRC tumor-bearing mice. This may indicate that CRC cells require high levels of amino acids to maintain metabolism. In addition, we found that L-methionine was decreased in CRC xenograft mice, which is consistent with previous studies that found that many human tumor cells are methionine-dependent (21-24).
Next, we integrated the differentially expressed metabolites with the gene expression data obtained from CRC and mucosal tissues. We found 2,094 differentially expressed genes involved in (I) drug metabolism—other enzymes, (II) nitrogen metabolism, (III) glutathione metabolism, (IV) alanine, aspartate and glutamate metabolism, (V) glyoxylate and dicarboxylate metabolism, (VI) mucin type O-glycan biosynthesis, (VII) glycerolipid metabolism, (VIII) TCA cycle, (IX) phenylalanine, tyrosine and tryptophan biosynthesis, (X) purine metabolism, (XI) pentose and glucuronate interconversions, (XII) ascorbate and aldarate metabolism, (XIII) cysteine and methionine metabolism, (XIV) lysine degradation, and (XV) phenylalanine metabolism. These genes may regulate unique pathways that promote abnormal proliferation of intestinal cells. Glutamine is one the most important amino acids in the metabolism and proliferation of cancer cells (25). Therefore, the glutamate metabolic pathway may be relevant for the development of future CRC biomarkers.
Interestingly, we found that the amino acid transporter, SLC7A5 (FC =3.8) was dramatically upregulated in CRC while changes in SLC1A5 (FC =1.4) were more modest. Rapid proliferation of cancer cells is highly dependent on nutrition and essential amino acids are vital for cellular proliferation (26,27). The uptake of amino acids across the cell membrane is predominantly controlled by membrane transporters (28). Amino acid transporters are membrane-bound proteins that mediate the transport of amino acids across membranes (29). The L-type amino acid transporter 1, LAT1 (solute carrier family 7 members 5, SLC7A5), which transports large neutral amino acids such as serine, cysteine, methionine, and glutamine, is reportedly overexpressed in many cancers (28). In addition, SLC7A5 has been shown to be required for intracellular L-glutamine efflux (30,31). In this study, an increase in serum L-glutamine levels was consistent with high SLC7A5 expression levels. Previous studies have shown that SLC7A5 upregulation is a general phenomenon in many cancers and may be a promising anticancer biomarker. Ogawa et al. (32) identified SLC7A5 as a marker linked to a worse prognosis in CRC-resected tumor specimens, while Cormerais et al. found that knockdown of SLC7A5 disrupted mTORC1 activity and inhibited tumor growth. The alanine-serine-cysteine transporter 2, ASCT2 (solute carrier family 1 member 5, SLC1A5), is a major transporter of the amino acid, glutamine (33). SLC1A5 plays an important role in glutamine transport and tumor growth (34). Previously, SLC1A5 siRNA was found to suppress proliferation and apoptosis in CRC and significantly promoted the inhibitory efficacy of cetuximab for CRC (35,36).
In summary, our study demonstrates that amino acid metabolism is the most dysregulated metabolic pathway in CRC. Amino acids are important for tumor growth. Expression of the amino acid transporters, SLC7A5 and SLC1A5, is increased in CRC, and could therefore be exploited as potential biomarkers for early diagnosis. Although promising, our preliminary findings may be limited by the small sample size, as well as our focus on only the highly upregulated and downregulated metabolites. Future studies addressing these issues may lead to the identification of additional metabolic biomarkers in CRC.
Conclusions
CRC is a common gastrointestinal tumor with a poor prognosis. Here, we successfully combined a GC-MS metabolomics analysis approach with a PDX model of CRC and identified a panel of potential diagnostic circulating metabolites. Dysregulated amino acid metabolism was found to be a significant feature of CRC, and the amino acid transporters, SLC7A5 and SLC1A5, were identified as potential metabolic therapeutic targets. Taken together, our study furthers the understanding of the dysregulated metabolic processes underlying CRC.
Acknowledgments
We thank Ruibing Jiang, Danying Wan and Yun Gao for H&E staining assistance, Junzhou Wu for data analysis assistance and Zhenying Guo for pathological score assistance. We thank International Science Editing (http://www.internationalscienceediting.com) for editing this manuscript.
Funding: This work (in vivo and in vitro experiments, data collection and analyses) was supported by
Footnote
Reporting Checklist: The authors have completed the ARRIVE reporting checklist. Available at http://dx.doi.org/10.21037/tcr-20-3406
Data Sharing Statement: Available at http://dx.doi.org/10.21037/tcr-20-3406
Peer Review File: Available at http://dx.doi.org/10.21037/tcr-20-3406
Conflicts of Interest: All authors have completed the ICMJE uniform disclosure form (available at http://dx.doi.org/10.21037/tcr-20-3406). The authors have no conflicts of interest to declare.
Ethical Statement: The authors are accountable for all aspects of the work in ensuring that questions related to the accuracy or integrity of any part of the work are appropriately investigated and resolved. The study was conducted in accordance with the Declaration of Helsinki (as revised in 2013). The study was approved by the ethical committee of Zhejiang Cancer Hospital (ethics approval number: IRB-2020-1), and informed consent was obtained from all the patients. Experiments were performed under a project license [SYXK (Zhe) 2017-0012, No. 2019-02-010] granted by the Institutional Animal Care and Ethics Committee of Zhejiang Cancer Hospital, in compliance with national or institutional guidelines for the care and use of animals.
Open Access Statement: This is an Open Access article distributed in accordance with the Creative Commons Attribution-NonCommercial-NoDerivs 4.0 International License (CC BY-NC-ND 4.0), which permits the non-commercial replication and distribution of the article with the strict proviso that no changes or edits are made and the original work is properly cited (including links to both the formal publication through the relevant DOI and the license). See: https://creativecommons.org/licenses/by-nc-nd/4.0/.
References
- Bray F, Ferlay J, Soerjomataram I, et al. Global cancer statistics 2018: GLOBOCAN estimates of incidence and mortality worldwide for 36 cancers in 185 countries. CA Cancer J Clin 2018;68:394-424. [Crossref] [PubMed]
- Schreuders EH, Ruco A, Rabeneck L, et al. Colorectal cancer screening: a global overview of existing programmes. Gut 2015;64:1637-49. [Crossref] [PubMed]
- Li JN, Yuan SY. Fecal occult blood test in colorectal cancer screening. J Dig Dis 2019;20:62-4. [Crossref] [PubMed]
- Tsang AH, Cheng KH, Wong AS, et al. Current and future molecular diagnostics in colorectal cancer and colorectal adenoma. World J Gastroenterol 2014;20:3847-57. [Crossref] [PubMed]
- Kim HJ, Yu MH, Kim H, et al. Noninvasive molecular biomarkers for the detection of colorectal cancer. BMB Rep 2008;41:685-92. [Crossref] [PubMed]
- Pakiet A, Kobiela J, Stepnowski P, et al. Changes in lipids composition and metabolism in colorectal cancer: a review. Lipids Health Dis 2019;18:29. [Crossref] [PubMed]
- Liu W, Li W, Liu H, et al. Xanthohumol inhibits colorectal cancer cells via downregulation of Hexokinases II-mediated glycolysis. Int J Biol Sci 2019;15:2497-508. [Crossref] [PubMed]
- Spratlin JL, Serkova NJ, Eckhardt SG. Clinical applications of metabolomics in oncology: a review. Clin Cancer Res 2009;15:431-40. [Crossref] [PubMed]
- Seyfried TN, Mukherjee P, Iyikesici MS, et al. Consideration of ketogenic metabolic therapy as a complementary or alternative approach for managing breast cancer. Front Nutr 2020;7:21. [Crossref] [PubMed]
- Seyfried TN, Flores RE, Poff AM, et al. Cancer as a metabolic disease: implications for novel therapeutics. Carcinogenesis 2014;35:515-27. [Crossref] [PubMed]
- Iemoto T, Nishiumi S, Kobayashi T, et al. Serum level of octanoic acid predicts the efficacy of chemotherapy for colorectal cancer. Oncol Lett 2019;17:831-42. [PubMed]
- Clos-Garcia M, Garcia K, Alonso C, et al. Integrative analysis of fecal metagenomics and metabolomics in colorectal cancer. Cancers (Basel) 2020;12:1142. [Crossref] [PubMed]
- Yuan F, Kim S, Yin X, et al. Integrating two-dimensional gas and liquid chromatography-mass spectrometry for untargeted colorectal cancer metabolomics: a proof-of-principle study. Metabolites 2020;10:343. [Crossref] [PubMed]
- Hidalgo M, Amant F, Biankin AV, et al. Patient-derived xenograft models: an emerging platform for translational cancer research. Cancer Discov 2014;4:998-1013. [Crossref] [PubMed]
- Kilkenny C, Browne WJ, Cuthill IC, et al. Improving bioscience research reporting: the ARRIVE guidelines for reporting animal research. PLoS Biol 2010;8:e1000412. [Crossref] [PubMed]
- Cheng W, Lu J, Lin W, et al. Effects of a galacto-oligosaccharide-rich diet on fecal microbiota and metabolite profiles in mice. Food Funct 2018;9:1612-20. [Crossref] [PubMed]
- Chong J, Wishart DS, Xia J. Using MetaboAnalyst 4.0 for comprehensive and integrative metabolomics data analysis. Curr Protoc Bioinformatics 2019;68:e86. [Crossref] [PubMed]
- Barberini L, Restivo A, Noto A, et al. A gas chromatography-mass spectrometry (GC-MS) metabolomic approach in human colorectal cancer (CRC): the emerging role of monosaccharides and amino acids. Ann Transl Med 2019;7:727. [Crossref] [PubMed]
- Chen KY, Liu X, Bu P, et al. A metabolic signature of colon cancer initiating cells. Annu Int Conf IEEE Eng Med Biol Soc 2014;2014:4759-62. [PubMed]
- Seyfried TN. Cancer as a mitochondrial metabolic disease. Front Cell Dev Biol 2015;3:43. [Crossref] [PubMed]
- Halpern BC, Clark BR, Hardy DN, et al. The effect of replacement of methionine by homocystine on survival of malignant and normal adult mammalian cells in culture. Proc Natl Acad Sci U S A 1974;71:1133-6. [Crossref] [PubMed]
- Thivat E, Farges MC, Bacin F, et al. Phase II trial of the association of a methionine-free diet with cystemustine therapy in melanoma and glioma. Anticancer Res 2009;29:5235-40. [PubMed]
- Breillout F, Antoine E, Poupon MF. Methionine dependency of malignant tumors: a possible approach for therapy. J Natl Cancer Inst 1990;82:1628-32. [Crossref] [PubMed]
- Hoffman RM. Clinical studies of methionine-restricted diets for cancer patients. Methods Mol Biol 2019;1866:95-105. [Crossref] [PubMed]
- Zhang J, Pavlova NN, Thompson CB. Cancer cell metabolism: the essential role of the nonessential amino acid, glutamine. EMBO J 2017;36:1302-15. [Crossref] [PubMed]
- Hosios AM, Hecht VC, Danai LV, et al. Amino acids rather than glucose account for the majority of cell mass in proliferating mammalian cells. Dev Cell 2016;36:540-9. [Crossref] [PubMed]
- Curi R, Lagranha CJ, Doi SQ, et al. Molecular mechanisms of glutamine action. J Cell Physiol 2005;204:392-401. [Crossref] [PubMed]
- Häfliger P, Charles RP. The L-type amino acid transporter LAT1-an emerging target in cancer. Int J Mol Sci 2019;20:2428. [Crossref] [PubMed]
- Kandasamy P, Gyimesi G, Kanai Y, et al. Amino acid transporters revisited: new views in health and disease. Trends Biochem Sci 2018;43:752-89. [Crossref] [PubMed]
- Chen R, Zou Y, Mao D, et al. The general amino acid control pathway regulates mTOR and autophagy during serum/glutamine starvation. J Cell Biol 2014;206:173-82. [Crossref] [PubMed]
- Pochini L, Scalise M, Galluccio M, et al. Membrane transporters for the special amino acid glutamine: structure/function relationships and relevance to human health. Front Chem 2014;2:61. [Crossref] [PubMed]
- Ogawa H, Kaira K, Motegi Y, et al. Role of amino acid transporter expression as a prognostic marker in patients with surgically resected colorectal cancer. Anticancer Res 2019;39:2535-43. [Crossref] [PubMed]
- Schulte ML, Fu A, Zhao P, et al. Pharmacological blockade of ASCT2-dependent glutamine transport leads to antitumor efficacy in preclinical models. Nat Med 2018;24:194-202. [Crossref] [PubMed]
- Cormerais Y, Massard PA, Vucetic M, et al. The glutamine transporter ASCT2 (SLC1A5) promotes tumor growth independently of the amino acid transporter LAT1 (SLC7A5). J Biol Chem 2018;293:2877-87. [Crossref] [PubMed]
- Huang F, Zhao Y, Zhao J, et al. Upregulated SLC1A5 promotes cell growth and survival in colorectal cancer. Int J Clin Exp Pathol 2014;7:6006-14. [PubMed]
- Ma H, Wu Z, Peng J, et al. Inhibition of SLC1A5 sensitizes colorectal cancer to cetuximab. Int J Cancer 2018;142:2578-88. [Crossref] [PubMed]